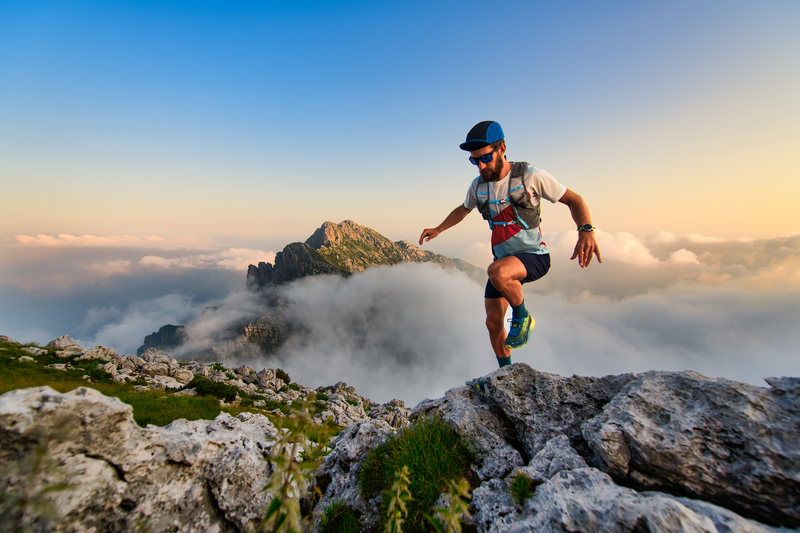
94% of researchers rate our articles as excellent or good
Learn more about the work of our research integrity team to safeguard the quality of each article we publish.
Find out more
ORIGINAL RESEARCH article
Front. Cell. Infect. Microbiol.
Sec. Clinical Infectious Diseases
Volume 15 - 2025 | doi: 10.3389/fcimb.2025.1466655
The final, formatted version of the article will be published soon.
You have multiple emails registered with Frontiers:
Please enter your email address:
If you already have an account, please login
You don't have a Frontiers account ? You can register here
Background: Although highly active antiretroviral therapy (HAART) has greatly enhanced the prognosis for people living with HIV (PLWH), some individuals fail to achieve adequate immune reconstitution, known as immunological nonresponse (INR), which is linked to poor prognosis and higher mortality. However, the early prediction and intervention of INR remains challenging in South China.Methods: This study included 1,577 PLWH who underwent at least two years of HAART and clinical follow-up between 2017 and 2022 at two major tertiary hospitals in South China. We utilized logistic multivariate regression to identify independent predictors of INR and employed restricted cubic splines (RCS) for nonlinear analysis. We also developed several machine-learning models, assessing their performance using internal and external datasets to generate receiver operating characteristic (ROC) curves, calibration curves, and decision curve analysis (DCA). The best-performing model was further interpreted using Shapley additive explanations (SHAP) values.Results: Independent predictors of INR included baseline, 6-month and 12-month CD4+ T cell counts, baseline hemoglobin, and 6-month hemoglobin levels. RCS analysis highlighted significant nonlinear relationships between baseline CD4+ T cells, 12-month CD4+ T cells and baseline hemoglobin with INR. The Random Forest model demonstrated superior predictive accuracy, with ROC areas of 0.866, 0.943, and 0.897 across the datasets. Calibration was robust, with Brier scores of 0.136, 0.102, and 0.126. SHAP values indicated that early CD4+T cell counts and CD4/CD8 ratio were crucial in predicting INR.Conclusions: This study introduces the random forest model to predict incomplete immune reconstitution in PLWH, which can significantly assist clinicians in the early prediction and intervention of INR among PLWH.
Keywords: hiv/aids, CD4+ T cell counts, Highly Active Antiretroviral Therapy, Immune reconstitution, Immunological Nonresponse
Received: 18 Jul 2024; Accepted: 21 Feb 2025.
Copyright: Ā© 2025 Chen, Zhang, Mao, Qian, Jiang, Gao, Tao, Liang, Peng and Cai. This is an open-access article distributed under the terms of the Creative Commons Attribution License (CC BY). The use, distribution or reproduction in other forums is permitted, provided the original author(s) or licensor are credited and that the original publication in this journal is cited, in accordance with accepted academic practice. No use, distribution or reproduction is permitted which does not comply with these terms.
* Correspondence:
Jie Peng, Nanfang Hospital, Southern Medical University, Guangzhou, China
Disclaimer: All claims expressed in this article are solely those of the authors and do not necessarily represent those of their affiliated organizations, or those of the publisher, the editors and the reviewers. Any product that may be evaluated in this article or claim that may be made by its manufacturer is not guaranteed or endorsed by the publisher.
Research integrity at Frontiers
Learn more about the work of our research integrity team to safeguard the quality of each article we publish.