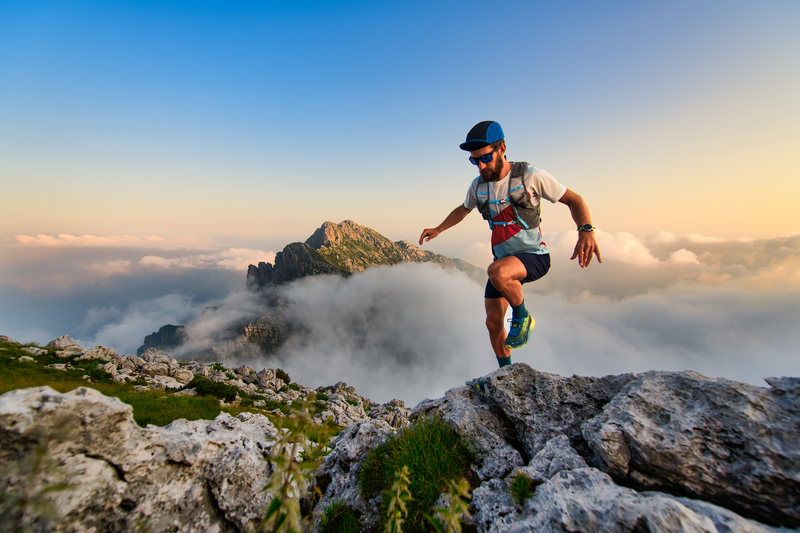
94% of researchers rate our articles as excellent or good
Learn more about the work of our research integrity team to safeguard the quality of each article we publish.
Find out more
EDITORIAL article
Front. Cell. Infect. Microbiol. , 26 August 2024
Sec. Virus and Host
Volume 14 - 2024 | https://doi.org/10.3389/fcimb.2024.1474097
This article is part of the Research Topic Exploring Genetic Characteristics and Molecular Mechanisms of Host Adaptation of Viruses with Artificial Intelligence (AI) or (and) Biological (BIO) Approaches View all 6 articles
Editorial on the Research Topic
Exploring genetic characteristics and molecular mechanisms of host adaptation of viruses with artificial intelligence (AI) or (and) biological (BIO) approaches
Most types of viruses cause infection and transmission in a limited range of hosts, indicating viral species tropism or host adaptation. Such host adaptation by viruses manifests as various genetic characteristics-associated molecular mechanisms in both the virus and host. Viral glycoprotein cleavage by host protease repertoire exerts a role in the zoonotic potential and risk posed by influenza A virus and coronavirus (Heindl and Bottcher-Friebertshauser, 2023). Interactions between viral polymerase PB2 subunit and host ANP32A (Camacho-Zarco et al., 2020), between polymerase units (Li et al., 2011), or even between the untranslated region of polymerase gene and the polymerase (Sun et al., 2014) are also involved in viral host adaptation. Artificial intelligence (AI)-based approaches have revealed the importance of viral genomic dinucleotides or dinucleotide clusters (Li et al., 2022, 2020) or mutations in viral proteins (Nan et al., 2022; Serna et al., 2022) to the host adaptation of severe acute respiratory syndrome coronavirus 2 (SARS-CoV-2). In light of the high performance by attention mechanism to capture the contextual words or sentences in natural language processing (NLP) (Vaswani et al., 2017), more NLP and other AI approaches should promise to swiftly and accurately facilitate the identification adaptive mutations with epistatic/hypostatic interactions or other types of significant association. And such genomic contexts or mutations important to adaptation have also been validated by experimental biology (BIO) approaches (Bugatti et al., 2023; He et al., 2022; Lista et al., 2022; Mishra et al., 2022; Supasa et al., 2021). Particularly, high-throughput methods such as deep mutational scanning have identified highly important mutations underlying the molecular mechanisms of host adaptation of SARS-CoV-2 (Dadonaite et al., 2024; Frank et al., 2022; Starr et al., 2020). Several molecular mechanisms by which other viruses adapt to the host have been recognized by AI and/or BIO approaches. An in-depth mutational analysis has revealed the potential role of host APOBEC3 in human adaptation of monkeypox virus (MPXV) in ongoing microevolution (Isidro et al., 2022). GP-A82V mutation-mediated increase in infectivity was found to correlate with disease severity during the Ebola virus disease epidemic, suggesting that this mutation was related to the adaptation of the virus to the human host (Diehl et al., 2016). However, previous studies or methodologies with multiple biological or virological methods was time-consuming, sporadic, and lacked predictability. Moreover, the accumulated huge amount of BIO results has not been deep learned to uncover the molecular mechanism in more details underlining viral adaptation to host. AI approaches for exploring genetic characteristics and its associated molecular mechanisms underlying host adaptation of viruses would be beneficial.
Recent studies have explored the genome-adaptation association using AI and/or BIO approaches. A cohort study by Silva et al. (Analysis of associations between the TLR3 SNPs rs3775291 and rs3775290 and COVID-19 in a cohort of professionals of Belém-PA, Brazil) revealed an association of single nucleotide polymorphisms (rs3775291 and rs3775290) in toll-like receptor 3 (TLR3) with COVID-19 severity in a cohort of professionals in Belém-PA, Brazil, implying host restriction to the SARS-CoV-2 adaptation to humans. The involvement of TLR3 has also been implied in the SARS-CoV-2-induced senescence in human cells (Tripathi et al., 2021). A more complicated virus-host co-evolution or viral adaptation to host was indicated in a study by Wang et al. (Comprehensive characterization of ERV-K (HML-8) in the chimpanzee genome revealed less genomic activity than humans); the study comprehensively characterized an endogenous retrovirus (HML-8), which originate from ancestral germline infection, in chimpanzee and humans, revealing less activity of the chimpanzee genome compared to that of the human genome.
Meanwhile, adaptive viral genomes have been recognized in various types of viruses. A study by Rashid et al. (Characterization of HIV-1 CRF02_AG/A3/G unique recombinant forms identified among children in Larkana, Pakistan) indicated that highly mutated retroviruses of human immunodeficiency virus (HIV) recombined with each other to adapt to the host. The rapid mutation and adaptation of HIVs has been associated with molecular mechanisms such as the adaptation to human leukocyte antigen-associated immune pressures (Avila-Rios et al., 2019; Carlson et al., 2015; Kloverpris et al., 2015) or to specific immunity (Da, 2003). The host adaptation of DNA viruses has also been revealed, particularly with AI approaches. A linear adaptation of MPXV has been indicated by genomic composition-based machine learning approach (Zhang et al., 2024). Genome composition-based deep learning approaches have also predicted the oncogenic potential of human papillomaviruses in a study by Hao et al. (Genome composition-based deep learning predicts oncogenic potential of HPVs). The virus-host adaptation has also been recognizable in bacteriophages. A spontaneous tail tubular mutation was indicated to drive the host range expansion of Acinetobacter phage vB_Ab4_Hep4 in a study by He et al. (Host range expansion of Acinetobacter phage vB_Ab4_Hep4 driven by a spontaneous tail tubular mutation). Such kind of adaptation has also been validated experimentally through strong parallel adaptation, a repeated and independent evolution of similar genotypes/traits from a common ancestor (Burmeister et al., 2023).
Taken together, although virus-host co-evolution or viral adaptation to host has been uncovered in several studies, it is far from being recognized systematically, particularly, in the context of an association between genetic characteristics and molecular phenotypes (mechanisms). Progress in this area has been slow owing to the high complexity of virus-host interactions, which is difficult to elucidate with traditional analysis or experiments. However, this methodological bottleneck is expected to be overcome using AI and high-throughput BIO approaches. Therefore, it will be exciting to see how these approaches will propel this research field in the future by uncovering the genetic characteristics and molecular mechanisms of host adaptation of viruses.
JL: Conceptualization, Funding acquisition, Writing – original draft, Writing – review & editing. X-HL: Writing – original draft, Writing – review & editing. EE: Writing – review & editing. LH: Conceptualization, Writing – original draft, Writing – review & editing.
The authors declare that the research was conducted in the absence of any commercial or financial relationships that could be construed as a potential conflict of interest.
All claims expressed in this article are solely those of the authors and do not necessarily represent those of their affiliated organizations, or those of the publisher, the editors and the reviewers. Any product that may be evaluated in this article, or claim that may be made by its manufacturer, is not guaranteed or endorsed by the publisher.
Avila-Rios, S., Carlson, J. M., John, M., Mallal, S., Brumme, Z. L. (2019). Clinical and evolutionary consequences of HIV adaptation to HLA: Implications for vaccine and cure. Curr. Opin. HIV AIDS. 14, 194–204. doi: 10.1097/COH.0000000000000541
Bugatti, A., Filippini, F., Messali, S., Giovanetti, M., Ravelli, C., Zani, A., et al. (2023). The D405N mutation in the spike protein of SARS-CoV-2 omicron BA.5 inhibits Spike/Integrins interaction and viral infection of human lung microvascular endothelial cells. Viruses. 15. doi: 10.3390/v15020332
Burmeister, A. R., Tzintzun-Tapia, E., Roush, C., Mangal, I., Barahman, R., Bjornson, R. D., et al. (2023). Experimental evolution of the TolC-Receptor phage U136B functionally identifies a tail fiber protein involved in adsorption through strong parallel adaptation. Appl. Environ. Microbiol. 89, e7923. doi: 10.1128/aem.00079-23
Camacho-Zarco, A. R., Kalayil, S., Maurin, D., Salvi, N., Delaforge, E., Milles, S., et al. (2020). Molecular basis of host-adaptation interactions between influenza virus polymerase PB2 subunit and ANP32A. Nat. Commun. 11, 3656. doi: 10.1038/s41467-020-17407-x
Carlson, J. M., Le, A. Q., Shahid, A., Brumme, Z. L. (2015). HIV-1 adaptation to HLA: A window into virus-host immune interactions. Trends Microbiol. 23, 212–224. doi: 10.1016/j.tim.2014.12.008
Da, S. J. (2003). The evolutionary adaptation of HIV-1 to specific immunity. Curr. HIV Res. 1, 363–371. doi: 10.2174/1570162033485249
Dadonaite, B., Brown, J., McMahon, T. E., Farrell, A. G., Figgins, M. D., Asarnow, D., et al. (2024). Spike deep mutational scanning helps predict success of SARS-CoV-2 clades. Nature. 631, 617–626. doi: 10.1038/s41586-024-07636-1
Diehl, W. E., Lin, A. E., Grubaugh, N. D., Carvalho, L. M., Kim, K., Kyawe, P. P., et al. (2016). Ebola virus glycoprotein with increased infectivity dominated the 2013-2016 epidemic. Cell. 167, 1088–1098. doi: 10.1016/j.cell.2016.10.014
Frank, F., Keen, M. M., Rao, A., Bassit, L., Liu, X., Bowers, H. B., et al. (2022). Deep mutational scanning identifies SARS-CoV-2 Nucleocapsid escape mutations of currently available rapid antigen tests. Cell. 185, 3603–3616. doi: 10.1016/j.cell.2022.08.010
He, P., Liu, B., Gao, X., Yan, Q., Pei, R., Sun, J., et al. (2022). SARS-CoV-2 Delta and Omicron variants evade population antibody response by mutations in a single spike epitope. Nat. Microbiol. 7, 1635–1649. doi: 10.1038/s41564-022-01235-4
Heindl, M. R., Bottcher-Friebertshauser, E. (2023). The role of influenza-A virus and coronavirus viral glycoprotein cleavage in host adaptation. Curr. Opin. Virol. 58, 101303. doi: 10.1016/j.coviro.2023.101303
Isidro, J., Borges, V., Pinto, M., Sobral, D., Santos, J. D., Nunes, A., et al. (2022). Phylogenomic characterization and signs of microevolution in the 2022 multi-country outbreak of monkeypox virus. Nat. Med. 28, 1569–1572. doi: 10.1038/s41591-022-01907-y
Kloverpris, H. N., Leslie, A., Goulder, P. (2015). Role of HLA adaptation in HIV evolution. Front. Immunol. 6. doi: 10.3389/fimmu.2015.00665
Li, J., Li, Y., Hu, Y., Chang, G., Sun, W., Yang, Y., et al. (2011). PB1-mediated virulence attenuation of H5N1 influenza virus in mice is associated with PB2. J. Gen. Virol. 92, 1435–1444. doi: 10.1099/vir.0.030718-0
Li, J., Wu, Y., Zhang, S., Kang, X., Jiang, T. (2022). Deep learning based on biologically interpretable genome representation predicts two types of human adaptation of SARS-CoV-2 variants. Brief. Bioinform. 23(3), 1–13. doi: 10.1093/bib/bbac036
Li, J., Zhang, S., Li, B., Hu, Y., Kang, X., Wu, X., et al. (2020). Machine learning methods for predicting Human-Adaptive influenza a viruses based on viral nucleotide compositions. Mol. Biol. Evol. 37, 1224–1236. doi: 10.1093/molbev/msz276
Lista, M. J., Winstone, H., Wilson, H. D., Dyer, A., Pickering, S., Galao, R. P., et al. (2022). The P681H mutation in the spike glycoprotein of the alpha variant of SARS-CoV-2 escapes IFITM restriction and is necessary for type i interferon resistance. J. Virol. 96, e125022. doi: 10.1128/jvi.01250-22
Mishra, T., Dalavi, R., Joshi, G., Kumar, A., Pandey, P., Shukla, S., et al. (2022). SARS-CoV-2 spike E156G/Delta157-158 mutations contribute to increased infectivity and immune escape. Life Sci. Alliance. 5. doi: 10.26508/lsa.202201415
Nan, B. G., Zhang, S., Li, Y. C., Kang, X. P., Chen, Y. H., Li, L., et al. (2022). Convolutional neural networks based on sequential spike predict the high human adaptation of SARS-CoV-2 omicron variants. Viruses. 14. doi: 10.3390/v14051072
Serna, G. G., Al, K. R., Invernici, F., Ceri, S., Bernasconi, A. (2022). CoVEffect: Interactive system for mining the effects of SARS-CoV-2 mutations and variants based on deep learning. Gigascience. 12. doi: 10.1093/gigascience/giad036
Starr, T. N., Greaney, A. J., Hilton, S. K., Ellis, D., Crawford, K., Dingens, A. S., et al. (2020). Deep mutational scanning of SARS-CoV-2 receptor binding domain reveals constraints on folding and ACE2 binding. Cell. 182, 1295–1310. doi: 10.1016/j.cell.2020.08.012
Sun, W., Li, J., Han, P., Yang, Y., Kang, X., Li, Y., et al. (2014). U4 at the 3’ UTR of PB1 segment of H5N1 influenza virus promotes RNA polymerase activity and contributes to viral pathogenicity. PloS One 9, e93366. doi: 10.1371/journal.pone.0093366
Supasa, P., Zhou, D., Dejnirattisai, W., Liu, C., Mentzer, A. J., Ginn, H. M., et al. (2021). Reduced neutralization of SARS-CoV-2 B.1.1.7 variant by convalescent and vaccine sera. Cell. 184, 2201–2211. doi: 10.1016/j.cell.2021.02.033
Tripathi, U., Nchioua, R., Prata, L., Zhu, Y., Gerdes, E., Giorgadze, N., et al. (2021). SARS-CoV-2 causes senescence in human cells and exacerbates the senescence-associated secretory phenotype through TLR-3. Aging (Albany NY). 13, 21838–21854. doi: 10.18632/aging.203560
Vaswani, A., Shazeer, N., Parmar, N., Uszkoreit, J., Jones, L., Gomez, A. N., et al. (2017). “Attention is all you need,” in Proceedings of the 31st International Conference on Neural Information Processing Systems (Curran Associates Inc, Long Beach, California, USA).
Keywords: host adaptation, genetic characteristics, molecular mechanisms, virus, artificial intelligence, biological approach
Citation: Li J, Li X-H, Ebrahimie E and Huang L (2024) Editorial: Exploring genetic characteristics and molecular mechanisms of host adaptation of viruses with artificial intelligence (AI) or (and) biological (BIO) approaches. Front. Cell. Infect. Microbiol. 14:1474097. doi: 10.3389/fcimb.2024.1474097
Received: 01 August 2024; Accepted: 14 August 2024;
Published: 26 August 2024.
Edited and Reviewed by:
Curtis Brandt, University of Wisconsin-Madison, United StatesCopyright © 2024 Li, Li, Ebrahimie and Huang. This is an open-access article distributed under the terms of the Creative Commons Attribution License (CC BY). The use, distribution or reproduction in other forums is permitted, provided the original author(s) and the copyright owner(s) are credited and that the original publication in this journal is cited, in accordance with accepted academic practice. No use, distribution or reproduction is permitted which does not comply with these terms.
*Correspondence: Jing Li, bGotcGJzQDE2My5jb20=; Lei Huang, aHVhbmdsZWl3YUBzaW5hLmNvbQ==
†These authors have contributed equally to this work
Disclaimer: All claims expressed in this article are solely those of the authors and do not necessarily represent those of their affiliated organizations, or those of the publisher, the editors and the reviewers. Any product that may be evaluated in this article or claim that may be made by its manufacturer is not guaranteed or endorsed by the publisher.
Research integrity at Frontiers
Learn more about the work of our research integrity team to safeguard the quality of each article we publish.