- 1Department of Genetics and Bioinformatics, Dasman Diabetes Institute, Kuwait City, Kuwait
- 2Department of Population Health, Dasman Diabetes Institute, Kuwait City, Kuwait
- 3Department of Pediatrics, Farwaniya Hospital, Ministry of Health, Farwaniya, Kuwait
- 4Department of Paediatrics, Sabah Hospital, Ministry of Health, Kuwait City, Kuwait
- 5Department of Paediatrics, Adan Hospital, Ministry of Health, Ahmadi, Kuwait
Background: Multisystem inflammatory syndrome in children (MIS-C) is a severe complication arising from SARS-CoV-2 infection, with indications that rare inborn errors of immunity may play a role in its pathogenesis. Recent studies suggest that genetic predispositions, particularly monogenic forms, could significantly influence the immune responses to SARS-CoV-2 in MIS-C.
Methods: We analysed 24 children under 12 years old, all of whom met the criteria provided by the World Health Organization, 2020 for MIS-C diagnosis, from the Paediatric COVID-19 Registry in Kuwait (PCR-Q8). Demographic and clinical data were collected from medical records, and exome sequencing was performed on the children and their parents to identify rare exonic variants. These variants were prioritized using two approaches: a candidate genes approach employing trio segregation analysis, and a candidate variants approach using a gene panel informed by previous studies on MIS-C-related genetic variants and datasets of differentially expressed genes in MIS-C patients.
Results: The candidate genes approach identified 53 unique genes in 20 of the 24 probands, including DDX60 and TMEM154, which were also differentially expressed between MIS-C and control groups. The candidate variants approach identified 33 rare, predicted deleterious heterozygous variants across 19 unique genes in 19 of the 24 probands, including both previously described and novel candidate variants for MIS-C. Pathway analysis of the identified genes from both approaches revealed significant involvement in immune response, viral defence, and inflammatory pathways.
Conclusion: This study underscores the monogenic susceptibility to MIS-C, enhancing the evidence base through comprehensive genetic analysis. The findings highlight the critical role of genetic predispositions in MIS-C and suggest that further functional genomics work is necessary to explore the mechanistic contributions of these genes, facilitating the development of targeted diagnostic strategies.
Introduction
Multisystem inflammatory syndrome in children (MIS-C) is a serious complication associated with current or recent SARS-CoV-2 infection or COVID-19 exposure, typically occurring weeks after the initial infection. MIS-C was first identified in April 2020 in regions with high COVID-19 rates across Europe, North, and South America (Whittaker et al., 2020; Verdoni et al., 2020; Toubiana et al., 2020; Riphagen et al., 2020; Jones et al., 2020; de Farias et al., 2020). A recent study reported MIS-C cases in the Middle East, specifically in the United Arab Emirates and Jordan (Abuhammour et al., 2022).
Population-based incidence estimates for MIS-C indicate approximately 316 cases per million SARS-CoV-2 infections (Payne et al., 2021). Incidence rates vary by ethnicity, race, and pandemic phases. A U.S. study reported higher incidence among Black, Hispanic or Latino, and Asian or Pacific Islander populations compared to whites. In Israel, MIS-C incidence per 100,000 persons under 18 years was 54.5 during the Alpha, 49.2 during the Delta, and 3.8 during the Omicron waves (Levy et al., 2022). In Cape Town, South Africa, the incidence was 22 per 100,000 exposures, with black children over-represented in MIS-C cases. In the U.S., MIS-C incidence after vaccination was as low as 1.0 case per million individuals receiving one or more doses of any COVID-19 vaccine in the age group with a median age of 16 years (Yousaf et al., 2022).
Epidemiological data suggest that SARS-CoV-2 infection triggers MIS-C onset, typically about one month post-infection. MIS-C is a heterogeneous condition resembling Kawasaki disease (KD), a clinically diverse paediatric inflammatory disorder (Verdoni et al., 2020). It has been proposed that rare inborn errors of immunity (IEIs) can modify the immune response to SARS-CoV-2, contributing to MIS-C pathogenesis in some children (Sancho-Shimizu et al., 2021). Discovering monogenic IEIs underlying MIS-C could provide insights into its pathogenesis. Chou et al. (2021) found monogenic susceptibility to inflammation in their MIS-C patient cohort. Investigations into rare recessive genetic variants have linked severe SARS-CoV-2 outcomes to defects in genes involved in type I interferon response (Zhang et al., 2020). The Middle Eastern study (Abuhammour et al., 2022) identified rare, likely deleterious heterozygous variants in immune-related genes, including TLR3, TLR6, IL22RA2, IFNB1, and IFNA6, in 19 of 45 MIS-C patients, with 7 patients carrying multiple variants.
Given the rarity of inflammatory disorders and the genetic predisposition observed in MIS-C patients (Chou et al., 2021), our study aims to further elucidate monogenic susceptibility to MIS-C. We analysed a cohort of family trios of MIS-C patients from Kuwait, focusing on familial segregation of autosomal recessive, X-linked recessive, and de novo mutations. Using exome sequencing, we identified ultra-rare and potentially damaging variants, highlighting novel candidate genes for MIS-C potentially unique to the Middle Eastern population. We employed stringent criteria and methodologies used by other research groups to prioritize variants in known MIS-C associated genes (Abuhammour et al., 2022; Vagrecha et al., 2022; Reis et al., 2023; Lee et al., 2023). By integrating these approaches, we aim to deepen our understanding of the genetic basis of MIS-C and contribute to the development of targeted therapeutic strategies.
Materials and methods
Study cohort
Sixty-seven children aged ≤ 12 years who met the MIS-C diagnosis criteria provided by the World Health Organization, 2020 (World Health Organization, 2020) were identified, during April 2020 and October 2021, from the national Paediatric COVID-19 Registry in Kuwait (PCR-Q8) (Qabazard et al., 2022). The criteria for diagnosis of MIS-C included age ≤12 years, fever ≥38.0°C, positive SARS-CoV-2 RT-PCR or positive antibody or positive antigen test. Multisystem involvement was shown by two or more categories involving (i) Kawasaki disease (KD) or toxic shock syndrome, (ii) rash (bilateral non-purulent conjunctivitis, or mucocutaneous inflammation signs), (iii) gastrointestinal involvement (abdominal pain, vomiting, or diarrhoea) and (iv) cardiovascular involvement (elevated troponin or Left Ventricular Ejection Fraction (LVEF) <55% or Coronary Artery (CA) dilation, aneurysm, or ectasia on echocardiogram).
Detailed demographic and clinical phenotype data were obtained from medical charts. Out of the 67 children initially diagnosed with MIS-C and included in the study, exome sequencing was performed on 24 children for whom DNA samples from both parents were available. This formed 24 trios, which were analysed as part of this study (Supplementary Table 1).
The study protocol was approved by the Ethical Review Committee at Dasman Diabetes Institute and the Ethical review Committee at the Ministry of Health of Kuwait and was in accordance with guidelines of the Declaration of Helsinki, and of the United States Federal Policy for the Protection of Human Subjects. Prior to participating in the study, every adult participant signed the informed consent form. As regards paediatric subjects, informed consent was obtained from the parents/legal guardians and assent was obtained from children aged 7 years or more.
DNA extraction
Blood samples were collected in ethylenediaminetetraacetic acid (EDTA)-treated tubes and genomic DNA was extracted using a QIAamp Blood DNA kit (Qiagen, Germany). A Qubit Fluorometer (Thermofisher, USA) was used to quantify spectrophotometry according to the manufacturer’s protocol. Absorbance values at 260–280 nm was checked for adherence to an optical density range of 1.8–2.1.
Exome sequencing
Exome sequencing of 72 individuals (24 trio families) was performed on the Illumina NovaSeq 6000 platform using Illumina DNA Prep with Enrichment kit (Illumina, CA, USA). Burrows wheeler aligner tool (Li and Durbin, 2009) was used to map short paired-ends to human Genome Reference Consortium Human Build 37 (GRCh37). Sequence Alignment Map tool (Li et al., 2009) was used to convert mapped data to compressed binary version (BAM) and PCR duplicate reads were marked using Picard software (http://picard.sourceforge.net). Variant calling was performed using the Genome Analysis Toolkit (GATK) (McKenna et al., 2010) on multiple Genomic Variant Call Format (GVCF) files generated for all samples after performing local realignments of reads that overlapped with insertion or deletion of bases (INDEL) as well as recalibration of base quality. Subsequently, a single Variant Calling Format file (VCF) was generated using GATK, which was later subjected to hard filtering parameters that include (“QD < 2.0”, “FS > 60.0”, “MQ < 40.0”, “MQRankSum < -12.5”, and “ReadPosRankSum < -8.0”) for single nucleotide variant (SNV) and (“QD < 2.0”,”FS > 200.0”, and “ReadPosRankSum < -20.0”) for INDEL. Variants that pass quality filtering were annotated and functionally categorized using ANNOVAR tool (Wang et al., 2010) version de74a7d59955d769c6cbb92a0d64d12c90c8eede.
Approach 1: MIS-C monogenic candidate genes prioritization workflow
We analysed the entire exome data (~20,000 genes) of MIS-C-afflicted children to identify genetic variants for further prioritization. High-quality variants were annotated for functionality using ANNOVAR (Wang et al., 2010). Only functional types of variants, such as missense, loss-of-function, stop-gain/loss, and splice-site mutations, were considered. These variants were then examined for global frequencies reported in ExAC (version hg19_r0.2), 1000 Genomes Project (phase1 release v3), and gnomAD exome (version 4.0) databases, retaining only ultra-rare variants with a minor allele frequency (MAF) of ≤0.01%.
To evaluate pathogenicity, we used the Combined Annotation Dependent Depletion (CADD) score via the Ensembl Variant Effect Predictor (VEP: McLaren et al., 2016), applying a Phred-like score cut-off of 20 (top 1% of deleterious variants in the human genome). In the absence of controls from siblings or extended family members, candidate variants were analysed for segregation within the family based on specific modes of inheritance, including autosomal recessive, X-linked recessive, and de novo.
This workflow employed stringent criteria to prioritize the most promising candidate genes for MIS-C, focusing on ultra-rare variants (MAF ≤0.01%) to capture potentially novel and pathogenic mutations. This rigorous filtering minimized false positives and ensured that only significant variants segregating according to expected inheritance patterns were considered.
Approach 2: MIS-C monogenic candidate variants prioritization workflow
We adopted a second prioritization workflow focused on a panel of 84 genes associated with MIS-C (Supplementary Table 2). This panel was compiled from published studies and consortium data, with gene selections based on genomic variants and differential gene expression studies linked to MIS-C (van der Made et al., 2020; Zhang et al., 2020; Lee et al., 2020; Chou et al., 2021; Solanich et al., 2021; Asano et al., 2021; Abolhassani et al., 2022; Abuhammour et al., 2022; Vagrecha et al., 2022; Reis et al., 2023; Lee et al., 2023).
This workflow prioritized rare exonic variants (MAF ≤1%) and potentially damaging mutations, adapting filtering criteria from Reis et al., 2023. Variants were further evaluated for pathogenicity using the American College of Medical Genetics and Genomics (ACMG) classification (Richards et al., 2015) via Varsome (http://varsome.com) and inheritance patterns obtained from Online Mendelian Inheritance in Man (OMIM: http://www.omim.org).
By adopting a curated gene panel and a more relaxed MAF threshold (≤1%), this approach ensured consistency with similar studies and focused on identifying rare, clinically relevant variants for MIS-C.
Examining the prioritized candidate genes and variants against the publicly available database on differentially expressed genes in MIS-C versus control individuals
Publicly-available data sets of 1,077 differentially expressed genes between individuals with SARS-CoV-2 associated MIS-C and controls (de Cevins et al., 2021), performed on all peripheral blood mononuclear cells (PBMCs) and on major cell types (monocytes/conventional dendritic cells (cDCs)/plasmacytoid dendritic cells (pDCs), T cells, and B cells), were used to further prioritise our candidate MIS-C genes and variants.
Integrated analysis of pathways, gene ontology, and protein-protein interactions for MIS-C candidate genes and variants
To elucidate the biological processes and pathways impacted by the prioritised candidate genes and candidate variants linked to MIS-C, we conducted several analyses. These included functional enrichment analysis, protein functional interaction network analysis covering protein-protein, protein-DNA-genetic interactions, and biological pathways, and tissue enrichment analysis. For the analysis of protein functional interaction networks associated with variant genes, we utilized GeneMania (Franz et al., 2018), an online platform. Gene ontology enrichment analysis was performed on genes showing a statistically significant increase in genetic load using WebGestalt (Zhang et al., 2005; Wang et al., 2013). Additionally, we carried out biological process enrichment analysis for variant-associated genes using tools such as DAVID (Shannon et al., 2003) and Enrichr (Kuleshov et al., 2016). Pathway enrichment analysis was conducted using KEGG, Reactome, and WikiPathways databases. The most statistically significant GO terms were visualized using the ggplot2 package in R. Cytoscape software was employed to build the co-expression network.
Results
Demographic and clinical characteristics of the MIS-C patients
A total of 24 patients, predominantly of Middle Eastern origin, from Kuwait, with MIS-C were prospectively recruited into the study cohort. The cohort comprised 18 males (75%) and 6 females (25%), with the majority being Arab, including 10 Kuwaiti, 2 Syrian, and 6 Egyptian patients. The remaining patients were of Asian descent, consisting of 4 Indian, 1 Sri Lankan, and 1 Afghan individuals. The mean age ± standard deviation of the participants was 6.35 ± 3.96 years. Most patients, 83.3% (20/24), had evidence of direct SARS-CoV-2 infection, as confirmed by serology or PCR testing.
Clinical manifestations across the cohort included gastrointestinal symptoms in 54.2% (13/24) of patients, skin rashes in 75% (18/24), and neurological complications in 12.5% (3/24) of the cases. Cardiac complications were noted, with 9.5% (2 out of 21 probands with available data) diagnosed with myocarditis, and 25% (6 out of 24) with coronary aneurysm/dilatation. Furthermore, 8.3% (2/24) of the cohort were diagnosed with classic Kawasaki disease, while a significant majority, 83.3% (20/24), were diagnosed with MIS-C or fulfilled the criteria of MIS-C diagnosis. Critical conditions necessitating intensive care were observed in 29.2% (7/24) of the patients. Parental consanguinity was documented in 16.7% (4/24) of cases. The comprehensive clinical characteristics and outcomes for these probands are detailed in Supplementary Table 1.
Results of variant calling using MIS-C monogenic candidate genes approach
In our exome sequencing study of 24 trio families, we identified a total of 115,182 exonic variants. Among these, 53,960 were non-synonymous, 49,804 were synonymous, 821 were stop-gain mutations, and 124 were stop-loss mutations. Additionally, 2,989 frameshift and non-frameshift mutations were identified, along with 497 splice-site variants. The remaining variants were either unknown or labelled as “.”.
In applying candidate genes prioritization workflow to the exonic variants, we successfully identified 55 variants from 53 genes from 20 of the 24 probands (Table 1). This set of prioritized variants encompassed 7 de novo, 27 X-linked recessive hemizygous, and 21 autosomal recessive homozygous mutations. 51 of these 55 variations were non-synonymous, two were stop-gain and two were splice-altering mutations. Interestingly, 15 probands were found to have multiple prioritized variants. However, a direct comparison of their phenotypic profiles revealed no distinct shared characteristics when compared to the nine probands that did not have multiple prioritized variants.
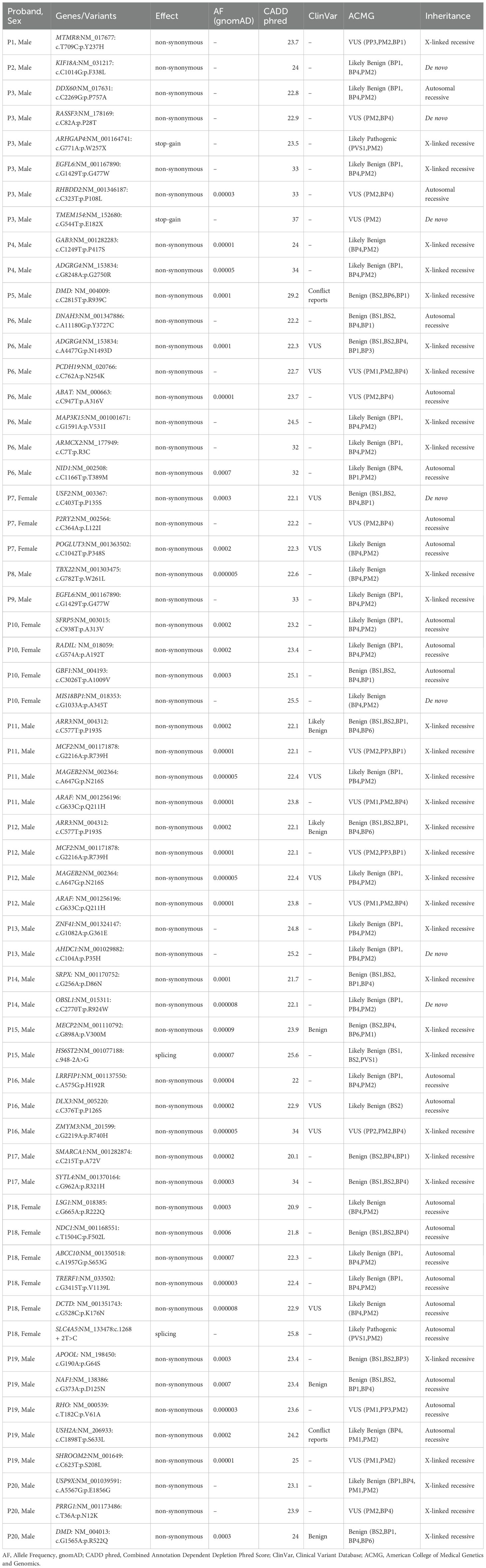
Table 1. Prioritised candidate genes for MIS-C by way of using exome data of the 24 families and using the candidate gene approach.
We annotated the above-mentioned 55 variants using the VEP tool against ClinVar entries (https://www.ncbi.nlm.nih.gov/clinvar/). This revealed that 13 of the 42 variants were reported in ClinVar database. Of those 13 variants, seven (namely ADGRG4:p.N1493D, DLX3:p.P126S, PCDH19:p.N254K, MAGEB2:p.N216S, USF2:p.P135S, RHO:p.V61A, and DCTD:p.K176N) were reported as ‘uncertain significance’, four (namely NAF1:p.D125N, MECP2:p.V300M, ARR3:p.P193S and DMD:p.R522Q) were reported as ‘benign or likely benign’, and the remaining two (namely USH2A:p.S633L and DMD:p.R939C) had conflicting pathogenicity reports. More than one variant from same gene were seen in two instances: ADGRG4:p.G2750R and ADGRG4:p.N1493D in P4 and P6, respectively, and DMD:p.R939C and DMD:p.R522Q in P5 and P20, respectively (see Table 1). Further, we noted a recurrence of specific X-linked recessive variants (namely EGFL6:p.G477W, ARAF:p.Q211H, ARR3:p.P193S, MAGEB2:p.N216S, and MCF2:p.R739H) in male probands.
Results of variant calling using MIS-C candidate variants approach
In a complementary approach focused specifically on MIS-C monogenic candidate genes, our targeted analysis of exome sequence data identified a total of 537 variants located within the 84-gene panel for MIS-C. Among these, 534 were exonic variants and 3 were splicing variants. The exonic variants included 204 nonsynonymous, 3 frameshift deletions, 2 nonframeshift deletions, 2 stopgain mutations, 3 stoploss mutations, and 235 synonymous. The remaining 85 variants were classified as unknown. Of these, 121 variants were deemed to have potential functional consequences based on their nonsynonymous, frameshift, splicing, or stop-loss/gain effects.
By way of applying the MIS-C candidate variants prioritization workflow to the identified variants, we prioritised 33 rare predicted deleterious heterozygous variants, spread across 19 unique genes. These 33 prioritised variants were seen in 19 of the 24 probands (Table 2).
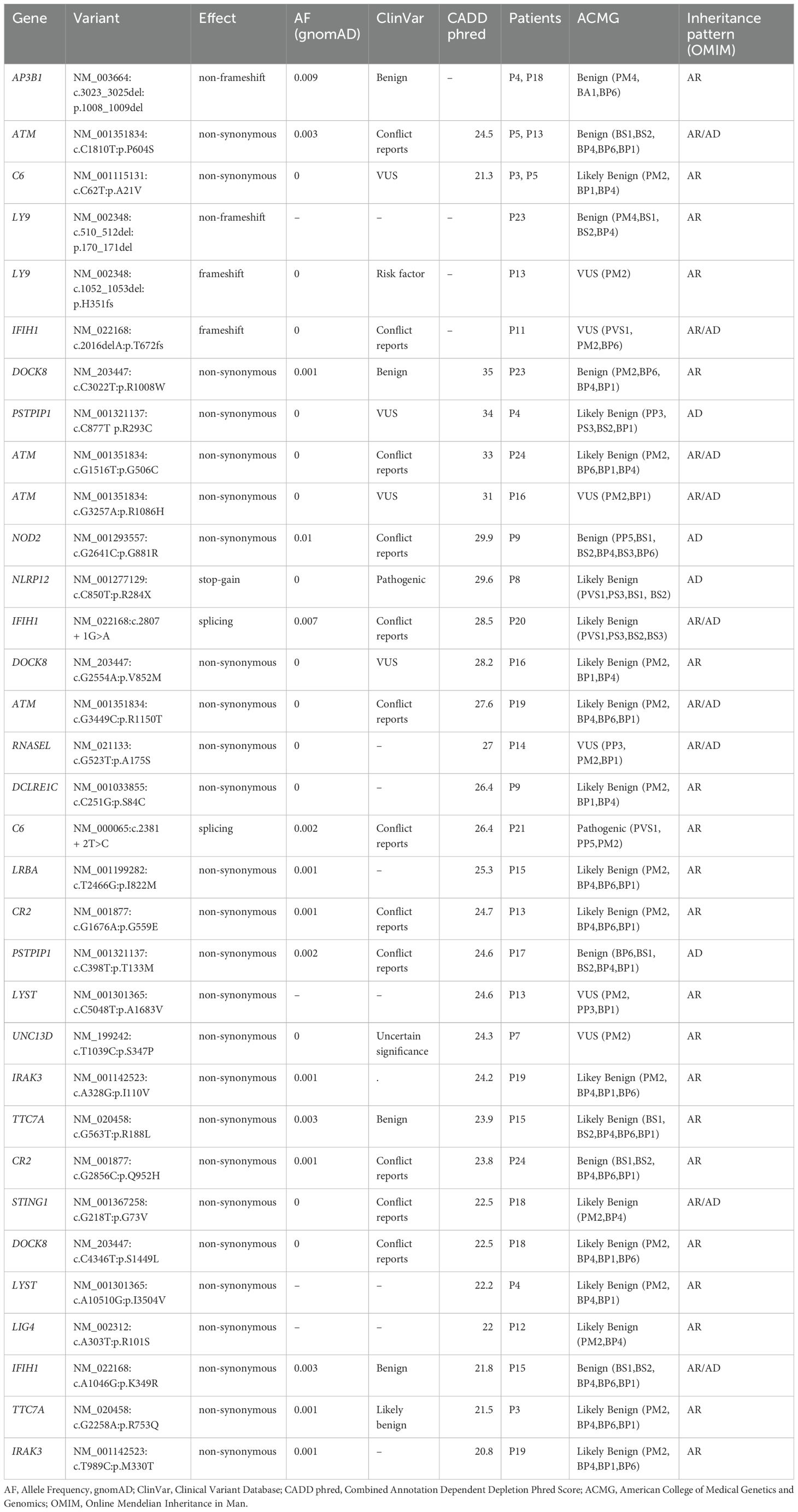
Table 2. Prioritised candidate variants for MIS-C using 24 probands exome data by way of using gene panel associated with MIS-C and applying the candidate variant approach.
We annotated the 33 variants prioritized through the gene panel approach using the VEP tool against the ClinVar database. This classified one variant, namely NLRP12:p.R284X, as ‘Pathogenic’ and another variant, namely LY9:p.H351fs, as ‘Risk factor ‘. We encountered 5 variants (from genes such as C6 and DOCK8) annotated as of ‘Uncertain significance’. Significantly, 26 of the 33 variants were not reported in ClinVar, highlighting the potential for reporting novel genetic associations with MIS-C. Using ACMG guidelines, one variant, namely C6:c.2381 + 2T>C, was classified as ‘Pathogenic’, six variants, namely LY9:p.H351fs, IFIH1:p.T672fs, ATM:p.R1086H, RNASEL:p.A175S, LYST:p.A1683V, and UNC13D:p.S347P, were classified as ‘Variants of Unknown Significance’ (VUS), and the remaining variants were classified as either ‘Benign’ or ‘Likely Benign’.
Furthermore, our findings underscore the recurrence of specific variants in multiple probands, potentially pinpointing causative candidate variants or delineating their relevance in the pathogenesis of MIS-C. For example, the ATM:p.P604S variant appeared in probands P5 and P13 and the C6:p.A21V variant appeared in probands P3 and P5. Additionally, the AP3B1 gene harbouring the p.1008_1009del variant was identified in probands P4 and P18. It is worth noting that the probands P5 and P13, sharing the ATM gene variant, as well as the P4 and P18 probands, sharing the AP3B1 gene variant, were presented with ‘Critical’ severity of MIS-C symptoms and some overlapping clinical features. This observation may suggest a contributory role for these genetic variants in determining the severity of MIS-C presentations.
Differential expression of MIS-C candidate genes and variants
The prioritised genes were examined against significant results from the study of de Cevins et al. (2021); this study examined differentially expressed genes in MIS-C (CoV-2+) afflicted children compared to control children in different cell types such as PBMCs (200 genes), Monocytes/cDCs/pDCs (653 genes), T cells (177 genes) and B cells (423 genes). Of the 1,077 unique differentially expressed genes analysed for overlap with prioritized monogenic candidate genes in the gene panel approach, three genes—DDX60, TMEM154, and RASSF3—were found to be common. Notably, all three genes were prioritized from proband P3 (Table 3). Additionally, five genes—LY9, IFIH1, DOCK8, DCLRE1C, and LYST—were identified as common among the prioritized monogenic candidate genes using the family trio approach, observed in nine patients (Table 3).
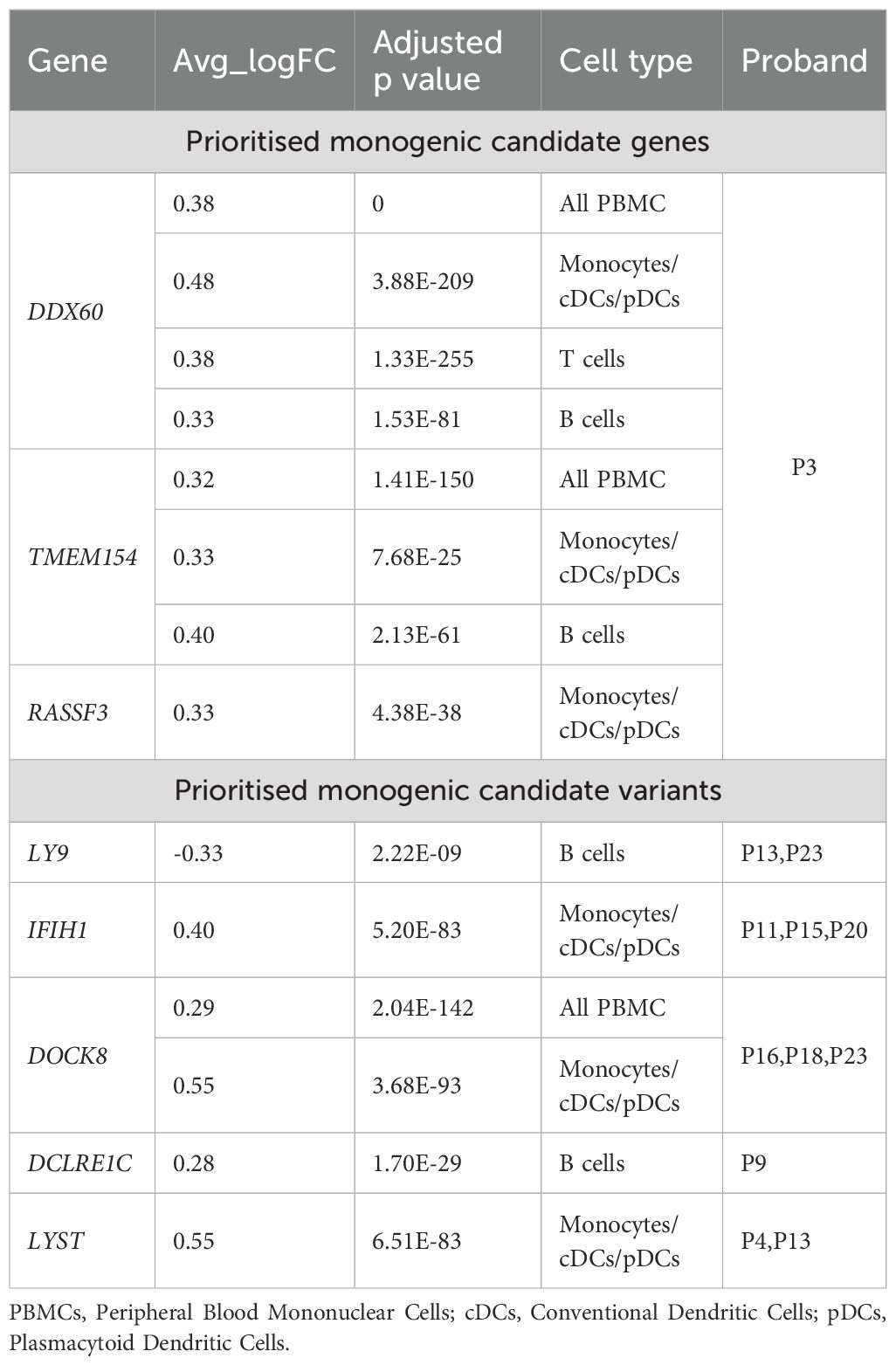
Table 3. Prioritised MIS-C genes common with differentially expressed genes in MIS-C (CoV-2+) afflicted children compared to control children in different cell types (de Cevins et al., 2021).
Analysis of functional enrichment, examination of pathways, and investigation of protein-protein interactions
Upon investigating the regulatory networks of the identified 53 unique genes from the candidate gene approach for MIS-C (as presented in Table 1) using Protein-protein Interaction (PPI) networks and protein-DNA-genetic interactions networks of genes constructed by GeneMANIA, we identified relationships between genes that could indicate possible disruptions in signalling pathways in MIS-C (Figure 1A). Functional enrichment analysis of these genes indicated that the candidate genes mainly enriched biological pathways, such as IFN production, Rho activation, Wnt, VEGF, EGFR signalling pathways, and visual perception, relevant to MIS-C symptoms (Figure 1B).
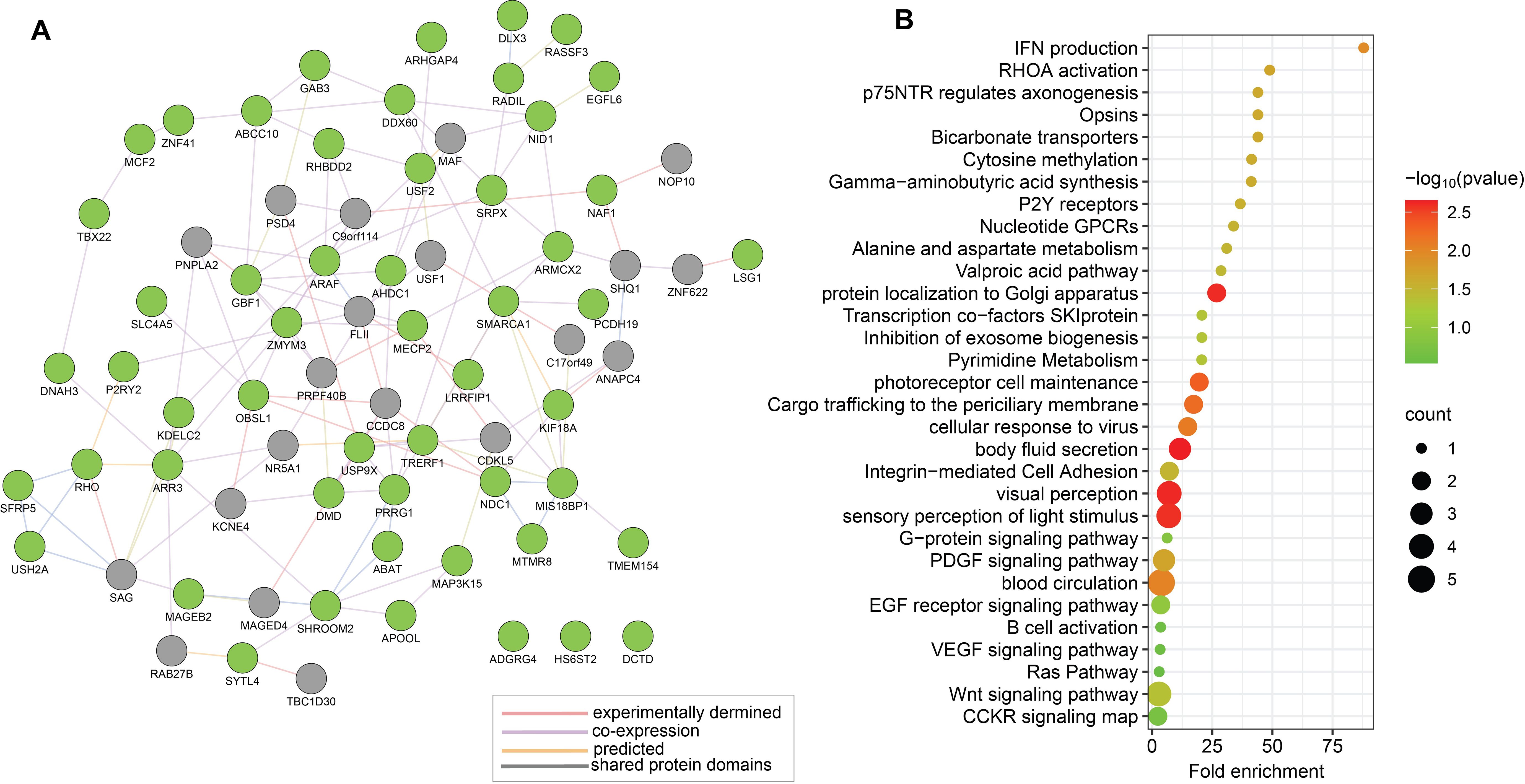
Figure 1. Functional relationships between the 53 genes prioritised by candidate genes approach (Approach 1) for MIS-C. (A) Schematic illustration of a PPI network based on experimental evidence and expert-curated databases by using GeneMANIA. The network features a subset of 53 candidate genes for MIS-C, represented as green nodes. Grey nodes represent genes that interact with the candidate genes, as supported by experimental evidence and database curation. (B) Representative results from enrichment analyses of the prioritized 53 candidate genes for MIS-C using gene ontology terms for biological process and KEGG pathways. Each identified category shows enrichment, including the -log10 of the statistical enrichment P-value from the DAVID database where maximum enrichment was observed, alongside the count of involved genes. The dot size reflects the number of genes enriched in each category.
Results of similar analyses on the 19 unique genes identified through the candidate variants approach (depicted in Table 2) are presented in Figure 2. PPI network of these 19 genes is presented in Figure 2A with the 19 genes shown as blue nodes, and their interacting genes (supported by experimental evidence and database curation) shown as grey nodes. Figure 2B illustrates the functional enrichment analysis that identifies relevant pathways, including viral response, immune response, inflammation, lymphocyte activation, interferon signalling, and the innate immune system.
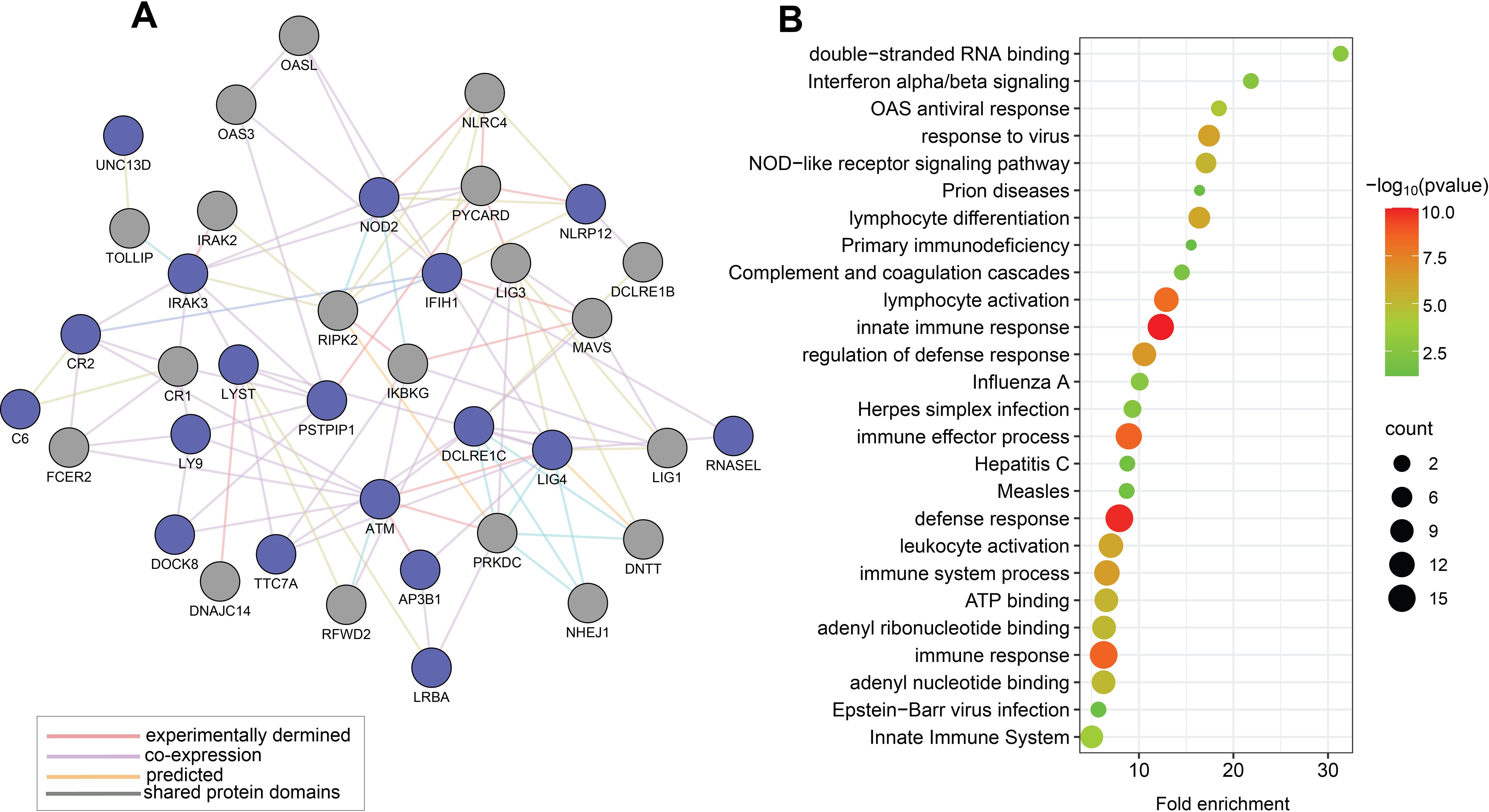
Figure 2. Functional relationships of the 19 genes prioritised by the candidate variants approach for MIS-C (Approach 2). (A) This map displays a PPI network of MIS-C-associated genes with prioritized rare candidate variants, represented by blue nodes, and their interacting genes derived from experimental data and expert-curated databases, depicted in grey nodes. (B) Representative outcomes from enrichment analyses on selected rare candidate variants in genes linked to MIS-C using gene ontology terms for biological processes and KEGG pathways. Each identified category was enriched, including the -log10 of the statistical enrichment P-value from the DAVID database where the enrichment and the respective gene counts were most notable. The dot size reflects the count of genes enriched in each category.
To better understand the potential interactions and collective impact of genes identified through the two prioritization approaches for MIS-C, we merged the genes from both analyses into a set of 72 unique genes. Using the GeneMANIA database, we constructed a PPI network consisting of 93 nodes interconnected by 333 edges (Figure 3A). This network highlighted significant connections between the genes identified by both the approaches, suggesting they share regulatory pathways relevant to the pathogenesis of MIS-C.
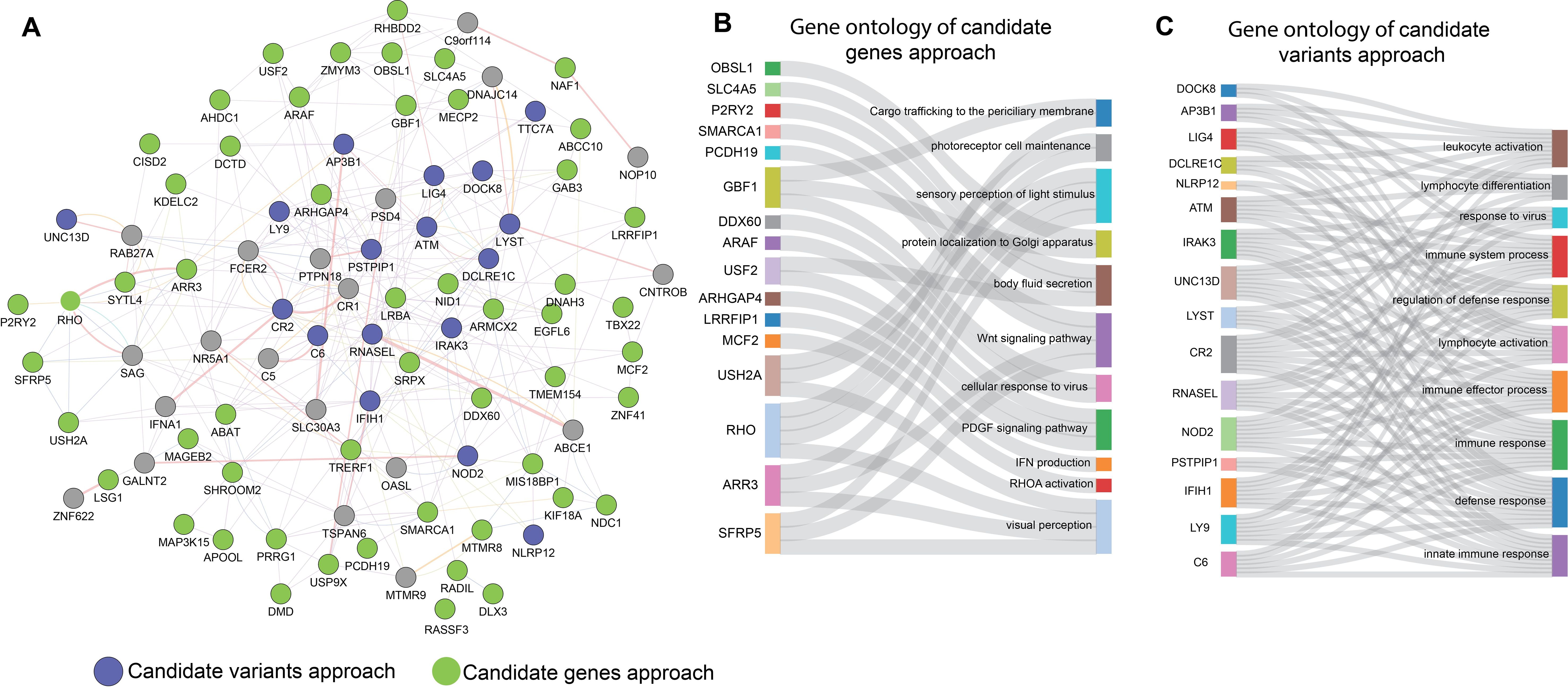
Figure 3. Functional relationships between the two prioritising approaches of candidate genes and candidate variants for MIS-C. (A) PPI Networks based on experimental evidence and expert-curated databases. Green node represents the genes with candidate variants identified in our study. Blue colour nodes represent the genes previously reported for MIS-C. (B, C) depict Sankey diagrams illustrating the functional relationships between the candidates for MIS-C; (B) the prioritised candidate genes approach and (C) the prioritised candidate variants approach via biological processes that enrich them according to GO functional enrichment analysis.
Further analysis was conducted individually on each set of prioritized genes, as depicted in the Sankey diagrams (Figures 3B, C). These diagrams demonstrate the functional and biological processes enriched in each approach, including immune response, inflammation, and response to viral pathogens. The overlap of these biological processes in both data sets confirms that both prioritization methods effectively capture the key aspects of the phenotype associated with MIS-C. This phenotype is typically triggered by a viral infection like COVID-19 and manifests as MIS-C. The consistency across both methods underscores their effectiveness in identifying genes that are crucial to the development of MIS-C.
While the 53 candidate genes prioritized for MIS-C by candidate gene approach (Approach 1) did not overlap with the established 84-gene panel for MIS-C, we attempted to explore the possibility of existence of potential indirect relationships via intermediary genes and to further assesses whether these intermediary genes are functionally relevant to the pathogenesis of MIS-C. This approach would enable us to consider the possibility that, despite the absence of direct gene overlap, interconnected pathways could still play a crucial role in disease mechanisms. To investigate this, we constructed extended PPI networks by way of including both the sets of genes (i.e. the 53 genes derived by the candidate gene approach (Approach 1) and the 84 genes from MIS-C gene panel), aiming to identify intermediary genes mediating secondary connections between the genes. This exercise resulted in 20 intermediary genes (Figure 4A). We conducted pathway enrichment analyses on these intermediary genes using the Gene Ontology and KEGG pathway databases (Figure 4B). Our findings indicated that several of these intermediary genes are significantly involved in biological pathways critical for immune response, inflammation, and cell signalling processes linked to MIS-C, such as interferon, TLR, NF-kappa beta, cytokine signalling pathway and responses to SARS-CoV infection. This suggests that while there is no direct overlap between the identified candidate genes and the MISC-gene panel, the regulatory networks involving intermediary genes may still significantly influence the pathogenesis of MIS-C.
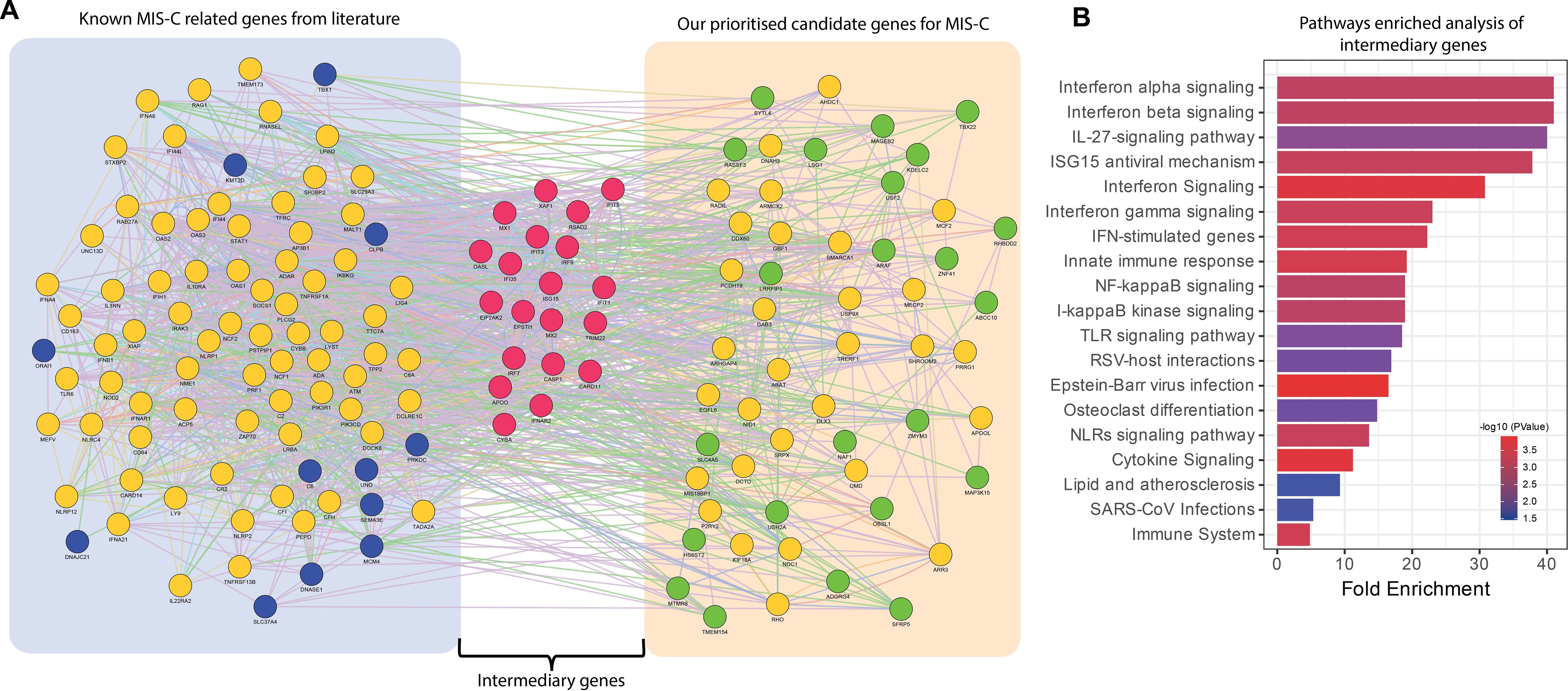
Figure 4. Functional relationships of intermediary genes forming connections between the known genes in MIS-C gene panel and the genes from the prioritized candidate gene approach. (A) PPI networks based on experimental evidence and expert-curated databases from GeneMANIA. The left cluster represents genes reported in the literature, known as the MIS-C gene panel (blue nodes). The right cluster shows the prioritized candidate genes identified in our study (green nodes). The middle cluster displays the intermediary genes (red nodes) bridging the two groups of gene sets. Yellow nodes represent genes that interact with both prioritized candidate genes and the MIS-C gene panel. (B) Bar plots showing the significant biological processes and pathways of intermediary genes. The x-axis represents fold enrichment, with significance indicated by order and colour trend (P < 0.05).
Discussion
In our study, we employed two distinct monogenic approaches to elucidate the genetic underpinnings of MIS-C in a predominantly Arab cohort, each proposed different assumptions about inheritance patterns and implications for disease expression.
In our investigation of candidate genes for MIS-C, we adopted a unique methodology by analysing family trios, hypothesizing that heterozygosity in parents could lead to homozygosity or de novo mutations in the probands. This approach enabled us to prioritize 53 unique genes, distinguishing our findings from those of broader genomic scans and studies involving control groups, such as those conducted by Chou et al. (2021); Abolhassani et al. (2022); Abuhammour et al. (2022); Gelzo et al., 2022; Santos-Rebouças et al. (2022), and Reis et al. (2023). While these studies provide valuable insights through comparisons across general populations or with COVID-19 positive control children, our targeted exploration within familial settings offers a complementary perspective, enhancing our understanding of the genetic architecture of MIS-C with greater precision.
Following this approach, our functional enrichment analysis and pathway interrogations highlighted key biological processes and pathways associated with these candidate genes (Figure 1). Notably, the identified pathways—such as those involved in IFN production, Rho activation, and EGFR signalling—align with a potential state of inflammation that may persist excessively, likely triggered by a COVID-19 infection. This excessive inflammatory response could potentially precipitate the onset of MIS-C, suggesting a critical role for these pathways in the disease’s pathogenesis.
In the candidate genes approach, we observed hemizygous variants in male probands, including recurrent ones such as EGFL6:p.G477W, ARAF:p.Q211H, ARR3:p.P193S, MAGEB2:p.N216S, and MCF2:p.R739H, alongside different variants in genes like DMD and ADGRG4, across multiple individuals. These findings suggest a genetic predisposition to MIS-C. Specifically, the EGFL6 gene is associated with obesity-related inflammation in children (Landgraf et al., 2022), and the DMD gene with muscle inflammation (Bez Batti Angulski et al., 2023). Variants in ARAF, ARR3, MAGEB2, and MCF2 were also found in the same two families presenting identical clinical symptoms. Except for the benign variants ARR3:p.P193S and DMD:p.R522Q, and the variant DMD:p.R939C with conflicting pathogenicity reports, the clinical significance of the other variants remains either uncertain or unreported in ClinVar database. While other prioritized genes from single families show no direct link to MIS-C, this variability could indicate the heterogeneity of this condition. DDX60 is involved in the antiviral immune response (Miyashita et al., 2011), and the TMEM154 gene is implicated in viral susceptibility in animals (Heaton et al., 2012). Importantly, both these two genes were observed to be differentially expressed between MIS-C and control children (de Cevins et al., 2021), suggesting their roles in immune regulation and inflammatory response to viral infections.
Our candidate variants approach for MIS-C aligns with pioneering efforts by other groups (Chou et al., 2021; Abolhassani et al., 2022; Abuhammour et al., 2022; Santos-Rebouças et al., 2022; Reis et al., 2023) by focusing on exome data from probands to delineate rare deleterious variants in a population which accommodate incomplete penetrance inheritance in immune and inflammation-related genes that is crucial for MIS-C development. This prioritised approach led to the identification of 33 rare exonic variants with predicted deleterious variants across 19 unique genes known to be associated with MIS-C.
The pathway enrichment analysis performed on the prioritized variants within the known MIS-C gene panel revealed significant involvement in pathways crucial for immune response, viral defence, and inflammation—all key components of MIS-C symptomatology (Figure 2). This analysis highlights the relevance of these genes and their pathways in the pathogenesis of MIS-C, thereby reinforcing the validity of the gene panel in identifying causative variants essential for accurate diagnostics.
Following the ACMG guidelines, we identified the splice site variant C6:c.2381 + 2T>C in the complement component 6 gene, classified as pathogenic, in one proband from our study cohort. The gene has been implicated in MIS-C development through its role in immune system regulation (Santos-Rebouças et al., 2022; Reis et al., 2023). Gelzo et al. (2022) described this variant in a male MIS-C patient, associating it with severe immune dysregulation, which highlights its contribution to the hyperinflammatory response observed in the syndrome. Another non-synonymous variant (p.A21V) in C6 gene was prioritised in two probands. However, it is annotated as ‘likely benign’ in ACMG classifications and ‘variant of unknown significance’ in ClinVar.
Moreover, we identified six variants with the annotation of ‘variant of unknown significance’ by ACMG guidelines in 6 genes: IFIH1, LYST, UNC13D, RNASEL, ATM and LY9. One of these, a frameshift variant IFIH1:p.T672fs, is involved in the induction of type 1 interferons and proinflammatory cytokines and a candidate gene for MIS-C (Abuhammour et al., 2022; Santos-Rebouças et al., 2022; Vagrecha et al., 2022; Reis et al., 2023). This variant was also described for MIS-C proband by another study (Abuhammour et al., 2022). Additionally, we identified two unreported variants in IFIH1 for MIS-C; a splice variant c.2807 + 1G>A that is classified as ‘likely benign’ in ACMG classifications and had conflicting reports in ClinVar, and a non-synonymous variant p.K349R that is classified as ‘benign’ in ACMG classifications and ClinVar annotations. Another non-synonymous variant of unknown significance in the LYST gene (p.A1683V), which is autosomal recessive monogenic gene for systemic inflammatory syndrome (Schulert et al., 2016), and a potential combination of heterozygous mutations in LYST in children with viral infection can lead to MIS-C (Vagrecha et al., 2022). We identified another non-synonymous variant in LYST:p.I3504V annotated as ‘likely benign’ in ACMG classifications. Another systemic inflammatory response syndrome gene (Song et al., 2022) and candidate gene for MIS-C (Vagrecha et al., 2022) is UNC13D, from which we prioritised a non-synonymous variant, namely p.S347P, annotated as ‘variant of unknown significance’ in both the ACMG classifications and ClinVar annotations. ATM is a gene involved in autoimmunity (Gelzo et al., 2022) from which we prioritised a ‘variant of unknown significance’ variant, namely ATM:p.R1086H, annotated as ‘variant of unknown significance’ in ACMG classifications. We also identified another non-synonymous variant (benign as per ACMG classification) in ATM, namely ATM:p.P604S, in multiple probands. We also prioritized the RNASEL variant p.A175S, a heterozygous non-synonymous variant annotated as ‘variant of unknown significance’ in ACMG classifications. RNASEL plays a key role in antiviral defence, by being involved in IFN-stimulated pathways and cytokine signalling. Lee et al. (2023) linked its homozygous variants to MIS-C, suggesting that our heterozygous variant finding could influence susceptibility to this condition.
The last variant, annotated as ‘variant of unknown significance’ in ACMG classifications but as ‘risk factor’ for MIS-C in ClinVar is LY9:p.H351fs. Abuhammour et al. (2022) have previously reported this variant in individuals with MIS-C. LY9 is involved in the IL-2 signalling pathway and is essential for modulating immune responses and activating lymphocytes. In addition, we have identified a non-frameshift deletion in the LY9 gene, namely p.170_171del, which has not been reported in ClinVar and is categorized as benign in ACMG classifications.
Although variants classified as benign or likely benign according to ACMG criteria typically do not suggest a disease association, they may still hold potential for novel genetic links with MIS-C. This potential arises from observations by other research groups, who have prioritized variants within the same genes, even when not all variants have been evaluated using ACMG guidelines. Such genes, including NLRP12, DCLRE1C, IRAK3, PSTPIP1, NOD2, LRBA, CR2, AP3B1, and DOCK8 (Abolhassani et al., 2022; Abuhammour et al., 2022; Reis et al., 2023; Gelzo et al., 2022; Vagrecha et al., 2022), are also noted for their differential expression in MIS-C versus control groups (Table 3). For instance, the NLRP12:p.R284X variant, while classified as likely benign by ACMG, is labelled as pathogenic in ClinVar for the rare monogenic autosomal dominant condition of familial cold autoinflammatory syndrome 2 in childhood (Wang, 2022). This discrepancy underscores the complexity of variant classification and the potential for re-evaluation in the context of MIS-C.
To gain a comprehensive understanding of the monogenic determinants of MIS-C, we integrated the findings from our two approaches (namely the candidate gene approach and the candidate variant approach) into a unified analysis (Figure 3). This integration revealed significant connections and shared regulatory pathways between the two sets of resultant genes, emphasizing the consistency between the genes identified through each of the two approaches. Such a convergence highlights the crucial roles of immune response, inflammation, and viral pathogenesis in the development of MIS-C, justifying the use of these approaches in parallel to capture the multifaceted nature of the disease.
To further substantiate the relevance of the 53 candidate genes identified for MIS-C by the candidate variant approach, we utilized a protein-protein interaction network analysis to explore their relationships with the established 84-gene MIS-C panel (Figure 4). Despite the absence of direct overlap between these two gene sets, our analysis identified intermediary genes that functionally connect them, significantly enriching pathways critical for immune response, inflammation, and cell signalling. This demonstrates that these intermediary genes bridge the candidate and known gene sets, playing pivotal roles in MIS-C pathogenesis through shared biological pathways.
Our dual prioritization approach for identifying candidate genes and variants did not yield conclusive results for some probands, highlighting several important limitations of our study. The diagnostic yield of exome sequencing for monogenic disorders, which stands at approximately 25-58%, suggests that many causative variants may evade detection with this method alone (Dillon et al., 2018). In addition, we did not focus on compound heterozygous variants due to the nascent state of candidate gene identification for MIS-C. Moreover, the absence of prioritized variants in certain probands suggests a need for more comprehensive genetic analysis techniques, such as genome sequencing, that have a higher diagnostic yield and coverage range compared to exome sequencing (Nurchis et al., 2023). Our findings may also be influenced by factors such as variant frequencies exceeding our predefined thresholds, in silico predictions of variants being benign or tolerated, absence of COVID-19 positive control group, or the presence of large deletions or insertions, copy number variations, and epigenetic modifications (Davalos et al., 2022). Given the emergent and severe nature of MIS-C, and its overlap with other immunological conditions that can complicate prognosis and diagnosis, as well as the provisional status and incomprehensiveness of the associated gene panel, we proceed with caution. The genes and variants identified in this study are currently proposed to be associated with MIS-C, without a clear mechanistic link to its pathogenesis. This necessitates additional functional genomics studies to validate these associations and to further explore their roles in the disease. Additionally, confirming the findings of our study across cohorts of diverse ethnicities, while accounting for genetic differences among individuals, could enhance the robustness of our results.
In conclusion, our study underscores the significant influence of genetic predispositions, particularly through monogenic mechanisms, on the risk and presentation of MIS-C post-COVID-19. By employing dual approaches to identify candidate genes and variants, we have highlighted potential monogenic origins of MIS-C among children of primarily Arab descent. Future research should focus on confirming these preliminary associations and detailing their mechanistic contributions, thereby aiding in the development of targeted therapeutic strategies.
Data availability statement
The data analysed in this study pertains to minor children and hence the data cannot be made publicly available. However, requests for data may be addressed to HA at aGVzc2EuYWxrYW5kYXJpQGRhc21hbmluc3RpdHV0ZS5vcmc=.
Ethics statement
The studies involving humans were approved by Ethical Review Committee at Dasman Diabetes Institute and the Ethical review Committee at the Ministry of Health of Kuwait. The studies were conducted in accordance with the local legislation and institutional requirements. Written informed consent for participation in this study was provided by the participants’ legal guardians/next of kin.
Author contributions
MD: Conceptualization, Data curation, Formal analysis, Funding acquisition, Investigation, Methodology, Project administration, Resources, Software, Supervision, Validation, Visualization, Writing – original draft, Writing – review & editing. HA: Conceptualization, Data curation, Formal analysis, Funding acquisition, Investigation, Methodology, Project administration, Resources, Supervision, Validation, Visualization, Writing – original draft, Writing – review & editing. MM: Conceptualization, Data curation, Formal analysis, Funding acquisition, Investigation, Methodology, Project administration, Resources, Software, Supervision, Validation, Visualization, Writing – original draft. RN: Data curation, Methodology, Writing – original draft. SEJ: Data curation, Methodology, Writing – original draft. SJ: Data curation, Methodology, Writing – original draft. AC: Data curation, Methodology, Writing – original draft. FO: Data curation, Writing – original draft. SA-S: Conceptualization, Data curation, Investigation, Methodology, Writing – original draft. OA-H: Data curation, Methodology, Writing – original draft. MA-M: Data curation, Methodology, Writing – original draft. TT: Writing – original draft, Writing – review & editing. FA-M: Writing – original draft, Writing – review & editing.
Funding
The author(s) declare financial support was received for the research, authorship, and/or publication of this article. This study was supported by Kuwait Ministry of Health and by institutional funding from the Kuwait Foundation for the Advancement of Sciences to Dasman Diabetes Institute.
Acknowledgments
We thank all referring physicians and patients for their willingness to contribute to the study. We extend our sincere gratitude to the National Dasman Biobank staff, and Mr. Sriraman Devarajan for their extended support throughout the study.
Conflict of interest
The authors declare that the research was conducted in the absence of any commercial or financial relationships that could be construed as a potential conflict of interest.
Publisher’s note
All claims expressed in this article are solely those of the authors and do not necessarily represent those of their affiliated organizations, or those of the publisher, the editors and the reviewers. Any product that may be evaluated in this article, or claim that may be made by its manufacturer, is not guaranteed or endorsed by the publisher.
Supplementary material
The Supplementary Material for this article can be found online at: https://www.frontiersin.org/articles/10.3389/fcimb.2024.1444216/full#supplementary-material
Supplementary Table 1 | Demographic and Clinical Characteristics of the MIS-C Patients.
Supplementary Table 2 | MIS-C associated genes panel.
References
Abolhassani, H., Landegren, N., Bastard, P., Materna, M., Modaresi, M., Du, L., et al. (2022). Inherited IFNAR1 deficiency in a child with both critical COVID-19 pneumonia and multisystem inflammatory syndrome. J. Clin. Immunol. 42, 471–483. doi: 10.1007/s10875-022-01215-7
Abuhammour, W., Yavuz, L., Jain, R., Abu Hammour, K., Al-Hammouri, G. F., El Naofal, M., et al. (2022). Genetic and clinical characteristics of patients in the middle east with multisystem inflammatory syndrome in children. JAMA Netw. Open 5, e2214985. doi: 10.1001/jamanetworkopen.2022.14985
Asano, T., Boisson, B., Onodi, F., Matuozzo, D., Moncada-Velez, M., Maglorius Renkilaraj, M. R. L., et al. (2021). X-linked recessive TLR7 deficiency in ~1% of men under 60 years old with life-threatening COVID-19. Sci. Immunol. 6. doi: 10.1126/sciimmunol.abl4348
Bez Batti Angulski, A., Hosny, N., Cohen, H., Martin, A. A., Hahn, D., Bauer, J., et al. (2023). Duchenne muscular dystrophy: disease mechanism and therapeutic strategies. Front. Physiol. 14. doi: 10.3389/fphys.2023.1183101
Chou, J., Platt, C. D., Habiballah, S., Nguyen, A. A., Elkins, M., Weeks, S., et al. (2021). Mechanisms underlying genetic susceptibility to multisystem inflammatory syndrome in children (MIS-C). J. Allergy Clin. Immunol. 148, 732–738.e731. doi: 10.1016/j.jaci.2021.06.024
Davalos, V., García-Prieto, C. A., Ferrer, G., Aguilera-Albesa, S., Valencia-Ramos, J., Rodríguez-Palmero, A., et al. (2022). Epigenetic profiling linked to multisystem inflammatory syndrome in children (MIS-C): A multicenter, retrospective study. EClinicalMedicine 50, 101515. doi: 10.1016/j.eclinm.2022.101515
de Cevins, C., Luka, M., Smith, N., Meynier, S., Magérus, A., Carbone, F., et al. (2021). A monocyte/dendritic cell molecular signature of SARS-CoV-2-related multisystem inflammatory syndrome in children with severe myocarditis. Med 2, 1072–1092.e1077. doi: 10.1016/j.medj.2021.08.002
de Farias, E. C. F., Pedro Piva, J., de Mello, M. L. F. M., do Nascimento, L. M. P. P., Costa, C. C., MaChado, M. M. M., et al. (2020). Multisystem inflammatory syndrome associated with coronavirus disease in children: A multi-centered study in belém, pará, Brazil. Pediatr. Infect. Dis. J. 39, e374–e376. doi: 10.1097/INF.0000000000002865
Dillon, O. J., Lunke, S., Stark, Z., Yeung, A., Thorne, N., Gaff, C., et al. (2018). Exome sequencing has higher diagnostic yield compared to simulated disease-specific panels in children with suspected monogenic disorders. Eur. J. Hum. Genet. 26, 644–651. doi: 10.1038/s41431-018-0099-1
Franz, M., Rodriguez, H., Lopes, C., Zuberi, K., Montojo, J., Bader, G. D., et al. (2018). GeneMANIA update 2018. Nucleic Acids Res. 46, W60–W64. doi: 10.1093/nar/gky311
Gelzo, M., Castaldo, A., Giannattasio, A., Scalia, G., Raia, M., Esposito, M. V., et al. (2022). MIS-C: A COVID-19-as sociated condition between hypoimmunity and hyperimmunity. Front. Immunol. 13. doi: 10.3389/fimmu.2022.985433
Heaton, M. P., Clawson, M. L., Chitko-Mckown, C. G., Leymaster, K. A., Smith, T. P., Harhay, G. P., et al. (2012). Reduced lentivirus susceptibility in sheep with TMEM154 mutations. PloS Genet. 8, e1002467. doi: 10.1371/journal.pgen.1002467
Jones, V. G., Mills, M., Suarez, D., Hogan, C. A., Yeh, D., Segal, J. B., et al. (2020). COVID-19 and kawasaki disease: novel virus and novel case. Hosp Pediatr. 10, 537–540. doi: 10.1542/hpeds.2020-0123
Kuleshov, M. V., Jones, M. R., Rouillard, A. D., Fernandez, N. F., Duan, Q., Wang, Z., et al. (2016). Enrichr: a comprehensive gene set enrichment analysis web server 2016 update. Nucleic Acids Res. 44, W90–W97. doi: 10.1093/nar/gkw377
Landgraf, K., Kühnapfel, A., Schlanstein, M., Biemann, R., Isermann, B., Kempf, E., et al. (2022). Transcriptome analyses of adipose tissue samples identify. Int. J. Mol. Sci. 23. doi: 10.3390/ijms23084349
Lee, D., Le Pen, J., Yatim, A., Dong, B., Aquino, Y., Ogishi, M., et al. (2023). Inborn errors of OAS-RNase L in SARS-CoV-2-related multisystem inflammatory syndrome in children. Science 379, eabo3627. doi: 10.1126/science.abo3627
Lee, P. Y., Platt, C. D., Weeks, S., Grace, R. F., Maher, G., Gauthier, K., et al. (2020). Immune dysregulation and multisystem inflammatory syndrome in children (MIS-C) in individuals with haploinsufficiency of SOCS1. J. Allergy Clin. Immunol. 146, 1194–1200.e1191. doi: 10.1016/j.jaci.2020.07.033
Levy, N., Koppel, J. H., Kaplan, O., Yechiam, H., Shahar-Nissan, K., Cohen, N. K., et al. (2022). Severity and incidence of multisystem inflammatory syndrome in children during 3 SARS-CoV-2 pandemic waves in Israel. JAMA 327, 2452–2454. doi: 10.1001/jama.2022.8025
Li, H., Durbin, R. (2009). Fast and accurate short read alignment with Burrows-Wheeler transform. Bioinformatics 25, 1754–1760. doi: 10.1093/bioinformatics/btp324
Li, H., Handsaker, B., Wysoker, A., Fennell, T., Ruan, J., Homer, N., et al. (2009). The sequence alignment/map format and SAMtools. Bioinformatics 25, 2078–2079. doi: 10.1093/bioinformatics/btp352
McKenna, A., Hanna, M., Banks, E., Sivachenko, A., Cibulskis, K., Kernytsky, A., et al. (2010). The Genome Analysis Toolkit: a MapReduce framework for analyzing next-generation DNA sequencing data. Genome Res. 20, 1297–1303. doi: 10.1101/gr.107524.110
McLaren, W., Gil, L., Hunt, S. E., Riat, H. S., Ritchie, G. R., Thormann, A., et al. (2016). The ensembl variant effect predictor. Genome Biol. 17, 122. doi: 10.1186/s13059-016-0974-4
Miyashita, M., Oshiumi, H., Matsumoto, M., Seya, T. (2011). DDX60, a DEXD/H box helicase, is a novel antiviral factor promoting RIG-I-like receptor-mediated signaling. Mol. Cell Biol. 31, 3802–3819. doi: 10.1128/MCB.01368-10
Nurchis, M. C., Altamura, G., Riccardi, M. T., Radio, F. C., Chillemi, G., Bertini, E. S., et al. (2023). Whole genome sequencing diagnostic yield for paediatric patients with suspected genetic disorders: systematic review, meta-analysis, and GRADE assessment. Arch. Public Health 81, 93. doi: 10.1186/s13690-023-01112-4
Payne, A. B., Gilani, Z., Godfred-Cato, S., Belay, E. D., Feldstein, L. R., Patel, M. M., et al. (2021). Incidence of multisystem inflammatory syndrome in children among US persons infected with SARS-CoV-2. JAMA Netw. Open 4, e2116420. doi: 10.1001/jamanetworkopen.2021.16420
Qabazard, S., Al-Abdulrazzaq, D., Al-Kandari, H., Ayed, M., Alanezi, A., Al-Shammari, N., et al. (2022). The pediatric COVID-19 registry in Kuwait: methodology and results of pilot phase. Med. Princ Pract. 31, 471–479. doi: 10.1159/000524756
Reis, B. C. S. D., Soares Faccion, R., de Carvalho, F. A. A., Moore, D. C. B. C., Zuma, M. C. C., Plaça, D. R., et al. (2023). Rare genetic variants involved in multisystem inflammatory syndrome in children: a multicenter Brazilian cohort study. Front. Cell Infect. Microbiol. 13. doi: 10.3389/fcimb.2023.1182257
Richards, S., Aziz, N., Bale, S., Bick, D., Das, S., Gastier-Foster, J., et al. (2015). Standards and guidelines for the interpretation of sequence variants: a joint consensus recommendation of the American College of Medical Genetics and Genomics and the Association for Molecular Pathology. Genet. Med. 17, 405–424. doi: 10.1038/gim.2015.30
Riphagen, S., Gomez, X., Gonzalez-Martinez, C., Wilkinson, N., Theocharis, P. (2020). Hyperinflammatory shock in children during COVID-19 pandemic. Lancet 395, 1607–1608. doi: 10.1016/S0140-6736(20)31094-1
Sancho-Shimizu, V., Brodin, P., Cobat, A., Biggs, C. M., Toubiana, J., Lucas, C. L., et al. (2021). SARS-CoV-2-related MIS-C: A key to the viral and genetic causes of Kawasaki disease? J. Exp. Med. 218. doi: 10.1084/jem.20210446
Santos-Rebouças, C. B., Piergiorge, R. M., Dos Santos Ferreira, C., Seixas Zeitel, R., Gerber, A. L., Rodrigues, M. C. F., et al. (2022). Host genetic susceptibility underlying SARS-CoV-2-associated Multisystem Inflammatory Syndrome in Brazilian Children. Mol. Med. 28, 153. doi: 10.1186/s10020-022-00583-5
Schulert, G. S., Zhang, M., Fall, N., Husami, A., Kissell, D., Hanosh, A., et al. (2016). Whole-exome sequencing reveals mutations in genes linked to hemophagocytic lymphohistiocytosis and macrophage activation syndrome in fatal cases of H1N1 influenza. J. Infect. Dis. 213, 1180–1188. doi: 10.1093/infdis/jiv550
Shannon, P., Markiel, A., Ozier, O., Baliga, N. S., Wang, J. T., Ramage, D., et al. (2003). Cytoscape: a software environment for integrated models of biomolecular interaction networks. Genome Res. 13, 2498–2504. doi: 10.1101/gr.1239303
Solanich, X., Vargas-Parra, G., van der Made, C. I., Simons, A., Schuurs-Hoeijmakers, J., Antolí, A., et al. (2021). Genetic screening for. Front. Immunol. 12. doi: 10.3389/fimmu.2021.719115
Song, P., Yang, W., Lou, K. F., Dong, H., Zhang, H., Wang, B., et al. (2022). UNC13D inhibits STING signaling by attenuating its oligomerization on the endoplasmic reticulum. EMBO Rep. 23, e55099. doi: 10.15252/embr.202255099
Toubiana, J., Poirault, C., Corsia, A., Bajolle, F., Fourgeaud, J., Angoulvant, F., et al. (2020). Kawasaki-like multisystem inflammatory syndrome in children during the covid-19 pandemic in Paris, France: prospective observational study. BMJ 369, m2094. doi: 10.1136/bmj.m2094
Vagrecha, A., Zhang, M., Acharya, S., Lozinsky, S., Singer, A., Levine, C., et al. (2022). Hemophagocytic lymphohistiocytosis gene variants in multisystem inflammatory syndrome in children. Biol. (Basel) 11. doi: 10.3390/biology11030417
van der Made, C. I., Simons, A., Schuurs-Hoeijmakers, J., van den Heuvel, G., Mantere, T., Kersten, S., et al. (2020). Presence of genetic variants among young men with severe COVID-19. JAMA 324, 663–673. doi: 10.1001/jama.2020.13719
Verdoni, L., Mazza, A., Gervasoni, A., Martelli, L., Ruggeri, M., Ciuffreda, M., et al. (2020). An outbreak of severe Kawasaki-like disease at the Italian epicentre of the SARS-CoV-2 epidemic: an observational cohort study. Lancet 395, 1771–1778. doi: 10.1016/S0140-6736(20)31103-X
Wang, H. F. (2022). NLRP12-associated systemic autoinflammatory diseases in children. Pediatr. Rheumatol Online J. 20, 9. doi: 10.1186/s12969-022-00669-8
Wang, K., Li, M., Hakonarson, H. (2010). ANNOVAR: functional annotation of genetic variants from high-throughput sequencing data. Nucleic Acids Res. 38, e164. doi: 10.1093/nar/gkq603
Wang, J., Duncan, D., Shi, Z., Zhang, B. (2013). WEB-based GEne SeT AnaLysis Toolkit (WebGestalt): update 2013. Nucleic Acids Res 41 (Web Server issue), W77–83.
Whittaker, E., Bamford, A., Kenny, J., Kaforou, M., Jones, C. E., Shah, P., et al. (2020). Clinical characteristics of 58 children with a pediatric inflammatory multisystem syndrome temporally associated with SARS-CoV-2. JAMA 324, 259–269. doi: 10.1001/jama.2020.10369
World Health Organization. (2020). Multisystem Inflammatory Syndrome in Children and Adolescents with COVID-19 (Geneva, Switzerland: World Health Organization). Available at: https://www.who.int/publications/i/item/multisystem-inflammatory-syndrome-in-children-and-adolescents-with-covid-19 (Accessed March 25, 2024).
Yousaf, A. R., Cortese, M. M., Taylor, A. W., Broder, K. R., Oster, M. E., Wong, J. M., et al. (2022). Reported cases of multisystem inflammatory syndrome in children aged 12-20 years in the USA who received a COVID-19 vaccine, December 2020, through August 2021: a surveillance investigation. Lancet Child Adolesc. Health 6, 303–312. doi: 10.1016/S2352-4642(22)00028-1
Zhang, B., Kirov, S. A., Snoddy, J. R. (2005). WebGestalt: an integrated system for exploring gene sets in various biological contexts. Nucleic Acids Res 33 (Web Server issue), W741–748.
Keywords: multisystem inflammatory syndrome in children, coronavirus infection, MIS-C, exome sequencing, monogenic
Citation: Dashti M, AlKandari H, Malik MZ, Nizam R, John SE, Jacob S, Channanath A, Othman F, Al-Sayed S, Al-Hindi O, Al-Mutari M, Thanaraj TA and Al-Mulla F (2025) Genetic insights into MIS-C Post-COVID-19 in Kuwaiti children: investigating monogenic factors. Front. Cell. Infect. Microbiol. 14:1444216. doi: 10.3389/fcimb.2024.1444216
Received: 29 July 2024; Accepted: 16 December 2024;
Published: 08 January 2025.
Edited by:
Marceline Djuidje Ngounoue, University of Yaounde I, CameroonReviewed by:
Eliane Chouery, Lebanese American University, LebanonLacramioara Butnariu, Grigore T. Popa University of Medicine and Pharmacy, Romania
Copyright © 2025 Dashti, AlKandari, Malik, Nizam, John, Jacob, Channanath, Othman, Al-Sayed, Al-Hindi, Al-Mutari, Thanaraj and Al-Mulla. This is an open-access article distributed under the terms of the Creative Commons Attribution License (CC BY). The use, distribution or reproduction in other forums is permitted, provided the original author(s) and the copyright owner(s) are credited and that the original publication in this journal is cited, in accordance with accepted academic practice. No use, distribution or reproduction is permitted which does not comply with these terms.
*Correspondence: Fahd Al-Mulla, ZmFoZC5hbG11bGxhQGRhc21hbmluc3RpdHV0ZS5vcmc=; Thangavel Alphonse Thanaraj, YWxwaG9uc2UudGhhbmdhdmVsQGRhc21hbmluc3RpdHV0ZS5vcmc=
†These authors have contributed equally to this work