- 1Department of Microbiology Laboratory, Children’s Hospital Affiliated to Shandong University, Jinan, China
- 2Department of Clinical Microbiology, Shandong Provincial Clinical Research Center for Children’s Health and Disease, Jinan, China
- 3Department of Outpatient, 94201 Military Hospital, Jinan, China
- 4Department of Scientific Affairs, Vision Medicals Center for Infectious Diseases, Guangzhou, China
Objectives: This study aimed to identify the risk factors and construct the diagnostic model associated with pneumocystis pneumonia (PCP) in pediatric patients.
Methods: This retrospective observational study analyzed 34 cases of PCP and 51 cases of other types of pneumonia treated at Children’s Hospital Affiliated to Shandong University between January 2021 and August 2023. Multivariate binary logistic regression was used to identify the risk factors associated with PCP. Receiver operating characteristic curves and calibration plots were constructed to evaluate the diagnostic model.
Results: Twenty clinical variables significantly differed between the PCP and non-PCP groups. Multivariate binary logistic regression analysis revealed that dyspnea, body temperature>36.5°C, and age<1.46 years old were risk factors for PCP. The area under the curve of the diagnostic model was 0.958, the P-value of Hosmer‐Lemeshow calibration test was 0.346, the R2 of the calibration plot for the actual and predicted probability of PCP was 0.9555 (P<0.001), and the mean Brier score was 0.069. In addition, metagenomic next-generation sequencing revealed 79.41% (27/34) and 52.93% (28/53) mixed infections in the PCP and non-PCP groups, respectively. There was significantly more co-infection with cytomegalovirus and Streptococcus pneumoniae in the PCP group than that in the non-PCP group (p<0.05).
Conclusions: Dyspnea, body temperature>36.5°C, and age<1.46 years old were found to be independent risk factors for PCP in pediatric patients. The probability of co-infection with cytomegalovirus and S. pneumoniae in the PCP group was significantly higher than that in the non-PCP group.
Introduction
Pneumocystis pneumonia (PCP) is a fungal infectious disease of the respiratory system caused by Pneumocystis jirovecii. Pneumocystis pneumonia is the most common opportunistic infection among patients with human immunodeficiency virus (HIV) infection. Recently, the prevalence of PCP in patients with HIV has decreased due to the use of highly effective antiretroviral therapy and prevention. However, with the increase in the number of patients with cancer, solid organ transplantation, and autoimmune diseases, along with advances in bronchoscopy and microbiological testing techniques, the morbidity and mortality of non-HIV-related PCP have significantly increased (Niu et al., 2023). In the early stage of P. jirovecii infection, patients may be asymptomatic. However, in the late stage, PCP progresses rapidly, leading to severe respiratory failure, poor prognosis, and high mortality rates (Liu et al., 2019).
The prognosis for pediatric patients with PCP largely depends on early, timely, and accurate diagnosis (Lu et al., 2022). Polymerase chain reaction (PCR) is more commonly used in the diagnosis of PCP, but the infection rate of P. jirovecii in pediatric patients is very low, and many children’s specialized hospitals do not carry out PCR methods to detect PCP (Liu et al., 2020). It has been reported that combining the detection of P. jirovecii sequences in bronchoalveolar lavage fluid (BALF) and peripheral blood by metagenomic next-generation sequencing (mNGS), with the patient’s clinical manifestations and computed tomography imaging features, can confirm the diagnosis of PCP, but only a few cases have been reported in the clinic (Zhang et al., 2019). In this study, we reviewed clinical data and mNGS reports from 85 children diagnosed with pneumonia, including 34 cases of PCP and 51 cases of other pneumonia types. We identified potential risk factors associated with PCP and developed a diagnostic model based on these factors. This model aims to serve as an early indicator of PCP infection risk in non-HIV patients.
Methods
Study design
A total of 34 children with PCP were retrospectively enrolled between January 2021 and August 2023. The diagnostic criteria (Donnelly et al., 2020; Lagrou et al., 2021; Huang et al., 2023)for PCP group were as follows: 1) Clinical symptoms with fever, cough, or shortness of breath; 2) Chest computerized tomography (CT) showed multiple rounds of glassy interstitial exudate, reticulated or solid shadows in both lungs on an chest computerized tomography; 3) The sequence of P. jirovecii was detected by mNGS; 4) The HIV test was negative. At the same time, 51 non-HIV patients who were admitted to the hospital with lung infections and diagnosed with non-PCP pneumonia as the non-PCP group during the same period were included. The clinical diagnosis of PCP and non-PCP was made by two senior respiratory specialists based on the clinical symptoms, laboratory findings, chest CT images, etiology of mNGS, and clinical response to treatment. The clinical data, including gender, age, underlying disease, clinical manifestations, CT imaging, mNGS test, and laboratory examinations, were collected from the patient’s medical records.
This study was approved by the Ethics Committee of the Children’s Hospital Affiliated to Shandong University (No. SDFE-IRB/P-2022017) and was conducted by the Declaration of Helsinki (revised 2013). The Ethics Committee of the Children’s Hospital Affiliated with Shandong University waived the need for individual informed consent for this retrospective analysis.
Metagenomic next-generation sequencing
The DNA was extracted from BALF using a QIAamp® UCP Pathogen DNA Kit (Qiagen), adhering to the manufacturer’s instructions. Human DNA was removed using Benzonase (Qiagen) and Tween 20 (Sigma). Total RNA was extracted with a QIAamp ® Viral RNA Kit (Qiagen) and ribosomal RNA was removed with a Ribo-Zero rRNA Removal Kit (Illumina). cDNA was generated using reverse transcriptase and dNTPs (Thermos Fisher). Libraries were constructed for the DNA and cDNA samples using a NextEra XT DNA Library Prep Kit (Illumina, San Diego, CA). The library was purified, and magnetic beads selected the fragments. The library quality was assessed with a Qubit dsDNA HS Assay Kit followed by a High Sensitivity DNA kit (Agilent) on an Agilent 2100 Bioanalyzer. The library pools were then loaded onto an Illumina NextSeq CN500 sequencer for 75 cycles of single-end sequencing to generate approximately 20 million reads for each library. For negative controls, we also prepared sterile deionized water in parallel with each batch to serve as a non-template control, using the same protocol.
High-quality sequencing data were generated by removing low-quality and short (length < 40 bp) reads, followed by computational subtraction of human host sequences mapped to the human reference genome (hg38 and YH sequences) using Burrows-Wheeler alignment. The remaining data obtained by removing low-complexity reads were classified by simultaneous alignment to four microbial genome databases, consisting of viruses, bacteria, fungi, and parasites. The classification reference databases were downloaded and optimized from public databases such as NCBI and GenBank. In the end, the multi-parameters of Species in the microbial genome databases were calculated and exported, and professionals with microbiology and clinical backgrounds interpreted the results.
Statistical analysis
The data were analyzed using IBM SPSS 26.0 statistical software. Normally distributed continuous data are presented as the mean ± SD and were compared using a t-test. Continuous data that did not fit a normal distribution are presented as the median (Q1, Q3) and were compared using the Mann−Whitney U test. The discontinuous data are presented as n (%) and were compared using the χ2 test or Fisher’s test. Univariate logistic regression was used to identify the risk factors associated with PCP. Variables with P-value ≤0.1 were further analysed via multiple logistic regression, and variables with P-value < 0.05 were used to construct the diagnostic model. The diagnostic model was evaluated using receiver operating characteristic (ROC) curves and Hosmer-Lemeshow goodness-of-fit tests. GraphPad Prism 9.3 was utilized to create figures, including ROC curves and forest plots.
Results
Patient characteristics
As shown in Table 1, the 20 clinical variables showed significant differences between the PCP and non-PCP groups. The PCP group had 21 (61.76%) boys, while the no-PCP group had 31 (60.78%) boys, with no significant difference observed. The median age was 0.58 years old in the PCP group and 5.33 years in the non-PCP group, and the difference was remarkable (P <0.001). In terms of underlying diseases, the PCP group had significantly higher rates of surgery (50.00% vs 9.80%, P <0.001), tracheal dysplasia (55.88% vs 13.72%, P <0.001), and premature delivery (35.29% vs 5.88%, P <0.001) compared to the non-PCP group. Regarding clinical manifestations, dyspnea (94.12% vs 13.73%, P <0.001), body temperature (37.40°C vs 36.50°C, P =0.002), heart rate (135 beats vs 105 beats, P <0.001), and respiratory rate (35 beats vs 28 beats, P <0.001) were significantly greater in the PCP groups than in the non-PCP group.
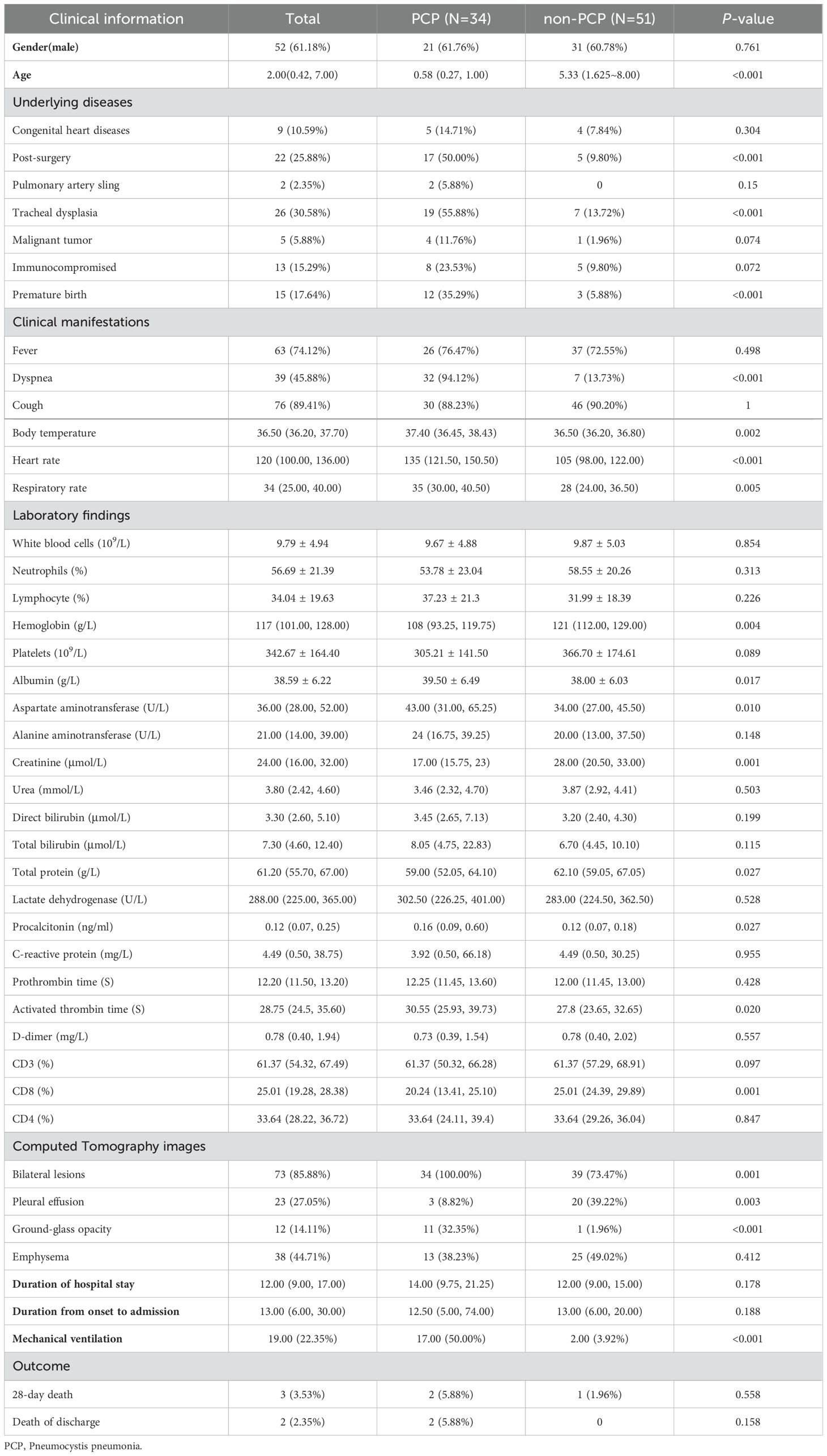
Table 1. Patient’ characteristics, laboratory findings and CT images of PCP and non-PCP pediatric patients.
There were no remarkable differences in routine blood parameters, such as white blood cell count, neutrophils count, or lymphocyte percentage between the two groups. However, in terms of blood biochemistry, hemoglobin (108 vs 121, p=0.004), creatinine (17 vs 28, P =0.001), and total protein (59.00 vs 62.10, P =0.27) were significantly decreased in the PCP group. On the other hand, the levels of albumin (39.50 vs 38.00, P =0.017), aspartate aminotransferase (43 vs 34, P =0.01), procalcitonin (0.16 vs 0.12, P =0.27), and activated partial thromboplastin time (30.55 vs 27.80, P =0.02) were significantly increased in the PCP group. Additionally, the PCP group exhibited a significant increase in CD8+ T lymphocytes (20.24 vs 25.01, P =0.001). Computerized tomography images revealed that bilateral lesions (100.00% vs 73.60%, P =0.001) and ground glass shadow (32.40% vs 1.90%, P <0.001) were more common in the PCP group, whereas pleural effusion (8.80% vs 26.40%, P =0.003) was more prevalent in the non-PCP group. Furthermore, mechanical ventilation (50% vs 3.80%, P <0.001) was significantly more common in the PCP group.
Risk factors for PCP
As depicted in Table 2, we analyzed the ability of the above-mentioned 20 clinical variables to predict PCP using the ROC curves and binary logistic regression. By applying the principle of maximum sensitivity and specificity, ROC curves allowed us to determine the cut-off values for continuous variables and convert them into categorical variables (Nahm, 2022). Based on the ROC curves, the thresholds for age, body temperature, heart rate, CD8+ T lymphocytes, and serum creatinine were found to be 1.46, 36.65, 127.00, 20.84, and 24.50, respectively. The areas under the ROC curves for dyspnea, mechanical ventilation, post-surgery, tracheal dysplasia, age, temperature, heart rate, CD8+ T lymphocytes, and serum creatinine all exceeded 0.70 (0.905, 0.731, 0.703, 0.713, 0.752, 0.700, 0.794, 0.711, and 0.719, respectively; 95% confidence intervals (CIs) were 0.834-0.975, 0614-0.848, 0.584-0.822, 0.596-0.830, 0.641-0.862, 0.579-0.821, 0.691-0.896, 0.579-0.821, 0.691-0.896, 0.596-0.825, and 0.579-0.841; all P-values were less than 0.05 (<0.001, <0.001, 0.001, 0.001, <0.001, 0.002, <0.001, 0.001, 0.001, respectively). Subsequently, univariate binary logistic regression analysis was used to screen for risk factors. Table 2 and Figure 1 illustrated those 18 variables, excluding bilateral lung lesions and albumin, the serum ALB concentration, were significantly different, with P-values lower than 0.05.
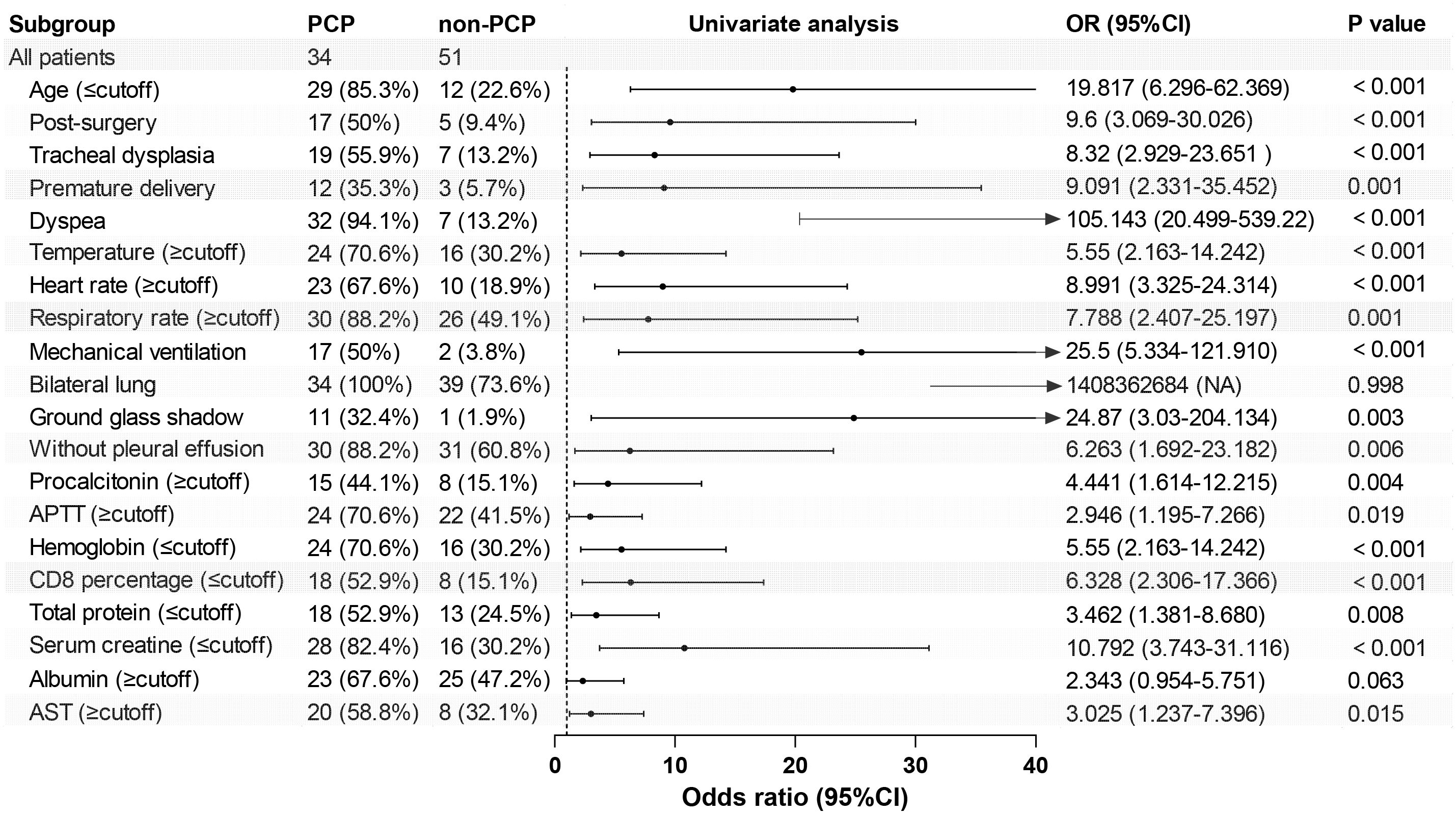
Figure 1. Forest map of 20 risk factors identified in the univariate logistic analysis for the PCP group. APTT, activated partial thromboplastin time; AST, aspartate aminotransferase.
A diagnostic model for PCP
Based on the results of the ROC curve and univariate logistic analysis, nine variables, including dyspnea, mechanical ventilation, post-surgery, tracheal dysplasia, age, temperature, heart rate, CD8+ T lymphocytes, and serum creatinine, were subjected to multivariate logistic regression analysis. As shown in Table 3 and Figure 2, dyspnea, age, and body temperature were found to be significantly associated with PCP, with odds ratios of 52.003, 10.233, and 16.556; 95% CIs of 7.023-385.037, 1.216-86.147, and 2.037-134.538; and P-values of <0.001, 0.032, and 0.009, respectively.
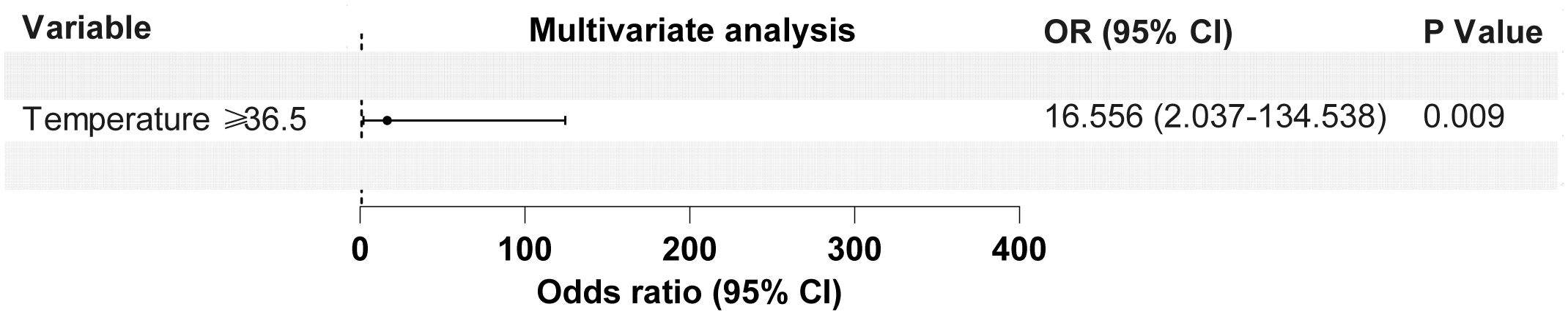
Figure 2. Forest map of 3 risk factors identified by the multivariate logistic analysis for the PCP group.
The predictive accuracy for PCP as measured by the ROC curves (Figure 3A), yielded an area under the curve (AUC) of 0.958 (95% CI: 0.916–0.999, P <0.001). The Hosmer‐Lemeshow calibration test was significant (P =0.346), and the calibration plot (Figure 3B) for predicting PCP showed moderate agreement between the actual observed outcome and the prediction (R2 = 0.9555, P <0.001). Overall, the prediction performance was good, with a mean Brier score of 0.069 (95% CI: 0.032–0.104).
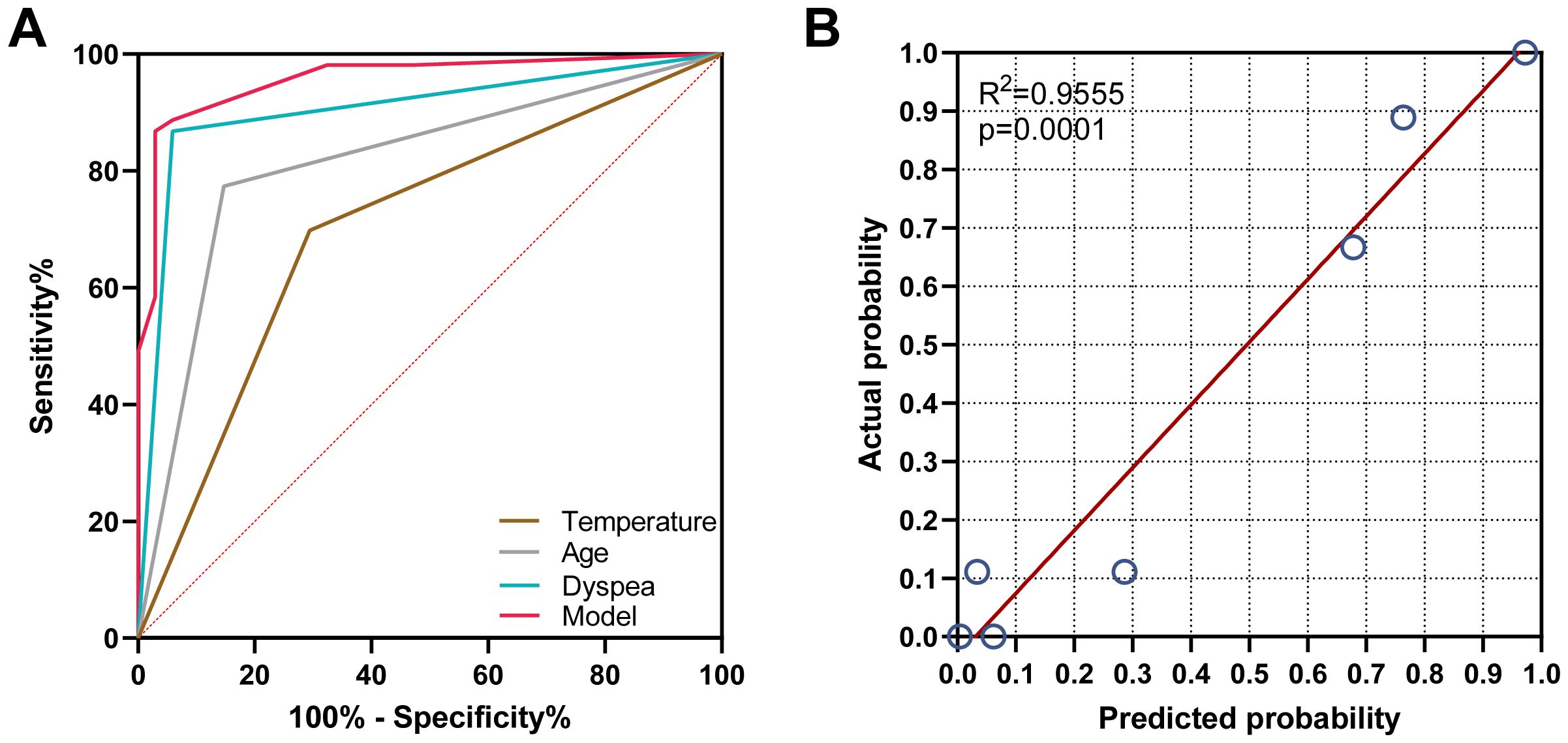
Figure 3. Validation of the model for predicting PCP probability. (A) The area under the receiver operating characteristic curve was 0.958, 0.905, 0.813, and 0.702 for the model group, dyspnea group, and age and body temperature group, respectively. (B) The calibration plot indicated that the predicted probability of PCP had a moderate agreement with the actual observed outcome (R2 = 0.9555, P<0.001).
Diagnostic performance of mNGS for mixed infections
The mixed infections were identified in 79.41% of patients in the PCP group and 52.93% of those in the non-PCP group using mNGS (Figures 4A, B). In the PCP group, the major co-pathogens associated with P. jirovecii, included cytomegalovirus (CMV), S. pneumoniae, Pseudomonas aeruginosa, and Haemophilus influenzae (Figure 4C). The study using mNGS revealed a significantly higher rate of co-infection with CMV (41.18% vs 13.21%, P =0.003) and S. pneumoniae (29.41% vs 7.55%, P =0.007) in the PCP group (Figure 4C).
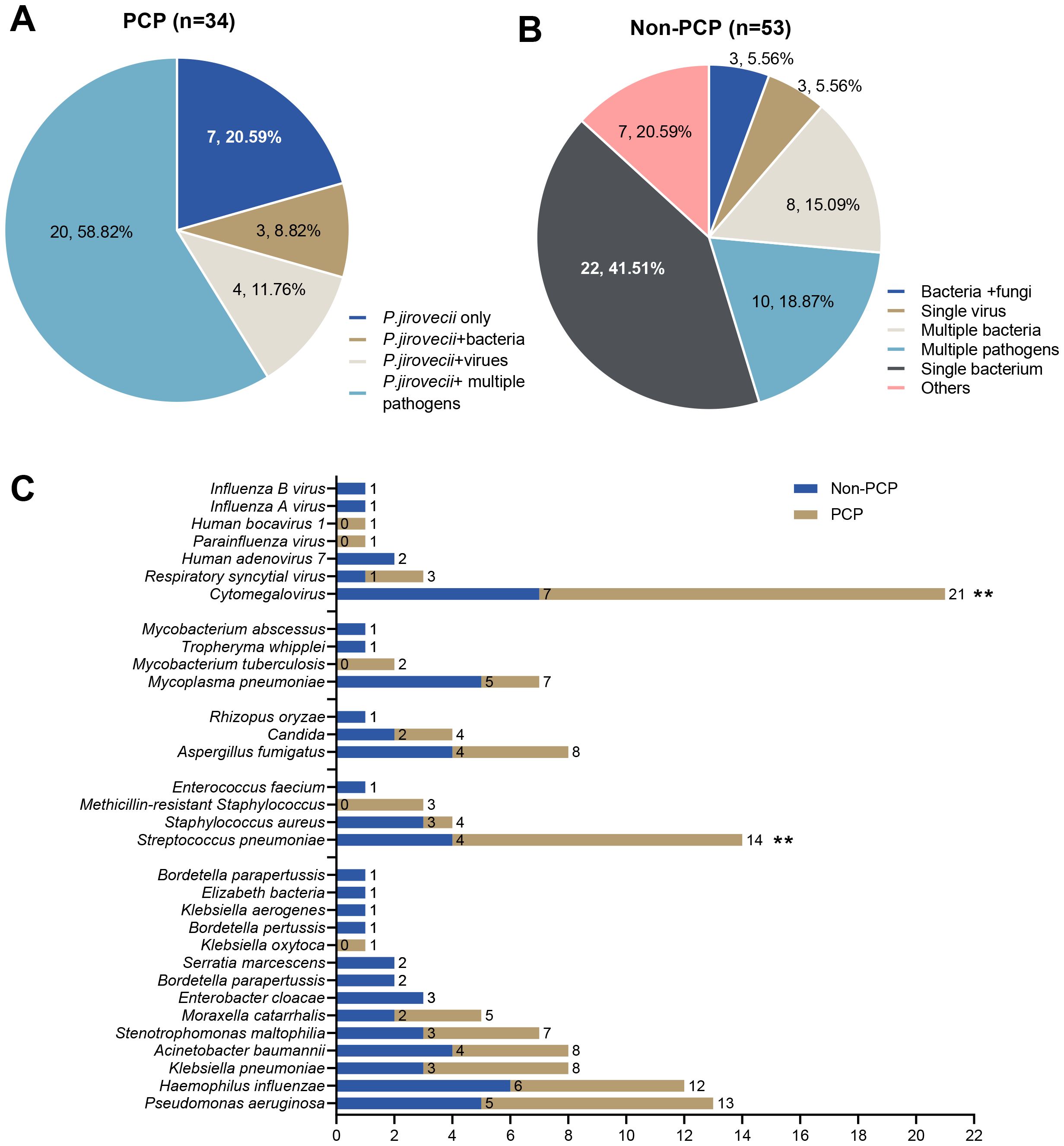
Figure 4. (A) The mixed infections were identified by mNGS in the PCP group. (B) The mixed infections were identified by mNGS in the non-PCP group. (C) Comparative analysis of major co-pathogenic in PCP group and non-PCP group (** Indicates p<0.01).
Discussion
P. jirovecii is an opportunistic pathogen that is extremely rare in children and often becomes a pathogen when the host’s immune function is compromised, leading to severe lung infections. The clinical symptoms of the majority of patients with PCP were nonspecific. Due to the uncultivable nature of P. jirovecii in vitro, the gold standard for diagnosing PCP is the presence of characteristic encapsulated or trophozoite bodies in specimens from the lower respiratory tract (Huang et al., 2024). However, the low load of P. jirovecii in the lower respiratory tract of the patients and the reliance on the ability to examine physicians significantly hinder the early diagnosis, leading to misdiagnosis and underdiagnosis (Delliere et al., 2020; McDonald et al., 2024).
In this retrospective study, we developed a new diagnostic model for PCP using non-invasive and easily accessible clinical indicators. This diagnostic model demonstrated reliable discriminatory power in assessing the probability of PCP (Zhou and Aitken, 2023). It is expected to assist clinicians in early and rapid bedside screening for PCP in children, ultimately improving the prognosis of these patients in the future (Lagrou et al., 2021). We first conducted univariate logistic analysis and ROC curve analysis on 20 clinical variables from 85 patients with severe pneumonia. Among these nine variables exhibited a P-value <0.05 in the logistic analysis and an AUC>0.7 in the ROC curve, leading to their further inclusion in multivariate binary logistic regression. Subsequently, dyspnea, age<1.46 years, and temperature>36.5 were identified as the risk factors in the multivariate logistic analysis. Upon combining these three factors, the diagnostic model achieved an AUC of 0.958. The P-value of the Hosmer‐Lemeshow calibration test was 0.346, the R2 of the calibration plot for the actual and predicted probability of PCP was 0.9555 (p<0.001), and the mean Brier score was 0.069 (95% CI: 0.032–0.104), demonstrating good overall prediction performance.
Various specimens, such as blood, sputum, nasopharyngeal secretions, and BALF, can be utilized for microbiological testing. Among these methods, BALF is particularly effective in diagnosing PCP (Guegan and Robert-Gangneux, 2019; Georges et al., 2020). Various laboratory detection methods for PCP exist, including microscopic, serological, and imaging examinations. However, these methods have a high false-negative rate in early infection. Tissue biopsy, while effective, is an invasive procedure with associated risks and may not be suitable for all patients. In recent years, PCR detection technology has advanced significantly, with the emergence of molecular detection methods that offer high specificity and sensitivity in detecting specific target sequences. Despite these advancements, these technologies still have limitations in detecting rare or unknown pathogens. Due to the atypical clinical manifestations and the high prevalence of mixed infections in PCP patients, the mNGS assay offers direct detection of microorganism sequences in the clinical samples with shorter turn-around time, high sensitivity, and notably high detection rates for mixed bacterial, viral, fungal, and parasitic infections (Chiu and Miller, 2019; Wang et al., 2019; Zhang et al., 2021; Wang et al., 2022; Chang et al., 2023). Consequently, mNGS has demonstrated excellent performance in PCP diagnosis and co-pathogen detection (Jiang et al., 2021).
In our study, we observed mixed infections in 79.41% and 52.93% of patients in the PCP and non-PCP groups, respectively. Cytomegalovirus, S. pneumoniae, P. aeruginosa, and H. influenzae were the major co-pathogens in PCP patients, with significantly higher detection rates of CMV and S. pneumoniae in PCP compared to non-PCP patients (P < 0.005). Cytomegalovirus infection, typically preceding or concurrent with PCP, represents a risk factor for PCP and can significantly increase the morbidity and mortality of PCP in solid organ transplant recipients (Faure et al., 2017; Hosseini-Moghaddam et al., 2018). Infection with CMV has been suggested to be the most common cause of PCP and may be linked to immunosuppression by suppressing the function of helper T-cells and antigen-presenting cells, indicating severe immunosuppression (Lee et al., 2017). In terms of PCP co-infection, S. pneumoniae infection ranks second, primarily colonizing the nasopharynx of children (especially those under five years of age). In states of reduced immune function, it can proliferate rapidly and spread to other parts of the body, leading to infection (Morilla et al., 2021).
In conclusion, age<1.46 years, dyspnea, and temperature>36.5°C are identified as risk factors for PCP in non-HIV patients, and the diagnostic model based on these three factors demonstrated good predictive diagnostic value for PCP infection. The technology of mNGS technology plays a crucial role in confirming the diagnosis of PCP, especially for identifying mixed infections involving multiple pathogens, exhibiting strong diagnostic performance. However, among 1481 patients tested for mNGS, only 34 positive children were detected in this study, and the positive rate was only 2.30%. Aware of the limited incidence of PCP infection in children and currently few studies published focus on children with PCP who are not infected with HIV, in order to attract the attention of global pediatric experts on PCP in non-HIV, we first need to report the small size of study population. In the future, our team needs to accumulate clinical cases for another 3-5 years. Combining we create Chinese children’s bacteria and fungus resistance monitoring network member unit (https://www.etyy.com/respro.html), later we will have a longer period of clinical big queue (such as expanding time, try using multicenter retrospective). As well as prospective ways to expand the number of children) to validate, while conducting more in-depth subgroup analyses.
Data availability statement
The datasets generated during or analyzed during the current study are not publicly available but are available from the corresponding author upon reasonable request.
Ethics statement
This study underwent rigorous review and was approved by the Ethical Review Committee of Children's Hospital Affiliated to Shandong University (approval no. SDFE-IRB/P-2022017). All procedures were conducted in strict compliance with the Ethical Review of Biomedical Research Involving Human Subjects (2016), the Declaration of Helsinki, and the International Ethical Guidelines for Biomedical Research Involving Human Subjects. Written informed consent was obtained from the legal guardian/next of kin of the minor(s) for the publication without any potentially identifiable images or data included in this article.
Author contributions
SW: Conceptualization, Data curation, Formal analysis, Funding acquisition, Investigation, Methodology, Project administration, Resources, Software, Supervision, Validation, Visualization, Writing – original draft, Writing – review & editing. CZ: Conceptualization, Data curation, Formal analysis, Funding acquisition, Investigation, Methodology, Resources, Writing – original draft. XC: Conceptualization, Formal analysis, Writing – review & editing. ZL: Data curation, Investigation, Resources, Writing – review & editing. MW: Conceptualization, Formal analysis, Funding acquisition, Project administration, Writing – review & editing. EY: Investigation, Methodology, Software, Writing – review & editing. HX: Data curation, Formal analysis, Methodology, Software, Supervision, Writing – review & editing.
Funding
The author(s) declare financial support was received for the research, authorship, and/or publication of this article. This work was supported by the Shandong Children’s Health and Disease Clinical Medical Research Center Project (grant number: RC006), the special fund for high-level talents in the medical and health industry of Jinan City (SW), and the Science and Technology Development Program of Jinan Municipal Health Commission (2022-1-45 and 2022-2-149).
Conflict of interest
The authors declare that the research was conducted in the absence of any commercial or financial relationships that could be construed as a potential conflict of interest.
Publisher’s note
All claims expressed in this article are solely those of the authors and do not necessarily represent those of their affiliated organizations, or those of the publisher, the editors and the reviewers. Any product that may be evaluated in this article, or claim that may be made by its manufacturer, is not guaranteed or endorsed by the publisher.
Abbreviations
PCP, Pneumocystis pneumonia; non-PCP, none Pneumocystis pneumonia; HIV, human immunodeficiency virus; mNGS, metagenomic Next-Generation Sequencing; PCR, polymerase chain reaction; ROC, receiver operating characteristic; AUC, areas under the ROC curve; BALF, bronchoalveolar lavage fluid; Cis, confidence intervals; B, coefficient; S.E., standard error; OR, odds ratio; CIs, confidence intervals; APTT, activated partial thromboplastin time; AST, aspartate aminotransferase.
References
Chang, C., Wang, H., Zhang, L., Hao, J., Wang, X., Wang, Y., et al. (2023). Clinical efficiency of metagenomic next-generation sequencing in sputum for pathogen detection of patients with pneumonia according to disease severity and host immune status. Infect. Drug Resist. 16, 5869–5885. doi: 10.2147/IDR.S419892
Chiu, C. Y., Miller, S. A. (2019). Clinical metagenomics. Nat. Rev. Genet. 20, 341–355. doi: 10.1038/s41576-019-0113-7
Delliere, S., Gits-Muselli, M., Bretagne, S., Alanio, A. (2020). Outbreak-causing fungi: pneumocystis jirovecii. Mycopathologia 185, 783–800. doi: 10.1007/s11046-019-00408-w
Donnelly, J. P., Chen, S. C., Kauffman, C. A., Steinbach, W. J., Baddley, J. W., Verweij, P. E., et al. (2020). Revision and update of the consensus definitions of invasive fungal disease from the European organization for research and treatment of cancer and the mycoses study group education and research consortium. Clin. Infect. Dis. 71, 1367–1376. doi: 10.1093/cid/ciz1008
Faure, E., Lionet, A., Kipnis, E., Noel, C., Hazzan, M. (2017). Risk factors for Pneumocystis pneumonia after the first 6 months following renal transplantation. Transpl Infect. Dis. 19. doi: 10.1111/tid.12735
Georges, O., Risso, K., Lemiale, V., Schlemmer, F. (2020). The place of bronchoalveolar lavage in the diagnosis of pneumonia in the immunocompromised patient. Rev. Mal Respir. 37, 652–661. doi: 10.1016/j.rmr.2020.06.016
Guegan, H., Robert-Gangneux, F. (2019). Molecular diagnosis of Pneumocystis pneumonia in immunocompromised patients. Curr. Opin. Infect. Dis. 32, 314–321. doi: 10.1097/QCO.0000000000000559
Hosseini-Moghaddam, S. M., Krishnan, R. J., Guo, H., Kumar, D. (2018). Cytomegalovirus infection and graft rejection as risk factors for pneumocystis pneumonia in solid organ transplant recipients: A systematic review and meta-analysis. Clin. Transplant. 32, e13339. doi: 10.1111/ctr.13339
Huang, L., Xu, S., Huang, Z., Chen, Y., Xu, N., Xie, B. (2023). Risk factors associated with Pneumocystis jirovecii pneumonia in non-HIV immunocompromised patients and co-pathogens analysis by metagenomic next-generation sequencing. BMC Pulm Med. 23, 72. doi: 10.1186/s12890-022-02300-8
Huang, M. Q., Zheng, T. T., Wang, X. R., Xiang, F. (2024). The clinical value of metagenomic next-generation sequencing in pneumocystis jirovecii pneumonia. Infect. Drug Resist. 17, 69–80. doi: 10.2147/IDR.S444571
Jiang, J., Bai, L., Yang, W., Peng, W., An, J., Wu, Y., et al. (2021). Metagenomic next-generation sequencing for the diagnosis of pneumocystis jirovecii pneumonia in non-HIV-infected patients: A retrospective study. Infect. Dis. Ther. 10, 1733–1745. doi: 10.1007/s40121-021-00482-y
Lagrou, K., Chen, S., Masur, H., Viscoli, C., Decker, C. F., Pagano, L., et al. (2021). Pneumocystis jirovecii disease: basis for the revised EORTC/MSGERC invasive fungal disease definitions in individuals without human immunodeficiency virus. Clin. Infect. Dis. 72, S114–S120. doi: 10.1093/cid/ciaa1805
Lee, S. H., Huh, K. H., Joo, D. J., Kim, M. S., Kim, S. I., Lee, J., et al. (2017). Risk factors for Pneumocystis jirovecii pneumonia (PJP) in kidney transplantation recipients. Sci. Rep. 7, 1571. doi: 10.1038/s41598-017-01818-w
Liu, B., Totten, M., Nematollahi, S., Datta, K., Memon, W., Marimuthu, S., et al. (2020). Development and Evaluation of a Fully Automated Molecular Assay Targeting the Mitochondrial Small Subunit rRNA Gene for the Detection of Pneumocystis jirovecii in Bronchoalveolar Lavage Fluid Specimens. J. Mol. Diagn. 22, 1482–1493. doi: 10.1016/j.jmoldx.2020.10.003
Liu, C. J., Lee, T. F., Ruan, S. Y., Yu, C. J., Chien, J. Y., Hsueh, P. R. (2019). Clinical characteristics, treatment outcomes, and prognostic factors of Pneumocystis pneumonia in non-HIV-infected patients. Infect. Drug Resist. 12, 1457–1467. doi: 10.2147/IDR.S199761
Lu, X., Zhang, J., Ma, W., Xing, L., Ning, H., Yao, M. (2022). Pneumocystis jirovecii pneumonia diagnosis via metagenomic next-generation sequencing. Front. Med. (Lausanne) 9. doi: 10.3389/fmed.2022.812005
McDonald, E. G., Afshar, A., Assiri, B., Boyles, T., Hsu, J. M., Khuong, N., et al. (2024). Pneumocystis jirovecii pneumonia in people living with HIV: a review. Clin. Microbiol. Rev. 37, e0010122. doi: 10.1128/cmr.00101-22
Morilla, R., Medrano, F. J., Calzada, A., Quintana, E., Campano, E., Friaza, V., et al. (2021). Pneumocystis jirovecii among patients with cystic fibrosis and their household members. Med. Mycol 59, 849–854. doi: 10.1093/mmy/myab010
Nahm, F. S. (2022). Receiver operating characteristic curve: overview and practical use for clinicians. Korean J. Anesthesiol 75, 25–36. doi: 10.4097/kja.21209
Niu, J., Wang, J., Jia, P., Zhang, M., Wei, E. (2023). Clinical features and diagnostic value of metagenomic next -generation sequencing in five cases of non-HIV related Pneumocystis jirovecii pneumonia in children. Front. Cell Infect. Microbiol. 13. doi: 10.3389/fcimb.2023.1132472
Wang, J., Han, Y., Feng, J. (2019). Metagenomic next-generation sequencing for mixed pulmonary infection diagnosis. BMC Pulm Med. 19, 252. doi: 10.1186/s12890-019-1022-4
Wang, C., You, Z., Fu, J., Chen, S., Bai, D., Zhao, H., et al. (2022). Application of metagenomic next-generation sequencing in the diagnosis of pulmonary invasive fungal disease. Front. Cell Infect. Microbiol. 12. doi: 10.3389/fcimb.2022.949505
Zhang, Y., Ai, J. W., Cui, P., Zhang, W. H., Wu, H. L., Ye, M. Z. (2019). A cluster of cases of pneumocystis pneumonia identified by shotgun metagenomics approach. J. Infect. 78, 158–169. doi: 10.1016/j.jinf.2018.08.013
Zhang, F., Chen, J., Huang, H., Deng, X., Zhang, W., Zeng, M., et al. (2021). Application of metagenomic next-generation sequencing in the diagnosis and treatment guidance of Pneumocystis jirovecii pneumonia in renal transplant recipients. Eur. J. Clin. Microbiol. Infect. Dis. 40, 1933–1942. doi: 10.1007/s10096-021-04254-x
Keywords: pneumocystis pneumonia, metagenomic next-generation sequencing, receiver operating characteristic curve, area under the curve, pediatric
Citation: Zhang C, Li Z, Chen X, Wang M, Yang E, Xu H and Wang S (2024) Risk factors for identifying pneumocystis pneumonia in pediatric patients. Front. Cell. Infect. Microbiol. 14:1398152. doi: 10.3389/fcimb.2024.1398152
Received: 09 March 2024; Accepted: 25 September 2024;
Published: 23 October 2024.
Edited by:
Li Ang, First Affiliated Hospital of Zhengzhou University, ChinaReviewed by:
Benjamin M. Liu, George Washington University, United StatesFrancisco Antunes, University of Lisbon, Portugal
Copyright © 2024 Zhang, Li, Chen, Wang, Yang, Xu and Wang. This is an open-access article distributed under the terms of the Creative Commons Attribution License (CC BY). The use, distribution or reproduction in other forums is permitted, provided the original author(s) and the copyright owner(s) are credited and that the original publication in this journal is cited, in accordance with accepted academic practice. No use, distribution or reproduction is permitted which does not comply with these terms.
*Correspondence: Shifu Wang, d3NoZnU3MDlAMTYzLmNvbQ==
†These authors have contributed equally to this work and share first authorship