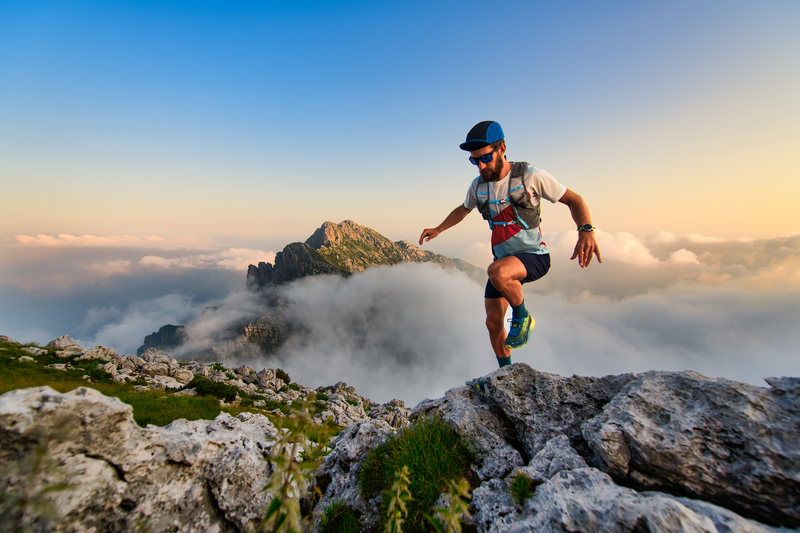
94% of researchers rate our articles as excellent or good
Learn more about the work of our research integrity team to safeguard the quality of each article we publish.
Find out more
ORIGINAL RESEARCH article
Front. Cell. Infect. Microbiol. , 18 July 2024
Sec. Clinical Microbiology
Volume 14 - 2024 | https://doi.org/10.3389/fcimb.2024.1386201
This article is part of the Research Topic Rising Stars in Clinical Microbiology: 2023 View all 5 articles
Objective: To explore the underlying mechanisms the airway microbiome contributes to Acute Exacerbation of Chronic Obstructive Pulmonary Disease(AECOPD).
Methods: We enrolled 31 AECOPD patients and 26 stable COPD patients, their sputum samples were collected for metagenomic and RNA sequencing, and then subjected to bioinformatic analyses. The expression of host genes was validated by Quantitative Real-time PCR(qPCR) using the same batch of specimens.
Results: Our results indicated a higher expression of Rothia mucilaginosa(p=0.015) in the AECOPD group and Haemophilus influenzae(p=0.005) in the COPD group. The Different expressed genes(DEGs) detected were significantly enriched in "type I interferon signaling pathway"(p<0.001, q=0.001) in gene function annotation, and "Cytosolic DNA-sensing pathway"(p=0.002, q=0.024), "Toll-like receptor signaling pathway"(p=0.006, q=0.045), and "TNF signaling pathway"(p=0.006, q=0.045) in KEGG enrichment analysis. qPCR amplification experiment verified that the expression of OASL and IL6 increased significantly in the AECOPD group.
Conclusion: Pulmonary bacteria dysbiosis may regulate the pathogenesis of AECOPD through innate immune system pathways like type I interferon signaling pathway and Toll-like receptor signaling pathway.
Chronic Obstructive Pulmonary Disease (COPD) is a heterogeneous disease underpinned by persistent airflow restriction and reacted airway inflammation in response to harmful exposure, resulting in a progressive decline in lung function and respiratory symptoms (Christenson et al., 2022). While inflammation, alpha1-antitrypsin deficiency, and oxidative stress response have long been considered major pathogenic factors of COPD (Barnes, 2020), novel research elucidated that airway microbiome dysbiosis is a critical factor as well (Zuo et al., 2015; Wang et al., 2016).
The lower airway microbiome structure bears difference to stable-stage COPD when COPD is aggravated, usually manifested as a decline in microbial abundance and diversity. Sputum analysis of AECOPD patients usually described the elevated abundance of Proteobacteria, Haemophilus, and Staphylococcus are presented as aggravation factor, Veillonella was described as protective factor (Mayhew et al., 2018; Wang et al., 2018; Leitao et al., 2019; Dicker et al., 2021; Su et al., 2022), but the relative abundance of Firmicutes in the acute stage contradicted in some studies (Wang et al., 2010; Huang and Boushey, 2015). No comprehensive conclusion relating the pulmonary microbiome in AECOPD has been reached so far.
Besides, microbiome-host interaction might play a crucial role in the pathogenesis of AECOPD (Huang et al., 2014). The expression of host genes concerning inflammation pathways and immune response, especially macrophage, were increased in AECOPD patients (Yang et al., 2022). In vivo and in vitro studies conducted by Yan Z et al., indole-3-acetic acid derived by airway microbiome, especially lactobacilli, mitigates neutrophilic inflammation, emphysema, apoptosis, and lung function decline in COPD patients (Yan et al., 2022). Furthermore, a multi-omic meta-analysis deduced that airway microbiota promoted the biosynthesis of palmitate, homocysteine, and urate in COPD. These compounds enhanced the airway inflammation through the activation of pro-inflammatory agents (Wang et al., 2020).
While the aforementioned research presented notable findings, their sample size is limited. Also, there exists a deficiency in the identification of host genes when COPD patients experienced exacerbation. A larger qualified patients were recruited and categorized into the Acute Exacerbation of COPD group (AECOPD group) and stable-stage COPD group (COPD group) in our protocol. Their sputum specimens were collected for metagenomics Next Generation Sequencing (mNGS) and RNA sequencing, to explore the potential mechanisms by which airway microbiome contributes to AECOPD and prepare for further research and intervention.
This study recruited a total of 57 COPD patients in Huai'an First People's Hospital (inpatient or outpatient), of which 31 patients underwent exacerbation and 26 patients remained stable in the past 6 months. All participants provided consent and underwent thorough gargling procedures thrice using 10ml of 0.9% normal saline each time, before collecting sputum samples following a full rinse of their oral cavities and posterior pharynx wall. These samples were sent to the laboratory for storage in the refrigerator at -80°C for further sequencing within 2 hours. The inclusion criteria were listed: (1) all recruiters required a post-bronchodilator FEV1/FVC ratio that is less than 0.70, an exposure history of risk factors and/or clinical symptoms (Labaki and Rosenberg, 2020); (2) stable COPD patients means that the symptoms of cough, phlegm or wheezing were stable in 6 months; (3) no systemic steroids or antibiotics use in the past 2 months (Faner et al., 2017); (4) a smoking history or current smokers. Patients complicated with other respiratory tract infections, systematic inflammatory diseases, immunodeficiency or malignant tumor were excluded. All the informed consent forms were signed before the specimens were collected. The study was compatible with the Declaration of Helsinki and was approved by the ethics committee of our hospital (Ethics Number: YX-2021–098-01).
Samples of sputum collected were liquefied by 0.1% dithiothreitol (DTT) for 20 minutes at 56°C before extraction. The Qubit (Thermo Fisher Scientific) was used for the quantity assessment of samples and NanoDrop (Thermo Fisher Scientific) for the quality assessment. The KAPA Hyper Prep kit (KAPA Biosystems) was used for the preparation of DNA libraries. The constructed DNA library was sequenced 150bp on Illumina NovaSeq 6000 (Illumina). After splitting raw sequencing data with bcl2fastq2, Trimmomatic was used to remove adapter contamination, low low-quality reads, and duplicated and shot (length<36 bp) reads for high-quality sequencing data. By using bowtie2, reads that could be mapped to human reference genome (hs37d5) were subtracted, reads that couldn't be mapped were retained for comparison with microorganism genome database (downloaded from GenBank release 238, ftp://ftp.ncbi.nlm.nih.gov/genomes/genbank/), the matching reads were obtained for further microbial identification and abundance estimating, using Kraken2 version 2.1.0 and Bracken version 2.5.0 respectively. Here we present criteria that were considered positive results of mNGS: 1. the read number≥1 at the species level for Legionella, Mycobacterium, and Legionella pneumophila; 2. non-overlapping read number≥3 at the species level for other bacteria (except for the above three in criterion 1), virus, fungi, and parasite. We generally didn't consider the pathogen in the Negative 'no-template' control (NTC) positive, unless the reads detected were more than 10 times that in the NTC.
We used Trizol regent to extract RNA in the sputum samples, RNase H to eliminate ribosomal RNA, and KAPA Stranded RNA-seq Kit with RiboErase (HMR) (KAPA Biosystems) to prepare library that was sequenced on Illumina NovaSeq NGS platforms (Illumina) afterward. We used bcl2fastq v2.19.0.316 (Illumina, Inc.) to perform base calling for the generation of sequence reads in FASTQ format (Illumina 1.8+ encoding). We used Trimmomatic (version 0.36) to perform Quality control (QC), STAR (version 2.5.3a) to map transcriptome, and RSEM (version 1.2.31) to carry out isoform and gene-level quantification. We used R packages DESeq2 (version 1.22.2) to conduct differential expression analysis and select Differentially Expressed Genes (DEGs) following the principles below: Log2 Fold Change > 2 and P value < 0.05. We used in-house R scripts to plot associated volcano plots and heatmaps, and KOBAS (version 3.0) to conduct GO and KEGG enrichment analysis.
TRNzol total RNA extraction reagent was used for sample RNA extraction; NanoDrop® ND-2000 was used to determine RNA concentration and purity; denatured agarose gel electrophoresis was used to detect RNA integrity; reverse transcription was used to synthesize cDNA; Real-Time PCR was used to detect 3 multiple pores in each sample.
Quantitative data that displayed normal distribution and homogeneity of variance was expressed as (x ± S). To compare averages between the two groups, we used a two-independent sample t-test. When the data did not display normal distribution, we used the Mann–Whitney U-test, and data was expressed as Median(M). Qualitative data were expressed as percentages. To compare the data between groups, we used the Pearson chi-square (χ2) test, and when necessary, Fisher's exact probability test (SPSS 25.0, IBM Inc). Statistical analysis was performed by R software (v4.0.1). Differential relative abundance of taxonomic groups at the genus level among groups was tested by using Kruskal-Wallis rank sum test (R package "kruskal.test"). The genera with mean relative abundances greater than 1% and penetrance greater than 40% among all samples were compared. Spearman's correlations between the clinical features and the relative abundances of genera, as well as the DEGs and the relative abundance of species were calculated by the R package "cor.test", and FDR correction was adopted to adjust all p values. The comparison of host genes used Student's t test or non-parametric test(Graphpad Prism 9).
This study recruited 31 AECOPD patients and 26 stable COPD patients, whose sputum samples were collected and analyzed to seek discrepancies in their microbial composition. The demographic data was summarized in Table 1 (original data can be found in Supplementary Material 1), with patients' age ranging from 42 to 87 years. Male smokers account for a higher proportion of all recruits. No statistical difference of age, sex, Body Mass Index(BMI), Nutritional Risk Screening(NRS) 2002, FEV1/FVC, Inhaled Corticosteroids(ICS) rate, and Exacerbation Frequency Over the Past Year was observed. Smoking Index(p=0.040), COPD Assessment Test(CAT) score(p<0.001) and mMRC questionnaire(p<0.001) increased in the AECOPD group, and FEV1(%pred) (p=0.030) decreased in the AECOPD group. GOLD classification(p=0.042) and GOLD Groups(p=0.029) differed as well.
The microbiota compositional profiles were exhibited in Figure 1. In the genus level, Fusobacterium(accounts for 0.38% in the AECOPD group vs 1.78% in the COPD group, p<0.001) and Haemophilus(5.31% vs 10.11%, p=0.007) increased in the AECOPD group, Moraxella(1.20% vs 0.20%, p=0.039), Rothia(24.07% vs 14.43%, p=0.032) and Granulicatella(1.43% vs 0.40%, p=0.018) deceased in the AECOPD group. In the species level, Rothia mucilaginosa (21.07% in the AECOPD group vs 11.41% in the COPD group, p=0.015) increased and Haemophilus influenzae (2.41% vs 5.76%, p=0.015) decreased when compared the AECOPD group to the COPD group.
Figure 1 Microbiota compositional profiles and difference analysis between the AECOPD group and the COPD group. (A, B) Depicted the abundance of microbiota with bar charts in the genus level (A) and species level (B) respectively. The y-axis represents the percentage of each bacterium. The legend represents the color of each bacterium. (C, D) Depicted the distinguished microbiota with boxplots in the genus level (C) and species level (D) respectively, *p < 0.05, **p < 0.01, ***p < 0.001.
After RNA sequencing and analysis, 2229 DEGs were selected, 2014 of them were upregulated in AECOPD and 215 of them were downregulated in AECOPD. After p-value adjustment, a total of 28 upregulated DEGs were identified in the AECOPD group (Table 2). The corresponding volcano plots and heatmaps are displayed in Figure 2. Among them, Interferon Alpha Inducible Protein 6 (IFI6) showed the largest expression difference (q<0.001), while Oligoadenylate synthase-like (OASL) (q<0.001) demonstrated the most significant variation between the two groups.
Figure 2 Differentially Expressed Genes between AECOPD and COPD patients. According to the relative abundance of DEGs in each sample, the Top 50 different DEGs were selected to draw a heatmap (A) between the AECOPD group and the COPD group. All 50 DEGs were upregulated. The color gradient and similarity degree reflect the similarity and difference of two samples at different classification levels, the warmer the tone, the greater the value. (B) Illustrated the volcano map of DEGs between AECOPD and COPD patients. Red dots, p-adjusted<0.05. Black dots, p<0.05. Bounded by Log2 Fold Change=0 on the abscissa, the left side represents down-regulated genes and the right side represents up-regulated genes.
Principal Component Analysis(PCA) analysis was carried to present the difference of microbiota structure between groups. The two groups were not significantly separated at PC1 and PC2 coordinates(p=0.067), considering that there was no significant difference in microflora deconstruction between the two groups (Figure 3A). The Venn diagram is used to visualize the number of DEGs in both groups. The two groups shared 22,466 genes, accounting for 54.7% of group AECOPD and 42.3% of group COPD (Figure 3B).
Figure 3 Comparison of microbial and transcriptional composition between groups. (A) PCA analysis of microbial data between groups. Microbiota composition bear no significant difference between the AECOPD group and the COPD group. (B) Venn map illustrating the abundance and similarities of different expressed genes.
Among the categories of "Cellular Component" (CC), "Molecular Function" (MF), and "Biological Process" (BP) in GO enrichment analysis, we observed significant enrichment of DEGs in "type I interferon signaling pathway" (p<0.001, q=0.001) and "defense response to virus" (p<0.001, q=0.023). The integrated gene annotation was presented in Figure 4A, from which we can observe the aggregation of DEGs in intracellular material production. KEGG Enrichment Analysis revealed five distinguished upregulated pathways. These pathways include "Influenza A" (p<0.001, q=0.012), "Herpes simplex infection" (p<0.001, q=0.014), "Cytosolic DNA-sensing pathway" (p=0.002, q=0.024), "Toll-like receptor signaling pathway" (p=0.006, q=0.045), and "TNF signaling pathway" (p=0.006, q=0.045), The plotdots representing these pathways are displayed in Figure 4B. Based on the aforementioned results, we produced Table 3 to integrate key pathways and related genes.
Figure 4 GO and KEGG enrichment analysis. (A) Depicted the GO term annotation of DEGs in a histogram. The x-axis represents the gene function in the GO standard. The y-axis represents the number of genes gathered, and the ratio of DEGs aggregated in this term. (B) Demonstrated a Plotdot portrayed accordingly according to the KEGG Pathway enrichment analysis of the AECOPD and COPD groups. The Y-axis represents the pathway of each molecule to play a function. The X-axis means Gene Ratio, which is the proportion of genes enriched to the target pathway gene in the gene list. Bubble area size means the number of enriched genes. Bubble color means enrichment significance, that is, the size of the q value.
According to the RNA isolation results and DEG analysis, we used Quantitative Real-time PCR(qPCR) to quantify the gene expression of OASL, IL6, IFI35, IFI6 and CXCL10 in each sample. Figure 5 was plot after gene quantification analysis and discriminant analysis. The expression of IL6(p<0.001) and OASL(p=0.003) were significantly elevated in the AECOPD group. IFI35(p=0.31), IFI6(p=0.16) and CXCL10(p=0.06) demonstrated no difference. Original data can be found in Supplementary Material 2.
Figure 5 Relative quantification of DEGs by qPCR and gene expression comparison analysis. The horizontal coordinate of the column bar graph represents the grouping, and the vertical coordinate represents the relative quantitative data of each gene. Student's t test or non-parametric test were used for comparison between the two groups. ***p<0.001, **p<0.01. (A) IL6. (B) OASL. (C) IFI35. (D) IFI6. (E) CXCL10.
Spearman's rank correlation coefficient was calculated between related clinical indicators and DEGs, revealing that the pseudogene "RP11–497H16.4" exhibited a positive correlation with CAT (r=0.40, p<0.01), mMRC (r=0.37, p<0.01), and GOLD (r=0.32, p<0.05), as well as a negative correlation with FEV1/FVC (r=-0.32, p<0.05). Additionally, "OASL" had a positive correlation with CAT as well (r=0.34, p<0.05) (Figure 6). The original data can be found in Supplementary Material 3.
Figure 6 A heatmap plot according to the correlation of DEGs and clinical indicators. Cool tones represent a positive correlation and warm tones represent a negative correlation. The color gradient represents the degree of correlations, the darker the color, the higher the correlation. **p<0.01, *p<0.05, two tailed.
We built a correlation network in the species level in Figure 7. Entercoccus gallinarum(Bacillota phylum) and Bergeyella cardium(Bacteroidota phylum) were recognized as the core microbiome of the COPD group. Leptotrichia hofstadii(Fusobacteriota phylum) and Neisseria mucosa(Pseudomonadota phylum) were the most associated microbiome in the AECOPD group. We correlated the microbial data with the DEGs in Figure 8. The results exhibited a positive correlation in the abundance of Rothia mucilaginosa with RANGAP1 and PARP12 expression, it manifested the negative correlation in the abundance of OASL expression with Actinobacillus ureae, Fusobacterium necrophorum, Peptostreptococcus anaerobius, and Peptostreptococcus stomatis as well.
Figure 7 Microbiota network analysis. The triangle represents species originated form the SCOPD group, the circle represents species originated form the AECOPD group. The results show that different nodes represent different dominant species, and the connections between nodes indicate that there is a correlation between the two species. The stronger the correlation, the obvious connection lines, where the red line indicates a positive correlation and the blue line indicates a negative correlation. In addition, node colors are defined by gate level, and node colors are the same, indicating that these species belong to the same gate. Through the number of node connections, it is possible to identify species that are more related to other members of the flora, and then explore the biological significance of the correlation between these species.
Figure 8 Correlation analysis between different expressed genes and microbiota. Spearman correlation analysis was performed using the relative abundance of all bacteria at the species level and the DEGs. The green circle represents the DEGs, the orange circle represents the microbiota. The red line means positive correlation, and the blue line means negative correlation.
Previous study showed that Proteobacteriato-Firmicutes ratio and Prevotella were found to be easily influenced by Inhaled Corticosteroids (ICS) use (Ramsheh et al., 2021), and smoking generally resulted in an elevated abundance of Haemophilus (Hunt et al, 2020). To eliminate these interferences, we controlled the ICS use and smoking history with no statistic difference while recruitment. In our study, we presented microbial composition and host transcriptional profiles of 31 patients underwent exacerbation and 26 patients at stable stage. This is the first study to discuss the potential mechanism underlying the exacerbation of COPD, as far as we know.
The microbial compositional analysis elucidated a higher expression of Fusobacterium and Haemophilus in the stable stage COPD, and Rothia, Moraxella, and Granulicatella were more prevalent during acute exacerbation. The transcriptional profile indicated an integration of DEGs in type I interferon (IFN) signaling pathway and TLRs signaling pathway.
Type I IFNs mainly include IFN-α and IFN-β, which are important effector molecules involved in antiviral immunity. Bacterial infection induces type I IFNs as well (Gonzalez-Navajas et al., 2012). Its production is mainly induced by surface or internal receptors (such as TLRs and cGAS) on innate immune cells (mainly macrophages) upon their contact with virus-specific antigenic substances (DNA, RNA). Then it is transmitted by intracellular signaling molecules, and activated transcription factor IRF3/7 to initiate the expression of type I IFN genes. This pathway promotes the pathogenesis of COPD via miR-21/SATB1/S100A9/NF-κB axis (Kim et al., 2021).
Toll-like receptors (TLRs) are associated with microbiome-COPD interplay. TLRs are momentous mediators for pathogens to recognize exogenous pathogen-associated molecular patterns (PAMPs) and host-derived damage-associated molecular patterns (DAMPs), inducing and sustaining the inflammation caused by the microbiome in COPD selectively (Zuo et al., 2015). TLR4 acted as a pathogen recognition receptor to identify lipopolysaccharides (LPS) or endotoxins produced by gram-negative bacteria (Knobloch et al., 2011; Oliveira and Reygaert, 2023), subsequently initiates the TIR-domain-containing adapter-inducing interferon-β signaling pathway and upregulated the expression of type I IFN (De Nardo, 2015; Zuo et al., 2015; Gajanayaka et al., 2021). Haemophilus, Moraxella, and Fusobacterium all functioned in this way to some extent.
Through gene function annotation, we discovered that genes associated with type I IFN signaling pathways, such as IFI6, IFI35, OASL, OAS2 and IFIT1, were integrated in the AECOPD group. Among them, OASL was verified to upregulated in AECOPD group by qPCR experiment using the same sample. OASL is a crucial factor in the activation of type I IFNs and it was acknowledged as the product induced by virus infection, but many studies revealed the elevated expression of OASL during bacterial infection (Kutsch et al., 2008; Weiss et al., 2010). However, the mechanism of his role in bacterial infection is not clear, and scholars speculate that it may be consistent with the pathway of viral infection (Leisching et al., 2017), which is associated with the phagocytosis of macrophages. OAS2 is part of OAS family, its expression was relatively low in this study. IFI6 has been reported to exhibit antiviral activity toward the Hepatitis C Virus (HCV) (Liu et al., 2019) and the Influenza A Virus. IFI35 negatively regulates NF-κB when complexed with an N-Mye interactor (Jian et al., 2018), thus, acting negatively toward the development of COPD. Additionally, IFI35 can also promote inflammation via activating macrophages through DAMPs, making it a potential treatment therapy for COPD.
Through KEGG pathway enrichment analysis, IL6 and CXCL10 expressed higher and were integrated in pathways concerning TLRs, TNFs, and Cytosolic DNA-sensing. qPCR amplification experiment validated that the expression of IL6 resembled significant increasement in the AECOPD group than the stable COPD group. Previous study suggested the negative correlation between IL6 expression and pulmonary function (Zhu et al., 2022). Besides, IL6 was related to bacterial infection and inflammation response (Tang et al., 2012; Zhu et al., 2022), correlated IL6ST was reported to elevate when COPD aggravates (Ko et al., 1988). However, no specific study in relation to COPD pathogenesis and IL6 expression has been done so far. It needs to be further explored for the intervention value. CXCL10 is involved in a wide variety of processes during pathogen invasion and has been reported to modulate an individual's susceptibility to COPD (Wang et al., 2018). Inhibited CXCL10 protects against COPD progression by reducing the secretion of inflammatory factors (Jing et al., 2018; Ju and He, 2021). Therefore, inhibiting type I IFNs and upstream inflammatory factors could potentially postpone COPD progression. We also found a certain positive relationship between OAS2, CXCL10, and herpes simplex infection.
This study identified several other host genes. CHPF encodes a protein that mainly functions in the chondroitin sulfate biosynthetic process, which is strongly correlated with matrix metalloproteinases, a proteinase that contributes to airway remodeling in COPD (Papakonstantinou et al., 2016). Studies have shown that MAFB participation in the pathophysiology of COPD affects the maturation and differentiation of macrophages and generates MMPs, ultimately leading to aggravated pulmonary emphysema and airflow restriction (Sato et al., 2011; Aida et al., 2014). The protein encoded by ST14 is an epithelial-derived, integral membrane serine protease, while MT2A is a member of the metallothionein family and acts as an antioxidant in vivo. No research related to COPD and these genes has been carried out so far. Genes detected by microbiome-host analysis could have the potential to screen those who were susceptible to pathogen infections and intervene at an early stage.
Our research identified an unprocessed pseudogene, RP11–497H16.4, which was remarkably elevated and strongly associated with many clinical assessment indicators. This 1180 bp length gene has no protein transcripted and possibly originated from sequence changes after gene duplication.
H.influenzae, which belongs to the phyla proteobacteria, has been reported to act as an opportunistic pathogen by inducing a neutrophilic-mediated inflammation via the activation of PAMPs and the inflammatory cascade that follows (Bafadhel et al., 2015; Barker et al., 2015; Brightling and Greening, 2019). This process recruited both innate and adaptive immune cells, like macrophages, endothelial and epithelial cells (Sidletskaya et al., 2020). Furthermore, CD40, CD83, and CD86 expressed in monocyte-derived cells have also been found to be involved (Larsen et al., 2012; Oliveira and Reygaert, 2023). Haemophilus was commonly considered to be one of the initiating pathogens of type I IFNs (Lu et al., 2018; Yang et al., 2019) via TLRs. Moraxella was acknowledged as an inducement (Ramsheh et al., 2021) and a major factor (Wang et al., 2019) of AECOPD. As a gram-negative bacteria, it can induce TLR2-initiated inflammatory responses generated by pulmonary epithelial cells (Slevogt et al., 2008). Our research also found elevated levels of Moraxella in the AECOPD group. However, previous scholars were prone to a negative linear relationship between the expression level of type I IFNs production and the abundance of airway microbiota. Yoshihiko et al (Raita and Eur, 2022). deduced that a higher proportion of Haemophilus colonized in the nasopharynx was closely related to downregulated type I IFNs expression, which is consistent with our research observation. Wang Z et al (Wang et al., 2019). recognized Haemophilus as a stable stage colonizer as well. Haemophilus was thought to be an inducement of AECOPD before, now we think comprehensive research is needed in the future.
Elimination of type I IFN signaling was also found to improve clearance and survival following secondary bacterial pneumonia (Tian et al., 2012). However, Klaile E et al (Klaile et al., 2013). stressed that type I IFN could reduce the inflammation responses by enhancing the expression of The Carcinoembryonic Antigen-related Cell Adhesion Molecules (CEACAM) 1 due to negative interactions between Moraxella and CEACAM1. These research findings align with the hypothesis that type I IFNs are protective in acute viral infections but can have either protective or deleterious roles in bacterial infections (Trinchieri, 2010). We suppose that the viral-bacterial co-infection via type I IFNs could exhibit a competitive relationship, and it often occurs in the early stage of exacerbation in COPD. Once the damage has been caused by a virus infection, it tends to evolve into a Synergistic relationship. Unfortunately, our study did not analyze the viral load of research samples. However, through KEGG enrichment analysis, it is not difficult to find that microbe-host differential genes were enriched in viral infection pathways such as influenza A Virus and Herpes Simplex Virus.
The co-infection of viruses and bacteria has been acknowledged as a synergistic effect that correlates with the severity of COPD (Wilkinson et al., 2006; George et al., 2014). Preceding or concurrent viral respiratory tract infection can predispose to secondary bacterial co-infection via damaging the airway and dysregulating immune responses (Bakaletz, 2017). These infections can also induce neutrophil elastase, which can cleave the antimicrobial peptides SLPI and elafin (Mallia et al., 2012).
R.mucilaginosa is a normal flora of the oropharynx and is often detected in induced sputa samples. It has been reported to have an inhibitory effect on pathogen- or lipopolysaccharide-induced pro-inflammatory response via inhibiting NF-κB pathway activation and negatively correlated with pro-inflammatory markers (IL-8, IL-1β) and MMPs (Rigauts et al., 2022; Melo-Dias et al., 2023). Additionally, Rothia triggered a Th17 immune response and reduced the frequency of exacerbation in COPD patients as well (Ren et al., 2018). Rothia was observed to be significantly elevated in the AECOPD group but reduced in the stable COPD group in our research. Based on the anti-inflammatory effect of Rothia and our results, we suppose that Rothia may increase the bacterial load and play an anti-inflammatory role in the acute exacerbation of COPD through some potential mechanisms that need to be further explored. Therefore, Rothia may present itself as a potential biologic therapy to intervene in stable COPD patients, thus reducing the number of acute exacerbations and improving long-term prognosis.
Microbiota network analysis identified a few species that play central roles in microbial interactions, yet the abundance detected was low, and no statistical significance was observed after calculation as well. A multi-center or longitudinal analysis would be more suitable under this situation.
However, some drawbacks to our experiment must be acknowledged. Firstly, ICS can affect respiratory microflora over a long period. Although we controlled for no statistical difference in the history of ICS use between AECOPD and stable COPD, the type and dorse of ICS still need to be discussed. Secondly, although we obtained a rough estimate of viral load in our samples, further analysis relating to the virus load could refine our study. Thirdly, though the participants were all smokers, but the smoking index needs to stratify to explore the influence concerning smoking degree or smoking status(current or past).
The original data are available in NLM (nih.gov): PRJNA1022832.
The studies involving humans were approved by Huaian First People's Hospital: YX-2021-098-01. The studies were conducted in accordance with the local legislation and institutional requirements. The participants provided their written informed consent to participate in this study.
YL: Formal analysis, Methodology, Project administration, Writing – original draft. XM: Data curation, Methodology, Writing – review & editing. PS: Data curation, Writing – review & editing. ZW: Resources, Writing – review & editing. DY: Investigation, Writing – review & editing. TM: Data curation, Writing – review & editing. BW: Visualization, Writing – review & editing. JPW: Software, Writing – review & editing. JJW: Funding acquisition, Writing – review & editing. RZ: Conceptualization, Supervision, Writing – review & editing.
The author(s) declare financial support was received for the research, authorship, and/or publication of this article. This work was supported by the National Natural Science Foundation of China (grant number: No.82100063).
We thank all the patients for participating in this study. We thank all the colleagues in the pulmonary function examination room for the assessment of the enrolled patients. We thank all the colleagues in the laboratory for their guidance on the way to collect sputum. We thank all our colleagues for helping us collect patient information. We thank Dinfectome Medical Technology (Nanjing) Co., Ltd for the bioinformatics help in this study. We thank the National Natural Science Foundation of China (No.82100063) for financial aid. We thank Research Square for the preprint service. We also thank the reviewers for their comments on the manuscript.
The authors declare that the research was conducted in the absence of any commercial or financial relationships that could be construed as a potential conflict of interest.
All claims expressed in this article are solely those of the authors and do not necessarily represent those of their affiliated organizations, or those of the publisher, the editors and the reviewers. Any product that may be evaluated in this article, or claim that may be made by its manufacturer, is not guaranteed or endorsed by the publisher.
The Supplementary Material for this article can be found online at: https://www.frontiersin.org/articles/10.3389/fcimb.2024.1386201/full#supplementary-material
Supplementary Material 1 | Original data of recruiters
Supplementary Material 2 | Original data of qPCR, including amplification curve and dissolution curve
Supplementary Material 3 | Correlation analysis between DEGs and clinical markers
Aida, Y., Shibata, Y., Abe, S., Inoue, S., Kimura, T., Igarashi, A., et al. (2014). Inhibition of elastase-pulmonary emphysema in dominant-negative MafB transgenic mice. Int. J. Biol. Sci. 10, 882–894. doi: 10.7150/ijbs.8737
Bafadhel, M., Haldar, K., Barker, B., Patel, H., Mistry, V., Barer, M. R., et al. (2015). Airway bacteria measured by quantitative polymerase chain reaction and culture in patients with stable COPD: relationship with neutrophilic airway inflammation, exacerbation frequency, and lung function. Int. J. Chron Obstruct Pulmon Dis. 10, 1075–1083. doi: 10.2147/COPD
Bakaletz, L. O. (2017). Viral-bacterial co-infections in the respiratory tract. Curr. Opin. Microbiol. 35, 30–35. doi: 10.1016/j.mib.2016.11.003
Barker, B. L., Haldar, K., Patel, H., Pavord, I. D., Barer, M. R., Brightling, C. E., et al. (2015). Association between pathogens detected using quantitative polymerase chain reaction with airway inflammation in COPD at stable state and exacerbations. Chest 147, 46–55. doi: 10.1378/chest.14-0764
Barnes, P. J. (2020). COPD 2020: new directions needed. Am. J. Physiol. Lung Cell Mol. Physiol. 319, L884–L886. doi: 10.1152/ajplung.00473.2020
Brightling, C., Greening, N. (2019). Airway inflammation in COPD: progress to precision medicine. Eur. Respir. J. 54 (2). doi: 10.1183/13993003.00651-2019
Christenson, S. A., Smith, B. M., Bafadhel, M., Putcha, N. (2022). Chronic obstructive pulmonary disease. Lancet 399, 2227–2242. doi: 10.1016/S0140-6736(22)00470-6
De Nardo, D. (2015). Toll-like receptors: Activation, signalling and transcriptional modulation. Cytokine 74, 181–189. doi: 10.1016/j.cyto.2015.02.025
Dicker, A. J., Huang, J. T.J., Lonergan, M., Keir, H. R., Fong, C. J., Tan, B., et al. (2021). The sputum microbiome, airway inflammation, and mortality in chronic obstructive pulmonary disease. J. Allergy Clin. Immunol. 147, 158–167. doi: 10.1016/j.jaci.2020.02.040
Faner, R., Sibila, O., Agusti, A., Bernasconi, E., Chalmers, J. D., Huffnagle, G. B., et al. (2017). The microbiome in respiratory medicine: current challenges and future perspectives. Eur. Respir. J. 49 (4). doi: 10.1183/13993003.02086-2016
Gajanayaka, N., Dong, S. X . M., Ali, H., Iqbal, S., Mookerjee, A., Lawton, D. A., et al. (2021). TLR-4 Agonist Induces IFN-gamma Production Selectively in Proinflammatory Human M1 Macrophages through the PI3K-mTOR- and JNK-MAPK-Activated p70S6K Pathway. J. Immunol. 207, 2310–2324. doi: 10.4049/jimmunol.2001191
George, S. N., Garcha, D. S., Mackay, A. J., Patel, A. R.C., Singh, R., Sapsford, R. J., et al. (2014). Human rhinovirus infection during naturally occurring COPD exacerbations. Eur. Respir. J. 44, 87–96. doi: 10.1183/09031936.00223113
Gonzalez-Navajas, J. M., Lee, J., David, M., Raz, E. (2012). Immunomodulatory functions of type I interferons. Nat. Rev. Immunol. 12, 125–135. doi: 10.1038/nri3133
Huang, Y. J., Boushey, H. A. (2015). The sputum microbiome in chronic obstructive pulmonary disease exacerbations. Ann. Am. Thorac. Soc. 12 Suppl 2, S176–S180. doi: 10.1513/AnnalsATS.201506-319AW
Huang, Y. J., Sethi, S., Murphy, T., Nariya, S., Boushey, H. A., Lynch, S. V. (2014). Airway microbiome dynamics in exacerbations of chronic obstructive pulmonary disease. J. Clin. Microbiol. 52, 2813–2823. doi: 10.1128/JCM.00035-14
Hunt, B. C., Stanford, D., Xu, X., Li, J., Gaggar, A., Rowe, S. M., et al. (2020). Haemophilus influenzae persists in biofilm communities in a smoke-exposed ferret model of COPD. ERJ Open Res. 6 (3). doi: 10.1183/23120541.00200-2020
Jian, D., Wang, W., Zhou, X., Jia, Z., Wang, J., Yang, M., et al. (2018). Interferon-induced protein 35 inhibits endothelial cell proliferation, migration and re-endothelialization of injured arteries by inhibiting the nuclear factor-kappa B pathway. Acta Physiol. (Oxf) 223, e13037. doi: 10.1111/apha.13037
Jing, H., Liu, L., Zhou, J., Yao, H. (2018). Inhibition of C-X-C motif chemokine 10 (CXCL10) protects mice from cigarette smoke-induced chronic obstructive pulmonary disease. Med. Sci. Monit 24, 5748–5753. doi: 10.12659/MSM.909864
Ju, J., He, Y. (2021). PRMT5 promotes inflammation of cigarette smoke extract-induced bronchial epithelial cells by up-regulation of CXCL10. Allergol Immunopathol. (Madr) 49, 131–136. doi: 10.15586/aei.v49i5.482
Kim, R. Y., Sunkara, K. P., Bracke, K. R., Jarnicki, A. G., Donovan, C., Hsu, A. C., et al. (2021). A microRNA-21-mediated SATB1/S100A9/NF-kappaB axis promotes chronic obstructive pulmonary disease pathogenesis. Sci. Transl. Med. 13, eaav7223. doi: 10.1126/scitranslmed.aav7223
Klaile, E., Klassert, T. E., Scheffrahn, I., Mueller, M. M., Heinrich, A., Heyl, K. A., et al. (2013). Carcinoembryonic antigen (CEA)-related cell adhesion molecules are co-expressed in the human lung and their expression can be modulated in bronchial epithelial cells by non-typable Haemophilus influenzae, Moraxella catarrhalis, TLR3, and type I and II interferons. Respir. Res. 14, 85. doi: 10.1186/1465-9921-14-85
Knobloch, J., Schild, K., Jungck, D., Urban, K., Mueller, K., Schweda, E. K. H., et al. (2011). The T-helper cell type 1 immune response to gram-negative bacterial infections is impaired in COPD. Am. J. Respir. Crit. Care Med. 183, 204–214. doi: 10.1164/rccm.201002-0199OC
Ko, G. N., Jimerson, D. C., Wyatt, R. J., Bigelow, L. B. (1988). Plasma 3-methoxy-4-hydroxyphenylglycol changes associated with clinical state and schizophrenic subtype. Arch. Gen. Psychiatry 45, 842–846. doi: 10.1001/archpsyc.1988.01800330072009
Kutsch, S., Degrandi, D., Pfeffer, K. (2008). Immediate lymphotoxin beta receptor-mediated transcriptional response in host defense against L. monocytogenes. Immunobiology 213, 353–366. doi: 10.1016/j.imbio.2007.10.011
Labaki, W. W., Rosenberg, S. R. (2020). Chronic obstructive pulmonary disease. Ann. Intern. Med. 173, ITC17–ITC32. doi: 10.7326/AITC202008040
Larsen, J. M., Steen-Jensen, D. B., Laursen, J. M., Sondergaard, J. N., Musavian, H. S., Butt, T. M., et al. (2012). Divergent pro-inflammatory profile of human dendritic cells in response to commensal and pathogenic bacteria associated with the airway microbiota. PloS One 7, e31976. doi: 10.1371/journal.pone.0031976
Leisching, G., Wiid, I., Baker, B. (2017). The association of OASL and type I interferons in the pathogenesis and survival of intracellular replicating bacterial species. Front. Cell Infect. Microbiol. 7, 196. doi: 10.3389/fcimb.2017.00196
Leitao, F. F., Alotaibi, N. M., Ngan, D., Tam, S., Yang, J., Hollander, Z., et al. (2019). Sputum microbiome is associated with 1-year mortality after chronic obstructive pulmonary disease hospitalizations. Am. J. Respir. Crit. Care Med. 199, 1205–1213. doi: 10.1164/rccm.201806-1135OC
Liu, X., Duan, X., Holmes, J. A., Li, W., Lee, SH., Tu, Z., et al. (2019). A long noncoding RNA regulates hepatitis C virus infection through interferon alpha-inducible protein 6. Hepatology 69, 1004–1019. doi: 10.1002/hep.30266
Lu, C., Zhang, X., Ma, C., Xu, W., Gan, L., Cui, J., et al. (2018). Nontypeable Haemophilus influenzae DNA stimulates type I interferon expression via STING signaling pathway. Biochim. Biophys. Acta Mol. Cell Res. 1865, 665–673. doi: 10.1016/j.bbamcr.2018.01.011
Mallia, P., Footitt, J., Sotero, R., Jepson, A., Contoli, M., Trujillo-Torralbo, M.-B., et al. (2012). Rhinovirus infection induces degradation of antimicrobial peptides and secondary bacterial infection in chronic obstructive pulmonary disease. Am. J. Respir. Crit. Care Med. 186, 1117–1124. doi: 10.1164/rccm.201205-0806OC
Mayhew, D., Devos, N., Lambert, C., Brown, J. R., Clarke, S. C., Kim, V. L., et al. (2018). Longitudinal profiling of the lung microbiome in the AERIS study demonstrates repeatability of bacterial and eosinophilic COPD exacerbations. Thorax 73, 422–430. doi: 10.1136/thoraxjnl-2017-210408
Melo-Dias, S., Cabral, M., Furtado, A., Souto-Miranda, S., Mendes, M. A., Cravo, J., et al. (2023). Responsiveness to pulmonary rehabilitation in COPD is associated with changes in microbiota. Respir. Res. 24, 29. doi: 10.1186/s12931-023-02339-z
Oliveira, J., Reygaert, W. C. (2023). "Gram negative bacteria, " in StatPearls [Internet]. (Treasure Island (FL): StatPearls Publishing).
Papakonstantinou, E., Klagas, I., Roth, M., Tamm, M., Stolz, D. (2016). Acute exacerbations of COPD are associated with increased expression of heparan sulfate and chondroitin sulfate in BAL. Chest 149, 685–695. doi: 10.1378/chest.14-2868
Raita, Y., Perez-Losada, M., Freishtat, RJ., Hahn, A., Castro-Nallar, E., Ramos-Tapia, I., et al. (2022). Nasopharyngeal metatranscriptome profiles of infants with bronchiolitis and risk of childhood asthma: a multicentre prospective study. Respir. J. 60 (1). doi: 10.1183/13993003.02293-2021
Ramsheh, M. Y., Haldar, K., Esteve-Codina, A., Purser, L. F., Richardson, M., Mueller-Quernheim, J., et al. (2021). Lung microbiome composition and bronchial epithelial gene expression in patients with COPD versus healthy individuals: a bacterial 16S rRNA gene sequencing and host transcriptomic analysis. Lancet Microbe 2, e300–e310. doi: 10.1016/S2666-5247(21)00035-5
Ren, L., Zhang, R., Rao, J., Xiao, Y., Zhang, Z., Yang, B., et al. (2018). Transcriptionally active lung microbiome and its association with bacterial biomass and host inflammatory status. mSystems 3 (5). doi: 10.1128/mSystems.00199-18
Rigauts, C., Aizawa, J., Taylor, S. L., Rogers, G. B., Govaerts, M., Cos, P., et al. (2022). R othia mucilaginosa is an anti-inflammatory bacterium in the respiratory tract of patients with chronic lung disease. Eur. Respir. J. 59 (5). doi: 10.1183/13993003.01293-2021
Sato, M., Shibata, Y., Kimura, T., Yamauchi, K., Abe, S., Inoue, S., et al. (2011). Immunohistochemical staining for transcription factor MafB in alveolar macrophages is correlated with spirometric measures of airflow limitation in smokers. Respirology 16, 124–130. doi: 10.1111/j.1440-1843.2010.01886.x
Sidletskaya, K., Vitkina, T., Denisenko, Y. (2020). The role of toll-like receptors 2 and 4 in the pathogenesis of chronic obstructive pulmonary disease. Int. J. Chron Obstruct Pulmon Dis. 15, 1481–1493. doi: 10.2147/COPD.S249131
Slevogt, H., Zabel, S., Opitz, B., Hocke, A., Eitel, J., N'Guessan, P. D., et al. (2008). CEACAM1 inhibits Toll-like receptor 2-triggered antibacterial responses of human pulmonary epithelial cells. Nat. Immunol. 9, 1270–1278. doi: 10.1038/ni.1661
Su, L., Qiao, Y., Luo, J., Huang, R., Li, Z., Zhang, H., et al. (2022). Characteristics of the sputum microbiome in COPD exacerbations and correlations between clinical indices. J. Transl. Med. 20, 76. doi: 10.1186/s12967-022-03278-x
Tang, Y., Liao, C., Xu, X., Song, H., Shi, S., Yang, S. (2012). Th1/Th2 cytokine profiles in G+/G- bacteremia in pediatric hematology/oncology patients. Pediatr. Blood Cancer 58, 50–54. doi: 10.1002/pbc.22692
Tian, X., Xu, F., Lung, W. Y., Meyerson, C., Ghaffari, A. A., Cheng, G., et al. (2012). Poly I:C enhances susceptibility to secondary pulmonary infections by gram-positive bacteria. PloS One 7, e41879. doi: 10.1371/journal.pone.0041879
Trinchieri, G. (2010). Type I interferon: friend or foe? J. Exp. Med. 207, 2053–2063. doi: 10.1084/jem.20101664
Wang, Y., Zhou, Q., Dong, L., Xiong, M., Jiang, H., Guo, M., et al. (2018). The effects of CXCL10 polymorphisms on COPD susceptibility. Mol. Genet. Genomics 293, 649–655. doi: 10.1007/s00438-017-1408-z
Wang, Y. P., Cheng, M.-L., Zhang, B.-F., Mu, M., Wu, J. (2010). Effects of blueberry on hepatic fibrosis and transcription factor Nrf2 in rats. World J. Gastroenterol. 16, 2657–2663. doi: 10.3748/wjg.v16.i21.2657
Wang, Z., Bafadhel, M., Haldar, K., Spivak, A., Mayhew, D., Miller, B. E., et al. (2016). Lung microbiome dynamics in COPD exacerbations. Eur. Respir. J. 47, 1082–1092. doi: 10.1183/13993003.01406-2015
Wang, Z., Singh, R., Miller, B. E., Tal-Singer, R., Van Horn, S., Tomsho, L., et al. (2018). Sputum microbiome temporal variability and dysbiosis in chronic obstructive pulmonary disease exacerbations: an analysis of the COPDMAP study. Thorax 73, 331–338. doi: 10.1136/thoraxjnl-2017-210741
Wang, Z., Maschera, B., Lea, S., Kolsum, U., Michalovich, D., Van Horn, S., et al. (2019). Airway host-microbiome interactions in chronic obstructive pulmonary disease. Respir. Res. 20, 113. doi: 10.1186/s12931-019-1085-z
Wang, Z., Yang, Y., Yan, Z., Liu, H., Chen, B., Liang, Z., et al. (2020). Multi-omic meta-analysis identifies functional signatures of airway microbiome in chronic obstructive pulmonary disease. ISME J. 14, 2748–2765. doi: 10.1038/s41396-020-0727-y
Weiss, G., Rasmussen, S., Zeuthen, L. H., Nielsen, BN., Jarmer, H., Jespersen, L., et al. (2010). Lactobacillus acidophilus induces virus immune defence genes in murine dendritic cells by a Toll-like receptor-2-dependent mechanism. Immunology 131, 268–281. doi: 10.1111/j.1365-2567.2010.03301.x
Wilkinson, T., Hurst, J. R., Perera, W. R., Wilks, M., Donaldson, G. C., Wedzicha, J. A. (2006). Effect of interactions between lower airway bacterial and rhinoviral infection in exacerbations of COPD. Chest 129, 317–324. doi: 10.1378/chest.129.2.317
Yan, Z., Chen, B., Yang, Y., Yi, X., Wei, M., Ecklu-Mensah, G., et al. (2022). Multi-omics analyses of airway host-microbe interactions in chronic obstructive pulmonary disease identify potential therapeutic interventions. Nat. Microbiol. 7, 1361–1375. doi: 10.1038/s41564-022-01196-8
Yang, S., Yin, Y., Xu, W., Zhang, X., Gao, Y., Liao, H., et al. (2019). Type I interferon induced by DNA of nontypeable Haemophilus influenza modulates inflammatory cytokine profile to promote susceptibility to this bacterium. Int. Immunopharmacol 74, 105710. doi: 10.1016/j.intimp.2019.105710
Yang, J., Zhang, Q., Zhang, J., Ouyang, Y., Sun, Z., Liu, X., et al. (2022). Exploring the change of host and microorganism in chronic obstructive pulmonary disease patients based on metagenomic and metatranscriptomic sequencing. Front. Microbiol. 13, 818281. doi: 10.3389/fmicb.2022.818281
Zhu, Q., Li, H., Zheng, S., Wang, B., Li, M., Zeng, W., et al. (2022). IL-6 and IL-10 are associated with gram-negative and gram-positive bacteria infection in lymphoma. Front. Immunol. 13, 856039. doi: 10.3389/fimmu.2022.856039
Keywords: airway microbiome, chronic obstructive pulmonary disease, innate immune system, IFN, TLR, Rothia mucilaginosa, Haemophilus influenzae
Citation: Li Y, Mao X, Shi P, Wan Z, Yang D, Ma T, Wang B, Wang J, Wang J and Zhu R (2024) Microbiome-host interactions in the pathogenesis of acute exacerbation of chronic obstructive pulmonary disease. Front. Cell. Infect. Microbiol. 14:1386201. doi: 10.3389/fcimb.2024.1386201
Received: 14 February 2024; Accepted: 24 June 2024;
Published: 18 July 2024.
Edited by:
Alessandro Russo, Magna Græcia University, ItalyReviewed by:
Mudassir Banday, Harvard University, United StatesCopyright © 2024 Li, Mao, Shi, Wan, Yang, Ma, Wang, Wang, Wang and Zhu. This is an open-access article distributed under the terms of the Creative Commons Attribution License (CC BY). The use, distribution or reproduction in other forums is permitted, provided the original author(s) and the copyright owner(s) are credited and that the original publication in this journal is cited, in accordance with accepted academic practice. No use, distribution or reproduction is permitted which does not comply with these terms.
*Correspondence: Rong Zhu, bHN6aHVyb25nQDE2My5jb20=
†These authors have contributed equally to this work and share first authorship
Disclaimer: All claims expressed in this article are solely those of the authors and do not necessarily represent those of their affiliated organizations, or those of the publisher, the editors and the reviewers. Any product that may be evaluated in this article or claim that may be made by its manufacturer is not guaranteed or endorsed by the publisher.
Research integrity at Frontiers
Learn more about the work of our research integrity team to safeguard the quality of each article we publish.