- Department of Public Health and Infectious Diseases, Section of Microbiology, University of Rome “Sapienza”, Rome, Italy
It is becoming increasingly clear that the human microbiota, also known as “the hidden organ”, possesses a pivotal role in numerous processes involved in maintaining the physiological functions of the host, such as nutrient extraction, biosynthesis of bioactive molecules, interplay with the immune, endocrine, and nervous systems, as well as resistance to the colonization of potential invading pathogens. In the last decade, the development of metagenomic approaches based on the sequencing of the bacterial 16s rRNA gene via Next Generation Sequencing, followed by whole genome sequencing via third generation sequencing technologies, has been one of the great advances in molecular biology, allowing a better profiling of the human microbiota composition and, hence, a deeper understanding of the importance of microbiota in the etiopathogenesis of different pathologies. In this scenario, it is of the utmost importance to comprehensively characterize the human microbiota in relation to disease pathogenesis, in order to develop novel potential treatment or preventive strategies by manipulating the microbiota. Therefore, this perspective will focus on the progress, challenges, and promises of the current and future technological approaches for microbiome profiling and analysis.
1 Introduction
The human organism hosts a wide variety of microorganisms, collectively known as the microbiota, capable to thrive in different bodily districts, including the gut, the skin, the lungs, the oral cavity, and the urogenital tract. Although most microorganisms (>90%) belonging to the resident microflora comes from the bacterial domain, also viruses, yeast and archaea coexist in the various sites of the human body, contributing to more than 150 times genetic information than that of the entire human genome (Grice and Segre, 2012; Hou et al., 2022).
For many years, bacterial culture has represented the primary approach for exploring the structure and function of the human microbiota, albeit with the important limitation that more than 99% of all microbes could not be cultured by standard laboratory techniques, especially a large number of anaerobic bacterial species (Rinke et al., 2013; Almeida et al., 2019). In 1996, the introduction of 16s ribosomal RNA gene polymerase chain reaction (PCR) has revolutionized the field of microbiota investigations; the first studies relied on molecular techniques such as the denaturing gradient gel electrophoresis (DGGE), the restriction fragment length polymorphism (RFLP), the terminal restriction fragment length polymorphism (TRFLP), and capillary sequencing of specific genes by Sanger’s methods (Eckburg et al., 2005; Thomas et al., 2012). These methodological approaches possessed severe challenges in addressing the complexity of the different microbial ecosystems, most importantly by showing only the presence of specific bacterial groups (Zoetendal et al., 2008; Carabeo-Pérez et al., 2019).
In this scenario, the development of metagenomic approaches based on the sequencing of the bacterial 16s rRNA gene via Next Generation Sequencing (NGS) has been, since around 2010, one of the great advances in molecular biology, allowing a better profiling of the human microbiota composition and, hence, a deeper understanding of the importance of microbiota in the etiopathogenesis of different pathologies (Carabeo-Pérez et al., 2019); indeed, in the past, most studies focused on the etiopathogenetic mechanisms underlying specific infectious agents, via in vitro, ex vivo or animal models, without acknowledging the important role of the microbiota (Di Pietro et al., 2015; Lenz and Dillard, 2018; Filardo et al., 2019b; Ansari and Yamaoka, 2022).
Thanks to advances in microbiota profiling through NGS, it is currently known that the human microbiota, also known as “the hidden organ”, possesses a pivotal role in numerous processes involved in maintaining the physiological functions of the host, such as nutrient extraction and biosynthesis of bioactive molecules, like vitamin, amino acids and lipids, interplay with the immune, endocrine, and nervous systems, namely the gut-organ axis, and resistance to the colonization of potential invading pathogens (Hanssen and Nieuwdorp, 2021; Hou et al., 2022; Saxami et al., 2023).
Given the key role of microbiota-host interplay in health and disease, it is becoming increasingly important to comprehensively characterize the composition and functional profiles of the human microbiota in relation to disease pathogenesis, in order to develop novel potential treatment or preventive strategies by manipulating the microbiota. Therefore, this perspective will focus on the progress, challenges, and promises of the current and future technological approaches for microbiome profiling and analysis.
2 Metagenomic sequencing of microbial communities
The most widely used technology for the profiling of human microbiota is the sequencing of hypervariable sub-regions of the 16s rRNA gene via NGS (Carabeo-Pérez et al., 2019). This is indeed a high-throughput sequencing technique that allows the simultaneous sequencing of a large number of short DNA fragments (150-250 base pairs) generated by amplification of a specific gene, or portion of a gene (Bonk et al., 2018). The hypervariable region, mainly the V4 or V3-V4, is considered for the characterization of bacterial taxa up to the genus level (Jones et al., 2022; Gao et al., 2023). NGS technologies include the pyrosequencing (454 Life Sciences), the massive sequencing via Illumina system, sequencing by ligation (SOLiD) and sequencing based on pH detection (Ion Torrent); amongst them, the Illumina sequencing platform has become the most extensively utilized in the field of microbiota research due to its high throughput and relatively low error rate, providing valuable contributions to the understanding of the complex interactions between the microbiota and the host (Heather and Chain, 2016; Carabeo-Pérez et al., 2019). However, the microbial profiling via metagenomic analysis of 16s rRNA gene has shown critical issues, including the unreliability of taxonomic classification at the species level, and the bias introduced by the sequencing platforms and the choice of diverse data processing software. Concerning the first aspect, different studies have highlighted that targeting one or few hypervariable sub-regions of the 16s rRNA gene may lead to the sub-optimal representation of certain bacterial species or even whole taxonomic groups, failing to capture the complex biodiversity of the human microbiota (López-Aladid et al., 2023). Moreover, the sequencing of short portions of the 16s rRNA gene hypervariable region, containing a total of 1542 base pairs, may miss relevant genetic variations outside of the sequenced area, leading to the impossibility to distinguish closely related bacterial species due to homology between sequences (Bailén et al., 2020). As for the second issue, it has been reported that the choice of different sequencing platform and data processing software might also introduce further variability, yielding differences in sample characterization depending on their accuracy, sensitivity, and error rate (Claesson et al., 2010, 2017). Similarly, the usage of different analytical pipelines applied to data processing might lead to differences in microbiota composition, as not all available pipelines include the same steps, and, to date, there is scarce literature on the advantages and disadvantages of available software packages (D’Argenio et al., 2014; Bailén et al., 2020).
In an attempt to overcome these limitations, full-length 16s rRNA gene sequencing, or whole-genome sequencing (WGS) via shotgun sequencing of all DNA fragments in a microbial community, have been developed (Johnson et al., 2019; Pérez-Cobas et al., 2020; Lin et al., 2021). In particular, the analysis of the full-length 16s rRNA gene sequence has provided evidence of additional variations at the species or strain levels, that is otherwise unseen through the regular short-read sequencing generally used in amplicon-based methods, leading to a more in-depth taxonomic classification of the human microbiota (Johnson et al., 2019; Bailén et al., 2020). For example, a higher resolution at the species level was obtained via full length 16s rRNA gene sequencing in patients with either Mycoplasma pneumoniae or unknown aetiology pneumonia, revealing high abundances of coinfections, or the presence of pathogenic bacteria like Campylobacter concisus, Solobacterium moorei, etc (Li et al., 2023b).
The WGS has, instead, enabled the identification of microbial functional genes by assembling all sequenced DNA fragments in a sample, making it possible to determine nearly the whole genetic potential of the previously uncharacterized microbial species (Pérez-Cobas et al., 2020). For example, WGS analysis has the capability to investigate, besides the composition of the microbiota, the presence of bacterial virulence factors as well as genes associated to drug-resistance; on this regard, a study has evidenced the higher abundances of adhesion, toxin, and type-III secretion system genes, alongside genes associated to tetracycline resistance, in the gut microbiota from HIV-1 positive patients taking antiretroviral therapy as compared to healthy controls (Bai et al., 2021). Furthermore, WGS has revealed the absence of drug-resistance genes in a novel marine multi-stress-tolerant aromatic yeast Pichia kudriavzevii HJ2, supporting its use as probiotic for its capability to achieve a 79.98% cholesterol removal rate, with a strong antioxidant capacity (Li et al., 2023c).
The full-length sequencing of 16s rRNA gene and the WGS has been rendered possible by the introduction of the so-called “third generation sequencing” technologies, such as in PacBio or Nanopore platforms (Carabeo-Pérez et al., 2019). Third generation sequencing provides the nucleotide sequence by reading at the single molecule level and avoiding, thus, the DNA amplification steps required for NGS technologies. By doing this, these methodological approaches have the capability to produce much longer reads than NGS methods, and to reduce the bias inherent in the PCR amplification, known to under-represent sequences from less abundant bacteria (van Dijk et al., 2018).
Long-read sequencing approaches hold the potential for improving metagenomic assembly, covering repetitive and low-coverage regions, and, hence, increasing assembly contiguity. This has facilitated the development of high-quality genome assemblies for the majority of microbes populating the human microbiota, although assembling all genomes of all bacteria within a single microbial niche remains challenging, due to the relatively shallow sequencing depth generally used in most studies (5-10 Giga base pairs, Gbp). To address this issue, the combination of long-read with short-read sequencing approaches might be a valid alternative for increasing coverage of low-abundance species, and consequently boosting assembly performance. This might enable a much more comprehensive overview of microbiota composition and, hence, a deeper understanding of the complex interactions between the different microbial communities and the host, although with the biggest disadvantage of a much higher (15-20 times more) cost than short-read sequencing approaches (Jin et al., 2022; Yue et al., 2023).
The main characteristics of long-read sequencing approaches lie in the production of a massive volume of complex data, with high throughput and multiple redundancies, making it challenging to efficiently analyse via traditional computational approaches and algorithms (Lin et al., 2021). Therefore, new bioinformatic tools are being developed, and, amongst them, machine learning (ML) has recently acquired great importance (Lin et al., 2021). ML are deep-learning algorithms able to learn from experience and discovery of data, exploring potential patterns and relationships that are difficult to infer via traditional methods (Li et al., 2022). Despite their potentials, ML models are computationally intensive, requiring more efficient computing facilities that are not often available to researchers, and are challenging to use as the primary method for analyzing metagenomic data due to the need for highly trained bioinformaticians (Frank et al., 2020); however, ML models are increasingly used in cutting-edge research and represent the path forward for investigating the growing complexity of the intricate interaction between microbiota and disease pathogenesis (Lin et al., 2021).
3 Towards a multi-omics approach
Current approaches for studying the microbiota are generally limited to only one or few aspects, typically the microbiota profiling through 16s rRNA amplicon sequencing, or through WGS via shotgun metagenomic. By contrast, the role of the human microbiota in disease pathogenesis involves a complex multi-dimensional network characterized by structural (the profiling of microbial composition) and functional (the determination of whole genetic pathways) interactions with the host, as well as by various small molecules, including metabolites, catabolites, and signal molecules, through which they interact (Chetty and Blekhman, 2024).
Indeed, it is becoming increasingly clear that the full scope of the multi-layered interplay between microorganisms and the host, affecting health outcomes, can only be revealed by integrating a multitude of different data layers along with the metagenomic analysis of the microbiome, leading to a multi-omics approach (Chetty and Blekhman, 2024). Multi-omics is defined as the integration of metagenomics with meta-transcriptomics, meta-proteomics, and metabolomics. Meta-transcriptomics can be performed via short- or long- read sequencing of mRNA, usually after filtering out highly abundant ribosomal rRNA, whereas both meta-proteomics and metabolomics data are generated via mass spectrometry. Specifically, meta-transcriptomics can provide insights into the microbial genetic pathways, by quantifying the abundance of transcripts in a sample reflecting bacterial gene expression activity (Aitmanaitė et al., 2023); Meta-proteomics is complementary to metagenomics and meta-transcriptomic since it reflects the activity of cellular translational and post-translational processes, by measuring the levels of all the proteins synthesized by the microbiota and the host, and, hence, providing insights into the functional role of microorganisms in host health (Hardouin et al., 2021); Metabolomics allow researchers to acquire a better understanding of metabolic and biochemical processes involving the microbiota and the host, by determining the levels of all small molecules in the microenvironment (Bartsch et al., 2023). An interesting application of multi-omics is described in a study on the dopamine metabolism in the marine yeast Meyerozyma guilliermondii GXDK6, by using WGS for identifying the key genes for dopamine biosynthesis, and transcriptomics as well as proteomics for showing the level of activation of the related metabolic pathway (Sun et al., 2024).
Multi-omics possess their own unique set of challenges to face, including the variability of results due to different software pipelines, and the excessive reliance on a paucity of curated and publicly available microbiome databases, limiting the information only to well-characterized microorganisms, transcripts, proteins, and metabolites (Chetty and Blekhman, 2024). However, these approaches provide more comprehensive information than the study of a single aspect, since all these datasets can generate a 360-degree view of the complex interactome between the microbiota and the host, elucidating the relationship between different cellular processes, such as, for example, between mRNA and proteins, or between different type of biomolecules synthesized by the microbiota or the host.
4 Discussion
Thanks to advanced metagenomic analysis, the composition of human microbiota has been shown as highly variable across body sites, with the gut microbiota receiving considerable interest, followed by the microbiota in other districts, like the oral cavity and the cervicovaginal microenvironment (Hou et al., 2022).
In fact, in the last decade, the gut microbiota has been regarded as the most significant microbial community in maintaining individual health; it is more complex than other microbiota niches, for instance, the cervicovaginal microbiota, and accounts for approximately 1013 bacteria; the most dominant bacterial phyla are Firmicutes (60–80%) and Bacteroidetes (20–40%), while Proteobacteria, Actinobacteria, and Fusobacteria are in the minority (Filardo et al., 2022a). The balance between obligate and facultative anaerobes is essential for the integrity and function of the intestinal epithelial barrier; certain conditions, like antibiotic treatment or unhealthy lifestyles, may alter the gut microbiota composition, named dysbiosis, leading to the onset and development of different pathologies (Naito, 2024). For example, the overgrowth of potentially harmful Proteobacteria, including Enterobacteriaceae, has been linked to the emergence of a pro-inflammatory microenvironment contributing to the pathogenesis of Inflammatory Bowel Diseases, as well as cancer and metabolic disorders like obesity, dyslipidaemia, type-2 diabetes, and non-alcoholic fatty liver disease (Jia et al., 2023; Li et al., 2023a). Specifically, it has been suggested that the relationship between the two dominant phyla, expressed as Firmicutes/Bacteroidetes ratio, may be associate to the development of metabolic diseases induced by high-fat diet (Jia et al., 2023); also, certain bacteria in the intestine, such as Escherichia coli, Campylobacter jejuni, Fusobacterium nucleatum, and Bacteroides fragilis, have been described to express toxin proteins causing intracellular reactive oxygen species accumulation and DNA damage, and contributing to colorectal carcinogenesis (Li et al., 2023a). Furthermore, changes in pH values as well as the presence of histologic alterations of the gastric mucosa, as in chronic atrophic gastritis, were also associated to increased gut dysbiosis characterized by the prevalence of oral bacteria, like Rothia mucilaginosa, Streptococcus salivarius and Granulicatella adiacens (Filardo et al., 2022b).
Beside the gut microbiota, a growing body of evidence has also been provided for the microbial community populating the oral cavity, that can be divided into multiple habitats, including saliva, tongue, subgingival/supragingival plaque, etc., harboring substantially similar microbiota populations with small scale differences due to pH, contact with hard or soft tissue surfaces, and bacterial interactions (Segata et al., 2012). The major bacteria present in the oral microbiota belong to the phyla Firmicutes, Proteobacteria, and Bacteroidetes; in dysbiosis conditions, the microbial imbalance characterized by the predominance of Porphyromonas gingivalis has been associated with periodontal diseases, including periodontitis, a chronic inflammatory condition leading to tissue destruction (Demkovych et al., 2023).
Less explored, but equally relevant for its health promoting activities, is the cervicovaginal microbiota, that harbors the simplest microbial community in the human organism; in fact, this is characterized, in healthy reproductive-age women, by the predominance of one or a few bacterial species, mainly Lactobacillus spp. (over 80%), alongside other bacteria present in much lower abundances, including Staphylococcus spp., Streptococcus spp., and Bifidobacterium spp. (France et al., 2022). The depletion of Lactobacillus spp. and the overgrowth of anaerobic bacteria belonging to the genera Gardnerella spp., Prevotella spp., Atopobium spp., etc., is well known to increase the risk for acquiring infections by sexually transmitted pathogens like Chlamydia trachomatis and Human Papilloma Virus (Filardo et al., 2019a; Kaliterna et al., 2023).
Overall, all this evidence led interesting application for the development of novel therapeutic or prevention strategies, by manipulating the microbiome with interventions like the supplementation with pre- or pro-biotics, or the fecal transplant.
However, our knowledge of the complex network of interactions between resident microorganisms and the host is still limited, due to the numerous challenges that current microbiota studies have to face. In particular, most research, due to budget restraints, are limited to a partial analysis of human microbiota from a compositional point of view, lacking information on the intricate web of gene pathways, proteins and metabolic processes that may be essential for the discovery of its etiopathogenetic role.
In the future, it will be essential to integrate multiple layers of information, from the analysis of gene transcripts to their protein products, as well as all the small molecules produced by microbial or host metabolism, in a process called multi-omics, with the aim to clearly describe the etiopathogenetic relationships between resident microorganisms and host disease conditions (Figure 1). On this regard, there are still important challenges to overcome: the need of increased availability of more advanced and comprehensive bioinformatic tools, allowing also non-bioinformaticians to extract useful information from meta-omics data, as well as a reduction in the analytical cost per sample so to increase its accessibility. Furthermore, another source of bias might be introduced by the absent standardization of sample collection from different sites, transportation, and storage, as well as by the heterogeneous methods for genomic DNA extraction, as they may introduce further variability in the quality of bacterial DNA isolation, alongside the partial removal of contaminating host DNA. Overall, improving on all these aspects will be fundamental steps to be taken for a future deep understanding of the intricate interplay between microbiome-host, potentially leading to the early diagnosis of chronic diseases for improved preventive and treatment approaches.
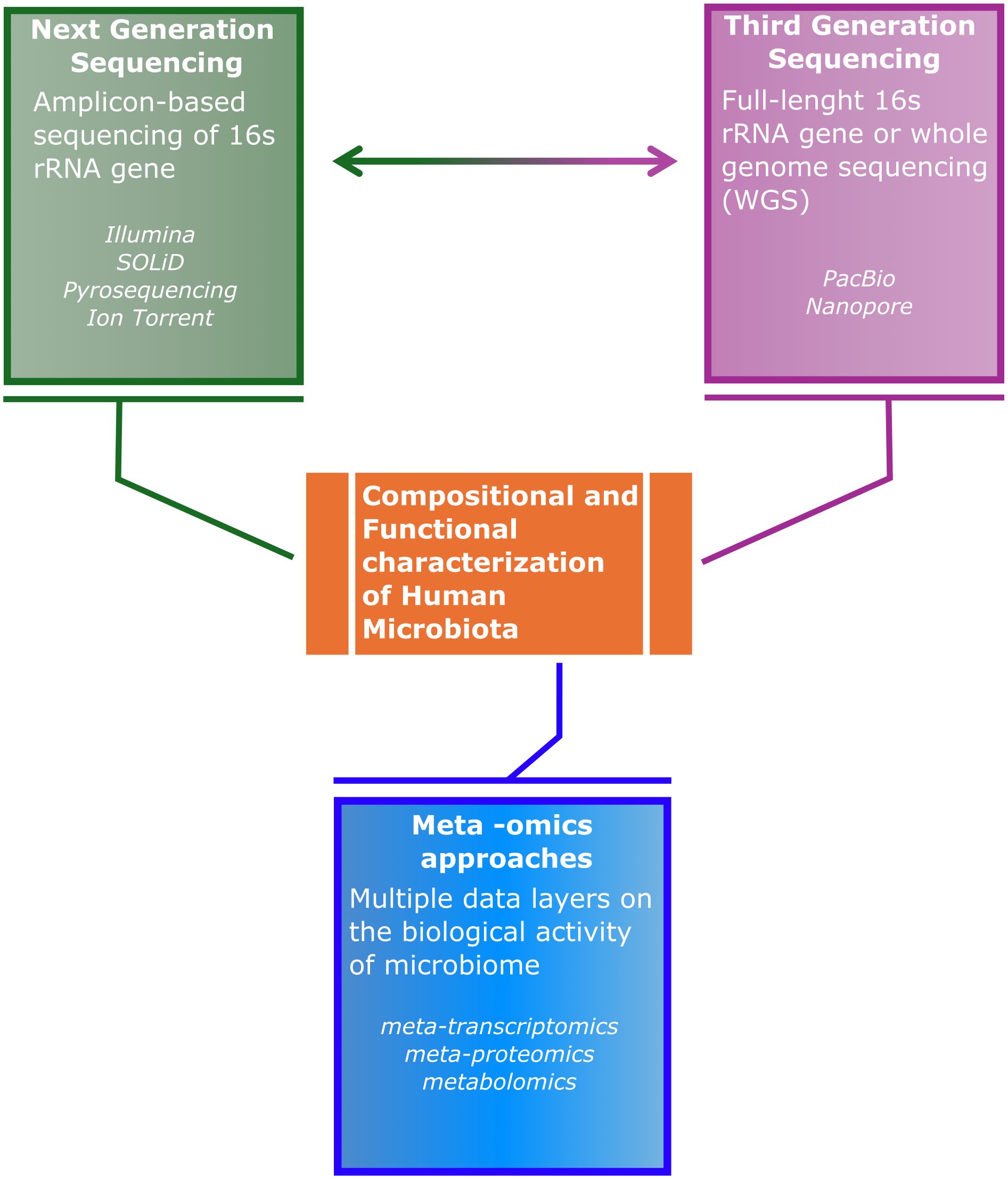
Figure 1 Schematic representation of the need for a better integration amongst Next Generation Sequencing, Third Generation Sequencing and -omics approaches, in order to reach a comprehensive characterization of the composition and functional aspect of microbiota-host interaction.
Data availability statement
The original contributions presented in the study are included in the article/supplementary material. Further inquiries can be directed to the corresponding author.
Author contributions
SF: Writing – review & editing, Writing – original draft, Conceptualization. MD: Writing – review & editing, Writing – original draft, Conceptualization. RS: Writing – review & editing, Writing – original draft, Supervision, Conceptualization.
Funding
The author(s) declare that no financial support was received for the research, authorship, and/or publication of this article. This research received no specific grant from any funding agency in the public, commercial, or not-for-profit sectors.
Conflict of interest
The authors declare that the research was conducted in the absence of any commercial or financial relationships that could be construed as a potential conflict of interest.
The author(s) declared that they were an editorial board member of Frontiers, at the time of submission. This had no impact on the peer review process and the final decision.
Publisher’s note
All claims expressed in this article are solely those of the authors and do not necessarily represent those of their affiliated organizations, or those of the publisher, the editors and the reviewers. Any product that may be evaluated in this article, or claim that may be made by its manufacturer, is not guaranteed or endorsed by the publisher.
References
Aitmanaitė, L., Širmonaitis, K., Russo, G. (2023). Microbiomes, their function, and cancer: how metatranscriptomics can close the knowledge gap. Int. J. Mol. Sci. 24, 13786. doi: 10.3390/ijms241813786
Almeida, A., Mitchell, A. L., Boland, M., Forster, S. C., Gloor, G. B., Tarkowska, A., et al. (2019). A new genomic blueprint of the human gut microbiota. Nature 568, 499–504. doi: 10.1038/s41586-019-0965-1
Ansari, S., Yamaoka, Y. (2022). Animal models and helicobacter pylori infection. J. Clin. Med. 11, 3141. doi: 10.3390/jcm11113141
Bai, X., Narayanan, A., Nowak, P., Ray, S., Neogi, U., Sönnerborg, A. (2021). Whole-genome metagenomic analysis of the gut microbiome in HIV-1-infected individuals on antiretroviral therapy. Front. Microbiol. 12. doi: 10.3389/fmicb.2021.667718
Bailén, M., Bressa, C., Larrosa, M., González-Soltero, R. (2020). Bioinformatic strategies to address limitations of 16rRNA short-read amplicons from different sequencing platforms. J. Microbiol. Methods 169, 105811. doi: 10.1016/j.mimet.2019.105811
Bartsch, M., Hahn, A., Berkemeyer, S. (2023). Bridging the gap from enterotypes to personalized dietary recommendations: A metabolomics perspective on microbiome research. Metabolites 13, 1182. doi: 10.3390/metabo13121182
Bonk, F., Popp, D., Harms, H., Centler, F. (2018). PCR-based quantification of taxa-specific abundances in microbial communities: Quantifying and avoiding common pitfalls. J. Microbiol. Methods 153, 139–147. doi: 10.1016/j.mimet.2018.09.015
Carabeo-Pérez, A., Guerra-Rivera, G., Ramos-Leal, M., Jiménez-Hernández, J. (2019). Metagenomic approaches: effective tools for monitoring the structure and functionality of microbiomes in anaerobic digestion systems. Appl. Microbiol. Biotechnol. 103, 9379–9390. doi: 10.1007/s00253-019-10052-5
Chetty, A., Blekhman, R. (2024). Multi-omic approaches for host-microbiome data integration. Gut Microbes 16, 2297860. doi: 10.1080/19490976.2023.2297860
Claesson, M. J., Clooney, A. G., O’Toole, P. W. (2017). A clinician’s guide to microbiome analysis. Nat. Rev. Gastroenterol. Hepatol. 14, 585–595. doi: 10.1038/nrgastro.2017.97
Claesson, M. J., Wang, Q., O’Sullivan, O., Greene-Diniz, R., Cole, J. R., Ross, R. P., et al. (2010). Comparison of two next-generation sequencing technologies for resolving highly complex microbiota composition using tandem variable 16S rRNA gene regions. Nucleic Acids Res. 38, e200. doi: 10.1093/nar/gkq873
D’Argenio, V., Casaburi, G., Precone, V., Salvatore, F. (2014). Comparative metagenomic analysis of human gut microbiome composition using two different bioinformatic pipelines. BioMed. Res. Int. 2014, 1–10. doi: 10.1155/2014/325340
Demkovych, A., Kalashnikov, D., Hasiuk, P., Zubchenko, S., Vorobets, A. (2023). The influence of microbiota on the development and course of inflammatory diseases of periodontal tissues. Front. Oral. Health 4. doi: 10.3389/froh.2023.1237448
Di Pietro, M., Filardo, S., De Santis, F., Mastromarino, P., Sessa, R. (2015). Chlamydia pneumoniae and oxidative stress in cardiovascular disease: State of the art and prevention strategies. Int. J. Mol. Sci. 16, 724–735. doi: 10.3390/ijms16010724
Eckburg, P. B., Bik, E. M., Bernstein, C. N., Purdom, E., Dethlefsen, L., Sargent, M., et al. (2005). Diversity of the human intestinal microbial flora. Sci. (1979) 308, 1635–1638. doi: 10.1126/science.1110591
Filardo, S., Di Pietro, M., Protano, C., Antonucci, A., Vitali, M., Sessa, R. (2022a). Impact of air pollution on the composition and diversity of human gut microbiota in general and vulnerable populations: A systematic review. Toxics 10, 579. doi: 10.3390/toxics10100579
Filardo, S., Di Pietro, M., Tranquilli, G., Latino, M. A., Recine, N., Porpora, M. G., et al. (2019a). Selected immunological mediators and cervical microbial signatures in women with chlamydia trachomatis infection. mSystems 4, e00094-19. doi: 10.1128/msystems.00094-19
Filardo, S., Scalese, G., Virili, C., Pontone, S., Di Pietro, M., Covelli, A., et al. (2022b). The potential role of hypochlorhydria in the development of duodenal dysbiosis: A preliminary report. Front. Cell Infect. Microbiol. 12. doi: 10.3389/fcimb.2022.854904
Filardo, S., Skilton, R. J., O’Neill, C. E., Di Pietro, M., Sessa, R., Clarke, I. N. (2019b). Growth kinetics of Chlamydia trachomatis in primary human Sertoli cells. Sci. Rep. 9, 5847. doi: 10.1038/s41598-019-42396-3
France, M., Alizadeh, M., Brown, S., Ma, B., Ravel, J. (2022). Towards a deeper understanding of the vaginal microbiota. Nat. Microbiol. 7, 367–378. doi: 10.1038/s41564-022-01083-2
Frank, M., Drikakis, D., Charissis, V. (2020). Machine-learning methods for computational science and engineering. Computation 8, 15. doi: 10.3390/computation8010015
Gao, J., Yi, X., Wang, Z. (2023). The application of multi-omics in the respiratory microbiome: Progresses, challenges and promises. Comput. Struct. Biotechnol. J. 21, 4933–4943. doi: 10.1016/j.csbj.2023.10.016
Grice, E. A., Segre, J. A. (2012). The human microbiome: our second genome. Annu. Rev. Genomics Hum. Genet. 13, 151–170. doi: 10.1146/annurev-genom-090711-163814
Hanssen, N. M. J., Nieuwdorp, M. (2021). The gut microbiome, a hidden endocrine organ with widespread metabolic implications? Best Pract. Res. Clin. Endocrinol. Metab. 35, 101543. doi: 10.1016/j.beem.2021.101543
Hardouin, P., Chiron, R., Marchandin, H., Armengaud, J., Grenga, L. (2021). Metaproteomics to decipher CF host-microbiota interactions: overview, challenges and future perspectives. Genes (Basel) 12, 892. doi: 10.3390/genes12060892
Heather, J. M., Chain, B. (2016). The sequence of sequencers: The history of sequencing DNA. Genomics 107, 1–8. doi: 10.1016/j.ygeno.2015.11.003
Hou, K., Wu, Z.-X., Chen, X.-Y., Wang, J.-Q., Zhang, D., Xiao, C., et al. (2022). Microbiota in health and diseases. Signal Transduct Target Ther. 7, 135. doi: 10.1038/s41392-022-00974-4
Jia, X., Chen, Q., Wu, H., Liu, H., Jing, C., Gong, A., et al. (2023). Exploring a novel therapeutic strategy: the interplay between gut microbiota and high-fat diet in the pathogenesis of metabolic disorders. Front. Nutr. 10. doi: 10.3389/fnut.2023.1291853
Jin, H., You, L., Zhao, F., Li, S., Ma, T., Kwok, L.-Y., et al. (2022). Hybrid, ultra-deep metagenomic sequencing enables genomic and functional characterization of low-abundance species in the human gut microbiome. Gut Microbes 14, 2021790. doi: 10.1080/19490976.2021.2021790
Johnson, J. S., Spakowicz, D. J., Hong, B.-Y., Petersen, L. M., Demkowicz, P., Chen, L., et al. (2019). Evaluation of 16S rRNA gene sequencing for species and strain-level microbiome analysis. Nat. Commun. 10, 5029. doi: 10.1038/s41467-019-13036-1
Jones, C. B., White, J. R., Ernst, S. E., Sfanos, K. S., Peiffer, L. B. (2022). Incorporation of Data From Multiple Hypervariable Regions when Analyzing Bacterial 16S rRNA Gene Sequencing Data. Front. Genet. 13. doi: 10.3389/fgene.2022.799615
Kaliterna, V., Kaliterna, P., Pejkovic, L., Vulic, R., Zanchi, L., Cerskov, K. (2023). Prevalence of human papillomavirus (HPV) among females in the general population of the split and dalmatia county and its association with genital microbiota and infections: A prospective study. Viruses 15, 443. doi: 10.3390/v15020443
Lenz, J. D., Dillard, J. P. (2018). Pathogenesis of neisseria gonorrhoeae and the host defense in ascending infections of human fallopian tube. Front. Immunol. 9. doi: 10.3389/fimmu.2018.02710
Li, P., Luo, H., Ji, B., Nielsen, J. (2022). Machine learning for data integration in human gut microbiome. Microb. Cell Fact 21, 241. doi: 10.1186/s12934-022-01973-4
Li, L., Ma, J., Li, M., Cheng, P., Li, M., Yu, Z., et al. (2023b). Species-level respiratory microbiome profiling for etiologic diagnosis of children pneumonia using full length 16S rRNA gene sequencing. Indian J. Med. Microbiol. 43, 11–17. doi: 10.1016/j.ijmmb.2022.09.012
Li, Y., Mo, X., Xiong, J., Huang, K., Zheng, M., Jiang, Q., et al. (2023c). Deciphering the probiotic properties and safety assessment of a novel multi-stress-tolerant aromatic yeast Pichia kudriavzevii HJ2 from marine mangroves. Food Biosci. 56, 103248. doi: 10.1016/j.fbio.2023.103248
Li, H., Wang, K., Hao, M., Liu, Y., Liang, X., Yuan, D., et al. (2023a). The role of intestinal microecology in inflammatory bowel disease and colorectal cancer: A review. Medicine 102, e36590. doi: 10.1097/MD.0000000000036590
Lin, Y., Wang, G., Yu, J., Sung, J. J. Y. (2021). Artificial intelligence and metagenomics in intestinal diseases. J. Gastroenterol. Hepatol. 36, 841–847. doi: 10.1111/jgh.15501
López-Aladid, R., Fernández-Barat, L., Alcaraz-Serrano, V., Bueno-Freire, L., Vázquez, N., Pastor-Ibáñez, R., et al. (2023). Determining the most accurate 16S rRNA hypervariable region for taxonomic identification from respiratory samples. Sci. Rep. 13, 3974. doi: 10.1038/s41598-023-30764-z
Naito, Y. (2024). Gut frailty: its concept and pathogenesis. Digestion 105, 49–57. doi: 10.1159/000534733
Pérez-Cobas, A. E., Gomez-Valero, L., Buchrieser, C. (2020). Metagenomic approaches in microbial ecology: an update on whole-genome and marker gene sequencing analyses. Microb. Genom. 6, mgen000409. doi: 10.1099/mgen.0.000409
Rinke, C., Schwientek, P., Sczyrba, A., Ivanova, N. N., Anderson, I. J., Cheng, J.-F., et al. (2013). Insights into the phylogeny and coding potential of microbial dark matter. Nature 499, 431–437. doi: 10.1038/nature12352
Saxami, G., Kerezoudi, E. N., Eliopoulos, C., Arapoglou, D., Kyriacou, A. (2023). The gut–organ axis within the human body: gut dysbiosis and the role of prebiotics. Life 13, 2023. doi: 10.3390/life13102023
Segata, N., Haake, S., Mannon, P., Lemon, K. P., Waldron, L., Gevers, D., et al. (2012). Composition of the adult digestive tract bacterial microbiome based on seven mouth surfaces, tonsils, throat and stool samples. Genome Biol. 13, R42. doi: 10.1186/gb-2012-13-6-r42
Sun, H., Bai, H., Hu, Y., He, S., Wei, R., Meng, D., et al. (2024). Regulatory mechanisms of dopamine metabolism in a marine Meyerozyma guilliermondii GXDK6 under NaCl stress as revealed by integrative multi-omics analysis. Synth. Syst. Biotechnol. 9, 115–126. doi: 10.1016/j.synbio.2024.01.002
Thomas, T., Gilbert, J., Meyer, F. (2012). Metagenomics - a guide from sampling to data analysis. Microb. Inform Exp. 2, 3. doi: 10.1186/2042-5783-2-3
van Dijk, E. L., Jaszczyszyn, Y., Naquin, D., Thermes, C. (2018). The third revolution in sequencing technology. Trends Genet. 34, 666–681. doi: 10.1016/j.tig.2018.05.008
Yue, Y., Read, T. D., Fedirko, V., Satten, G. A., Hu, Y.-J. (2023). Integrative analysis of microbial 16S gene and shotgun metagenomic sequencing data improves statistical efficiency. Res. Sq. 30, 2023.06.27.546795. doi: 10.21203/rs.3.rs-3376801/v1
Keywords: human microbiome, 16s metagenomic, whole genome sequencing, transcriptomics, metabolomics, proteomics, multi-omics
Citation: Filardo S, Di Pietro M and Sessa R (2024) Current progresses and challenges for microbiome research in human health: a perspective. Front. Cell. Infect. Microbiol. 14:1377012. doi: 10.3389/fcimb.2024.1377012
Received: 26 January 2024; Accepted: 21 March 2024;
Published: 04 April 2024.
Edited by:
Naile Dame-Teixeira, University of Brasilia, BrazilReviewed by:
Julien Santi-Rocca, Science and Healthcare for Oral Welfare, FranceChengjian Jiang, Guangxi University of Science and Technology, China
Copyright © 2024 Filardo, Di Pietro and Sessa. This is an open-access article distributed under the terms of the Creative Commons Attribution License (CC BY). The use, distribution or reproduction in other forums is permitted, provided the original author(s) and the copyright owner(s) are credited and that the original publication in this journal is cited, in accordance with accepted academic practice. No use, distribution or reproduction is permitted which does not comply with these terms.
*Correspondence: Rosa Sessa, cm9zYS5zZXNzYUB1bmlyb21hMS5pdA==
†These authors have contributed equally to this work