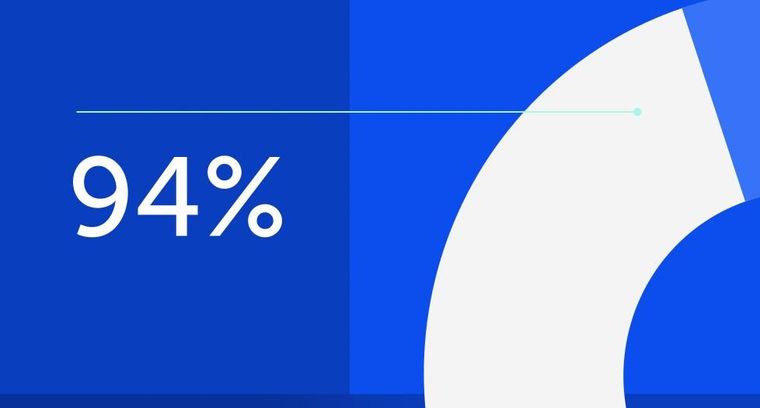
94% of researchers rate our articles as excellent or good
Learn more about the work of our research integrity team to safeguard the quality of each article we publish.
Find out more
ORIGINAL RESEARCH article
Front. Cell. Infect. Microbiol., 04 March 2024
Sec. Clinical Infectious Diseases
Volume 14 - 2024 | https://doi.org/10.3389/fcimb.2024.1348896
Purpose: This study aims to develop and validate a nomogram for predicting the risk of bloodstream infections (BSI) in critically ill patients based on their admission status to the Intensive Care Unit (ICU).
Patients and methods: Patients’ data were extracted from the Medical Information Mart for Intensive Care−IV (MIMIC−IV) database (training set), the Beijing Friendship Hospital (BFH) database (validation set) and the eICU Collaborative Research Database (eICU−CRD) (validation set). Univariate logistic regression analyses were used to analyze the influencing factors, and lasso regression was used to select the predictive factors. Model performance was assessed using area under receiver operating characteristic curve (AUROC) and Presented as a Nomogram. Various aspects of the established predictive nomogram were evaluated, including discrimination, calibration, and clinical utility.
Results: The model dataset consisted of 14930 patients (1444 BSI patients) from the MIMIC-IV database, divided into the training and internal validation datasets in a 7:3 ratio. The eICU dataset included 2100 patients (100 with BSI) as the eICU validation dataset, and the BFH dataset included 419 patients (21 with BSI) as the BFH validation dataset. The nomogram was constructed based on Glasgow Coma Scale (GCS), sepsis related organ failure assessment (SOFA) score, temperature, heart rate, respiratory rate, white blood cell (WBC), red width of distribution (RDW), renal replacement therapy and presence of liver disease on their admission status to the ICU. The AUROCs were 0.83 (CI 95%:0.81-0.84) in the training dataset, 0.88 (CI 95%:0.88-0.96) in the BFH validation dataset, and 0.75 (95%CI 0.70-0.79) in the eICU validation dataset. The clinical effect curve and decision curve showed that most areas of the decision curve of this model were greater than 0, indicating that this model has a certain clinical effectiveness.
Conclusion: The nomogram developed in this study provides a valuable tool for clinicians and nurses to assess individual risk, enabling them to identify patients at a high risk of bloodstream infections in the ICU.
There is a significant correlation between bloodstream infections (BSI) and increased morbidity, mortality, and healthcare costs (Goto and Al-Hasan, 2013; Lydeamore et al., 2022; Verway et al., 2022).The incidence of BSIs is rising, both in the general population and among hospitalized patients (Martínez Pérez-Crespo et al., 2021). In particular, intensive care unit (ICU) patients have twice incidence of non-ICU patients (Kassaian et al., 2023). Delayed effective therapy is associated with worse outcomes (Rhodes et al., 2017; Seymour et al., 2017). Blood culture is the gold standard for diagnosing bloodstream infections, but it typically takes 1-5 days to identify microorganisms and the positivity rate of blood cultures is only 10-20% (Zhou et al., 2023; Lamy et al., 2020), This raises concerns about their cost-effectiveness. Routine blood culture waiting times limit timely escalation or de-escalation of antibiotics, contributing to the development of resistance and increased hospital costs (Cunha, 2018). Therefore, early identification of patients at high-risk for bloodstream infections may facilitate targeted blood culture collection and antibiotic administration, potentially improving patient outcomes and reducing healthcare resource consumption.
The early diagnosis of bloodstream infections focuses on two main areas: the application of molecular pathogen detection and the construction of predictive models. While a number of molecular biology techniques may facilitate early diagnosis of bloodstream infections, clinical impact has been variable, in part due to the prescriber understanding of these rapidly evolving platforms (Briggs et al., 2021). Importantly, these techniques are usually more expensive, limiting their widespread use and not completely replacing the role of blood cultures. On the other hand, predictive models often focus on predicting a bacterial with the use of various biomarkers or aim to predict the occurrence of BSI in a particular group of people (Zhao et al., 2023; Nakamura et al., 2023; Wang et al., 2021; Lee et al., 2014), and their timing is usually concentrated around the time of blood culture collection or a period preceding suspected infection (Fabre et al., 2020). Several studies have developed a machine learning (ML) algorithm to predict BSI in patients with suspected infections in the intensive care unit, but valuable predictors are mainly trends in time-series variables, which makes them geographically limited and not widely generalisable (Roimi et al., 2020; Van Steenkiste et al., 2019). Last but not least, few articles on predicting BSI have been externally validated (Jones et al., 2021; Hertz et al., 2022).
To the best of our knowledge, there are no studies predicting the risk of bloodstream infections in critically ill patients based on their admission status to the Intensive Care Unit (ICU). We aim to construct a model of bloodstream infections at the time of ICU admission and external validate the utility of the model. This model is designed to promptly identify patients at an elevated risk of developing bacteremia among all ICU admissions. It has the potential to minimize unnecessary blood culture collection in low-risk patients while guiding the appropriate use of blood cultures in the ICU.
This study was performed with the data from the Medical Information Mart for Intensive Care (MIMIC-IV (version 1.0) (Timmer et al., 2021; Johnson et al., 2023,) the eICU Collaborative Research Database (eICU-CRD) version 1.2 (Pollard et al., 2018). and the Beijing Friendship Hospital (BFH).MIMIC-IV database consists of comprehensive and high-quality data of patients admitted to ICUs at the Beth Israel Deaconess Medical Center between 2008 and 2019. The data in the eICU database covers patients who were admitted to a combination of many critical care units throughout the continental United States in 2014 and 2015. Both MIMIC-IV and eICU databases are public databases for critically ill patients. All data from the two database studies were extracted by the first author (certification number: 39247526), who passed the Collaborative Institutional Training Initiative examination and was granted access to the database for data extraction. The Massachusetts Institute of Technology institutional review boards approved using the database. Beijing Friendship Hospital is a tertiary hospital in Beijing, China. The validation data came from the ICU of Beijing Friendship Hospital West Side Courtyard, a comprehensive ICU with 20 beds. We selected patients who were admitted to the ICU from January 1, 2019, to June 30, 2019, and obtained the informed consent of the ethics committee of Beijing Friendship Hospital (2021-P2-053).
The MIMIC-IV and eICU data extraction codes, are available on GitHub (https://github.com). The data from BFH was collected manually. We used MIMIC-IV data for modeling. The exclusion criteria of the modeling dataset were: 1) Patients ‘age < 18 years; 2) Time to ICU admission < 48 hours; 3) Repeated admission to ICU;4) Positive blood cultures prior to admission to the ICU. As the aim of our study was to predict BSIs based on patients’ admission to the ICU, we did not exclude patients admitted to the ICU for less than 48 hours from the validation set and then performed a sensitivity analysis.
BSI is defined as the growth of a clinically significant pathogen in at least one blood culture bottle. Potential contaminants (including coagulase-negative Staphylococci, Corynebacterium species, Bacillus species, diphtheroids, Aerococcus, and Propionibacterium sp) were defined according to the Center for Disease Control and Prevention (CDC)/National Health Safety Network (NHSN) guidelines for Laboratory Confirmed Bloodstream Infections (LCBI) and were not considered BSI (Karakullukçu et al., 2017; Hall and Lyman, 2006). For patients with positive blood cultures, only the first positive result was included. Only the first blood culture result was included for patients with negative blood cultures.
We constructed the model by extracting data from the database MIMIC-IV, included 3 basic information items, 4 critical illness scores, 4 first-day vital signs, 16 laboratory test parameters, 5 baseline conditions and 15 comorbidities before ICU admission, resulting in a total of 47 characteristics. Baseline ICU assessment scales such as SOFA and Simplified Acute Physiology Score II (SAPS II) were calculated and collected on admission. Intervention events included vasopressors, mechanical ventilation, renal replacement therapy, and central venous catheterization. Laboratory information was collected on access to the ICU. We randomly divided the modeling dataset into training and internal validation datasets, which comprised 70% and 30% of the dataset, respectively.
Data cleaning: In the modeling dataset, for missing values, we first removed variables with missing values ≥20%. We then deleted all individuals with any missing values. We defined plausible ranges for vital signs. We applied the capping method to handle implausible values (Supplementary Table 1). In the validation dataset, we deleted all the data with missing values. In the eICU validation dataset, we also applied the capping method to handle implausible values (Supplementary Table 2).
The eICU website (https://eicu-crd.mit.edu/eicutables/microlab/),states that the dataset is not well populated due to limited availability of microbiology interfaces, there were only 100 blood culture-positive patients. Referring to the MIMIC-IV and BFH datasets, the proportion of BSIs was approximately 4%-5% in the included population, we matched 2000 blood culture-negative patients, resulting in the inclusion of 2100 patients as a validation set. As for admissions data of the BFH dataset, due to the manual collection by our doctors, there is no abnormal value.
Medians and interquartile ranges were used for continuous variables with a skewed distribution. In cases where two independent samples adhered to a normal distribution and variance homogeneity was assumed, we utilized the independent samples t-test for comparing differences. The chi-squared test or Fisher’s exact test was used to assess whether there was a difference between the two overall rates and constituent ratios. To establish a well-calibrated nomogram for predicting outcomes, we performed univariate regression analyses to screen for predictors, in which variables with P < 0.05 in the univariate were entered into the Lasso regression. We used variance inflation factor method to detect multicollinearity in the regression model, and we removed variables with a VIF≥4. In the Lasso regression analysis, the selected coefficient for the screening threshold is Lambda=0.025. Based on these results, we then constructed a nomogram prediction model based on independent risk factors.
The model was then validated and evaluated: Firstly, the area under the receiver operating characteristic curve (AUROC) was used to analyze the model’s accuracy. Secondly, the calibration curve is drawn through 200 repeated sampling verifications, and the correlation between the calibration curve and the standard curve is verified and evaluated. Lastly, to ascertain the model’s clinical applicability, we employed both clinical impact curve and decision curve analyses.
The analysis software for this study was R version 4.2.2 (The R Foundation for Statistical Computing, Austria, Vienna).
The MIMIC-IV database concludes 76540 patients, all patients included in MIMIC-IV were older than 18 years, 39,682patients were admitted to the ICU for <48h, and 125 patients had positive blood cultures before ICU admission. According to the exclusion criteria, 33993 patients remained. After removing Potential contaminants and missing values there were 1444 patients with positive blood cultures, The positive rate of blood culture was 8.72%.
To construct our models, we gathered a diverse range of data from the patient’s first day in the ICU. This included basic information items, critical illness scores, first-day vital signs, laboratory test parameters, and comorbidities and baseline conditions before ICU admission. We compared the clinical characteristics between the BSI group, blood culture negative group and without blood culture group. Patients with BSI had significantly higher rates of sepsis, days in ICU and in-hospital mortality. It can be seen that the variables such as white blood cell(WBC), ventilator use and Diabetes were statistically significant in the patients with BSI and no blood culture group but not statistically significant between the BSI and blood culture negative group. The clinical characteristics of the three groups of patients were compared in Table 1. The model dataset consisted of 14930 patients, including those with positive blood cultures and those without blood cultures. The training dataset consisted of 10447 patients (999 with BSI) and the internal validation dataset consisted of 4483 patients (445 with BSI). The diagram of the selection process is shown in Supplementary Figure 1. We divided the model dataset into train dataset and internal validation dataset, and the comparison of the clinical characteristics of these two groups of patients is shown in Supplementary Table 3, which shows that there is no significant difference between the train and the internal validation group of patients in terms of baseline clinical characteristics.
Table 1 Univariate analysis the characteristic of BSI group, negative blood culture(BC) group and no blood cultures(No-BC) group in the MIMIC-IV dataset.
The eICU dataset contained 200859 patients, 75829 patients were included according to the exclusion criteria, finally, a total of 2100 patients included in the eICU validation dataset. In the BFH dataset, a total of 542 patients were admitted to the ICU during enrollment, and 419 patients were ultimately included in the validation dataset based on exclusion criteria. (Figure 1) In the eICU dataset 78553 patients were admitted to ICU <48 h and in the BFH dataset 243 patients were admitted to ICU <48 h. Our study aimed to predict positive blood culture results in patients on their admission status to the ICU, therefore we included all patients admitted to ICU in the validation dataset, and sensitivity analyses were performed with patients admitted to ICU <48h. Table 2 presents a comparison of clinical characteristics among the three databases.
Figure 1 Flow diagram of blood culture data processing. ICU, Intensive Care Unit; ICU LOS, Length of stay in the ICU. Age<18, ICU LOS <48.
The results of the univariate analysis in the training set are shown in Supplementary Table 4.Variables with P < 0.05 in the univariate were entered into the Lasso regression, with a Lasso regression coefficient threshold of Lambda=0.025, we ultimately selected 9 critical variables for inclusion as shown in Supplementary Figure 2. These variables encompassed the GCS, SOFA, heart rate, temperature, white blood cell count, RDW, Renal Replacement Therapy, and liver disease. In this training database, a nomogram to predict the risk of bloodstream infections in critically ill patients was constructed based on their admission data to the ICU (Figure 2).
After building the model, we evaluated its accuracy using the area under the receiver operating characteristic curve (AUROC). ROC analysis revealed an AUC (Area Under the Curve) value of 0.830 (95% CI 0.816-0.844) in the training dataset (Figure 3A), 0.838 (95% CI 0.818-0.858) in the internal validation dataset (Figure 3B), 0.878 (95% CI 0.797-0.958) in the BFH validation dataset (Figure 3C), and 0.751 (95% CI 0.705-0.797) in the eICU validation dataset (Figure 3D). These results indicate robust performance across different datasets. In addition, we excluded patients in the validation dataset who were admitted to the ICU for <48 h, and the AUROC results are shown in the Supplementary Figure 3, with an AUC of 0.698 (95% CI 0.653-0.756) in the eICU and an AUC of 0.838 (95% CI 0.720-0.957) in the BFH dataset.
Figure 3 ROC curves of the Training dataset (A), Internal validation dataset (B), eICU validation dataset (C) and BFH validation dataset (D).
Furthermore, calibration curves were constructed to validate the effectiveness of the model further (Figure 4). The calibration curves showed that the prediction probabilities of the model agreed well with the BFH dataset, but did not agree well with the eICU dataset. Finally, the clinical application value of this model was further evaluated. We plotted both the decision curve (Figure 5) and the clinical impact curve (Figure 6). In the decision curves,the horizontal coordinate represents the threshold probability of predicting blood culture positive, which ranges from 0-1; the vertical coordinate represents the net benefit after benefits minus drawbacks, with a larger interval of the probability distribution of the net benefit. Three models are compared in the figure, represented by lines of three different colors. The first one is the black parallel horizontal line above the horizontal coordinate, which is the ideal model, that is, it is considered that none of the patients will develop bloodstream infections, so there is no intervention at all, and the net benefit resulting from it is 0. The second one is the gray line in the figure, which is presented in three lines, and it will show its confidence intervals. And this set of lines indicates a pessimistic attitude that blood culture positive secondary will occur in all patients. That means the net benefit through intervention when all patients are at high risk and have a poor prognosis. The third is the group of lines in red, with the thicker line in the center being the actual modeling line and its confidence interval on either side. That is, the predicted probabilities below are used to determine the circumstances under which a higher net benefit can result when going to intervention. The bottom axis represents the ratio of the payoff to the benefit curve, and it can be seen that when intervening at 40%, the payoff to benefit ratio is 2:3, and at 60%, the payoff to benefit ratio is 3:2, at which point the benefit is much smaller, suggesting that the negative impact of blood culture positive would be significant. Most of the region of the decision curve for this model is greater than 0, indicating some clinical validity. However, it should be noted that the model did not agree well with the eICU dataset.
Figure 4 Calibration curves of the nomogram in the Training dataset (A), Internal validation dataset (B), eICU validation dataset (C) and BFH validation dataset (D).
Figure 5 Decision curve analysis (DCA) for the nomogram in theTraining dataset (A), Internal validation dataset (B), eICU validation dataset (C) and BFH validation dataset (D).
Figure 6 The clinical impact curve in the Training dataset (A), Internal validation dataset (B), eICU validation dataset (C) and BFH validation dataset (D).
BSI is a significant cause of healthcare-associated infections in the ICU, with high mortality rates and increased length of stay and costs. Approximately 47.3% of ICU patients with BSIs receive inappropriate or no antibiotic treatment within the first 24 hours of onset (Tabah et al., 2012; Lee et al., 2007). Study has shown that establishing an effective predictive model can minimize unnecessary antibiotic usage in pediatric patients (Esbenshade et al., 2020). However, to the best of our knowledge, there are no studies using data collected immediately on ICU admission to predict BSI and externally validate. Our study successfully developed a predictive model for identifying the likelihood of subsequent bloodstream infections. We presented the model using a nomogram that allows assessment of the risk of bloodstream infections in ICU patients at the time of admission using commonly used available clinical data. The AUROC of the training dataset was 0.83, and the AUROCs of the external validation dataset were 0.88 and 0.75. In a few minutes or less, healthcare professionals can assess the risk of bloodstream infections in ICU patients. This provides valuable information for making timely clinical decisions based on the risk of bloodstream infections.
MIMIC-IV database is a global widely known public database in the field of critical care, many scholars have applied MIMIC-IV database for their research, which is relatively comprehensive in terms of variables, mature in terms of data extraction, and reproducible, so we applied MIMIC-IV database for the construction of the model. When Mark Verway compared patients with positive cultures with those with negative cultures, the adjusted 30-day mortality risk for positive BSIs was 1.47. Interestingly, this risk escalated to 2.62 when these patients were compared with matched datasets without blood culture testing (Verway et al., 2022). Our study also found that more variables were statistically significant in the univariate analysis in patients with BSI and those without blood cultures. So we included both patients with BSI and those without blood cultures in the modeling process. We then validated the model using data from all ICU admissions in the two external validations.
The predictive model in our study is primarily based on clinically common variables, including Glasgow Coma Scale (GCS) score, Sequential Organ Failure Assessment (SOFA) score, heart rate, respiratory rate, body temperature, white blood cell count, red cell distribution width (RDW), whether renal replacement therapy was used on day 1 and presence of liver disease. Our study includes a comprehensive array of data, including critical illness scoring, vital signs, laboratory indicators and baseline conditions. The variables we included are consistent with other studies. Temperature, white blood cells, and SOFA scores are risk factors often incorporated into predictive models of bloodstream infections (Van Steenkiste et al., 2019; Hertz et al., 2022; Schwenzer et al., 1994; Pereira et al., 2012), some articles have incorporated heart rate, respiratory rate, and the presence of renal replacement therapy (Lorente et al., 2022; Montrucchio et al., 2022; Previsdomini et al., 2012).RDW is known to be associated with mortality, an elevated RDW at admission is linked to adverse outcomes in the short and long term for both adult and neonatal patients (Dankl et al., 2022). However, RDW has limited diagnostic value for sepsis (Hu et al., 2020). Nathan Jones and Frederik Boetius Hertz analyzed the value of some biomarkers in assessing the risk of bloodstream infections, the AUC reporting on the discriminatory power between 0.5017-0.8243, but they were not conduct externally validated (Jones et al., 2021; Hertz et al., 2022). The variables in our predictive model included criticality scores, baseline conditions and laboratory indicators, which may explain the better results of our model, in addition, we validated our predictive model with an external dataset.
In our study, AUC did not declined in the BFH dataset, but declined more significantly in the eICU dataset, and we considered several reasons for this: Firstly, The eICU dataset is not well populated due to limited availability of microbiology interfaces (Pollard et al., 2018). The eICU database contains more than 200,000 patients but only 100 blood culture positive patients were ultimately included in our study, so we randomly selected 2000 blood culture negative patients to collectively form the validation set, but this may have the problem of data bias. Secondly, comparison of the variables in the three datasets revealed statistically significant differences in baseline characters, suggesting that there may be differences in the type and severity of patients admitted, which may account for the decline in AUC. The AUC of our model is above 70% in both external validation sets, In our opinion, the presence of differences in the baseline data is more conducive to expand the application of the predictive model. In the BFH data, a large proportion of patients with cerebrovascular disease and tumors can be seen, which may indicate that the model may be more valuable for extension to patients with tumors and cerebrovascular disease. Thirdly, the small number of positive results in the eICU and BFH databases may also lead to bias in the performance of the models, which might be reduced by expanding the sample size.
Our study has several limitations. First, there is insufficient baseline data for the three centers, which may not be conducive to exploring why the efficiency of the model decreases, but this does not affect the verification and use of the model. Second, we did not collect data on the source of the pathogens or variables such as procalcitonin (PCT) and albumin due to the high missing values in the MIMIC-IV database and the non-routine measurement in some centers. The inclusion of these variables could potentially improve the efficiency of the predictive model. Third, the model has not been prospectively validated. We plan to conduct a multicenter, prospective validation study of this bloodstream infection prediction model.
In our study, we developed a predictive model for bloodstream infections in critically ill patients on the first day of ICU admission. We employed a nomogram as a visual tool to facilitate an intuitive and practical assessment of bloodstream infection risk. This nomogram utilizes available clinical data from the patient’s first day in the ICU. Such a tool may help determine whether to initiate or modify antibiotic therapy and reduce healthcare resources. Further research should focus on improving the classification performance and conducting a larger external validation to assess the clinical impact of the model.
The original contributions presented in the study are included in the article/Supplementary Material. Further inquiries can be directed to the corresponding author.
The studies involving humans were approved by Bioethics Committee of Beijing Friendship Hospital, Capital Medical University. The studies were conducted in accordance with the local legislation and institutional requirements. Written informed consent for participation was not required from the participants or the participants’ legal guardians/next of kin in accordance with the national legislation and institutional requirements.
ZQ: Data curation, Methodology, Software, Validation, Writing – original draft. LD: Data curation, Validation, Writing – review & editing. JL: Conceptualization, Writing – review & editing. MD: Conceptualization, Project administration, Supervision, Writing – review & editing.
The author(s) declare financial support was received for the research, authorship, and/or publication of this article. This work was supported by Beijing Friendship Hospital Scientific Research Start-up Fund (yyqdkt2020-27) and Beijing Tongzhou District Science and Technology Programme (KJ2023CX017).
The authors would like to thank Beijing Friendship Hospital Methodology Platform and Jiaqi Lu for methodological guidance.
The authors declare that the research was conducted in the absence of any commercial or financial relationships that could be construed as a potential conflict of interest.
The reviewer ZZ declared a past co-authorship with the authors JL and MD to the handling editor.
All claims expressed in this article are solely those of the authors and do not necessarily represent those of their affiliated organizations, or those of the publisher, the editors and the reviewers. Any product that may be evaluated in this article, or claim that may be made by its manufacturer, is not guaranteed or endorsed by the publisher.
The Supplementary Material for this article can be found online at: https://www.frontiersin.org/articles/10.3389/fcimb.2024.1348896/full#supplementary-material
Briggs, N., Campbell, S., Gupta, S. (2021). Advances in rapid diagnostics for bloodstream infections. Diagn. Microbiol. Infect. Dis. 99, 115219. doi: 10.1016/j.diagmicrobio.2020.115219
Cunha, C. B. (2018). Antimicrobial stewardship programs: principles and practice. Med. Clin. North Am. 102, 797–803. doi: 10.1016/j.mcna.2018.04.003
Dankl, D., Rezar, R., Mamandipoor, B., Emily, A.H., Daniel, E.D, Ritu, B., et al. (2022). Red cell distribution width is independently associated with mortality in sepsis. Med. Princ Pract. 31, 187–194. doi: 10.1159/000522261
Esbenshade, A. J., Zhao, Z., Baird, A., Holmes, E. A., Dulek, D. E., Banerjee, R., et al. (2020). Prospective implementation of a risk prediction model for bloodstream infection safely reduces antibiotic usage in febrile pediatric cancer patients without severe neutropenia. J. Clin. Oncol. 38, 3150–3160. doi: 10.1200/JCO.20.00591
Fabre, V., Sharara, S. L., Salinas, A. B., Karen, C. C., Sanjay, D., Sara, E. C., et al. (2020). Does this patient need blood cultures? A scoping review of indications for blood cultures in adult nonneutropenic inpatients. Clin. Infect. Dis. 71, 1339–1347. doi: 10.1093/cid/ciaa039
Goto, M., Al-Hasan, M. N. (2013). Overall burden of bloodstream infection and nosocomial bloodstream infection in North America and Europe. Clin. Microbiol. Infect. 19, 501–509. doi: 10.1111/1469-0691.12195
Hall, K. K., Lyman, J. A. (2006). Updated review of blood culture contamination. Clin. Microbiol. Rev. 19, 788–802. doi: 10.1128/CMR.00062-05
Hertz, F. B., Ahlström, M. G., Bestle, M. H., Lars, H., Thomas, M., Jens, D. L., et al. (2022). Early biomarker-guided prediction of bloodstream infection in critically ill patients: C-reactive protein, procalcitonin, and leukocytes. Open Forum Infect. Dis. 9, ofac467. doi: 10.1093/ofid/ofac467
Hu, Z.-D., Lippi, G., Montagnana, M. (2020). Diagnostic and prognostic value of red blood cell distribution width in sepsis: A narrative review. Clin. Biochem. 11, 1–6. doi: 10.1016/j.clinbiochem.2020.01.001
Johnson, A. E. W., Bulgarelli, L., Shen, L., Alvin, G., Ayad, S., Steven, H., et al. (2023). MIMIC-IV, a freely accessible electronic health record dataset. Sci. Data 10, 1. doi: 10.1038/s41597-022-01899-x
Jones, N., Tridente, A., Dempsey-Hibbert, N. C. (2021). Immature platelet indices alongside procalcitonin for sensitive and specific identification of bacteremia in the intensive care unit. Platelets 32, 941–949. doi: 10.1080/09537104.2020.1809646
Karakullukçu, A., Kuşkucu, M. A., Ergin, S., Gökhan, A., Kenan, M., Ömer, K., et al. (2017). Determination of clinical significance of coagulase-negative staphylococci in blood cultures. Diagn. Microbiol. Infect. Dis. 87, 291–294. doi: 10.1016/j.diagmicrobio.2016.12.006
Kassaian, N., Nematbakhsh, S., Yazdani, M., Soodabeh, R., Zary, N., Behrooz, A., et al. (2023). Epidemiology of bloodstream infections and antimicrobial susceptibility pattern in ICU and non-ICU wards A four-year retrospective study in isfahan, Iran. Adv. BioMed. Res. 27, 12:106. doi: 10.4103/abr.abr_320_22
Lamy, B., Sundqvist, M., Idelevich, E. A.(2020). ESCMID Study Group for Bloodstream Infections and Endocarditis and Sepsis (ESGBIES). Bloodstream infections - Standard and progress in pathogen diagnostics. Clin. Microbiol. Infect. 26, 142–150. doi: 10.1016/j.cmi.2019.11.017
Lee, A., Mirrett, S., Reller, L. B., Melvin, P. W. (2007). Detection of bloodstream infections in adults: how many blood cultures are needed? J. Clin. Microbiol. 45, 3546–3548. doi: 10.1128/JCM.01555-07
Lee, J., Hwang, S. S., Kim, K., You, H. J., Jae, H. L., Joonghee, K., et al. (2014). Bacteremia prediction model using a common clinical test in patients with community-acquired pneumonia. Am. J. Emerg. Med. 32, 700–704. doi: 10.1016/j.ajem.2014.04.010
Lorente, L., Lecuona, M., Pérez-Llombet, A., Adriana, G. M., Manuel, C., Alejandro, J., et al. (2022). Skin insertion site culture for the prediction of primary bloodstream infection. Irish J. Med. Sci. 191, 1269–1275. doi: 10.1007/s11845-021-02685-1
Lydeamore, M. J., Mitchell, B. G., Bucknall, T., Cheng, A. C., Russo, P. L., Stewardson, A. J., et al. (2022). Burden of five healthcare associated infections in Australia. Antimicrob. Resist. Infect. Control 11, 69. doi: 10.1186/s13756-022-01109-8
Martínez Pérez-Crespo, P. M., López-Cortés, L. E., Retamar-Gentil, P., Lanz, G. J. F., Vinuesa, G. D, León, E., et al. (2021). Epidemiologic changes in bloodstream infections in Andalucia (Spain) during the last decade. Clin. Microbiol. Infect. 27, 283.e9–283.e16. doi: 10.1016/j.cmi.2020.05.015
Montrucchio, G., Costamagna, A., Pierani, T., Alessandra, P., Gabriele, S., Emanuele, P., et al. (2022). Bloodstream infections caused by carbapenem-resistant pathogens in intensive care units: Risk factors analysis and proposal of a prognostic score. Pathogens 11 (7), 718. doi: 10.3390/pathogens11070718
Nakamura, K., Hayakawa, K., Tsuzuki, S., Satoshi, I., Hidetoshi, N., Takato, N., et al. (2023). Clinical outcomes and epidemiological characteristics of bacteremia in the older Japanese population. J. Infect. Chemother. 29, 971–977. doi: 10.1016/j.jiac.2023.06.015
Pereira, J. M., Teixeira-Pinto, A., Basílio, C., Conceição, S. D., Paulo, M., José, A. P., et al. (2012). Can we predict pneumococcal bacteremia in patients with severe community-acquired pneumonia? Intensive Care Med. 38, S129. doi: 10.1016/j.jcrc.2013.04.016
Pollard, T. J., Johnson, A. E. W., Raffa, J. D., Leo, A. C., Roger, G. M., Omar, B., et al. (2018). The eICU Collaborative Research Database, a freely available multi-center database for critical care research. Sci. Data 5, 180178. doi: 10.1038/sdata.2018.178
Previsdomini, M., Gini, M., Cerutti, B., Dolina, M., Perren, A. (2012). Predictors of positive blood cultures in critically ill patients: A retrospective evaluation. Croatian Med. J. 53, 30–39. doi: 10.3325/cmj.2012.53.30
Rhodes, A., Evans, L. E., Alhazzani, W., Massimo, A., Ricard, F., Anand, K., et al. (2017). Surviving sepsis campaign: international guidelines for management of sepsis and septic shock: 2016. Intensive Care Med. 43, 304–377. doi: 10.1007/s00134-017-4683-6
Roimi, M., Neuberger, A., Shrot, A., Mical, P., Yuval, G., Yaron, B., et al. (2020). Early diagnosis of bloodstream infections in the intensive care unit using machine-learning algorithms. Intensive Care Med. 46, 454–462. doi: 10.1007/s00134-019-05876-8
Schwenzer, K. J., Gist, A., Durbin, C. G. (1994). Can bacteremia be predicted in surgical intensive care unit patients? Intensive Care Med. 20, 425–430. doi: 10.1007/BF01710653
Seymour, C. W., Gesten, F., Prescott, H. C., Marcus, E. F., Theodore, J. I., Gary, S. P., et al. (2017). Time to treatment and mortality during mandated emergency care for sepsis. N Engl. J. Med. 376, 2235–2244. doi: 10.1056/NEJMoa1703058
Tabah, A., Koulenti, D., Laupland, K., Benoit, M., Jordi, V., Frederico, B. C., et al. (2012). Characteristics and determinants of outcome of hospital-acquired bloodstream infections in intensive care units: the EUROBACT International Cohort Study. Intensive Care Med. 38, 1930–1945. doi: 10.1007/s00134-012-2695-9
Timmer, B. J. J., Kooijman, A., Schaapkens, X., Tiddo, J. M. (2021). A synthetic galectin mimic. Angew Chem. Int. Ed Engl. 60, 16178–16183. doi: 10.1002/anie.202104924
Van Steenkiste, T., Ruyssinck, J., Baets, L., Johan, D., Filip, D. T., Femke, O., et al. (2019). Accurate prediction of blood culture outcome in the intensive care unit using long short-term memory neural networks. Artif. Intell. Med. 97, 38–43. doi: 10.1016/j.artmed.2018.10.008
Verway, M., Brown, K. A., Marchand-Austin, A., Christina, D., Samantha, L., Bradley, L., et al. (2022). Prevalence and mortality associated with bloodstream organisms: A population-wide retrospective cohort study. J. Clin. Microbiol. 60, e0242921. doi: 10.1128/jcm.02429-21
Wang, Y., Lin, Q., Chen, Z., Hongyan, H., Na, S., Zhen, W., et al. (2021). Construction of a risk prediction model for subsequent bloodstream infection in intestinal carriers of carbapenem-resistant enterobacteriaceae: A retrospective study in hematology department and intensive care unit. Infect. Drug Resist. 14, 815–824. doi: 10.2147/IDR.S286401
Zhao, Y., Lin, Q., Zhang, T., Sisi, Z., Jieru, W., Erlie, J., et al. (2023). Pseudomonas aeruginosa bloodstream infection in patients with hematological diseases: Clinical outcomes and prediction model of multidrug-resistant infections. J. Infect. 86, 66–117. doi: 10.1016/j.jinf.2022.08.037
Keywords: bloodstream infections, bacteremia, intensive care unit, critically ill, early diagnosis, nomogram, prediction model
Citation: Qi Z, Dong L, Lin J and Duan M (2024) Development and validation a nomogram prediction model for early diagnosis of bloodstream infections in the intensive care unit. Front. Cell. Infect. Microbiol. 14:1348896. doi: 10.3389/fcimb.2024.1348896
Received: 03 December 2023; Accepted: 12 February 2024;
Published: 04 March 2024.
Edited by:
Keliang Xie, Tianjin Medical University, ChinaReviewed by:
Dhammika Leshan Wannigama, Yamagata Prefectural Central Hospital, JapanCopyright © 2024 Qi, Dong, Lin and Duan. This is an open-access article distributed under the terms of the Creative Commons Attribution License (CC BY). The use, distribution or reproduction in other forums is permitted, provided the original author(s) and the copyright owner(s) are credited and that the original publication in this journal is cited, in accordance with accepted academic practice. No use, distribution or reproduction is permitted which does not comply with these terms.
*Correspondence: Meili Duan, ZG1laWxpQGNjbXUuZWR1LmNu
Disclaimer: All claims expressed in this article are solely those of the authors and do not necessarily represent those of their affiliated organizations, or those of the publisher, the editors and the reviewers. Any product that may be evaluated in this article or claim that may be made by its manufacturer is not guaranteed or endorsed by the publisher.
Research integrity at Frontiers
Learn more about the work of our research integrity team to safeguard the quality of each article we publish.