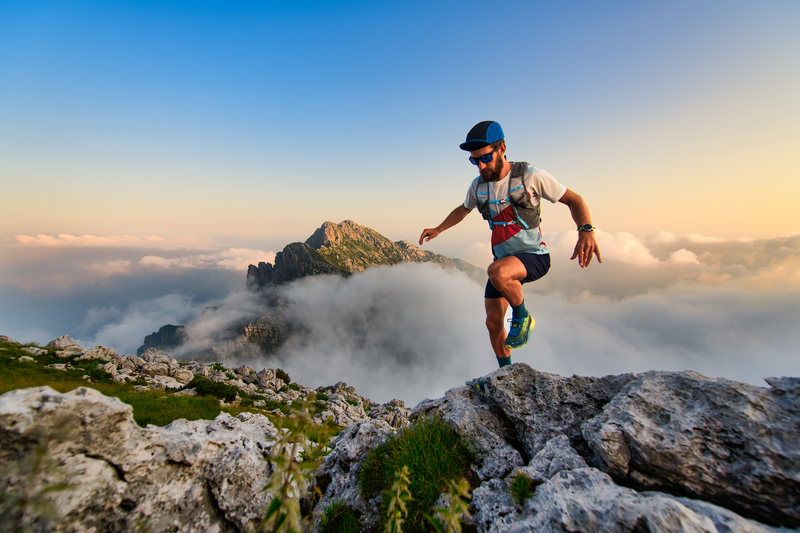
95% of researchers rate our articles as excellent or good
Learn more about the work of our research integrity team to safeguard the quality of each article we publish.
Find out more
ORIGINAL RESEARCH article
Front. Cell. Infect. Microbiol. , 26 March 2024
Sec. Intestinal Microbiome
Volume 14 - 2024 | https://doi.org/10.3389/fcimb.2024.1327032
Aim: Mendelian randomization (MR) analysis has been used in the exploration of the role of gut microbiota (GM) in type 2 diabetes mellitus (T2DM); however, it was limited to the genus level. This study herein aims to investigate the relationship of GM, especially at the species level, with T2DM in order to provide some evidence for further exploration of more specific GM taxa and pathway abundance in T2DM.
Methods: This two-sample MR study was based on the summary statistics of GM from the available genome-wide association study (GWAS) meta-analysis conducted by the MiBioGen consortium as well as the Dutch Microbiome Project (DMP), whereas the summary statistics of T2DM were obtained from the FinnGen consortium released data. Inverse variance weighted (IVW), MR-Egger, strength test (F), and weighted median methods were used to examine the causal association between GM and the onset of T2DM. Cochran’s Q statistics was employed to quantify the heterogeneity of instrumental variables. Bonferroni’s correction was conducted to correct the bias of multiple testing. We also performed reverse causality analysis.
Results: The corrected IVW estimates suggested the increased relative abundance of family Oxalobacteraceae (OR = 1.0704) and genus Oxalobacter (OR = 1.0874), respectively, were associated with higher odds of T2DM, while that of species faecis (OR = 0.9460) had a negative relationship with T2DM. The relationships of class Betaproteobacteria, family Lactobacillaceae, species finegoldii, and species longum with T2DM were also significant according to the IVW results (all P < 0.05).
Conclusions: GM had a potential causal association with T2DM, especially species faecis, finegoldii, and longum. Further studies are still needed to clarify certain results that are contradictory with previous findings.
Type 2 diabetes mellitus (T2DM) is growing at an alarming speed globally in the 21st century (International Diabetes Federation (IDF), 2022). T2DM as well as its complications have brought a heavy burden of disease in all regions (Ali et al., 2022). Identifying the factors that have a causal relationship with the development of T2DM can provide an important evidence base for disease prevention and facilitate the development of new treatment strategies.
The gut microbiota (GM) is a complex ecosystem and consists of approximately 4 × 1013 species of symbiotic bacteria, protozoa, fungi, archaea, and viruses (Chen et al., 2021; Martino et al., 2022). GM is involved in a variety of physiological activities in the human body, such as metabolism, inflammatory processes, and immune responses (Fan and Pedersen, 2021; Gill et al., 2022). Increasing evidence have shown that GM plays an important role in metabolic diseases such as T2DM (Gurung et al., 2020). Patients with T2DM have metabolic disorders and chronic inflammatory states accompanied by disturbances in the GM (Yang et al., 2021). A significant association of changes in the composition profile of GM with the development of T2DM as well as related complications have also been found (Iatcu et al., 2021)—for example, the disequilibrium of phylum Bacteroidetes/Firmicutes has been associated with increased intestinal permeability, with bacteria byproducts infiltrating through a leaky gut barrier triggering subsequent inflammatory responses characteristic of DM (Iatcu et al., 2021). Several bacteria, such as Lactobacillus fermentum, plantarum and casei, Roseburia intestinalis, Akkermansia muciniphila and Bacteroides fragilis, have also been reported to exert a protective role via reducing the risk of DM development through decreasing proinflammatory markers and maintaining intestinal barrier integrity (Iatcu et al., 2021). Nevertheless, it is necessary to distinguish between the characteristics of the GM that cause the disease and those caused by the disease or its treatment.
Mendelian randomization (MR) is a valuable tool to assess the causality of an observed relationship between a modifiable exposure or risk factor and a clinically relevant outcome (Sekula et al., 2016). Due to Mendelian law of segregation and independent classification, it can eliminate confounding bias in comparison to traditional observational epidemiological studies and facilitate the separation of causal pathways for phenotypic grouping risk variables that are difficult to randomize or susceptible to measurement errors (Davies et al., 2018). Moreover, MR results are less susceptible to bias caused by reverse causation because the genetic code is not influenced by environmental factors or preclinical diseases (Skrivankova et al., 2021).
In recent years, MR analysis has been gradually applied to explore the causal association of GM with the risk of T2DM (Sanna et al., 2019; Yuan et al., 2023). Current studies have been limited to exploring the causal association between the abundance of GM in specific families or genera and the occurrence of T2DM. Yang et al. (2018) identified genus Acidaminococcus, Aggregatibacter, Anaerostipes, Blautia, Desulfovibrio, Dorea, and Faecalibacterium to be nominally linked to T2DM. Xiang et al. (2022) suggested that Streptococcaceae was associated with a higher risk of T2DM in European populations, whereas there was a causal relationship between Acidaminococcaceae and T2DM in Asian populations. Most previous cohorts relied on 16s ribosomal RNA (rRNA) measurements, which could not allow bacterial or pathway abundance identification at the species level. In fact, the measurement of species and pathway abundance is essential for further investigation on the GM in an individual because even being placed in the same genus does not guarantee metabolic consistency in the physiological process (Vieira-Silva et al., 2016; Lopera-Maya et al., 2022).
This study herein performed a two‐sample MR analysis to investigate the potential causal relationship of GM with the occurrence of T2DM based on all existing GM taxa (including phylum, class, order, family, genus, and species) in order to provide some evidence-based evidence on T2DM prevention.
In this two-sample MR study, data on genome-wide association studies (GWASs) were extracted for GM and T2DM. Figure 1 shows a flowchart of the research procedure. GM taxa including phylum, class, order, family, and genus levels were extracted from the MiBioGen consortium (Kurilshikov et al., 2021), whereas the species-level data were extracted from the Dutch Microbiome Project (DMP) (Lopera-Maya et al., 2022). Data on patients with T2DM were extracted from the FinnGen consortium (Yuan and Larsson, 2022). More details on the source of exposures and outcomes are shown in Table 1.
This study was conducted in accordance with the local legislation and institutional requirements. The participants have provided their written informed consent to participate in each GWAS. The requirement of ethical approval was waived by the Shanghai Municipal Hospital of Traditional Chinese Medicine for the studies involving humans because these databases used in our study were publicly available, and all data were de-identified.
We first selected single-nucleotide polymorphisms (SNPs) that significantly associated with GM as potential instrument variables (IVs). The threshold to select IVs was P < 1.0 × 10-6. We then removed SNPs with minor allele frequency (MAF) ≤0.01. The linkage disequilibrium (LD) threshold was set to be r2 = 0.001, with a clumping distance of 10,000 kb. The MR-Egger regression test was used to monitor potential horizontal pleiotropy effect, namely, the confounding effect resulted from other diseases, which may violate the second assumption in MR analysis. The intercept item of MR-Egger that was significant represents the existence of pleiotropy. Additionally, palindromic SNPs were deleted according to the principle of MR to ensure that the same allele corresponds the effects between SNPs and the exposure and that on the outcome.
MR must conform to three important assumptions to minimize the impact of bias on the results. First, IVs must be independent of confounders related to both exposure and outcome. Second, IVs should be significantly associated with the exposure. We estimated the association strength between GM and IVs according to the formula: F = ((N – K - 1)/K) * (r2/(1 - r2)), r2 = 2 * EAF * (1 - EAF) * β2/SD2, where β was the regression coefficient for GM and IVs, EAF was the effect allele frequency, K was the number of IVs, N was the sample size, and SD was the standard difference. A weak association between IVs and exposure is recognized when F <10. Third, IVs influence outcomes through exposure only, that is, no horizontal pleiotropy effect of IVs on outcome.
The statistical analyses were performed by using R version 4.2.0 (Institute for Statistics and Mathematics, Vienna, Austria). The R package “TwoSampleMR” was used to explore the potential causal association between GM and T2DM. The statistical significance of evidence for the potential causal effect was indicated by P < 0.05. In the calculation for the causal effect values, inverse variance weighted (IVW) test was used, which is the primary method to obtain unbiased estimates when horizontal pleiotropy was absent. The evaluation indexes were odds ratios (ORs) and 95% confidence intervals (CIs). Cochrane’s Q test was used to test heterogeneity. IVs with P <0.05 were recognized as heterogeneous. When the associations were still significant by Bonferroni’s correction method, that is corrected P < 0.05/n, where n was the number of taxa in different levels, indicating that the potential causal relationship was reliable. The weighted-median method was utilized to provide a robust and consistent estimate of the effect, even if nearly 50% of the genetic variants were invalid instruments. The intercept of MR-Egger regression examined the presence of potential pleiotropy in IVs (P > 0.05 was deemed to have no horizontal pleiotropy). We used robust adjusted profile score (MR-RAPS) analysis to produce reliable inferences about systemic and specific pleiotropy when weak instruments were present.
Figure 2 shows the association of GM abundance at different levels with the risk of T2DM. Basing on the circular chart, we found that there were opposite relationships between different GM taxa and T2DM inside the same level. Table 2 similarly shows the potential causal relationships between GM at different levels and T2DM. To be specific, the increased relative abundances of family Oxalobacteraceae (OR = 1.0704) and genus Oxalobacter (OR = 1.0874) were both significantly associated with higher odds of T2DM, whereas that of species faecis had a negative relationship with T2DM (OR = 0.9460). Moreover, the IVW test suggested that the increased relative abundances of class Betaproteobacteria (OR = 1.1560) and species finegoldii (OR = 1.0493) were linked to higher odds of T2DM, while those of family Lactobacillaceae (OR = 0.9405) and species longum (OR = 0.9158) were associated with lower odds of T2DM. Similarly, Figure 3 clearly reflects the potential causal association from GM at different levels to the odds of T2DM occurrence.
Figure 2 Potential causal relationships of gut microbiota (GM) abundances with the risk of type 2 diabetes mellitus (T2DM). The different colored histograms represent the different levels of GMs. The outward orientation of the column represents the GM as a potential risk factor for T2DM (OR > 1), whereas the inward orientation represents that as a potential protective factor (OR < 1).
Figure 3 Potential causal associations between gut microbiota (GM) in different levels and type 2 diabetes mellitus (T2DM). Potential associations of class, family, genus, and species levels of GM with T2DM assessed using inverse variance weighted, MR-RAPS, and weighted median methods. The dark blue color represents class level, the red color represents family level, the green color represents genus level, and the light blue color represents species level.
Table 3 shows the results of the pleiotropy and heterogeneity tests. We confirmed the impact of relatively accurate MR results, that is, the potential causal relationship of GM with T2DM by the sensitivity analyses. No horizontal pleiotropy and heterogeneity were observed in the potential causal associations between the relative abundances of family Oxalobacteraceae, genus Oxalobacter, and species faecis and the odds of T2DM (MR-Egger P >0.05 and Cochrane’s Q test P >0.05). Besides this, no horizontal pleiotropy and heterogeneity existed in the potential causal relationships between class Betaproteobacteria, species finegoldii, family Lactobacillaceae, and species longum and T2DM (MR-Egger P >0.05, and Cochrane’s Q test P >0.05).
In addition, Table 4 shows the results of the reverse causality analysis. The relative abundance of family Lactobacillaceae had a negative reverse causal association with high odds of T2DM (MR-Egger OR = 0.8236, P = 0.0141); however, no reverse causality has been found between different GM taxa and T2DM.
We conducted a two-sample MR analysis to investigate the potential causal relationship of GM with the occurrence of T2DM. The study results showed that the relative abundances of class Betaproteobacteria, family Oxalobacteraceae, genus Oxalobacter, species faecis, species finegoldii, and species longum had potential causal associations with the odds of T2DM.
In recent years, there was only a limited number of research focused on the relationship of GM with T2DM on the basis of MR method. Yang et al. (2018) used separate-sample MR to obtain estimates of the associations of 27 genera of GM with T2DM and other metabolic diseases, which identified Acidaminococcus, Aggregatibacter, Anaerostipes, Blautia, Desulfovibrio, Dorea, and Faecalibacterium as being nominally associated with T2DM. Xiang et al. (2022) conducted MR analysis to investigate whether GM (in family level) was causally linked to T2DM risk and found that an increased relative abundance of Streptococcaceae was associated with a higher risk of T2DM in the European population. Recently, Sun et al. (2023) performed a two-sample MR study to explore the causal relationship of GM with T2DM, demonstrating that genus Alistipes, genus Allisonella, genus Flavonifractor, and genus Haemophilus acted as defense elements against T2DM, whereas family Clostridiaceae1, family Coriobacteriaceae, genus Actinomyces, and genus Candidatus Soleaferrea were risk factors for T2DM. In clinical practice, since even being placed in the same genus does not guarantee metabolic consistency in the physiological process, the measurement of species and pathway abundance is essential for further investigation on the GM in individuals. Compared with Sun’s research, although we similarly investigated the causal association of GM with T2DM, we further explored these relationships in the species level of GMs and found that the increased relative abundance of finegoldii was associated with higher odds of T2DM (OR = 1.0493). The GM features significantly associated with odds of T2DM which we observed were different and less than those in Sun’s study, which was possibly due to the fact that we set the threshold of IVs selection to P < 1.0 × 10-6 that was stricter. In addition, Bonferroni’s correction method was used to correct the causal relationships of GM with T2DM in our study, which can further correct the bias of multiple testing and made our findings more robust. Herein the difference in genus and species level may be beneficial to the development of microbial agents related to the treatment and prevention of T2DM—for example, specific species of GM can be very helpful in developing ideas on customized or personalized medicine (Popoviciu et al., 2023). However, another MR research on the impact of GM and associated metabolites on cardiometabolic traits, chronic diseases, and human longevity showed that their results cannot support a large causal impact of GM features on T2DM (Gagnon et al., 2023). In conclusion, our results could only indicate that there may be potential causal associations of GM at different levels with T2DM, and further basal and prospective cohort studies are needed to reveal the real roles of GM in the occurence of T2DM in the future.
Specifically, we observed that the increased relative abundance of class Betaproteobacteria, family Oxalobacteraceae, and genus Oxalobacter were all associated with higher odds of T2DM, whereas that of family Lactobacillaceae had a negative causal association with T2DM. The role of Betaproteobacteria in T2DM has not been reported (Larsen et al., 2010; Camargo et al., 2020). However, Blautia and Desulfovibrio in Yang’s study (Yang et al., 2018) and Haemophilus in Sun’s study (Sun et al., 2023) were potential risk factors for T2DM, which all belong to phylum Proteobacteria. A previous cross-sectional study in Japanese adults identified the Blautia genus as a commensal bacterium that is inversely correlated with T2DM, and a possible underlying mechanism was that its amino acid metabolites conferred anti-adipogenesis and anti-inflammatory properties to adipocytes (Hosomi et al., 2022). Another study in obese patients with T2DM found that the relative abundance of Roseburia species was increased after surgery among those achieving diabetes remission (Murphy et al., 2017). Furthermore, Haemophilus may affect the occurrence and development of T2DM via involving the body’s inflammatory response (Brueggemann et al., 2021; Lopez-Lopez et al., 2021). In an animal experiment of whole grain, fermentation affects the GM composition of T2DM; the researchers found that the abundance of family Oxalobacteraceae was increased, whereas genus Lactobacillus was decreased in mice fed a high-fat, high-fructose diet (the T2DM model), which is consistent with our findings (Costabile et al., 2022). Metabolic dysfunction was linked to proportionally higher levels of Proteobacteria (especially Oxalobacteraceae) and decreased Lactobacilli (Nguyen et al., 2015; Do et al., 2018). Nevertheless, the specific mechanisms of these bacteria taking part in T2DM progress are not clear so far, and the population-based studies are still lacking such that we cannot make reliable speculations due to these species differences.
Regarding the species level, finegoldii belongs to the genus Alistipes, which may be pathogenic (Zhao et al., 2020b). We found that the increased abundance of finegoldii was associated with higher odds of T2DM; however, Sun’s study came to the opposite conclusion, that is, the genus Alistipes acted as a defense element against T2DM. According to the animal experiment by He et al. (2022), the probiotic-mediated anti-obesity effect was considered associated with members of Alistipes finegoldii. Another population-based study also showed a negative association between the glycemic parameter and the abundance of Alistipes finegoldii (Companys et al., 2022). The underlying mechanism of the potential causal relationship between Alistipes finegoldii and T2DM has not been clarified. A possible reason for the opposite results between the current study and those in previous studies may be the pathogenesis of T2DM itself. Alistipes has protective effects against various diseases, such as liver fibrosis, cancer immunotherapy, colitis, and cardiovascular disease, but, in contrast, it is pathogenic in colorectal cancer and depression (Parker et al., 2020). In addition, we also found that the increased abundance of faecis and longum was negatively associated with high odds of T2DM. The role of faecis, a type of bacteria from human feces, in the pathogenesis of T2DM is still unknown. Among patients with chronic diseases, such as chronic kidney disease (Lohia et al., 2022), Chrohn’s disease (Bao et al., 2022), and gastrointestinal cancers (Li et al., 2021), the abundance of Roseburia faecis is obeserved to be significantly reduced. The more consistent speculation about the mechanism by which Roseburia faecis play a beneficial role in these diseases is that it can ferment dietary fiber into butyrate, which was considered to be a protector of the gut (Wang et al., 2012). The species longum was also significantly decreased in patients with both T1DM and T2DM, which was associated with the G protein-coupled receptor (GPR) 43 and GPR41 gene expression (Demirci et al., 2022). longum may also lower the levels of fasting blood glucose as well as alleviate insulin resistance in diabetic mice, enhancing the anti-oxidative capacity, increasing the hepatic glycogen content, decreasing the gene expression levels of glucose-6-phosphatase (G6Pase) and phosphoenolpyruvate carboxykinase (PEPCK) in the livers, and thus regulating the disturbance of GM (Hao et al., 2022). Moreover, longum has been made as a variety of pharmaceutical and probiotic supplements in recent years (Zhao et al., 2020a; Gou et al., 2023).
MR is a relatively superior study design to clarify the causal effect of potential risk factors on diseases of interest. By investigating the potential causal association of GM with the occurrence of T2DM, our study may provide some rederences for further exploration on flora regulation methods that benefit prevention and treatment in T2DM in clinical practice, which could effectively reduce the incidence and social burdens of T2DM. Compared to previous MR studies, the current study is more comprehensive because we first included the species level of GM in the analyses. However, there are still some limitations in this study. Our study is limited in the European population, so the causal association between GM and T2DM in other races needs to be further explored. GWAS on GM is still in the initial stage, so that the sample size, as well as the number of SNPs, is relatively small. Due to the small sample size and insufficient efficacy of the microbiome GWAS, there may not be enough IVs for certain bacterial characteristics at the genus or species level. Certain results may be contradicting due to age, dietary patterns, lifestyle behaviors, ethnicity, and geographical location because GM is influenced by multiple factors (Nitzan et al., 2023). However, we could not obtain information on the characteristics of the subjects because it was not available in the MIMIC-IV database.
There was a potential causal association between the relative abundance of GM and the risk of T2DM. The relative abundances of class Betaproteobacteria, family Oxalobacteraceae, genus Oxalobacter, and species finegoldii had positive associations with the occurrence of T2DM, whereas those of species faecis and longum had negative ones. However, certain results that are contradictory with previous findings needed further clarification.
Publicly available datasets were analyzed in this study. This data can be found here: The GWAS Catalog, https://www.ebi.ac.uk/gwas/.
The requirement of ethical approval was waived by Shanghai Municipal Hospital of Traditional Chinese Medicine for the studies involving humans. The studies were conducted in accordance with the local legislation and institutional requirements. The participants provided their written informed consent to participate in this study.
HZ: Conceptualization, Funding acquisition, Project administration, Supervision, Writing – original draft, Writing – review & editing. LM: Data curation, Formal analysis, Funding acquisition, Investigation, Methodology, Writing – review & editing. WP: Data curation, Formal analysis, Investigation, Methodology, Writing – review & editing. BW: Data curation, Formal analysis, Investigation, Methodology, Writing – review & editing. YS: Conceptualization, Supervision, Writing – review & editing.
The author(s) declare financial support was received for the research, authorship, and/or publication of this article. This study was supported by Shaanxi Key Laboratory of Research on TCM Physical Constitution and Diseases Prevention and Treatment (No. KF202310); the National Natural Science Foundation of China (No. 82104649).
The authors declare that the research was conducted in the absence of any commercial or financial relationships that could be construed as a potential conflict of interest.
All claims expressed in this article are solely those of the authors and do not necessarily represent those of their affiliated organizations, or those of the publisher, the editors and the reviewers. Any product that may be evaluated in this article, or claim that may be made by its manufacturer, is not guaranteed or endorsed by the publisher.
Ali, M. K., Pearson-Stuttard, J., Selvin, E., Gregg, E. W. (2022). Interpreting global trends in type 2 diabetes complications and mortality. Diabetologia 65, 3–13. doi: 10.1007/s00125-021-05585-2
Bao, C., Wu, L., Wang, D., Chen, L., Jin, X., Shi, Y., et al. (2022). Acupuncture improves the symptoms, intestinal microbiota, and inflammation of patients with mild to moderate Crohn's disease: A randomized controlled trial. EClinicalMedicine 45, 101300. doi: 10.1016/j.eclinm.2022.101300
Brueggemann, A. B., Jansen van Rensburg, M. J., Shaw, D., McCarthy, N. D., Jolley, K. A., Maiden, M. C. J., et al. (2021). Changes in the incidence of invasive disease due to Streptococcus pneumoniae, Haemophilus influenzae, and Neisseria meningitidis during the COVID-19 pandemic in 26 countries and territories in the Invasive Respiratory Infection Surveillance Initiative: a prospective analysis of surveillance data. Lancet Digit Health 3, e360–e370. doi: 10.1016/S2589-7500(21)00077-7
Camargo, A., Vals-Delgado, C., Alcala-Diaz, J. F., Villasanta-Gonzalez, A., Gomez-Delgado, F., Haro, C., et al. (2020). A diet-dependent microbiota profile associated with incident type 2 diabetes: from the CORDIOPREV study. Mol. Nutr. Food Res. 64, e2000730. doi: 10.1002/mnfr.202000730
Chen, Y., Zhou, J., Wang, L. (2021). Role and mechanism of gut microbiota in human disease. Front. Cell Infect. Microbiol. 11. doi: 10.3389/fcimb.2021.625913
Companys, J., Calderon-Perez, L., Pla-Paga, L., Llaurado, E., Sandoval-Ramirez, B. A., Gosalbes, M. J., et al. (2022). Effects of enriched seafood sticks (heat-inactivated B. animalis subsp. lactis CECT 8145, inulin, omega-3) on cardiometabolic risk factors and gut microbiota in abdominally obese subjects: randomized controlled trial. Eur. J. Nutr. 61, 3597–3611. doi: 10.1007/s00394-022-02904-0
Costabile, A., Corona, G., Sarnsamak, K., Atar-Zwillenberg, D., Yit, C., King, A. J., et al. (2022). Wholegrain fermentation affects gut microbiota composition, phenolic acid metabolism and pancreatic beta cell function in a rodent model of type 2 diabetes. Front. Microbiol. 13. doi: 10.3389/fmicb.2022.1004679
Davies, N. M., Holmes, M. V., Davey Smith, G. (2018). Reading Mendelian randomisation studies: a guide, glossary, and checklist for clinicians. BMJ 362, k601. doi: 10.1136/bmj.k601
Demirci, M., Taner, Z., Keskin, F. E., Ozyazar, M., Kiraz, N., Kocazeybek, B. S., et al. (2022). Similar bacterial signatures in the gut microbiota of type 1 and type 2 diabetes patients and its association with G protein-coupled receptor 41 and 43 gene expression. J. Diabetes Metab. Disord. 21, 1359–1368. doi: 10.1007/s40200-022-01068-2
Do, M. H., Lee, E., Oh, M. J., Kim, Y., Park, H. Y. (2018). High-glucose or -fructose diet cause changes of the gut microbiota and metabolic disorders in mice without body weight change. Nutrients 10 (6), 761. doi: 10.3390/nu10060761
Fan, Y., Pedersen, O. (2021). Gut microbiota in human metabolic health and disease. Nat. Rev. Microbiol. 19, 55–71. doi: 10.1038/s41579-020-0433-9
Gagnon, E., Mitchell, P. L., Manikpurage, H. D., Abner, E., Taba, N., Esko, T., et al. (2023). Impact of the gut microbiota and associated metabolites on cardiometabolic traits, chronic diseases and human longevity: a Mendelian randomization study. J. Transl. Med. 21, 60. doi: 10.1186/s12967-022-03799-5
Gill, P. A., Inniss, S., Kumagai, T., Rahman, F. Z., Smith, A. M. (2022). The role of diet and gut microbiota in regulating gastrointestinal and inflammatory disease. Front. Immunol. 13. doi: 10.3389/fimmu.2022.866059
Gou, G. H., Liu, L., Abdubakiev, S., Xin, X. L., Akber Aisa, H., Li, J. (2023). Anti-diabetic effects and molecular mechanisms of amide alkaloids from piper longum based on network pharmacology integrated with cellular assays. Chem. Biodivers 20, e202200904. doi: 10.1002/cbdv.202200904
Gurung, M., Li, Z., You, H., Rodrigues, R., Jump, D. B., Morgun, A., et al. (2020). Role of gut microbiota in type 2 diabetes pathophysiology. EBioMedicine 51, 102590. doi: 10.1016/j.ebiom.2019.11.051
Hao, J., Zhang, Y., Wu, T., Liu, R., Sui, W., Zhu, J., et al. (2022). The antidiabetic effects of Bifidobacterium longum subsp. longum BL21 through regulating gut microbiota structure in type 2 diabetic mice. Food Funct. 13, 9947–9958. doi: 10.1039/D2FO01109C
He, Q., Zhang, Y., Ma, D., Zhang, W., Zhang, H. (2022). Lactobacillus casei Zhang exerts anti-obesity effect to obese glut1 and gut-specific-glut1 knockout mice via gut microbiota modulation mediated different metagenomic pathways. Eur. J. Nutr. 61, 2003–2014. doi: 10.1007/s00394-021-02764-0
Hosomi, K., Saito, M., Park, J., Murakami, H., Shibata, N., Ando, M., et al. (2022). Oral administration of Blautia wexlerae ameliorates obesity and type 2 diabetes via metabolic remodeling of the gut microbiota. Nat. Commun. 13, 4477. doi: 10.1038/s41467-022-32015-7
Iatcu, C. O., Steen, A., Covasa, M. (2021). Gut microbiota and complications of type-2 diabetes. Nutrients 14 (1), 166. doi: 10.3390/nu14010166
International Diabetes Federation (IDF) (2022). IDF Diabetes Atlas. 10th ed (Brussels: International Diabetes Federation (IDF). Available at: https://diabetesatlas.org/atlas/tenth-edition/.
Kurilshikov, A., Medina-Gomez, C., Bacigalupe, R., Radjabzadeh, D., Wang, J., Demirkan, A., et al. (2021). Large-scale association analyses identify host factors influencing human gut microbiome composition. Nat. Genet. 53, 156–165. doi: 10.1038/s41588-020-00763-1
Larsen, N., Vogensen, F. K., van den Berg, F. W., Nielsen, D. S., Andreasen, A. S., Pedersen, B. K., et al. (2010). Gut microbiota in human adults with type 2 diabetes differs from non-diabetic adults. PloS One 5, e9085. doi: 10.1371/journal.pone.0009085
Li, N., Bai, C., Zhao, L., Sun, Z., Ge, Y., Li, X. (2021). The relationship between gut microbiome features and chemotherapy response in gastrointestinal cancer. Front. Oncol. 11. doi: 10.3389/fonc.2021.781697
Lohia, S., Vlahou, A., Zoidakis, J. (2022). Microbiome in chronic kidney disease (CKD): an omics perspective. Toxins (Basel) 14 (3), 176. doi: 10.3390/toxins14030176
Lopera-Maya, E. A., Kurilshikov, A., van der Graaf, A., Hu, S., Andreu-Sanchez, S., Chen, L., et al. (2022). Effect of host genetics on the gut microbiome in 7,738 participants of the Dutch Microbiome Project. Nat. Genet. 54, 143–151. doi: 10.1038/s41588-021-00992-y
Lopez-Lopez, N., Gil-Campillo, C., Diez-Martinez, R., Garmendia, J. (2021). Learning from -omics strategies applied to uncover Haemophilus influenzae host-pathogen interactions: Current status and perspectives. Comput. Struct. Biotechnol. J. 19, 3042–3050. doi: 10.1016/j.csbj.2021.05.026
Martino, C., Dilmore, A. H., Burcham, Z. M., Metcalf, J. L., Jeste, D., Knight, R. (2022). Microbiota succession throughout life from the cradle to the grave. Nat. Rev. Microbiol. 20, 707–720. doi: 10.1038/s41579-022-00768-z
Murphy, R., Tsai, P., Jullig, M., Liu, A., Plank, L., Booth, M. (2017). Differential changes in gut microbiota after gastric bypass and sleeve gastrectomy bariatric surgery vary according to diabetes remission. Obes. Surg. 27, 917–925. doi: 10.1007/s11695-016-2399-2
Nguyen, T. L., Vieira-Silva, S., Liston, A., Raes, J. (2015). How informative is the mouse for human gut microbiota research? Dis. Model. Mech. 8, 1–16. doi: 10.1242/dmm.017400
Nitzan, Z., Staun-Ram, E., Volkowich, A., Miller, A. (2023). Multiple sclerosis-associated gut microbiome in the Israeli diverse populations: associations with ethnicity, gender, disability status, vitamin D levels, and mediterranean diet. Int. J. Mol. Sci. 24, 15024. doi: 10.3390/ijms241915024
Parker, B. J., Wearsch, P. A., Veloo, A. C. M., Rodriguez-Palacios, A. (2020). The genus alistipes: gut bacteria with emerging implications to inflammation, cancer, and mental health. Front. Immunol. 11. doi: 10.3389/fimmu.2020.00906
Popoviciu, M. S., Kaka, N., Sethi, Y., Patel, N., Chopra, H., Cavalu, S. (2023). Type 1 diabetes mellitus and autoimmune diseases: A critical review of the association and the application of personalized medicine. J. Pers. Med. 13, 422. doi: 10.3390/jpm13030422
Sanna, S., van Zuydam, N. R., Mahajan, A., Kurilshikov, A., Vich Vila, A., Vosa, U., et al. (2019). Causal relationships among the gut microbiome, short-chain fatty acids and metabolic diseases. Nat. Genet. 51, 600–605. doi: 10.1038/s41588-019-0350-x
Sekula, P., Del Greco, M. F., Pattaro, C., Kottgen, A. (2016). Mendelian randomization as an approach to assess causality using observational data. J. Am. Soc. Nephrol. 27, 3253–3265. doi: 10.1681/ASN.2016010098
Skrivankova, V. W., Richmond, R. C., Woolf, B. A. R., Yarmolinsky, J., Davies, N. M., Swanson, S. A., et al. (2021). Strengthening the reporting of observational studies in epidemiology using mendelian randomization: the STROBE-MR statement. JAMA 326, 1614–1621. doi: 10.1001/jama.2021.18236
Sun, K., Gao, Y., Wu, H., Huang, X. (2023). The causal relationship between gut microbiota and type 2 diabetes: a two-sample Mendelian randomized study. Front. Public Health 11. doi: 10.3389/fpubh.2023.1255059
Vieira-Silva, S., Falony, G., Darzi, Y., Lima-Mendez, G., Garcia Yunta, R., Okuda, S., et al. (2016). Species-function relationships shape ecological properties of the human gut microbiome. Nat. Microbiol. 1, 16088. doi: 10.1038/nmicrobiol.2016.88
Wang, T., Cai, G., Qiu, Y., Fei, N., Zhang, M., Pang, X., et al. (2012). Structural segregation of gut microbiota between colorectal cancer patients and healthy volunteers. ISME J. 6, 320–329. doi: 10.1038/ismej.2011.109
Xiang, K., Zhang, J. J., Xu, Y. Y., Zhong, X., Ni, J., Pan, H. F. (2022). Genetically predicted causality of 28 gut microbiome families and type 2 diabetes mellitus risk. Front. Endocrinol. (Lausanne) 13. doi: 10.3389/fendo.2022.780133
Yang, G., Wei, J., Liu, P., Zhang, Q., Tian, Y., Hou, G., et al. (2021). Role of the gut microbiota in type 2 diabetes and related diseases. Metabolism 117, 154712. doi: 10.1016/j.metabol.2021.154712
Yang, Q., Lin, S. L., Kwok, M. K., Leung, G. M., Schooling, C. M. (2018). The roles of 27 genera of human gut microbiota in ischemic heart disease, type 2 diabetes mellitus, and their risk factors: A mendelian randomization study. Am. J. Epidemiol. 187, 1916–1922. doi: 10.1093/aje/kwy096
Yuan, S., Larsson, S. C. (2022). Coffee and caffeine consumption and risk of kidney stones: A mendelian randomization study. Am. J. Kidney Dis. 79, 9–14.e11. doi: 10.1053/j.ajkd.2021.04.018
Yuan, S., Merino, J., Larsson, S. C. (2023). Causal factors underlying diabetes risk informed by Mendelian randomisation analysis: evidence, opportunities and challenges. Diabetologia 66, 800–812. doi: 10.1007/s00125-023-05879-7
Zhao, R., Coker, O. O., Wu, J., Zhou, Y., Zhao, L., Nakatsu, G., et al. (2020b). Aspirin reduces colorectal tumor development in mice and gut microbes reduce its bioavailability and chemopreventive effects. Gastroenterology 159, 969–983.e964. doi: 10.1053/j.gastro.2020.05.004
Keywords: gut microbiota, type 2 diabetes mellitus, Mendelian randomization, causal association, species
Citation: Zhang H, Ma L, Peng W, Wang B and Sun Y (2024) Association between gut microbiota and onset of type 2 diabetes mellitus: a two-sample Mendelian randomization study. Front. Cell. Infect. Microbiol. 14:1327032. doi: 10.3389/fcimb.2024.1327032
Received: 24 October 2023; Accepted: 07 March 2024;
Published: 26 March 2024.
Edited by:
Yolanda López-Vidal, National Autonomous University of Mexico, MexicoReviewed by:
Cosmin Mihai Vesa, University of Oradea, RomaniaCopyright © 2024 Zhang, Ma, Peng, Wang and Sun. This is an open-access article distributed under the terms of the Creative Commons Attribution License (CC BY). The use, distribution or reproduction in other forums is permitted, provided the original author(s) and the copyright owner(s) are credited and that the original publication in this journal is cited, in accordance with accepted academic practice. No use, distribution or reproduction is permitted which does not comply with these terms.
*Correspondence: Yongning Sun, WW9uZ25zODUwMjE0QDE2My5jb20=
Disclaimer: All claims expressed in this article are solely those of the authors and do not necessarily represent those of their affiliated organizations, or those of the publisher, the editors and the reviewers. Any product that may be evaluated in this article or claim that may be made by its manufacturer is not guaranteed or endorsed by the publisher.
Research integrity at Frontiers
Learn more about the work of our research integrity team to safeguard the quality of each article we publish.