- 1Department of Endocrinology and Metabolism, The First Hospital of Jilin University, Jilin, Changchun, China
- 2Department of Gastroenterology, The First Hospital of Jilin University, Jilin, Changchun, China
Background: Graves’ disease (GD) is the most common cause of hyperthyroidism, and its pathogenesis remains incompletely elucidated. Numerous studies have implicated the gut microbiota in the development of thyroid disorders. This study employs Mendelian randomization analysis to investigate the characteristics of gut microbiota in GD patients, aiming to offer novel insights into the etiology and treatment of Graves’ disease.
Methods: Two-sample Mendelian randomization (MR) analysis was employed to assess the causal relationship between Graves’ disease and the gut microbiota composition. Gut microbiota data were sourced from the international consortium MiBioGen, while Graves’ disease data were obtained from FINNGEN. Eligible single nucleotide polymorphisms (SNPs) were selected as instrumental variables. Multiple analysis methods, including inverse variance-weighted (IVW), MR-Egger regression, weighted median, weighted mode, and MR-RAPS, were utilized. Sensitivity analyses were conducted employing MR-Egger intercept test, Cochran’s Q test, and leave-one-out analysis as quality control measures.
Results: The Mendelian randomization study conducted in a European population revealed a decreased risk of Graves’ disease associated with Bacteroidaceae (Odds ratio (OR) [95% confidence interval (CI)]: 0.89 [0.89 ~ 0.90], adjusted P value: <0.001), Bacteroides (OR: [95% CI]: 0.555 [0.437 ~ 0.706], adjusted P value: <0.001), and Veillonella (OR [95% CI]: 0.632 [0.492 ~ 0.811], adjusted P value: 0.016). No significant evidence of heterogeneity, or horizontal pleiotropy was detected. Furthermore, the preliminary MR analysis identified 13 bacterial species including Eubacterium brachy group and Family XIII AD3011 group, exhibiting significant associations with Graves’ disease onset, suggesting potential causal effects.
Conclusion: A causal relationship exists between gut microbiota and Graves’ disease. Bacteroidaceae, Bacteroides, and Veillonella emerge as protective factors against Graves’ disease development. Prospective probiotic supplementation may offer a novel avenue for adjunctive treatment in the management of Graves’ disease in the future.
1 Introduction
Graves’ disease(GD) is an autoimmune disorder and the most prevalent cause of hyperthyroidism, with an age peak of onset between 30 and 50 years (Smith and Hegedus, 2016). The incidence of GD varies by region and gender (Tellez et al., 1992). Clinical manifestations of GD encompass symptoms of hyperthyroidism, such as weight loss, palpitations, fatigue, and tremor, with some patients displaying thyroid-associated orbitopathy (Smith and Hegedus, 2016). Skin manifestations and clubbing of fingers are observed in 1-4% of GD patients, often accompanied by severe thyroid-associated orbitopathy (Fatourechi, 2012). Graves’ Disease can affect multiple systems throughout the body, significantly impacting patients’ quality of life, and in severe cases, may lead to life-threatening events such as thyrotoxic crisis. The exact etiology of GD remains elusive, although it is widely accepted that a combination of genetic, autoimmune, and environmental factors contributes to its development (Lee et al., 2015; Rayman, 2019).
The adult gut harbors 50 bacterial phyla and about 100–1000 bacterial species, encoding genes that are 150 times the size of the human genome (Gill et al., 2006; Adak and Khan, 2019). These gut microbiota play pivotal roles in maintaining host nutrition, metabolism, and immune equilibrium (Kho and Lal, 2018). Dysregulation of the gut ecosystem may contribute to autoimmune and metabolic disorders, including inflammatory bowel disease (Nishino et al., 2018), irritable bowel syndrome (Cao, 2018), obesity (Schwiertz et al., 2010), chronic kidney disease (Sircana et al., 2019), and cardiovascular disease (Jie et al., 2017). In recent years, the thyroid-gut axis has garnered increasing research attention, although its precise mechanisms remain to be fully elucidated (Lerner et al., 2017).
Alterations in gut microbiota composition could potentially exert influences on thyroid function. Gong et al. conducted a meta-analysis revealing reduced abundance of beneficial bacteria such as Bifidobacterium and Lactobacillus in autoimmune thyroid diseases (AITD), accompanied by a significant increase in detrimental microbial groups like Bacteroides fragilis (Gong et al., 2021). Yang et al. demonstrated elevated abundance of Firmicutes, Proteobacteria and Actinobacillus in GD patients compared to controls, with a notably higher Firmicutes/Bacteroidetes ratio (Yang et al., 2019). Biscarini et al. identified significant differences in the composition of the gut microbiota in GD/GO mouse models developed at various centers, suggesting a potential association with TSHR-induced disease heterogeneity (Masetti et al., 2018). To further confirm a causal relationship, Moshkelgosha et al. subsequently modified the gut microbiota based on this finding to determine its role in thyroid autoimmunity and ultimately demonstrated the critical role of gut microbiota in the development of TSHR-induced diseases (Moshkelgosha et al., 2021). This discovery was validated in a multicenter study conducted by Biscarini et al. (2023). Moreover, a prospective study similarly indicated distinctive features in the gut microbiota of GD patients, which might be linked to imbalances in the immune system and gut microbiota (Deng et al., 2023). These studies provide compelling evidence for a causal relationship between gut microbiota and GD.
Controversies persist regarding the mechanisms by which gut microbiota influence GD. The impact of gut microbiota on the thyroid could potentially occur through several main avenues: Intestinal dysbiosis leading to impaired gut barrier function and increased intestinal permeability, facilitating antigen entry into circulation and immune system activation (Cayres et al., 2021). Antibodies in circulation may react with bacterial antigens, enhancing the activation of inflammatory foci within the thyroid (Tomasello et al., 2015). In addition, Short Chain Fatty Acids (SCFAs) are the principal products of dietary fiber fermentation by gut microbiota, including acetate, propionate, and butyrate. Beyond regulating intestinal functions, SCFAs also exert regulatory effects on host metabolism and immune responses (den Besten et al., 2013). After absorption by intestinal epithelial cells, SCFAs partly regulate intestinal function within the cells, while the remainder exerts systemic effects through the bloodstream, modulating glucose and fat metabolism, as well as immune system regulation (Kim, 2021), including inhibition of histone deacetylase (HDAC) (Licciardi et al., 2011), G protein-coupled receptor (GPR) signaling, and acetyl-CoA production and metabolic integration. Relevant research suggests that SCFAs can enhance immune responses, and elevated SCFA levels have been linked to improved colitis (Scheppach et al., 1992; Ananthakrishnan et al., 2013), autoimmune neuroinflammation (Haghikia et al., 2016), renal inflammation (Andrade-Oliveira et al., 2015), and atherosclerosis. For instance, butyrate (a type of SCFA) can reduce TNF-α and IL-6 levels, and inhibit NLRP10 inflammasome activation through GPR3A (Pan et al., 2019).
Hence, this study employs Two-sample Mendelian randomization to investigate the causal relationship between gut microbiota and GD, aiming to provide evidence for the pathogenesis of GD and offer insights into its future treatment. Genetic variants (SNPs) associated with gut microbiota were selected as instrumental variables (IVs). Gut microbiota data were sourced from the international consortium MiBioGen, while Graves’ disease data were obtained from FINNGEN. Multiple analysis methods, including inverse variance-weighted (IVW), MR-Egger regression, weighted median, weighted mode, and MR-RAPS, were utilized. Sensitivity analyses were conducted employing MR-Egger intercept test, Cochran’s Q test, and leave-one-out analysis as quality control measures.
2 Methods
2.1 Data source
In this study, the gut microbiota’s genome-wide association study (GWAS) data were obtained from the international consortium MiBioGen. This database harmonized 16S rRNA gene sequencing profiles and genotyping data of 18,340 participants across 24 cohorts from the United States, Canada, Israel, South Korea, Germany, Denmark, the Netherlands, Belgium, Sweden, Finland, and the United Kingdom. It conducted a large-scale, multi-ethnic, whole-genome meta-analysis of autosomal human genetic variation and its association with gut microbiota composition (Kurilshikov et al., 2021). The GWAS dataset for GD was obtained from FINNGEN, a Finnish database comprising samples from 281,683 individuals, with 1,828 individuals having Graves’ disease and 279,855 individuals in the control group (Kurki et al., 2023). Given the disparities in racial and population stratification, populations of both exposure and outcome datasets were of European ancestry, encompassing both males and females, thereby mitigating biases due to population and gender stratification (Emdin et al., 2017). Detailed information on the dataset is provided in Table 1.
2.2 Study design
Two-sample Mendelian randomization (TSMR) is a genetic-level causal study that utilizes GWAS summary data from two distinct datasets. In one-sample Mendelian randomization studies where both the exposure and outcome datasets originate from the same database, the likelihood of false positives increases due to the “winner’s curse” and the increased probability of weak instrumental variables (Jiang et al., 2023). Conversely, in two-sample Mendelian randomization analyses where the genetic associations with exposure and outcome are derived from independent samples, the opportunistic correlations vary between datasets. This variation independently influences the associations with exposure and outcome, with the bias due to weaker instruments tending towards zero. In this study, we employed a two-sample Mendelian randomization (MR) approach to explore the causal impact of gut microbiota on GD, as illustrated in Figure 1. Genetic variants (SNPs) linked to gut microbiota were chosen as instrumental variables (IVs). Significant instrumental variables will be extracted from the gut microbiome dataset, and their associations with GD will be identified in the GD dataset. Subsequently, a range of MR analytical methods will be applied for causal investigation. Additionally, various sensitivity analyses will be conducted to assess the robustness of the results.
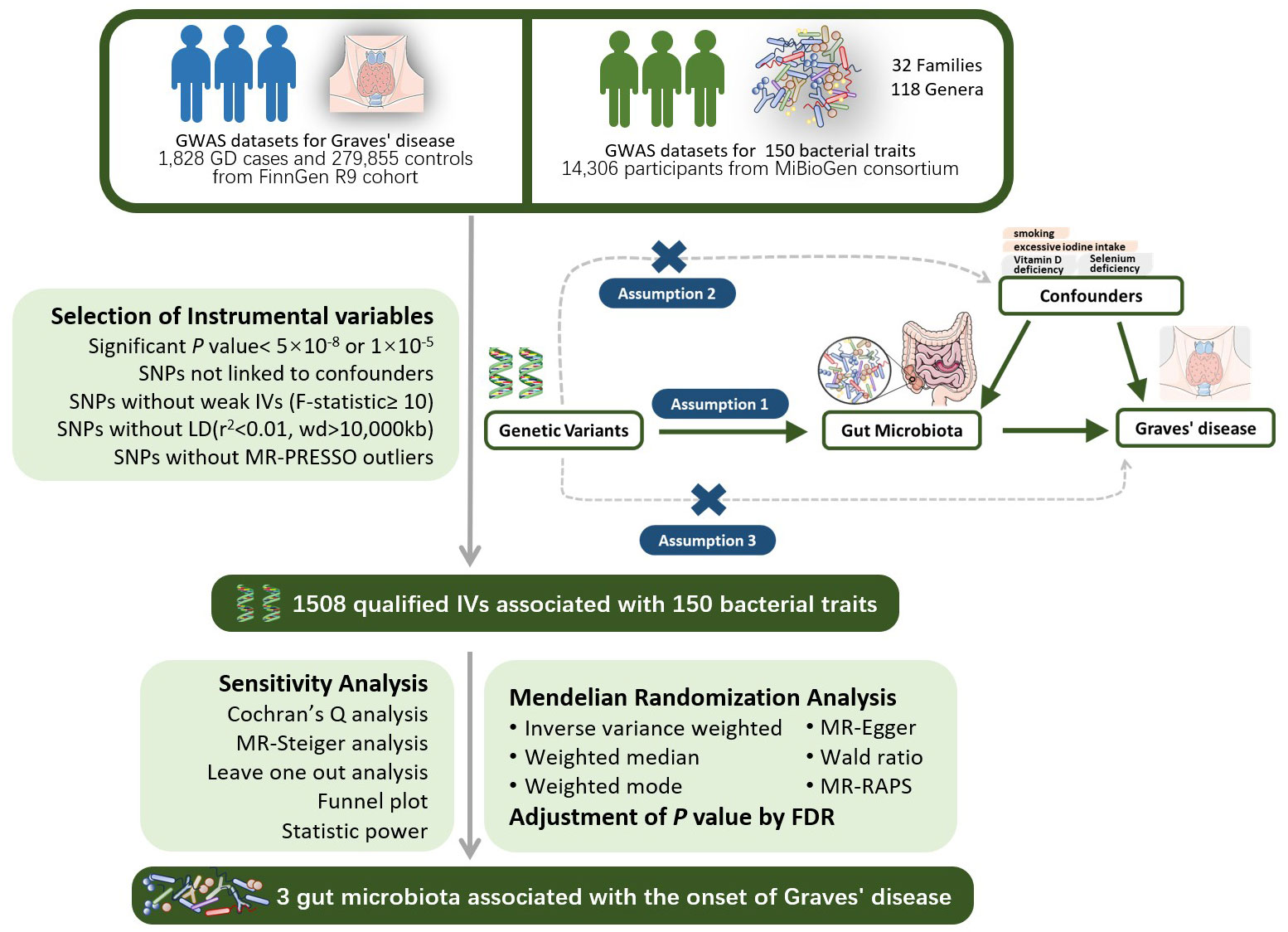
Figure 1 The design of the two-sample MR study for the association of Gut Microbiota on Graves’ disease. GWAS, Genome-wide association study; LD, linkage disequi-librium; MR-PRESSO, Mendelian Randomization Pleiotropy RESidual Sum and Outlier, a method test the pleiotropic biases in the SNPs and correct the pleiotropic effects; MR, Mendelian randomization; SNP, single nucleotide polymorphism, as instrumental variables for the exposures and outcomes; FDR, false discovery rate.
MR analysis must satisfy the following three assumptions: 1) The relevance assumption: A strong association between instrumental variables and the exposure factor (P<5×10-8 or P<1×10-5) is presumed; 2) The independence assumption: Instrumental variables must be independent of confounding factors influencing exposure and outcome; 3) The exclusion restriction assumption: Instrumental variables must affect the outcome variable solely through the exposure factor and not directly.
2.3 Selection of instrumental variables
Based on the aforementioned study design and assumptions, we initially extracted SNPs significantly associated with the exposure at a genome-wide significance level (alpha=5×10-8). For batches where extraction was not feasible, we reduced the threshold to alpha=1×10-5 to ensure an adequate number of SNPs. We set r2 < 0.01 and kb=10000 as the criteria for removing linkage disequilibrium (LD), ensuring the independence of SNPs and mitigating the risk of multicollinearity. The strength indicator F value (F=β2/se2) was calculated for each SNP, with F<10 considered weak instrumental variables that fail to satisfy assumption one and were thus excluded (Burgess et al., 2017). We extracted outcome data from the outcome dataset based on significant SNP and performed data matching. Ambiguous and palindromic SNPs were excluded during the matching process. The PhenoScanner database (Staley et al., 2016) was employed to search for each SNP, excluding those associated with confounding factors such as smoking (Wiersinga, 2013; Antonelli et al., 2020) and excessive iodine intake (Burgi, 2010). The MR-PRESSO test was utilized to detect outliers with horizontal pleiotropy, leading to the exclusion of outlier SNPs. MR-Steiger analysis was applied to assess the causal direction of all SNPs and SNPs with erroneous directions were removed (Li et al., 2022). Finally, we employed Bonferroni correction (P < 0.05/n, n referring to the number of remaining SNPs) to eliminate SNPs directly associated with the outcome. Following the rigorous screening outlined above, the remaining SNPs were deemed qualified instrumental variables.
2.4 MR analysis
We employed a variety of MR methods to calculate the causal relationship between gut microbiota and Graves’ disease, including IVW method, MR-Egger regression, weighted median, weighted mode, and MR-PAPS method.
Firstly, the effect size of each SNP on causal estimation was assessed using the Wald ratio, which represents the ratio of the individual SNP’s impact on the outcome to its impact on the risk factor under the assumption of linearity (Thomas and Conti, 2004). The IVW method is a weighted linear regression without an intercept term, where the slope parameter represents the causal estimate, and the weight is the inverse of the genetic association variance divided by the squared standard error of the outcome (Burgess et al., 2013). Following Mendelian randomization guidelines, we opted for the multiplicative random-effects model as the primary analysis in the absence of horizontal pleiotropy and heterogeneity (Burgess et al., 2019). Weighted median and weighted mode methods are common consensus methods. They compute the causal effect based on the majority valid assumption and the plurality valid assumption, respectively (Bowden et al., 2016a; Burgess et al., 2019). When there is heterogeneity between SNPs, both the weighted median and IVW methods need to support the significant conclusion. The MR-Egger method is similar to the IVW method but includes an intercept term in the regression model (Burgess et al., 2017). The MR-Egger method provides consistent causal effect estimates under the assumption of internal stability and unbiasedness. The intercept term in MR-Egger also offers a test for horizontal pleiotropy among IVs. In case of horizontal pleiotropy among SNPs, we employed the MR-Egger method as the primary analysis.MR-RAPS method estimates causal effects using a probabilistic profile likelihood function assuming that pleiotropy follows a normal distribution centered at zero with a location variance, enhancing robustness against outliers (Zhao et al., 2020). Finally, a False Discovery Rate (FDR) correction was applied to MR results. Causal relationships were inferred based on significant P-values < 0.05.
2.5 Sensitivity analysis
Cochran’s Q test was employed to assess heterogeneity among IVs, considering SNPs with Q test P-value < 0.05 as heterogeneous. Furthermore, we used the MR-Steiger model to validate the overall direction of estimates for result robustness (Hemani et al., 2017). To ascertain the influence of strong effect SNPs, we conducted a leave-one-out sensitivity test. The Instrument Strength Independent of Direct Effect (InSIDE) assumption (Burgess and Thompson, 2017) and the No Measurement Error (NoME) hypothesis (Bowden et al., 2016b) must be satisfied for MR-Egger regression. We constructed a funnel plot and calculated the I2 statistic to validate these assumptions. A correction for causal estimates is required when I2 < 90% and the primary analytical method is MR-Egger (Bowden et al., 2016b). Sample size analysis is particularly crucial for determining whether negative results truly indicate the absence of a causal relationship. We calculated the statistical power following Burgess’s method (Burgess, 2014).
2.6 Visualization
Scatter plots and regression curve plots were generated for each set of MR analyses in this study, along with forest plots illustrating SNP effects. These visualizations will be presented in the results. Additionally, a circular heatmap and forest plot were constructed for the overall results. A Manhattan plot was created for significant gut microbiome GWAS data to elucidate relevant SNP information. Several figures were partly generated using Servier Medical Art (smart.servier.com), provided by Servier, licensed under a Creative Commons Attribution 3.0 unported license.
2.7 Statistical analysis software
All statistical analyses and visualizations in this study were conducted using R software (version 4.1.2) with packages including “TwoSampleMR,” “MR-PRESSO,” “mr.raps,” “forestploter,” and several foundational R packages.
3 Results
3.1 Selection of instrumental variables
Initially, a total of 1918 SNPs related to gut microbiome were screened, and no weak instruments with F<10 were identified. 97 SNPs were excluded due to missing data in the outcome dataset, 294 SNPs were removed as ambiguous or palindromic SNPs during dataset integration, and 6 SNPs were deleted based on PhenoScanner search indicating associations with confounders such as smoking and vitamin D deficiency. MR-PRESSO test identified 11 SNPs with horizontal pleiotropy. After Bonferroni correction, 2 SNPs directly related to the outcome were removed.
Ultimately, 1508 eligible SNPs were included in the study.
3.2 Altered abundance of gut microbiota affects GD incidence
Detailed information regarding the MR study is summarized in the Supplementary Material. In this study, taxonomic families or genera of 150 gut microbiota were included for analysis. The number of SNPs per type of gut microbiota ranged from 1 to 40. Supplementary Information provides detailed IVs information for the 150 gut microbiota. Manhattan plots (Figure 2) were generated for significant genera, providing clarity on relevant SNP information. Through MR analysis, we identified 3 gut microbiota family/genera with a protective effect on GD incidence, while 13 genera were found to potentially influence GD incidence. Results of the MR analysis are depicted in the circular heatmap (Figure 3) and forest plot (Figure 4).
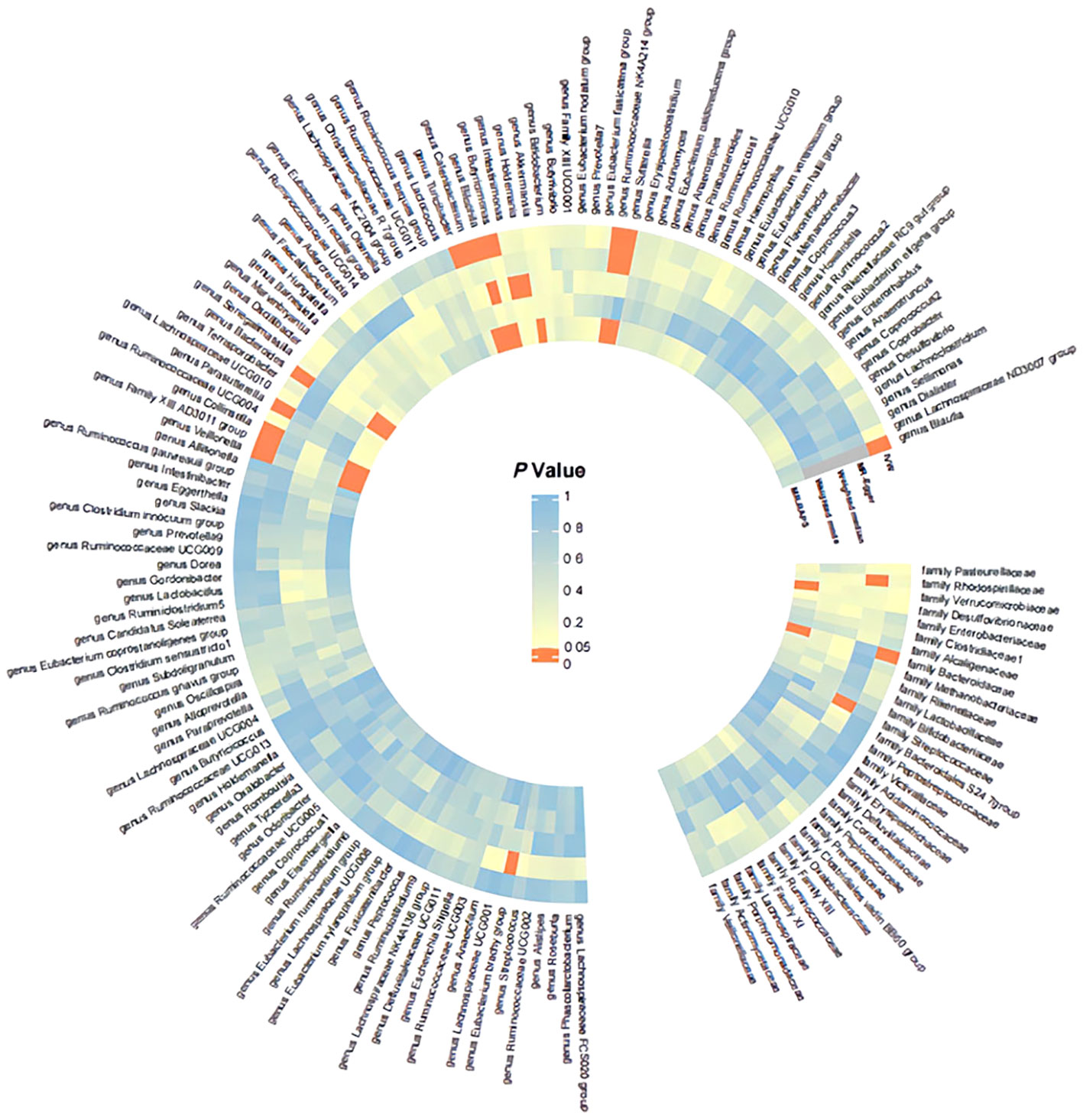
Figure 3 Significance Heatmap of MR Analysis. IVW, inverse variance weighted; MR, Mendelian randomization; RAPS, Robust Adjusted Profile Score.
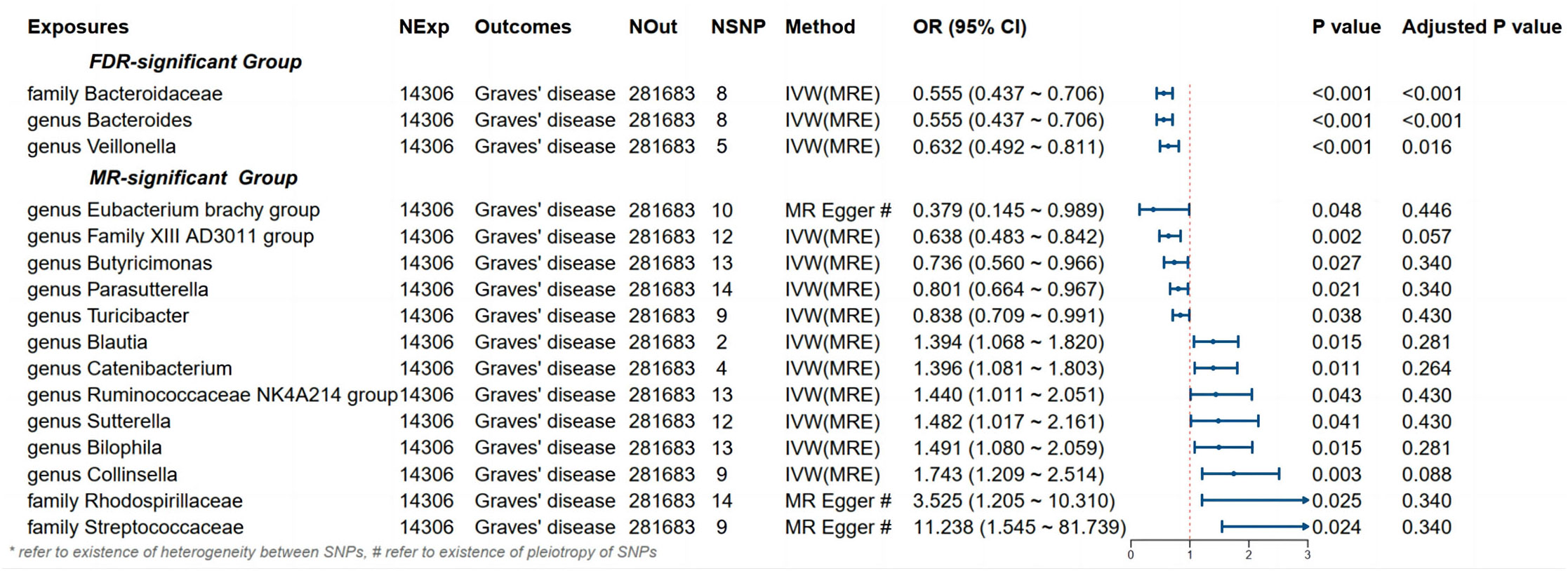
Figure 4 Results and forest plot of the significant MR analysis. IVW(MRE), inverse variance weighted (multiplicative random effects model); CI, confidence interval; NExp, sample size of exposure dataset; NOut, sample size of outcome dataset; NSNP, number of SNP included in MR analysis; * refer to existence of heterogeneity of SNPs, # refer to existence of pleiotropy between SNPs.
3.3 Positive results
MR analysis revealed a significant causal effect of the family Bacteroidaceae, genus Bacteroides, and genus Veillonella on GD incidence. The family Bacteroidaceae (OR (95%CI): 0.89 (0.89 ~ 0.90), adjusted P value: <0.001), the genus Bacteroides (OR (95%CI): 0.555 (0.437 ~ 0.706), adjusted P value: <0.001), and the genus Veillonella (OR (95%CI): 0.632 (0.492 ~ 0.811), adjusted P value: 0.016) were associated with reduced risk of GD incidence (Figure 4). Cochran’s Q-test and MR-Egger intercept showed no evidence of potential heterogeneity or pleiotropy bias in our findings (all P values > 0.05, Table 2).
3.4 Other results
Despite not passing FDR multiple testing correction, 13 bacterial types, including the Eubacterium brachy group, showed significant results in the initial MR analysis, indicating potential causal effects on GD incidence (Figure 4). Among them, Eubacterium brachy group (OR [95%CI]: 0.379 (0.145 ~ 0.989), P value: 0.048), Family XIII AD3011 group (OR [95%CI]: 0.638 (0.483 ~ 0.842), P value: 0.002), Butyricimonas (OR [95%CI]: 0.736 (0.560 ~ 0.966), P value: 0.027), Parasutterella (OR [95%CI]: 0.801 (0.664 ~ 0.967), P value: 0.021) and Turicibacter (OR [95%CI]: 0.838 (0.709 ~ 0.991), P value: 0.038) showed a potential reduced risk of GD incidence. Bilophila (OR [95%CI]: 1.394 (1.068 ~ 1.820), P value: 0.015), Catenibacterium (OR [95%CI]: 1.396 (1.081 ~ 1.803), P value: 0.011), Ruminococcaceae NK4A214 group (OR [95%CI]: 1.440 (1.011 ~ 2.051), P value: 0.043), Sutterella (OR [95%CI]: 1.482 (1.017 ~ 2.161), P value: 0.041), Blautia (OR [95%CI]: 1.491 (1.080 ~ 2.059), P value: 0.015), Collinsella (OR [95%CI]: 1.743 (1.209 ~ 2.514), P value: 0.003), Rhodospirillaceae (OR [95%CI]: 3.525 (1.205 ~ 10.310), P value: 0.025) and Streptococcaceae (OR [95%CI]: 11.238 (1.545 ~ 81.739), P value: 0.024) showed a potential increased risk of GD incidence. According to Cochran’s Q test, there is no evidence of heterogeneity (All P values > 0.05, Table 2).
However, based on the MR-Egger intercept, Eubacterium brachy group (MR-Egger intercept P value: 0.040), Rhodospirillaceae (MR-Egger intercept P value: 0.036) and Streptococcaceae (MR-Egger intercept P value: 0.024) exhibit pleiotropy, and MR-Egger will be used as the primary analytical method.
4 Discussion
Although previous studies have consistently demonstrated the impact of gut microbiota on Graves’ disease, there is still controversy regarding changes in gut microbiota abundance in GD patients. Most studies indicate a significant decrease in gut microbiota abundance in GD patients compared to healthy individuals, this aligns with our findings. For instance, Deng et al. analyzed the gut microbiota of Graves’ disease patients and controls and found that GD patients exhibited lower gut bacterial diversity (Deng et al., 2023). However, some studies have reported no significant changes in gut microbiota abundance and diversity in GD patients (Yang et al., 2019; Chang et al., 2021), which requires further investigation for confirmation. The Firmicutes and Bacteroidetes ratio (F/B) is believed to play a significant role in maintaining normal gut homeostasis. Variations in the F/B ratio are indicative of gut ecological imbalance and, to some extent, reflect human health status. An increased F/B ratio may suggest dysbiosis in obese patients (Magne et al., 2020), while a decreased F/B ratio is observed in certain autoimmune diseases such as systemic lupus erythematosus and inflammatory bowel disease (De Luca and Shoenfeld, 2019; Stojanov et al., 2020). A meta-analysis indicated alterations in the diversity and abundance of certain gut microbiota in patients with autoimmune thyroid disease (AITD) compared to control groups, including a decrease in Firmicutes abundance and an increase in Bacteroidetes abundance (i.e., decreased F/B ratio) (Gong et al., 2021); Studies by El-Zawawy et al. (2021), and Su et al. (2020) also suggest a decreased abundance of Firmicutes and an increased abundance of Bacteroidetes in GD patients, associated with a lower F/B ratio. However, Yang et al. (2019) found no significant differences in gut microbiota abundance between GD patients and controls.
Mendelian randomization studies can establish definite causal relationships in the absence of confounding factors. In our study, we identified a decreased risk of GD with the increased abundance of Bacteroides in Bacteroidetes and Veillonella in Firmicutes.
Previous research has yielded varying results regarding specific changes in gut microbiota among GD patients. Hafiz et al (Ishaq et al., 2018). recruited 27 GD patients and 11 healthy controls, collected and analyzed fecal samples, and found significantly increased relative abundances of Prevotellaceae and Pasteurellaceae in GD patients compared to controls, while Enterobacteriaceae, Veillonellaceae, and Rikenellaceae were significantly decreased in GD patients. At the genus level, GD patients exhibited significant increases in Prevotella 9 and Haemophilus, while Alistipes and Faecalibacterium were significantly decreased. Yang et al. (2019) found higher abundances of Oribacterium, Lactobacillus, Aggregatibacter, and Mogibacterium genera in GD patients compared to healthy controls. Additionally, GD patients exhibited higher counts of Bacteroides and Lactobacillus. Chen et al. found significantly higher relative abundances of Lactobacillus, Veillonella, and Streptococcus in GD patients (Jiang et al., 2021). Due to limited sample sizes in the aforementioned studies, related MR research is gradually emerging. A two-sample MR analysis investigating the causal relationship between gut microbiota and GD identified Deltaproteobacteria and Mollicutes classes, as well as Ruminococcus torques group, Oxalobacter, and Ruminococcaceae ucg011 as risk factors for GD (Cao et al., 2023). Streptococcaceae and Lachnospiraceae were protective factors (Cao et al., 2023). It is worth noting that the gut microbiota GWAS dataset used in this study was based on European populations, while the GD GWAS database was based on Asian populations. In contrast to the above findings, our study conducted a two-sample MR analysis using GWAS data from European populations for both exposure and outcome, effectively mitigating the impact of population stratification on conclusions, and confirming that Bacteroidaceae, Bacteroides, and Veillonella were protective factors against GD development.
In current research on factors influencing Graves’ disease through the gut microbiota, increasing attention is being paid to the immunomodulatory effects of short-chain fatty acids (SCFAs). SCFAs can serve as an energy source for epithelial cells, maintain intestinal barrier integrity, and reduce gut permeability and circulating lipopolysaccharide levels (Koh et al., 2016). Su et al. (2020) and a prospective study (Deng et al., 2023) both found reduced levels of propionate and butyrate in GD patients, thereby corroborating the notion that decreased SCFAs production in GD patients promotes disease occurrence and progression.
SCFAs are typically produced by certain subtypes of Firmicutes and Bacteroidetes phyla (Kim, 2021). Members of the Bacteroidetes (Gram-negative), Firmicutes (Gram-positive), and Actinobacteria (Gram-positive) phyla have the highest abundance in the gut. Bacteroidetes primarily produce acetate and propionate, while Firmicutes generate butyrate as a major end-product of metabolism (Fenneman et al., 2020). The genera mentioned in this study, Bacteroides and Veillonella, belong to the Bacteroidetes and Firmicutes phyla, respectively, and both are capable of producing SCFAs. Therefore, the significant decline in the abundance of these three bacteria may lead to reduced SCFAs production, subsequently resulting in impaired intestinal barrier function and dysbiosis (Lapidot et al., 2021).
The mechanism by which SCFAs impact the immune system primarily involves the modulation of innate and adaptive lymphocytes (Kim, 2021). On one hand, SCFAs increase the activity of type 3 innate lymphoid cells (ILC3s) while suppressing the activity of type 2 innate lymphoid cells (ILC2s). Ffar3 signaling in ILC2s and ILC3s triggers PI3K, AKT, and mTOR activity, promoting cell proliferation and activation. On the other hand, under normal conditions, there’s a balance between Th17 and T regulatory cells (Tregs). Excessive Th17 increase and reduced Tregs disrupt the Th17/Tregs balance, leading to GD development. SCFAs are involved in the pathogenesis and progression of GD through the immunomodulation of Th17/Tregs and cytokines (Kohling et al., 2017). Dietary fiber metabolite SCFAs, such as butyrate can directly promote the proliferation of CD4 regulatory T cells (Tregs) under normal circumstances (Arpaia et al., 2013). Tregs regulate intestinal homeostasis and control inflammation by expressing the transcription factor Foxp3 (Smith et al., 2013). For instance, SCFAs from fecal bacteria with chloroform-resistant phenotypes were identified as major bacterial metabolites responsible for inducing Tregs and suppressing inflammation in a colitis mouse model (Atarashi et al., 2013). Furthermore, SCFAs can indirectly regulate Treg proliferation and function through the modulation of antigen-presenting cells (APCs), such as dendritic cells (DCs) and macrophages (Mendoza-Leon et al., 2023). Butyrate can signal through the SCFA receptor GPR109a in macrophages, promoting the expansion of Tregs by DCs (Singh et al., 2014). In addition, SCFAs, especially butyrate, can have a significant effect on B cell function. For instance, intestinal macrophages treated with butyrate can induce the generation of regulatory B cells producing IL-10 (Foh et al., 2022). Overall, SCFAs support the effector functions of lymphocytes to defend against microbial pathogens and cancer, playing a significant role in GD development.
This study indicates that Bacteroidaceae, Bacteroides, and Veillonella are associated with a reduced risk of GD development, serving as protective factors against GD. The genus Bacteroides consists of more than 20 anaerobic, non-spore-forming, Gram-negative rods, and belongs to the Bacteroideaceae family. The impact of Bacteroides on autoimmune diseases is gradually being investigated. Mazmanian et al. (2005) demonstrated that Bacteroides fragilis-derived polysaccharides (PSA) can activate CD4+ T cells, with PSA-stimulated CD4+ T cells producing interleukin-10 (IL-10), which acts to prevent abscess formation and other inflammatory responses. Furthermore, PSA Production by B. fragilis corrects TH1/TH2 imbalance, directs Lymphoid Organogenesis, and promotes development and maturation of the immune system. In previous studies, an increase in the abundance of Bacteroides in GD patients was reported, whereas this study indicates the opposite trend. This discrepancy could be attributed to regional or population differences, an inverse causal relationship and insufficient sample size, warranting further research for validation. The family Bacteroidaceae comprises Acetofilamentum, Acetothermus, Bacteroides, Capsularis, and Phocaeicola. In this study, within the family Bacteroidaceae, significant findings were observed exclusively within the genus Bacteroides, leading us to posit that the impact of Bacteroidaceae on GD appears to be attributed to the genus Bacteroides. Nevertheless, given the limited existing literature on the relationship between Bacteroidaceae and GD, further in-depth research is warranted. Veillonella, belonging to the phylum Firmicutes, are anaerobic Gram-negative cocci that typically occur in pairs or short chains. They lack flagella, spores, or capsules (Delwiche et al., 1985) and are commonly associated with inflammatory conditions such as periodontitis, bacteremia, and pneumonia (Marriott et al., 2007; Shah et al., 2008). Members of the genus Veillonella utilize short-chain organic acids, particularly lactate, as an energy source rather than carbohydrates or amino acids, subsequently producing acetate and propionate (Mashima et al., 2021). It is commonly found in the oral cavity, gastrointestinal tract, and vagina. There is still debate regarding this bacterium. Deng et al. suggested an increased abundance of Veillonella in GD patients (Deng et al., 2023), while this study aligns with previous reports indicating lower levels of Veillonella in GD patients(Table 3).
Furthermore, it is noteworthy that despite not maintaining statistical significance after adjusting P-values, there exists a protective trend towards decreased GD occurrence for Eubacterium brachy group, Family XIII AD3011 group, Butyricimonas, Parasutterella, and Turicibacter. Conversely, a hazardous trend towards increased GD occurrence is observed for Blautia, Catenibacterium, Ruminococcaceae NK4A214 group, Sutterella, Bilophila, Collinsella, Rhodospirillaceae, and Streptococcaceae. However, further validation is warranted.
Presently, there are three main approaches for GD treatment: radioactive iodine (RAI) therapy, anti-thyroid drugs (ATDs), and surgical intervention. However, each method is accompanied by its respective adverse effects (Smith and Hegedus, 2016). Therefore, elucidating the pathogenesis of GD may facilitate the identification of novel therapeutic targets. Probiotics, as living organisms, modulate the gut microbiome in various ways to enhance gut health (Guo et al., 2021). Ingesting probiotics alters the composition of the gut microbiome, aiding in the prevention of the progression of autoimmune diseases.
Zhang et al. found that Lactobacillus alleviates inflammatory episodes in lupus-prone female mice (Zhang et al., 2014). Clusters IV and XIVa of the genus Clostridium improve IBD in a colitis model through inducing Treg cells and increasing Foxp3 transcription factor expression (Atarashi et al., 2011). In animal models, adjusting the gut microbiome through probiotic supplementation appears to ameliorate SLE symptoms and associated cardiovascular and renal complications (Guo et al., 2021). Despite the numerous favorable outcomes seen when various probiotic strains were used to counteract various autoimmune diseases in animal models, human clinical data remain limited. This limitation might, at least in part, result from poorly designed study protocols that fail to account for the interplay between diseases and dysbiosis (Kim et al., 2016). Therefore, clinical trials employing probiotics should meticulously consider alterations in microbial composition and their impacts on autoimmune diseases. This study aims to offer novel insights into future GD treatment.
Our study possesses several strengths. Firstly, we utilized the MR analysis method to assess the associations between various gut microbiota abundances and GD risk, mitigating potential confounding factors. The ample sample size affords us sufficient power to estimate the causal effect of gut microbiota on GD. Secondly, we conducted an investigation into 150 distinct families and genera of gut microbiota. Given the substantial variations among different phyla, classes, and orders of microbiota, we exclusively utilized data pertaining to families and genera for more precise conclusions.
However, this study is not without limitations. Firstly, some batches of SNP data still exhibited pleiotropy after MR-PRESSO correction, potentially undermining the robustness of MR conclusions. Moreover, our study population was predominantly of European descent, thereby limiting the generalizability of findings to broader populations. Further research is warranted to explore the impact of gut microbiota abundance on GD occurrence across different ethnic groups. As we employed summary data rather than individual-level data, stratified analysis by variables such as gender was unattainable. The statistical power of correlation-based conclusions for some batches in this study was relatively low, potentially elevating the likelihood of type II errors. The assumption of linearity in the causal relationship, inherent in the method of ratio estimation, prevents this study from excluding potential non-linear associations between gut microbiota and GD susceptibility. Lastly, although bacteria are the primary constituents of the intestinal microbiome, viruses, fungi, and archaea also inhabit the gut. Their interactions with the gut microbiota and GD remain largely unknown, necessitating further research. Nevertheless, it is crucial to note that as long as the SNPs utilized in this study satisfy the three assumptions of instrumental variables, the resultant MR conclusions remain valid.
5 Conclusion
There is a causal relationship between gut microbiota abundance and GD. Bacteroidaceae, Bacteroides, and Veillonella serve as protective factors against GD occurrence. Thirteen bacterial strains, including Eubacterium brachy group, potentially exert causal influences on GD occurrence. Probiotics may offer a novel avenue for adjunctive therapy in future GD treatment.
Data availability statement
Publicly available datasets were analyzed in this study. This data can be found here: https://mibiogen.gcc.rug.nl/. https://www.finngen.fi/.
Author contributions
SL: Writing – original draft. FL: Writing – original draft. YC: Investigation, Writing – review & editing. LR: Writing – review & editing. LS: Supervision, Writing – review & editing. XG: Supervision, Writing – review & editing. GW: Funding acquisition, Supervision, Writing – review & editing.
Funding
The author(s) declare financial support was received for the research, authorship, and/or publication of this article. This work was supported by grants from the Department of Science and Technology of Jilin Province (20190901006JC to GW).
Acknowledgments
We want to acknowledge the participants and investigators of the FinnGen study and MlBioGen study.
Conflict of interest
The authors declare that the research was conducted in the absence of any commercial or financial relationships that could be construed as a potential conflict of interest.
Publisher’s note
All claims expressed in this article are solely those of the authors and do not necessarily represent those of their affiliated organizations, or those of the publisher, the editors and the reviewers. Any product that may be evaluated in this article, or claim that may be made by its manufacturer, is not guaranteed or endorsed by the publisher.
Supplementary material
The Supplementary Material for this article can be found online at: https://www.frontiersin.org/articles/10.3389/fcimb.2024.1288222/full#supplementary-material
References
Adak, A., Khan, M. R. (2019). An insight into gut microbiota and its functionalities. Cell Mol. Life Sci. 76 (3), 473–493. doi: 10.1007/s00018-018-2943-4
Ananthakrishnan, A. N., Khalili, H., Konijeti, G. G., Higuchi, L. M., de Silva, P., Korzenik, J. R., et al. (2013). A prospective study of long-term intake of dietary fiber and risk of Crohn’s disease and ulcerative colitis. Gastroenterology 145 (5), 970–977. doi: 10.1053/j.gastro.2013.07.050
Andrade-Oliveira, V., Amano, M. T., Correa-Costa, M., Castoldi, A., Felizardo, R. J., de Almeida, D. C., et al. (2015). Gut bacteria products prevent AKI induced by ischemia-reperfusion. J. Am. Soc. Nephrol. 26 (8), 1877–1888. doi: 10.1681/ASN.2014030288
Antonelli, A., Ferrari, S. M., Ragusa, F., Elia, G., Paparo, S. R., Ruffilli, I., et al. (2020). Graves’ disease: Epidemiology, genetic and environmental risk factors and viruses. Best Pract. Res. Clin. Endocrinol. Metab. 34 (1), 101387. doi: 10.1016/j.beem.2020.101387
Arpaia, N., Campbell, C., Fan, X., Dikiy, S., van der Veeken, J., deRoos, P., et al. (2013). Metabolites produced by commensal bacteria promote peripheral regulatory T-cell generation. Nature 504 (7480), 451–455. doi: 10.1038/nature12726
Atarashi, K., Tanoue, T., Oshima, K., Suda, W., Nagano, Y., Nishikawa, H., et al. (2013). Treg induction by a rationally selected mixture of Clostridia strains from the human microbiota. Nature 500 (7461), 232–236. doi: 10.1038/nature12331
Atarashi, K., Tanoue, T., Shima, T., Imaoka, A., Kuwahara, T., Momose, Y., et al. (2011). Induction of colonic regulatory T cells by indigenous Clostridium species. Science 331 (6015), 337–341. doi: 10.1126/science.1198469
Biscarini, F., Masetti, G., Muller, I., Verhasselt, H. L., Covelli, D., Colucci, G., et al. (2023). Gut microbiome associated with Graves disease and Graves orbitopathy: the INDIGO multicenter European study. J. Clin. Endocrinol. Metab. 108 (8), 2065–2077. doi: 10.1210/clinem/dgad030
Bowden, J., Davey Smith, G., Haycock, P. C., Burgess, S. (2016a). Consistent estimation in Mendelian randomization with some invalid instruments using a weighted median estimator. Genet. Epidemiol. 40 (4), 304–314. doi: 10.1002/gepi.21965
Bowden, J., Del Greco, M. F., Minelli, C., Davey Smith, G., Sheehan, N. A., Thompson, J. R. (2016b). Assessing the suitability of summary data for two-sample Mendelian randomization analyses using MR-Egger regression: the role of the I2 statistic. Int. J. Epidemiol. 45 (6), 1961–1974. doi: 10.1093/ije/dyw220
Burgess, S. (2014). Sample size and power calculations in Mendelian randomization with a single instrumental variable and a binary outcome. Int. J. Epidemiol. 43 (3), 922–929. doi: 10.1093/ije/dyu005
Burgess, S., Butterworth, A., Thompson, S. G. (2013). Mendelian randomization analysis with multiple genetic variants using summarized data. Genet. Epidemiol. 37 (7), 658–665. doi: 10.1002/gepi.21758
Burgess, S., Davey Smith, G., Davies, N. M., Dudbridge, F., Gill, D., Glymour, M. M., et al. (2019). Guidelines for performing Mendelian randomization investigations: update for summer 2023. Wellcome Open Res. 4, 186. doi: 10.12688/wellcomeopenres.15555.1
Burgess, S., Small, D. S., Thompson, S. G. (2017). A review of instrumental variable estimators for Mendelian randomization. Stat. Methods Med. Res. 26 (5), 2333–2355. doi: 10.1177/0962280215597579
Burgess, S., Thompson, S. G. (2017). Interpreting findings from Mendelian randomization using the MR-Egger method. Eur. J. Epidemiol. 32 (5), 377–389. doi: 10.1007/s10654-017-0255-x
Burgi, H. (2010). Iodine excess. Best Pract. Res. Clin. Endocrinol. Metab. 24 (1), 107–115. doi: 10.1016/j.beem.2009.08.010
Cao, J., Wang, N., Luo, Y., Ma, C., Chen, Z., Chenzhao, C., et al. (2023). A cause-effect relationship between Graves’ disease and the gut microbiome contributes to the thyroid-gut axis: A bidirectional two-sample Mendelian randomization study. Front. Immunol. 14, 977587. doi: 10.3389/fimmu.2023.977587
Cao, S. S. (2018). Cellular stress responses and gut microbiota in inflammatory bowel disease. Gastroenterol. Res. Pract. 2018, 7192646. doi: 10.1155/2018/7192646
Cayres, L. C. F., de Salis, L. V. V., Rodrigues, G. S. P., Lengert, A. V. H., Biondi, A. P. C., Sargentini, L. D. B., et al. (2021). Detection of alterations in the gut microbiota and intestinal permeability in patients with Hashimoto thyroiditis. Front. Immunol. 12, 579140. doi: 10.3389/fimmu.2021.579140
Chang, S. C., Lin, S. F., Chen, S. T., Chang, P. Y., Yeh, Y. M., Lo, F. S., et al. (2021). Alterations of gut microbiota in patients with Graves’ disease. Front. Cell Infect. Microbiol. 11, 663131. doi: 10.3389/fcimb.2021.663131
De Luca, F., Shoenfeld, Y. (2019). The microbiome in autoimmune diseases. Clin. Exp. Immunol. 195 (1), 74–85. doi: 10.1111/cei.13158
Delwiche, E. A., Pestka, J. J., Tortorello, M. L. (1985). The veillonellae: gram-negative cocci with a unique physiology. Annu. Rev. Microbiol. 39, 175–193. doi: 10.1146/annurev.mi.39.100185.001135
den Besten, G., van Eunen, K., Groen, A. K., Venema, K., Reijngoud, D. J., Bakker, B. M. (2013). The role of short-chain fatty acids in the interplay between diet, gut microbiota, and host energy metabolism. J. Lipid Res. 54 (9), 2325–2340. doi: 10.1194/jlr.R036012
Deng, Y., Wang, J., Xie, G., Zou, G., Li, S., Zhang, J., et al. (2023). Correlation between gut microbiota and the development of Graves’ disease: A prospective study. iScience 26 (7), 107188. doi: 10.1016/j.isci.2023.107188
El-Zawawy, H. T., Ahmed, S. M., El-Attar, E. A., Ahmed, A. A., Roshdy, Y. S., Header, D. A. (2021). Study of gut microbiome in Egyptian patients with autoimmune thyroid diseases. Int. J. Clin. Pract. 75 (5), e14038. doi: 10.1111/ijcp.14038
Emdin, C. A., Khera, A. V., Kathiresan, S. (2017). Mendelian randomization. JAMA 318 (19), 1925–1926. doi: 10.1001/jama.2017.17219
Fatourechi, V. (2012). Thyroid dermopathy and acropachy. Best Pract. Res. Clin. Endocrinol. Metab. 26 (4), 553–565. doi: 10.1016/j.beem.2011.10.001
Fenneman, A. C., Rampanelli, E., Yin, Y. S., Ames, J., Blaser, M. J., Fliers, E., et al. (2020). Gut microbiota and metabolites in the pathogenesis of endocrine disease. Biochem. Soc. Trans. 48 (3), 915–931. doi: 10.1042/BST20190686
Foh, B., Buhre, J. S., Lunding, H. B., Moreno-Fernandez, M. E., Konig, P., Sina, C., et al. (2022). Microbial metabolite butyrate promotes induction of IL-10+IgM+ plasma cells. PloS One 17 (3), e0266071. doi: 10.1371/journal.pone.0266071
Gill, S. R., Pop, M., Deboy, R. T., Eckburg, P. B., Turnbaugh, P. J., Samuel, B. S., et al. (2006). Metagenomic analysis of the human distal gut microbiome. Science 312 (5778), 1355–1359. doi: 10.1126/science.1124234
Gong, B., Wang, C., Meng, F., Wang, H., Song, B., Yang, Y., et al. (2021). Association between gut microbiota and autoimmune thyroid disease: A systematic review and meta-analysis. Front. Endocrinol. (Lausanne) 12, 774362. doi: 10.37766/inplasy2021.4.0135
Guo, X., Yang, X., Li, Q., Shen, X., Zhong, H., Yang, Y. (2021). The microbiota in systemic lupus erythematosus: an update on the potential function of probiotics. Front. Pharmacol. 12, 759095. doi: 10.3389/fphar.2021.759095
Haghikia, A., Jorg, S., Duscha, A., Berg, J., Manzel, A., Waschbisch, A., et al. (2016). Dietary fatty acids directly impact central nervous system autoimmunity via the small intestine. Immunity 44 (4), 951–953. doi: 10.1016/j.immuni.2016.04.006
Hemani, G., Tilling, K., Davey Smith, G. (2017). Orienting the causal relationship between imprecisely measured traits using GWAS summary data. PloS Genet. 13 (11), e1007081. doi: 10.1371/journal.pgen.1007081
Ishaq, H. M., Mohammad, I. S., Shahzad, M., Ma, C., Raza, M. A., Wu, X., et al. (2018). Molecular alteration analysis of human gut microbial composition in Graves’ disease patients. Int. J. Biol. Sci. 14 (11), 1558–1570. doi: 10.7150/ijbs.24151
Jiang, T., Gill, D., Butterworth, A. S., Burgess, S. (2023). An empirical investigation into the impact of winner’s curse on estimates from Mendelian randomization. Int. J. Epidemiol. 52 (4), 1209–1219. doi: 10.1093/ije/dyac233
Jiang, W., Yu, X., Kosik, R. O., Song, Y., Qiao, T., Tong, J., et al. (2021). Gut microbiota may play a significant role in the pathogenesis of Graves’ disease. Thyroid 31 (5), 810–820. doi: 10.1089/thy.2020.0193
Jie, Z., Xia, H., Zhong, S. L., Feng, Q., Li, S., Liang, S., et al. (2017). The gut microbiome in atherosclerotic cardiovascular disease. Nat. Commun. 8 (1), 845. doi: 10.1038/s41467-017-00900-1
Kho, Z. Y., Lal, S. K. (2018). The human gut microbiome - A potential controller of wellness and disease. Front. Microbiol. 9, 1835. doi: 10.3389/fmicb.2018.01835
Kim, C. H. (2021). Control of lymphocyte functions by gut microbiota-derived short-chain fatty acids. Cell Mol. Immunol. 18 (5), 1161–1171. doi: 10.1038/s41423-020-00625-0
Kim, D., Yoo, S. A., Kim, W. U. (2016). Gut microbiota in autoimmunity: potential for clinical applications. Arch. Pharm. Res. 39 (11), 1565–1576. doi: 10.1007/s12272-016-0796-7
Koh, A., De Vadder, F., Kovatcheva-Datchary, P., Backhed, F. (2016). From dietary fiber to host physiology: short-chain fatty acids as key bacterial metabolites. Cell 165 (6), 1332–1345. doi: 10.1016/j.cell.2016.05.041
Kohling, H. L., Plummer, S. F., Marchesi, J. R., Davidge, K. S., Ludgate, M. (2017). The microbiota and autoimmunity: Their role in thyroid autoimmune diseases. Clin. Immunol. 183, 63–74. doi: 10.1016/j.clim.2017.07.001
Kurilshikov, A., Medina-Gomez, C., Bacigalupe, R., Radjabzadeh, D., Wang, J., Demirkan, A., et al. (2021). Large-scale association analyses identify host factors influencing human gut microbiome composition. Nat. Genet. 53 (2), 156–165. doi: 10.1038/s41588-020-00763-1
Kurki, M. I., Karjalainen, J., Palta, P., Sipila, T. P., Kristiansson, K., Donner, K. M., et al. (2023). FinnGen provides genetic insights from a well-phenotyped isolated population. Nature 613 (7944), 508–518. doi: 10.1038/s41586-022-05473-8
Lapidot, Y., Amir, A., Ben-Simon, S., Veitsman, E., Cohen-Ezra, O., Davidov, Y., et al. (2021). Alterations of the salivary and fecal microbiome in patients with primary sclerosing cholangitis. Hepatol. Int. 15 (1), 191–201. doi: 10.1007/s12072-020-10089-z
Lee, H. J., Li, C. W., Hammerstad, S. S., Stefan, M., Tomer, Y. (2015). Immunogenetics of autoimmune thyroid diseases: A comprehensive review. J. Autoimmun. 64, 82–90. doi: 10.1016/j.jaut.2015.07.009
Lerner, A., Jeremias, P., Matthias, T. (2017). Gut-thyroid axis and celiac disease. Endocr. Connect 6 (4), R52–R58. doi: 10.1530/EC-17-0021
Li, F., Liu, Y., Wang, Z., Zhao, Q., Li, Y., Tang, T. (2022). A mendelian randomization study with populations of European ancestry rules out a causal relationship between inflammatory bowel disease and colorectal cancer. Front. Genet. 13, 949325. doi: 10.3389/fgene.2022.949325
Licciardi, P. V., Ververis, K., Karagiannis, T. C. (2011). Histone deacetylase inhibition and dietary short-chain Fatty acids. ISRN Allergy 2011, 869647. doi: 10.5402/2011/869647
Magne, F., Gotteland, M., Gauthier, L., Zazueta, A., Pesoa, S., Navarrete, P., et al. (2020). The firmicutes/bacteroidetes ratio: A relevant marker of gut dysbiosis in obese patients? Nutrients 12 (5). doi: 10.3390/nu12051474
Marriott, D., Stark, D., Harkness, J. (2007). Veillonella parvula discitis and secondary bacteremia: a rare infection complicating endoscopy and colonoscopy? J. Clin. Microbiol. 45 (2), 672–674. doi: 10.1128/JCM.01633-06
Masetti, G., Moshkelgosha, S., Kohling, H. L., Covelli, D., Banga, J. P., Berchner-Pfannschmidt, U., et al. (2018). Gut microbiota in experimental murine model of Graves’ orbitopathy established in different environments may modulate clinical presentation of disease. Microbiome 6 (1), 97. doi: 10.1186/s40168-018-0478-4
Mashima, I., Theodorea, C. F., Djais, A. A., Kunihiro, T., Kawamura, Y., Otomo, M., et al. (2021). Veillonella nakazawae sp. nov., an anaerobic Gram-negative coccus isolated from the oral cavity of Japanese children. Int. J. Syst. Evol. Microbiol. 71 (1). doi: 10.1099/ijsem.0.004583
Mazmanian, S. K., Liu, C. H., Tzianabos, A. O., Kasper, D. L. (2005). An immunomodulatory molecule of symbiotic bacteria directs maturation of the host immune system. Cell 122 (1), 107–118. doi: 10.1016/j.cell.2005.05.007
Mendoza-Leon, M. J., Mangalam, A. K., Regaldiz, A., Gonzalez-Madrid, E., Rangel-Ramirez, M. A., Alvarez-Mardonez, O., et al. (2023). Gut microbiota short-chain fatty acids and their impact on the host thyroid function and diseases. Front. Endocrinol. (Lausanne) 14, 1192216. doi: 10.3389/fendo.2023.1192216
Moshkelgosha, S., Verhasselt, H. L., Masetti, G., Covelli, D., Biscarini, F., Horstmann, M., et al. (2021). Modulating gut microbiota in a mouse model of Graves’ orbitopathy and its impact on induced disease. Microbiome 9 (1), 45. doi: 10.1186/s40168-020-00952-4
Nishino, K., Nishida, A., Inoue, R., Kawada, Y., Ohno, M., Sakai, S., et al. (2018). Analysis of endoscopic brush samples identified mucosa-associated dysbiosis in inflammatory bowel disease. J. Gastroenterol. 53 (1), 95–106. doi: 10.1007/s00535-017-1384-4
Pan, X., Fang, X., Wang, F., Li, H., Niu, W., Liang, W., et al. (2019). Butyrate ameliorates caerulein-induced acute pancreatitis and associated intestinal injury by tissue-specific mechanisms. Br. J. Pharmacol. 176 (23), 4446–4461. doi: 10.1111/bph.14806
Rayman, M. P. (2019). Multiple nutritional factors and thyroid disease, with particular reference to autoimmune thyroid disease. Proc. Nutr. Soc. 78 (1), 34–44. doi: 10.1017/S0029665118001192
Scheppach, W., Sommer, H., Kirchner, T., Paganelli, G. M., Bartram, P., Christl, S., et al. (1992). Effect of butyrate enemas on the colonic mucosa in distal ulcerative colitis. Gastroenterology 103 (1), 51–56. doi: 10.1016/0016-5085(92)91094-K
Schwiertz, A., Taras, D., Schafer, K., Beijer, S., Bos, N. A., Donus, C., et al. (2010). Microbiota and SCFA in lean and overweight healthy subjects. Obes. (Silver Spring) 18 (1), 190–195. doi: 10.1038/oby.2009.167
Shah, A., Panjabi, C., Nair, V., Chaudhry, R., Thukral, S. S. (2008). Veillonella as a cause of chronic anaerobic pneumonitis. Int. J. Infect. Dis. 12 (6), e115–e117. doi: 10.1016/j.ijid.2008.03.018
Singh, N., Gurav, A., Sivaprakasam, S., Brady, E., Padia, R., Shi, H., et al. (2014). Activation of Gpr109a, receptor for niacin and the commensal metabolite butyrate, suppresses colonic inflammation and carcinogenesis. Immunity 40 (1), 128–139. doi: 10.1016/j.immuni.2013.12.007
Sircana, A., De Michieli, F., Parente, R., Framarin, L., Leone, N., Berrutti, M., et al. (2019). Gut microbiota, hypertension and chronic kidney disease: Recent advances. Pharmacol. Res. 144, 390–408. doi: 10.1016/j.phrs.2018.01.013
Smith, P. M., Howitt, M. R., Panikov, N., Michaud, M., Gallini, C. A., Bohlooly, Y. M., et al. (2013). The microbial metabolites, short-chain fatty acids, regulate colonic Treg cell homeostasis. Science 341 (6145), 569–573. doi: 10.1126/science.1241165
Smith, T. J., Hegedus, L. (2016). Graves’ disease. N Engl. J. Med. 375 (16), 1552–1565. doi: 10.1056/NEJMra1510030
Staley, J. R., Blackshaw, J., Kamat, M. A., Ellis, S., Surendran, P., Sun, B. B., et al. (2016). PhenoScanner: a database of human genotype-phenotype associations. Bioinformatics 32 (20), 3207–3209. doi: 10.1093/bioinformatics/btw373
Stojanov, S., Berlec, A., Strukelj, B. (2020). The influence of probiotics on the firmicutes/bacteroidetes ratio in the treatment of obesity and inflammatory bowel disease. Microorganisms 8 (11). doi: 10.3390/microorganisms8111715
Su, X., Yin, X., Liu, Y., Yan, X., Zhang, S., Wang, X., et al. (2020). Gut dysbiosis contributes to the imbalance of Treg and Th17 cells in Graves’ disease patients by propionic acid. J. Clin. Endocrinol. Metab. 105 (11). doi: 10.1210/clinem/dgaa511
Tellez, M., Cooper, J., Edmonds, C. (1992). Graves’ ophthalmopathy in relation to cigarette smoking and ethnic origin. Clin. Endocrinol. (Oxf.) 36 (3), 291–294. doi: 10.1111/j.1365-2265.1992.tb01445.x
Thomas, D. C., Conti, D. V. (2004). Commentary: the concept of ‘Mendelian randomization’. Int. J. Epidemiol. 33 (1), 21–25. doi: 10.1093/ije/dyh048
Tomasello, G., Tralongo, P., Amoroso, F., Damiani, P., Sinagra, E., Noto, M., et al. (2015). Dysmicrobism, inflammatory bowel disease and thyroiditis: analysis of the literature. J. Biol. Regul. Homeost. Agents 29 (2), 265–272.
Wiersinga, W. M. (2013). Smoking and thyroid. Clin. Endocrinol. (Oxf.) 79 (2), 145–151. doi: 10.1111/cen.12222
Yang, M., Sun, B., Li, J., Yang, B., Xu, J., Zhou, X., et al. (2019). Alteration of the intestinal flora may participate in the development of Graves’ disease: a study conducted among the Han population in southwest China. Endocr. Connect 8 (7), 822–828. doi: 10.1530/EC-19-0001
Zhang, H., Liao, X., Sparks, J. B., Luo, X. M. (2014). Dynamics of gut microbiota in autoimmune lupus. Appl. Environ. Microbiol. 80 (24), 7551–7560. doi: 10.1128/AEM.02676-14
Keywords: Graves’ disease, gut microbiota, autoimmune disease, Mendelian randomization, causal effect
Citation: Liu S, Li F, Cai Y, Ren L, Sun L, Gang X and Wang G (2024) Bacteroidaceae, Bacteroides, and Veillonella: emerging protectors against Graves’ disease. Front. Cell. Infect. Microbiol. 14:1288222. doi: 10.3389/fcimb.2024.1288222
Received: 07 September 2023; Accepted: 26 January 2024;
Published: 09 February 2024.
Edited by:
Valeriy Poroyko, Laboratory Corporation of America Holdings (LabCorp), United StatesReviewed by:
Filippo Biscarini, National Research Council (CNR), ItalyGeorge Grant, University of Aberdeen, United Kingdom
Copyright © 2024 Liu, Li, Cai, Ren, Sun, Gang and Wang. This is an open-access article distributed under the terms of the Creative Commons Attribution License (CC BY). The use, distribution or reproduction in other forums is permitted, provided the original author(s) and the copyright owner(s) are credited and that the original publication in this journal is cited, in accordance with accepted academic practice. No use, distribution or reproduction is permitted which does not comply with these terms.
*Correspondence: Guixia Wang, Z3dhbmcxNjhAamx1LmVkdS5jbg==
†This authors have contributed equally to this work, and share the first authorship