- 1Department of Medical Oncology, The First Hospital of China Medical University, Shenyang, China
- 2Department of Urology, The First Affiliated Hospital of China Medical University, Shenyang, China
- 3Phase I Clinical Trails Center, The First Hospital of China Medical University, Shenyang, China
Background: The gut microbiota has been found to be associated with the risk of lung cancer. However, its causal relationship with various types of lung cancer remains unclear.
Methods: We conducted a Mendelian randomization (MR) study using the largest genome-wide association analysis of gut microbiota data to date from the MiBioGen consortium, with pooled statistics for various types of lung cancer from the Transdisciplinary Research in Cancer of the Lung, the International Lung Cancer Consortium, and FinnGen Consortium R7 release data. Inverse variance weighted, weighted model, MR-Egger regression, and weighted median were adapted to assess the causal relationship between gut microbiota and various types of lung cancer. Sensitivity analysis was used to test for the presence of pleiotropy and heterogeneity in instrumental variables. A reverse MR analysis was performed on these bacteria to determine their potential role in causing lung cancer. A reverse MR analysis was performed on these bacteria to determine their potential role in causing lung cancer. Multivariable Mendelian randomization (MVMR) was conducted to assess the direct causal impact of gut microbiota on the risk of various types of lung cancer.
Results: Using IVW as the primary analytical method, we identified a total of 40 groups of gut microbiota with potential causal associations with various subtypes of lung cancer, of which 10 were associated with lung cancer, 10 with lung adenocarcinoma, 9 with squamous cell lung cancer, and 11 groups of bacteria associated with small cell lung cancer. After performing FDR correction, we further found that there was still a significant causal relationship between Peptococcaceae and lung adenocarcinoma. Sensitivity analyses demonstrated the robustness of these results, with no heterogeneity or pleiotropy found.
Conclusions: Our results confirm a causal relationship between specific gut microbiota and lung cancer, providing new insights into the role of gut microbiota in mediating the development of lung cancer.
1 Introduction
According to statistics, lung cancer accounts for 2.2 million new cases and 1.79 million fatalities each year (Thai et al., 2021), making it the second most commonly occurring cancer type globally and the primary cause of cancer-related deaths (Sung et al., 2021; Huang et al., 2022). Several well-known risk factors contribute to the development of lung cancer, including smoking, previous lung diseases, air pollutants, and occupational carcinogens (Samet et al., 2009; Wang et al., 2020). Identifying and discovering potentially modifiable risk factors is crucial to reducing the incidence of lung cancer, which is conducive to early diagnosis and treatment of lung cancer.
Recently, it has been suggested that the gut microbiota (GM) has an impact on the progression of lung cancer. GM refers to the highly complex community of microorganisms that reside within the gastrointestinal tract, including archaea, bacteria, eukaryotes, parasites, and viruses (Ge et al., 2021). Dysbiosis of microbial ecology may lead to changes in metabolism, immunosuppression, and the recruitment of inflammatory factors that can drive lung carcinogenesis. In the study by JinC and LagoudasGK et al., mouse models showed that the local microbiota associated with tumor development promotes inflammatory progression through lung-resident γδ T cells (Jin et al., 2019) and is thought to be associated with lung cancer. The most recent theory holds that even though the gastrointestinal tract and respiratory tract are physically separate, they share an embryonic origin and a high degree of structural similarity (Georgiou et al., 2021). There is a distinct crosstalk between the respiratory tract and the gastrointestinal tract known as the gut–lung axis (Dang and Marsland, 2019; Enaud et al., 2020; Zhang et al., 2020). The gut-lung axis is the pathway through which the GM interacts with the lung (Young et al., 2016; Liu et al., 2021), Microbiota-accessible carbohydrates can alter the GM and improve short-chain fatty acid (SCFA) levels, hence shaping lung immunity (Arrieta et al., 2015; Budden et al., 2017). Through T cell receptor signaling, SCFAs may activate innate lymphoid cells that produce IL-22 type 3, regulatory T cells, and Th2 cells in the lung to reduce inflammation and thus reduce the incidence of lung cancer (Corrêa et al., 2022). SCFAs can also exert effective anti-inflammatory and immunomodulatory effects by activating G protein-coupled cell surface receptors and inhibiting histone deacetylases, as well as exert immunomodulatory effects by activating G protein-coupled cell surface receptors and inhibiting histone deacetylases (Ubachs et al., 2021). Nonetheless, whether there is a clear causal relationship between lung cancer and GM still needs further proof.
In order to investigate the potential causal link between lung cancer and GM, Mendelian randomization (MR) analysis was used. MR analysis is a statistical strategy based on genome-wide association analysis, following the Mendelian rule of “parental alleles randomly assigned to offspring”. It is therefore a natural randomized controlled trial (RCT). MR analysis has been extensively utilized to investigate the connection between GM and diseases such as pre-eclampsia and eclampsia (Li et al., 2022), autoimmune diseases (Xu et al., 2021), and psychiatric disorders (Zhuang et al., 2020). In this research, we employed the two-sample MR analysis method to examine the potential impact of GM in various types of lung cancer. The findings of this research can serve as a foundation for understanding the etiology and diagnosis of lung cancer.
2 Materials and methods
2.1 Study design
A bidirectional two-sample MR analysis was conducted to investigate the causal relationship between GM and various subtypes of lung cancer. The flow chart of the study is shown in Figure 1. The aim of reverse MR analysis is to mitigate the potential effect of various subtypes of lung cancer on the GM and improve the confidence of the results.
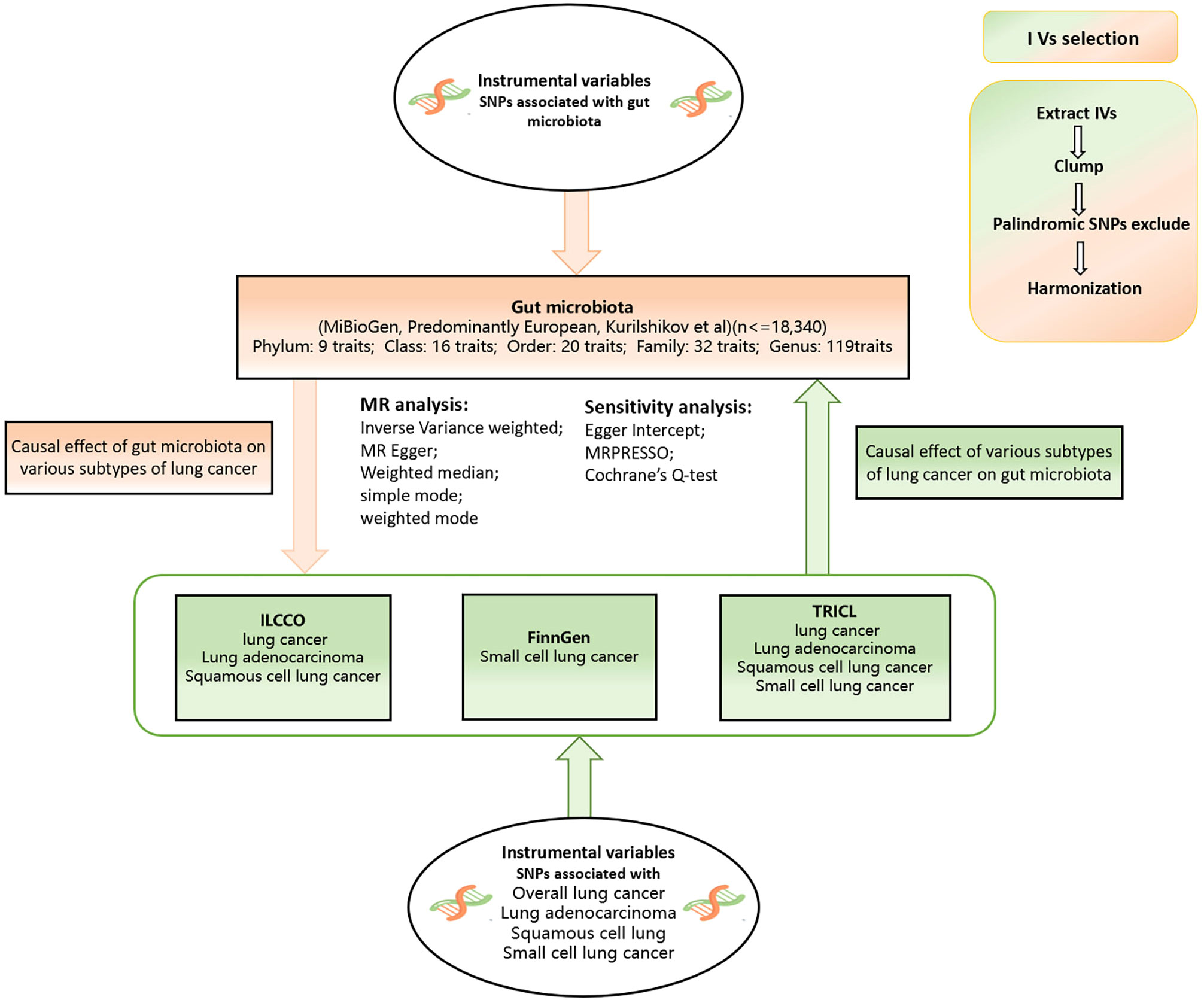
Figure 1 Flow chart of our study design. This study was a bidirectional Mendelian randomization analysis testing the causal effects between the gut microbiota and different types of lung cancer. Inverse variance weighted (IVW) was adopted as the primary method for univariable MR. To provide robust evidence of MR estimates, sensitivity analyses were used.
2.2 Data sources
The MiBioGen study provides genome-wide association summary data for the GM (Kurilshikov et al., 2023) and is the largest meta-analysis of its kind to date. The study includes 24 cohorts comprising 18,340 participants of various races and ages. To ensure data quality, the majority of cohorts employed comparable methods for interpolation and subsequent filtering. Direct taxonomic binning was used to classify the taxonomy, resulting in 211 taxa being included in the analysis, representing 131 genera, 35 families, 20 orders, 16 classes, and 9 phyla.
To bolster statistical power, validate research findings, and foster the exploration of potential new associations, we leveraged a comprehensive set of three lung cancer datasets. The Transdisciplinary Research in Cancer of the Lung (TRICL) is a member of an organization focused on Genetic Associations and Mechanisms in Oncology (GAME-ON consortium). The International Lung Cancer Consortium (ILCCO) is an international organization of lung cancer researchers that was founded in 2004 to share data from ongoing lung cancer epidemiological studies with the overall goal of maximizing statistical power. We obtained summary data from the MR-Base database for both TRICL and ILCCO. The FinnGen consortium R7 (http://r7.finngen.fi/) results provided us with summary data on small-cell lung cancer. All the data is listed in Table 1. We used all summary data from published studies and publicly available GWAS abstracts, and therefore did not require additional ethical approval or consent.
2.3 Instrumental variable selection
For the MR analysis, we examined 211 taxa of gut microbes, but excluded 15 taxa from unclassified groups, leaving 196 bacterial taxa (9 phyla, 16 classes, 20 orders, 32 families, and 119 genera) to be included in the analysis. To ensure data robustness and reliability of results, quality control of SNPs was performed to obtain compliant instrumental variables:(1) After consulting the relevant research (Lv et al., 2021; Liu et al., 2022; Ren et al., 2023; Su et al., 2023), we adjusted the threshold to the locus-wide significance level (p < 1 × 10−5); (2) As strong linkage disequilibrium may cause biased results, we performed linkage disequilibrium (LD) analysis with a threshold of r2< 0.001 and an aggregation window of 10,000kb, using a reference panel of 1000 Genome Project European samples; (3) Exclusion of palindromic sequences and SNPs with allelic inconsistencies between the two samples; and (4) To evaluate the strength of the SNP, we calculated the F-statistic. An F-statistic value of ≥10 indicates a lack of strong evidence for weak instrument bias. We excluded weak instruments with F-statistics less than <10.
2.4 Statistical analysis
2.4.1 MR analysis
To identify the connection between the GM and various types of lung cancer, we utilized four approaches in this study: inverse variance weighted (IVW), MR-Egger, weighted model, and weighted median. IVW is a method for MR to meta-summarize wald estimates for each locus when analyzing multiple SNPs. The IVW results would be objective if horizontal pleiotropy did not exist (Pierce and Burgess, 2013). The MR-Egger method employs the InSIDE assumption to perform a weighted linear regression of exposure outcomes, while the weighted median method assigns greater weight to SNPs with larger beta values during estimation. Weighted median is the median of the distribution function obtained by sorting all individual SNP effect values by weight. To obtain robust estimates in our research, we require at least 50% of the information to be derived from valid instrumental variables. We performed validation using the Benjamini-Hochberg correction. Only significant data with a corrected p-value of less than 0.05 were considered to have a strong causal relationship. However, we suggest that bacteria with a p-value of less than 0.05 but not significant after correction may still have a potential causal relationship with lung cancer. Furthermore, we conducted additional reverse MR analyses to investigate whether different types of lung cancer have a causal impact on the significant GM identified in our study. In order to investigate the influence of the GM on different subtypes of lung cancer, we conducted a multivariate Mendelian randomization analysis. This analytical approach allowed us to explore the causal relationship between the GM and lung cancer subtypes while controlling for potential confounding factors such as smoking, alcohol consumption, and chronic obstructive pulmonary disease (COPD).
2.4.2 Sensitivity analysis
We utilized MR-PRESSO to evaluate horizontal pleiotropy, while MR-Egger avoided enforcing the regression line through zero to account for the existence of directional genetic pleiotropy. In cases where the regression intercepts were non-zero and p<0.05, we regarded them as statistically significant indicators of genetic pleiotropy. Furthermore, we conducted Cochran’s Q test, and we observed heterogeneity if p<0.05. Finally, we carried out a leave-one-out sensitivity test to determine if a single instrumental variable had a significant impact on the causal effect.
The R software was used to perform all statistical analyses (Version 4.2.2). “TwoSampleMR” package (version 0.5.6) (Hemani et al., 2017) and “MRPRESSO” (version 1.0) package (Verbanck et al., 2018) were used to perform MR analysis. The code used for our analysis is available on GitHub [GitHub].
3 Results
The results of MR estimates for the relationship between the GM and different types of lung cancer are presented in Supplementary Table 1 and Figure 2. After correction, one microbial taxon was found to be significant at a significance level of 0.05.
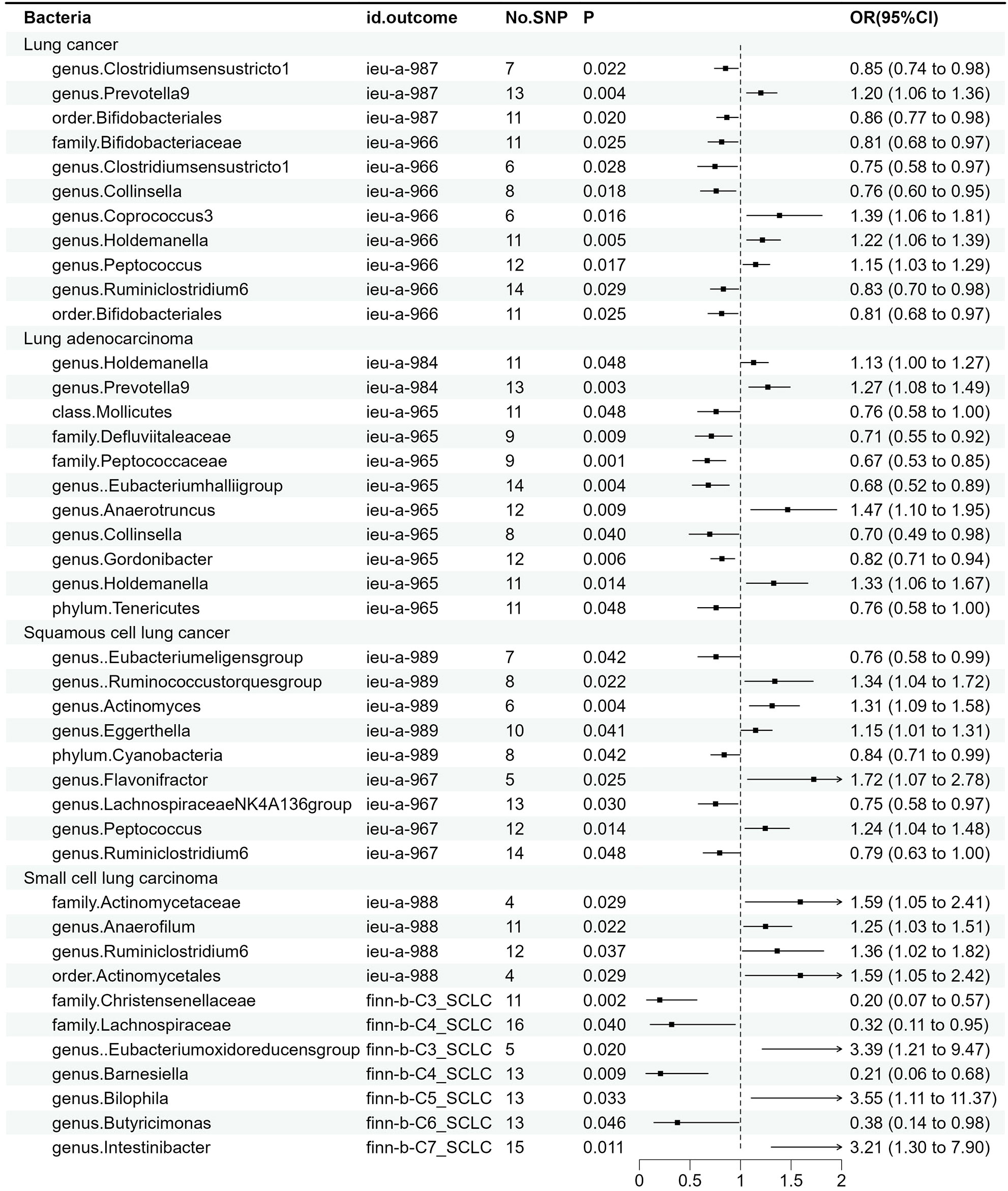
Figure 2 Associations between gut microbiota and various subtypes of lung cancer. The odds ratios are scaled per 1 standard deviation increase in gut microbiota.CI, confidence interval; OR, odds ratio.
3.1 Causal effect of GM on various subtypes of lung cancer
For overall lung cancer, as shown in Supplementary Figure 1, we identified a total of 11 causal relationships between the GM and lung cancer in the ILCCO and TRICL datasets. Among these 11 microbial communities, Prevotella9 (P = 0.004), Coprococcus3 (P = 0.016), Holdemanella (P = 0.005), and Peptococcus (P = 0.017) were considered positively correlated with the risk of lung cancer, while Bifidobacteriaceae (P = 0.025), Collinsella (P = 0.018), and Ruminiclostridium6 were (P =0.029) deemed negatively correlated with lung cancer risk. Clostridiumsensustricto1 (P1 = 0.022, P2 = 0.028) and “Bifidobacteriales” (P1 = 0.02, P2 = 0.025) both exhibited positive results in two lung cancer datasets. Notably, “family Bifidobacteriaceae” and “order Bifidobacteriales” demonstrated a consistent effect in the same lung cancer dataset (P = 0.025).
For lung adenocarcinoma, as shown in Supplementary Figure 2, we identified a total of 11 causal relationships between the GM and lung adenocarcinoma in two datasets, among which 7 demonstrated an inversely proportional relationship with the risk of lung adenocarcinoma. To be specific, these encompass Mollicutes (p = 0.048), Defluviitaleaceae (p = 0.009), Peptococcaceae (p =0.001), Eubacteriumhalliigroup (p = 0.004), Collinsella (p = 0.04), Gordonibacter (p=0.06), and Tenericutes (p= 0.048), possibly because Mollicutes represent the class level, while Tenericutes represent the phylum level, with the latter encompassing the former. This is why these two microbial taxa exhibited similar effects on lung adenocarcinoma. Conversely, Prevotella9 (p = 0.003) and Anaerotruncus (p= 0.009) displayed a direct correlation with the risk of lung adenocarcinoma. It is worth noting that the Holdemanella has exhibited remarkable efficacy in both datasets. The Peptococcaceae was also found to be significant after P-value adjustment (p_adj = 0.04).
For squamous cell lung cancer, as demonstrated in Supplementary Figure 3, nine bacteria were identified by MR analysis as being associated with squamous cell lung cancer. Genetically predicted risk of squamous cell lung cancer was correlated with Ruminococcustorquesgroup (P = 0.022), Actinomyces (p = 0.004), Eggerthella (P = 0.041), Flavonifractor (P = 0.025), and Peptococcus (P = 0.014). The genetically predicted protective roles of microbiota are Eubacterium eligens (P = 0.042), Collinsella (p = 0.04), Lachnospiraceae (p = 0.03), and Ruminiclostridium6 (p = 0.048).
Furthermore, we also identified 11 bacteria associated with small cell lung cancer, as illustrated in Supplementary Figure 4. The results of IVW showed a causal correlation of Actinomycetaceae (P = 0.029), Actinomycetales (P = 0.029), Anaerofilum (P = 0.022), Ruminiclostridium6 (P = 0.037) Eubacterium oxidoreducens (P = 0.02), Bilophila (p = 0.033), and Intestinibacter (P = 0.011) on the risk of small cell lung cancer. Furthermore, the microbial communities Christensenellaceae (P = 0.002), Lachnospiraceae (P = 0.04), Barnesiella (P = 0.009), and Butyricimonas (P = 0.046) conferred a protective effect against small cell lung cancer.
3.2 Sensitivity analysis
Sensitivity analyses were performed to ensure the results’ robustness. The findings of Cochran’s Q showed that the instrumental variables were homogeneous. According to the results of the MR-Egger regression, no evidence of pleiotropic effect was observed (all P intercept >0.05), as well as MR-PRESSO global test (all P global test >0.05); this finding shows that IVs seem to be unlikely to influence lung cancer risk through pathways other than the GM with significant results. All results can be found in the Supplementary File. Furthermore, Supplementary Figures 5-8 summarize the results of the causal effect of significant taxa on various subtypes of lung cancer in the leave-one-out analysis.
3.3 Reverse MR analysis
A reverse MR analysis was carried out to investigate whether different types of lung cancer have any causal impact on the observed significant bacteria. The reverse MR analysis process was identical to the former MR analysis. The majority of results indicated that there is no reverse causality (Supplementary Tables 2-4). Only the effect of small cell lung cancer on Holdemania exhibited a reverse causal relationship, and to ensure the rigor of our results, we excluded this finding.
3.4 MVMR analysis
To ascertain whether the significantly positive microbial community observed after calibration directly or indirectly affects cancer risk through common cancer risk factors, we performed additional MVMR analysis. Although multivariable Mendelian randomization (MR) has been used in some previous studies to consider the joint effects of multiple variables, we did not find that this approach was suitable for our current research. After considering important confounding factors such as smoking, alcohol consumption, and chronic obstructive pulmonary disease in our analysis, we were unable to identify any common SNPs across the majority of the GM, which limits the ability to establish a robust causal relationship using multivariable MR; the results are shown in Supplementary Table 5. Previous studies have shown that conducting multivariable MR analyses in the presence of insufficient common SNPs may lead to the accumulation of bias and errors in conclusions, lacking scientific justification (Sanderson, 2021). Only Peptococcus yielded common SNPs during the multivariable Mendelian randomization analysis; however, after adjustment, the results were no longer significant.
4 Discussion
In this study, we performed a two-sample MR analysis using the summary statistics of the GM from the MiBioGen consortium’s largest GWAS data and the summary statistics of various subtypes of lung cancer from the TRICL, ILCCO, and FinnGen consortium R7 release data to evaluate the causal association between GM and lung cancer. We found 10 GM to be causally associated with overall lung cancer, 10 with lung adenocarcinoma, 9 with squamous cell carcinoma, and 11 with small cell lung cancer. After FDR correction, we found Peptococcaceae to be causally associated with lung adenocarcinoma.
The GM primarily influences the occurrence of lung cancer via the gut-lung axis, exerting its effects by regulating the lung immune system, influencing lung inflammation response, and producing metabolites (Zhao et al., 2021). Alterations in the GM may lead to disruption of the intestinal mucosal barrier, resulting in the occurrence of inflammation. These inflammatory factors may enter the lungs through the gut-lung axis (Enaud et al., 2020; Zhao et al., 2021), and long-term inflammatory responses can promote the occurrence of lung cancer by activating signal transduction pathways. In addition, intestinal immune cells such as ILC2s, ILC3, and TH17 can migrate directly from the intestine to the respiratory tract via the bloodstream to influence the immune activity of the respiratory system (Ma et al., 2022). The GM can also produce various metabolites, such as SCFAs, carotenoids, and bile acids (Du et al., 2022; Soriano-Lerma et al., 2022). These metabolites may directly or indirectly affect the occurrence of lung cancer by entering the lungs through the gut-lung axis.
The family of Bifidobacteriaceae, as a probiotic, has been reported in a study to have a protective effect against oxidative stress-induced DNA damage in vitro. This study suggests that Bifidobacterium bifidum, a member of the Bifidobacteriaceae family, possesses antioxidant properties that may prevent diseases such as lung cancer by reducing DNA damage (Bhatt et al., 2017). Our research findings are consistent with the notion that Bifidobacteriaceae has a strong causal relationship with lung cancer and serves as a protective factor against it. With regard to cyanobacteria, phycocyanin can be produced, which has a variety of biological functions such as anti-tumor, anti-oxidant, immunomodulatory, and anti-inflammatory activities. Several studies have demonstrated that phycocyanin exerts a dual role in NSCLC cells by not only reducing the activity and proliferation of A549 cells (Hao et al., 2018a), but also inhibiting the proliferation of various NSCLC cell lines (such as H1299, H460, and LTEP-a2) while inducing apoptosis (Hao et al., 2018b). These findings provide a basis for future applications of cyanobacteria in the treatment of NSCLC. It is worth mentioning that our findings align with those of Nam et al., who found that patients with the inflammatory disease “rosacea” had lower levels of Peptococcaceae in the gut (Nam et al., 2018). Furthermore, chronic inflammation is known to be linked to lung cancer. Based on this, we propose that Peptococcaceae may play a role in mitigating lung inflammation damage and could potentially impact the development of lung cancer. However, there is a lack of relevant research evidence to confirm the specific mechanism of the potential causal link between these specific microbial taxa and the incidence of lung cancer.
The study by J et al. suggests that the dysbiosis of lung microbiota in lung cancer patients is associated with upregulation of the PI3K signaling pathway and cancer progression. Specific lung cancer-associated bacteria, such as Prevotella, may impact the tumor microenvironment and initiate a cascade reaction in cancer cells, leading to the upregulation of the PI3K/AKT signaling pathway and cancer development. Overall, this basic research reveals the potential role of Prevotella and other specific lung cancer-associated bacteria in lung cancer development, providing a possible avenue for researching new preventive and therapeutic strategies (He et al., 2022). There is also a study that shows a potential association between Actinomyces and lung cancer development. The relative abundance of Actinomyces is increased in both COPD and NSCLC patients, and its abundance is positively correlated with the risk of developing lung cancer. This study reveals an association between the dysbiosis of lung microbiota and the occurrence of lung cancer. However, further research is necessary to determine the precise impact of Actinomyces on lung cancer development (Peters et al., 2022). It is worth noting that there is currently no research directly determining how the genus Ruminiclostridium 6 and Actinomycetales influence lung cancer. However, a study has shown that patients with a higher relative abundance of Actinomycetales have a lower risk of lung cancer recurrence after surgery (Wei et al., 2023). Further research is needed to determine the exact mechanism of action of Actinomycetales and other bacterial genera in lung cancer development.
It is worth noting that our research shows significant differences in results when compared to two other studies, those by Long et al. and Wei et al (Long et al., 2023; Wei et al., 2023), with the primary difference being that we used a larger and more comprehensive database. This makes our results more reliable, confirming previous research and providing a novel perspective on the potential causal relationship between GM and lung cancer. Additionally, we had stringent requirements for maintaining consistency between beta values and the IVW method in our analysis, which led to the exclusion of certain results due to slight differences. Additionally, unlike studies with limited sample sizes or specific populations, the use of MR studies has the advantage of overcoming confounding factors. MR uses natural genetic variation as instrumental variables to explore causality between the exposure and outcome. This approach can eliminate reverse causality and collinearity and exclude most confounding factors during the estimation process, leading to results that are more likely to reflect the true cause-effect relationship. However, there may still be some confounding factors present in the study, such as smoking, diet, medications, and COPD, which could interfere with the composition of the GM and increase the risk of developing lung cancer. As such, future research should continue to explore and implement advanced methods to reduce the influence of confounding factors.
This research has several advantages. First, we utilized MR analysis to minimize the effects of reverse causation and confounding, thus enhancing the causal inference of lung cancer and the GM. Second, we chose a more comprehensive and larger database, which yielded more results. Additionally, to confirm the robustness of our results, we also employed the MR-Egger regression intercept term test and MR-PRESSO. Nevertheless, this study still has some limitations. One limitation is that the molecular biology of the GM is not yet fully understood, which limits our ability to deduce the molecular mechanisms that link GM and lung cancer. Additionally, the lung cancer cases are primarily from cross-sectional studies, which may introduce a survival bias effect. At the same time, potential influential factors such as smoking, diet, or treatment effects may also affect the results of genome-wide association studies (GWAS). Finally, the majority of data in this study comes from European populations, and while we have included some other ethnic groups, our findings may be somewhat biased.
5 Conclusions
By conducting an MR analysis on the causal relationship between the GM and various subtypes of lung cancer, we identified potential causal associations between 10 microbial communities and lung cancer, 10 with lung adenocarcinoma, 9 with lung squamous cell carcinoma, and 11 with small cell lung cancer. Following correction, Peptococcaceae still displayed a strong causal relationship with lung adenocarcinoma. However, definitive experimental research is required to further explore the precise mechanism underlying this relationship.
Data availability statement
The datasets presented in this study can be found in online repositories. The names of the repository/repositories and accession number(s) can be found in the article/Supplementary Material.
Author contributions
MZ and YW contributed to the concept and design of this study. YL and KW were responsible for the statistical analysis and writing of the report. YZ and JY reviewed the article and provided critical feedback to improve and structure the report. YL and KW are regarded as co-first authors with the same degree of contribution. All authors contributed to the article and approved the submitted version.
Funding
This work received support from the following funding sources: Scientific Research Foundation of Education Department in Liaoning Province (Grant LJKZ0739), Science and Technology Plan Project of Shenyang (Grant 21-173-9-30), Educational funding of Liaoning Province (Grant No: LJKZ0739), Social Scientific planning funding (Grant No: L21BGL059), the planned projects of Liaoning provincial central government guiding local science and technology development funding (Grant No: 2022010127-JH6/1001), and Scientific Research Funding Project of Education Department of Liaoning Province (Grant No. JCZR2020010).
Acknowledgments
We thank the ILCCO, TRICL, FinnGen consortium, and MiBioGen consortium for providing GWAS summary statistics data for our analysis.
Conflict of interest
The authors declare that the research was conducted in the absence of any commercial or financial relationships that could be construed as a potential conflict of interest.
Publisher’s note
All claims expressed in this article are solely those of the authors and do not necessarily represent those of their affiliated organizations, or those of the publisher, the editors and the reviewers. Any product that may be evaluated in this article, or claim that may be made by its manufacturer, is not guaranteed or endorsed by the publisher.
Supplementary material
The Supplementary Material for this article can be found online at: https://www.frontiersin.org/articles/10.3389/fcimb.2023.1200299/full#supplementary-material
Supplementary Figure 1 | Scatter plots for the causal association between gut microbiota and lung cancer. MR, Mendelian randomization; SNP, single-nucleotide polymorphism.
Supplementary Figure 2 | Scatter plots for the causal association between gut microbiota and lung adenocarcinoma. MR, Mendelian randomization; SNP, single-nucleotide polymorphism.
Supplementary Figure 3 | Scatter plots for the causal association between gut microbiota and squamous cell lung cancer. MR, Mendelian randomization; SNP, single-nucleotide polymorphism.
Supplementary Figure 4 | Scatter plots for the causal association between gut microbiota and small cell lung cancer. MR, Mendelian randomization; SNP, single-nucleotide polymorphism.
Supplementary Figure 5 | Leave-one-out plots for the causal association between gut microbiota and lung cancer. MR, Mendelian randomization.
Supplementary Figure 6 | Leave-one-out plots for the causal association between gut microbiota and lung adenocarcinoma. MR, Mendelian randomization.
Supplementary Figure 7 | Leave-one-out plots for the causal association between gut microbiota and squamous cell lung cancer. MR, Mendelian randomization.
Supplementary Figure 8 | Leave-one-out plots for the causal association between gut microbiota and small cell lung cancer. MR, Mendelian randomization.
References
Arrieta, M. C., Stiemsma, L. T., Dimitriu, P. A., Thorson, L., Russell, S., Yurist-Doutsch, S., et al. (2015). Early infancy microbial and metabolic alterations affect risk of childhood asthma. Sci. Trans. Med. 7 (307), 307ra152–307ra152. doi: 10.1126/scitranslmed.aab2271
Bhatt, A. P., Redinbo, M. R., Bultman, S. J. (2017). The role of the microbiome in cancer development and therapy. CA Cancer J. Clin. 67 (4), 326–344. doi: 10.3322/caac.21398
Budden, K. F., Gellatly, S. L., Wood, D. L. A., Cooper, M. A., Morrison, M., Hugenholtz, P., et al. (2017). Emerging pathogenic links between microbiota and the gut–lung axis. Nat. Rev. Microbiol. 15 (1), 55–63. doi: 10.1038/nrmicro.2016.142
Corrêa, R. O., Castro, P. R., Moser, R., Ferreira, C. M., Quesniaux, V. F. J., Vinolo, M. A. R., et al. (2022). Butyrate: Connecting the gut-lung axis to the management of pulmonary disorders. Front. Nutr. 9, 1011732. doi: 10.3389/fnut.2022.1011732
Dang, A. T., Marsland, B. J. (2019). Microbes, metabolites, and the gut–lung axis. Mucosal Immunol. 12 (4), 843–850. doi: 10.1038/s41385-019-0160-6
Du, Y., Li, X., An, Y., Song, Y., Lu, Y. (2022). Association of gut microbiota with sort-chain fatty acids and inflammatory cytokines in diabetic patients with cognitive impairment: A cross-sectional, non-controlled study. Front. Nutr. 9, 930626. doi: 10.3389/fnut.2022.930626
Enaud, R., Prevel, R., Ciarlo, E., Beaufils, F., Wieërs, G., Guery, B., et al. (2020). The gut-lung axis in health and respiratory diseases: A place for inter-organ and inter-kingdom crosstalks. Front. Cell Infect. Microbiol. 10, 9. doi: 10.3389/fcimb.2020.00009
Ge, Y., Wang, X., Guo, Y., Yan, J., Abuduwaili, A., Aximujiang, K., et al. (2021). Gut microbiota influence tumor development and Alter interactions with the human immune system. J. Exp. Clin. Cancer Res. 40, 42. doi: 10.1186/s13046-021-01845-6
Georgiou, K., Marinov, B., Farooqi, A. A., Gazouli, M. (2021). Gut microbiota in lung cancer: where do we stand? Int. J. Mol. Sci. 22 (19), 10429. doi: 10.3390/ijms221910429
Hao, S., Li, S., Wang, J., Zhao, L., Yan, Y., Cao, Q., et al. (2018a). Transcriptome analysis of phycocyanin-mediated inhibitory functions on non-small cell lung cancer A549 cell growth. Mar. Drugs 16 (12), 511. doi: 10.3390/md16120511
Hao, S., Yan, Y., Li, S., Zhao, L., Zhang, C., Liu, L., et al. (2018b). The in vitro anti-tumor activity of phycocyanin against non-small cell lung cancer cells. Mar. Drugs 16 (6), 178. doi: 10.3390/md16060178
He, J. Q., Chen, Q., Wu, S. J., Wang, D. Q., Zhang, S. Y., Zhang, S. Z., et al. (2022). Potential implications of the lung microbiota in patients with chronic obstruction pulmonary disease and non-small cell lung cancer. Front. Cell Infect. Microbiol. 12, 937864. doi: 10.3389/fcimb.2022.937864
Hemani, G., Tilling, K., Davey Smith, G. (2017). Orienting the causal relationship between imprecisely measured traits using GWAS summary data. PloS Genet. 13 (11), e1007081. doi: 10.1371/journal.pgen.1007081
Huang, J., Deng, Y., Tin, M. S., Lok, V., Ngai, C. H., Zhang, L., et al. (2022). Distribution, risk factors, and temporal trends for lung cancer incidence and mortality: A global analysis. CHEST. 161 (4), 1101–1111. doi: 10.1016/j.chest.2021.12.655
Jin, C., Lagoudas, G. K., Zhao, C., Bullman, S., Bhutkar, A., Hu, B., et al. (2019). Commensal microbiota promote lung cancer development via γδ T cells. Cell. 176 (5), 998–1013.e16. doi: 10.1016/j.cell.2018.12.040
Kurilshikov, A., Medina-Gomez, C., Bacigalupe, R., Radjabzadeh, D., Wang, J., Demirkan, A. (2023) Large-scale association analyses identify host factors influencing human gut microbiome composition - PMC. Available at: https://www.ncbi.nlm.nih.gov/pmc/articles/PMC8515199/.
Li, P., Wang, H., Guo, L., Gou, X., Chen, G., Lin, D., et al. (2022). Association between gut microbiota and preeclampsia-eclampsia: a two-sample Mendelian randomization study. BMC Med. 20 (1), 443. doi: 10.1186/s12916-022-02657-x
Liu, X., Cheng, Y., Zang, D., Zhang, M., Li, X., Liu, D., et al. (2021). The role of gut microbiota in lung cancer: from carcinogenesis to immunotherapy. Front. Oncol. 11, 720842. doi: 10.3389/fonc.2021.720842
Liu, K., Zou, J., Fan, H., Hu, H., You, Z. (2022). Causal effects of gut microbiota on diabetic retinopathy: A Mendelian randomization study. Front. Immunol. 13, 930318. doi: 10.3389/fimmu.2022.930318
Long, Y., Tang, L., Zhou, Y., Zhao, S., Zhu, H. (2023). Causal relationship between gut microbiota and cancers: a two-sample Mendelian randomisation study. BMC Med. 21, 66. doi: 10.1186/s12916-023-02761-6
Lv, W. Q., Lin, X., Shen, H., Liu, H. M., Qiu, X., Li, B. Y., et al. (2021). Human gut microbiome impacts skeletal muscle mass via gut microbial synthesis of the short-chain fatty acid butyrate among healthy menopausal women. J. Cachexia Sarcopenia Muscle. 12 (6), 1860–1870. doi: 10.1002/jcsm.12788
Ma, P. J., Wang, M. M., Wang, Y. (2022). Gut microbiota: A new insight into lung diseases. Biomedicine Pharmacotherapy. 155, 113810. doi: 10.1016/j.biopha.2022.113810
Nam, J. H., Yun, Y., Kim, H. S., Kim, H. N., Jung, H. J., Chang, Y., et al. (2018). Rosacea and its association with enteral microbiota in Korean females. Exp. Dermatol. 27 (1), 37–42. doi: 10.1111/exd.13398
Peters, B. A., Pass, H. I., Burk, R. D., Xue, X., Goparaju, C., Sollecito, C. C., et al. (2022). The lung microbiome, peripheral gene expression, and recurrence-free survival after resection of stage II non-small cell lung cancer. Genome Med. 14 (1), 121. doi: 10.1186/s13073-022-01126-7
Pierce, B. L., Burgess, S. (2013). Efficient design for mendelian randomization studies: subsample and 2-sample instrumental variable estimators. Am. J. Epidemiol. 178 (7), 1177–1184. doi: 10.1093/aje/kwt084
Ren, F., Jin, Q., Liu, T., Ren, X., Zhan, Y. (2023). Causal effects between gut microbiota and IgA nephropathy: a bidirectional Mendelian randomization study. Front. Cell Infect. Microbiol. 13, 1171517. doi: 10.3389/fcimb.2023.1171517
Samet, J. M., Avila-Tang, E., Boffetta, P., Hannan, L. M., Olivo-Marston, S., Thun, M. J., et al. (2009). Lung cancer in never smokers: clinical epidemiology and environmental risk factors. Clin. Cancer Res. 15 (18), 5626–5645. doi: 10.1158/1078-0432.CCR-09-0376
Sanderson, E. (2021). Multivariable mendelian randomization and mediation. Cold Spring Harb. Perspect. Med. 11 (2), a038984. doi: 10.1101/cshperspect.a038984
Soriano-Lerma, A., García-Burgos, M., Alférez, M. J. M., Pérez-Carrasco, V., Sanchez-Martin, V., Linde-Rodríguez, Á., et al. (2022). Gut microbiome–short-chain fatty acids interplay in the context of iron deficiency anaemia. Eur. J. Nutr. 61 (1), 399–412. doi: 10.1007/s00394-021-02645-6
Su, Q., Jin, C., Bo, Z., Yang, Y., Wang, J., Wang, J., et al. (2023). Association between gut microbiota and gastrointestinal cancer: a two-sample bi-directional Mendelian randomization study. Front. Microbiol. 14, 1181328. doi: 10.3389/fmicb.2023.1181328
Sung, H., Ferlay, J., Siegel, R. L., Laversanne, M., Soerjomataram, I., Jemal, A., et al. (2021). Global cancer statistics 2020: GLOBOCAN estimates of incidence and mortality worldwide for 36 cancers in 185 countries. CA: A Cancer J. Clin. 71 (3), 209–249. doi: 10.3322/caac.21660
Thai, A. A., Solomon, B. J., Sequist, L. V., Gainor, J. F., Heist, R. S. (2021). Lung cancer. Lancet 398 (10299), 535–554. doi: 10.1016/S0140-6736(21)00312-3
Ubachs, J., Ziemons, J., Soons, Z., Aarnoutse, R., van Dijk, D. P. J., Penders, J., et al. (2021). Gut microbiota and short-chain fatty acid alterations in cachectic cancer patients. J. Cachexia Sarcopenia Muscle. 12 (6), 2007–2021. doi: 10.1002/jcsm.12804
Verbanck, M., Chen, C. Y., Neale, B., Do, R. (2018). Detection of widespread horizontal pleiotropy in causal relationships inferred from Mendelian randomization between complex traits and diseases. Nat. Genet. 50 (5), 693–698. doi: 10.1038/s41588-018-0099-7
Wang, B. Y., Huang, J. Y., Chen, H. C., Lin, C. H., Lin, S. H., Hung, W. H., et al. (2020). The comparison between adenocarcinoma and squamous cell carcinoma in lung cancer patients. J. Cancer Res. Clin. Oncol. 146 (1), 43–52. doi: 10.1007/s00432-019-03079-8
Wei, Z., Yang, B., Tang, T., Xiao, Z., Ye, F., Li, X., et al. (2023). Gut microbiota and risk of five common cancers: A univariable and multivariable Mendelian randomization study. Cancer Med. 12 (9), 10393–10405. doi: 10.1002/cam4.5772
Xu, Q., Ni, J. J., Han, B. X., Yan, S. S., Wei, X. T., Feng, G. J., et al. (2021). Causal relationship between gut microbiota and autoimmune diseases: A two-sample mendelian randomization study. Front. Immunol. 12, 746998. doi: 10.3389/fimmu.2021.746998
Young, R. P., Hopkins, R. J., Marsland, B. (2016). The gut–liver–lung axis. Modulation of the innate immune response and its possible role in chronic obstructive pulmonary disease. Am. J. Respir. Cell Mol. Biol. 54 (2), 161–169. doi: 10.1165/rcmb.2015-0250PS
Zhang, D., Li, S., Wang, N., Tan, H. Y., Zhang, Z., Feng, Y. (2020). The cross-talk between gut microbiota and lungs in common lung diseases. Front. Microbiol. 11, 301. doi: 10.3389/fmicb.2020.00301
Zhao, Y., Liu, Y., Li, S., Peng, Z., Liu, X., Chen, J., et al. (2021). Role of lung and gut microbiota on lung cancer pathogenesis. J. Cancer Res. Clin. Oncol. 147 (8), 2177–2186. doi: 10.1007/s00432-021-03644-0
Keywords: lung cancer, gut microbiota, Mendelian randomization, causal inference, genome-wide association study
Citation: Li Y, Wang K, Zhang Y, Yang J, Wu Y and Zhao M (2023) Revealing a causal relationship between gut microbiota and lung cancer: a Mendelian randomization study. Front. Cell. Infect. Microbiol. 13:1200299. doi: 10.3389/fcimb.2023.1200299
Received: 04 April 2023; Accepted: 18 August 2023;
Published: 27 September 2023.
Edited by:
Tao Lin, Baylor College of Medicine, United StatesReviewed by:
Robert P. Young, The University of Auckland, New ZealandYotsawat Pomyen, Chulabhorn Research Institute, Thailand
Copyright © 2023 Li, Wang, Zhang, Yang, Wu and Zhao. This is an open-access article distributed under the terms of the Creative Commons Attribution License (CC BY). The use, distribution or reproduction in other forums is permitted, provided the original author(s) and the copyright owner(s) are credited and that the original publication in this journal is cited, in accordance with accepted academic practice. No use, distribution or reproduction is permitted which does not comply with these terms.
*Correspondence: Ying Wu, d3V5aW5nQGNtdS5lZHUuY24=; Mingfang Zhao, emhhb21mNjE4QDEyNi5jb20=
†These authors have contributed equally to this work