- 1Department of Spine Surgery and Orthopaedics, Xiangya Hospital, Central South University, Changsha, China
- 2National Clinical Research Center for Geriatric Disorders, Xiangya Hospital, Central South University, Changsha, China
Background: Early diagnosis of spinal tuberculosis (STB) remains challenging. The aim of this study was to develop a predictive model for the early diagnosis of STB based on conventional laboratory indicators.
Method: The clinical data of patients with suspected STB in four hospitals were included, and variables were screened by Lasso regression. Eighty-five percent of the cases in the dataset were randomly selected as the training set, and the other 15% were selected as the validation set. The diagnostic prediction model was established by logistic regression in the training set, and the nomogram was drawn. The diagnostic performance of the model was verified in the validation set.
Result: A total of 206 patients were included in the study, including 105 patients with STB and 101 patients with NSTB. Twelve variables were screened by Lasso regression and modeled by logistic regression, and seven variables (TB.antibody, IGRAs, RBC, Mono%, RDW, AST, BUN) were finally included in the model. AUC of 0.9468 and 0.9188 in the training and validation cohort, respectively.
Conclusion: In this study, we developed a prediction model for the early diagnosis of STB which consisted of seven routine laboratory indicators.
1 Introduction
Tuberculosis (TB) is a chronic infectious disease caused by infection with Mycobacterium tuberculosis, and it is the oldest infectious disease that has been identified in humans (Scorrano et al., 2022). According to the latest Global TB Report published by the World Health Organization in 2021, in 2020, an estimated 10 million people worldwide had tuberculosis. This included 5.6 million men, 3.3 million women, and 1.1 million children. In 2020, an estimated 1.5 million people died from TB, making it the second highest infectious disease killer after novel coronavirus pneumonia (World Health Organization, 2021).
Spinal tuberculosis (STB) is an extrapulmonary manifestation of tuberculosis that occurs as a secondary infection caused by Mycobacterium tuberculosis in the spinal vertebrae and adnexal tissues. STB accounts for approximately 2% of all tuberculosis cases and 50% of all osteoarticular tuberculosis cases. Worldwide, the annual incidence of spinal tuberculosis exceeds 100,000 (Khanna and Sabharwal, 2019a). STB can cause bone destruction, collapse, and fracture of the vertebral body, which can compress the spinal cord and cause paraplegia in approximately 10%-30% of affected patients (Hristea et al., 2008). The devastating and disabling effects of STB cannot be ignored, and early diagnosis of STB is essential to reduce the incidence of postoperative complications in patients. The gold standard for the diagnosis of spinal tuberculosis involves invasive tests such as surgical needle biopsy puncture to obtain a specimen of the lesion; diagnosis is then determined by using techniques such as Mycobacterium tuberculosis culture and Xpert, but this often occurs late in the patient’s hospitalization (Held et al., 2014; Beltran et al., 2022). If the patient receives an accurate diagnosis early in the course of the disease, the spine surgeon can recommend early treatment with anti-tuberculosis drugs, thereby improving the patient’s prognosis (Li et al., 2020).
However, early diagnosis in patients with spinal tuberculosis has been difficult, and cases are frequently missed or misdiagnosed (Mann et al., 2021). Patients with spinal tuberculosis typically lack early clinical symptoms; it is also difficult to distinguish STB from diseases such as septic spondylitis and spinal tumors, which have similar imaging manifestations as spinal tuberculosis, including bone destruction (Liu et al., 2021; Rule et al., 2021). Moreover, routine laboratory tests of inflammatory markers such as blood sedimentation, white blood cell count, and C-reactive protein are not specific for the diagnosis of tuberculosis, and these markers are also elevated in septic spondylitis (Liu et al., 2022). Interferon gamma release assays (IGRAs) have shown better diagnostic efficacy, as confirmed by our previously published study (Hu et al., 2022). However, we also observed that IGRAs alone do not meet the clinical need for diagnostic accuracy in spinal tuberculosis. Additionally, IGRAs cannot distinguish between active and latent tuberculosis and show no correlation with the duration of disease, limiting its use in the diagnosis of spinal tuberculosis (Hamada et al., 2021).
In our present study, we collected routine laboratory serology upon admission of patients with spinal tuberculosis, screened variables by lasso regression, built a model by logistic regression, and drew a nomogram for the early diagnosis of spinal tuberculosis based on a model used for patients in a multicenter retrospective study.
2 Method
2.1 Inclusion and exclusion criteria, and diagnosis criteria
This study included 206 patients with suspected spinal tuberculosis who were admitted to the Xiangya Hospital of Central South University, Xiangya Boai Hospital, Changsha First Hospital, and Hunan Chest Hospital from January 2016 to October 2022. The data of the included patients were taken from the first routine serological examination at the time of patient admission for treatment. The inclusion criteria were as follows: 1. CT and MRI reports of patients included in the study showed that patients had suspected spinal tuberculosis, including irregular lytic lesions or bone destruction that affect the vertebral body, endplate, or adjacent margin of the intervertebral disc, featuring fragmentary destruction, osteolytic destruction, and local destruction with sclerotic margins. Intervertebral disc destruction or decreased disc height, calcification or bone fragment formation accompanied by abscesses, vertebral collapse or posterior convex deformity, as well as soft tissue involvement and large paravertebral abscesses (Khanna and Sabharwal, 2019b); 2. Patients had complete clinical examination data. The exclusion criteria were as follows: 1. concomitant autoimmune diseases or HIV; 2. infections in multiple sites; and 3. patients with severe systemic chronic underlying diseases.
Patients included in this study were diagnosed with spinal tuberculosis if they met one of the following criteria (Pai et al., 2016): 1. positive culture for Mycobacterium tuberculosis from surgical needle biopsy; 2. positive molecular tests for Mycobacterium tuberculosis (including Xpert and mNGS); 3. relevant pathologic histologic features (at least one of the following features: caseous necrosis, positive acid-fast staining, and granulomatous inflammation) Meanwhile, anti-tuberculosis treatment of patients is effective.
Patients were classified into the NSTB group based on the following criteria: 1. microbiological evidence that the infection was caused by other bacteria, fungi or viruses; 2. pathological histological diagnosis of other lesions of the spine (including septicemia and tumors).
Ethical Approval: Institutional Review Board approval was obtained (IRB#: 201303232). This study was approved by the Ethics Committee of Xiangya Hospital Central South University, and written informed consent was obtained from all patients.
2.2 Routine laboratory test data collection
We collected the following routine laboratory test data at the time of admission for the study population: tubercle bacillus antibody (TB.antibody), interferon-γ release assays (IGRAs), white blood cell (WBC), red blood cell (RBC), hemoglobin (HGB), platelet (PLT), neutrophil (Neut), lymphocyte (lymph), eosinophil (EO), basophil (BASO), monocyte (Mono), neutrophil % (Neut%), lymphocyte % (Lymph%), basophil % (BASO%), eosinophil% (Eo%), monocyte % (Mono%), red blood cell distribution width (RDW), platelet volume (PCT), mean platelet volume (MPV), total protein (TP), albumin (A), globulin (G), albumin/globulin (AG), alanine aminotransferase (ALT), aspartate aminotransferase (AST), blood urea nitrogen (BUN), creatinine (Cr), triglyceride (TG), cholesterol (Chol), high-density lipoprotein (HDL), low-density lipoprotein (LDL), glucose (BS), prothrombin time (PT), activated partial thromboplatin time (APTT), international normalized ratio (INR), D dimer (DD), erythrocyte sedimentation rate (ESR), C-reactive protein (CRP).
2.3 Statistical analysis
All statistical analyses and graphing were based on R version 4.2.2. The “CBCgrps” (Zhang et al., 2017) package was used to draw baseline tables in which categorical variables were analyzed for differences in distribution between the two groups using chi-square tests (or Fisher’s exact probability method). Continuous data that conformed to a normal distribution are described as the mean (± standard deviation) and analyzed for differences between two groups using t tests, and data that did not conform to a normal distribution are described as the median (percentile), and differences between the two groups were analyzed using rank sum tests. The data that did not conform to the normal distribution are described as the median (percentile), and the differences between the two groups were analyzed using the rank sum test. The results of the comparison between the groups are expressed using p values. Heatmaps of correlations between variables were calculated and plotted using the “corrplot” package. The selection of variables was based on Lasso regression, and the continuous variables entered into the Lasso regression were standardized using the min-max transformation method. Lasso regression was performed by the “glmnet” package (nfolds were set to 20). The number of variables within one standard error was selected as the optimal number of variables. Eighty-five percent of the cases were randomly selected as the training set, and the rest of the cases were included in the validation set for the internal validation of the model. The “Rms” package was used to build the logistic regression model. The variables included in the logistic regression were selected based on the Lasso regression screening results, and variables lacking significance in the logistic model were excluded to refine the model. The calibration curves of the prediction model were plotted by the “caret” package. The column line plots were plotted using the “regplot” package, and the ROC curves were calculated and plotted using the “pROC” and “ggplot2” packages.
3 Result
3.1 Characteristics of the patient
A total of 206 patients with suspected spinal tuberculosis were enrolled according to the inclusion and exclusion criteria established for the study. We diagnosed 105 cases as spinal tuberculosis and 101 cases as non-STB according to the diagnostic criteria. The characteristics of the patients, routine laboratory data, and the results of statistical comparisons of these indicators between the STB and NSTB groups are presented in Table 1. There were no significant differences between the two groups in terms of age and sex. Detailed information on the underlying diseases included in the patients’ medical history is provided in Table 2. The NSTB group comprised 45 cases of pyogenic spinal infection, 20 cases of spinal brucellosis, 12 cases of spinal tumors, 18 cases of other non-specific infections, 4 cases of spinal fungal infections, and 2 cases of spinal viral infections (Supplementary Figure 1).
3.2 The performance of individual indicators in conventional laboratory tests for discriminating between STB and NSTB
The results of the univariate analyses for STB patients and NSTB patients are presented in Table 1. We observed significant differences between the two groups in TB.antibody, RBC, mono%, lymph, and IGRAs (p < 0.001). In addition, the BS, Lymph%, A, BUN, AG, ALT, TG, and HGB were significantly different between the two groups (p < 0.05). We plotted ROC curves by using these univariate indicators (Figure 1A) and presented the results of their AUCs in a Lollipop Chart (Figure 1B). In the univariate analysis, we observed that only IGRAs had a high diagnostic validity with an AUC over 0.80, while the other indicators had a low univariate diagnostic validity, none of which exceeded 0.7.
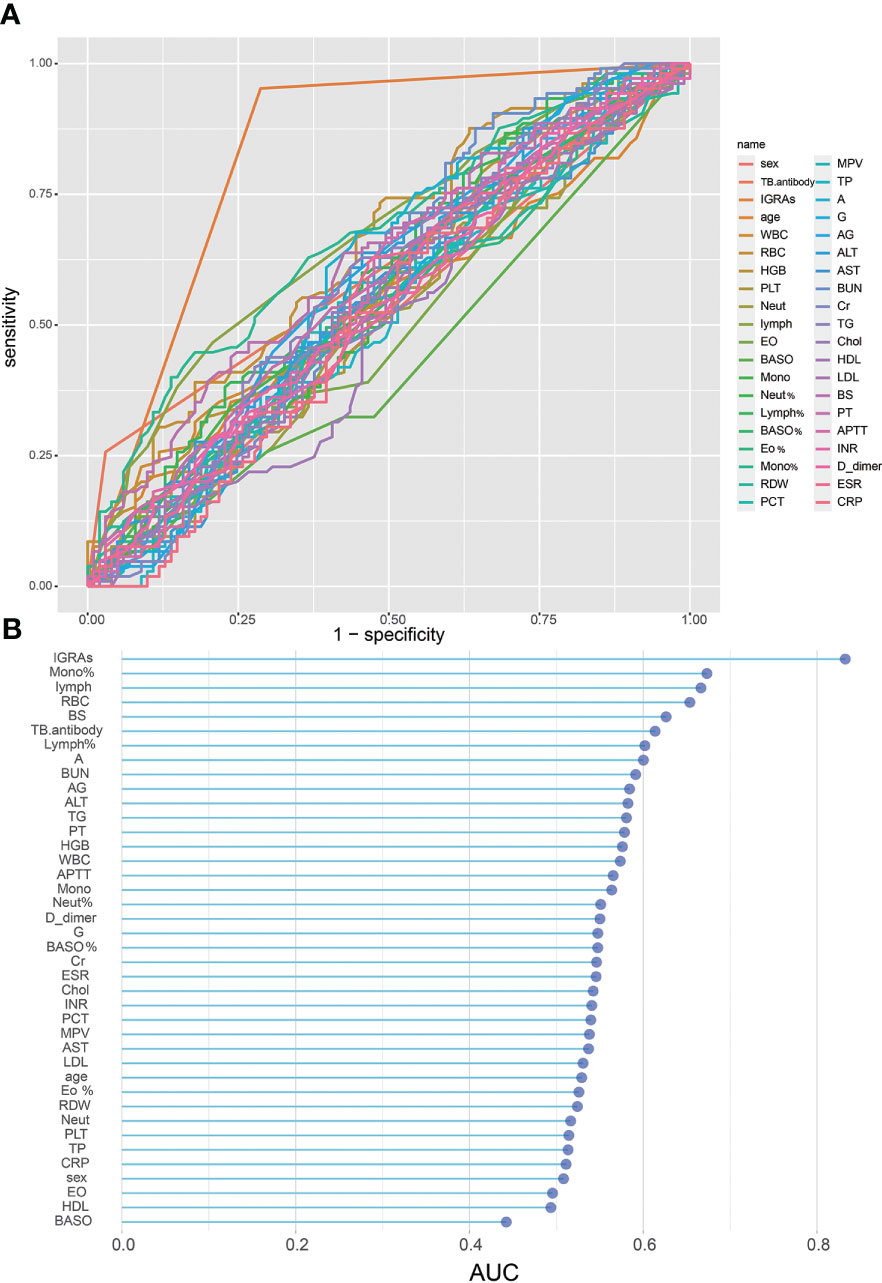
Figure 1 Single variable on STB diagnostic capability: (A), ROC curve of single variable on STB diagnosis; (B), AUC of single variables for STB diagnosis.
3.3 Screening of variables by Lasso regression
We observed a strong correlation between some of the variables in our dataset (Figure 2A). To minimize the effect of covariance between weighted variables for each indicator in the linear model, we used Lasso regression to screen 40 variables (Figures 2B, C), and we identified12 indicators that could be used for subsequent predictive model fitting, including TB.antibody, IGRAs, WBC, RBC, Lymph%, Mono%, RDW, A, AST, BUN, TG, and PT.
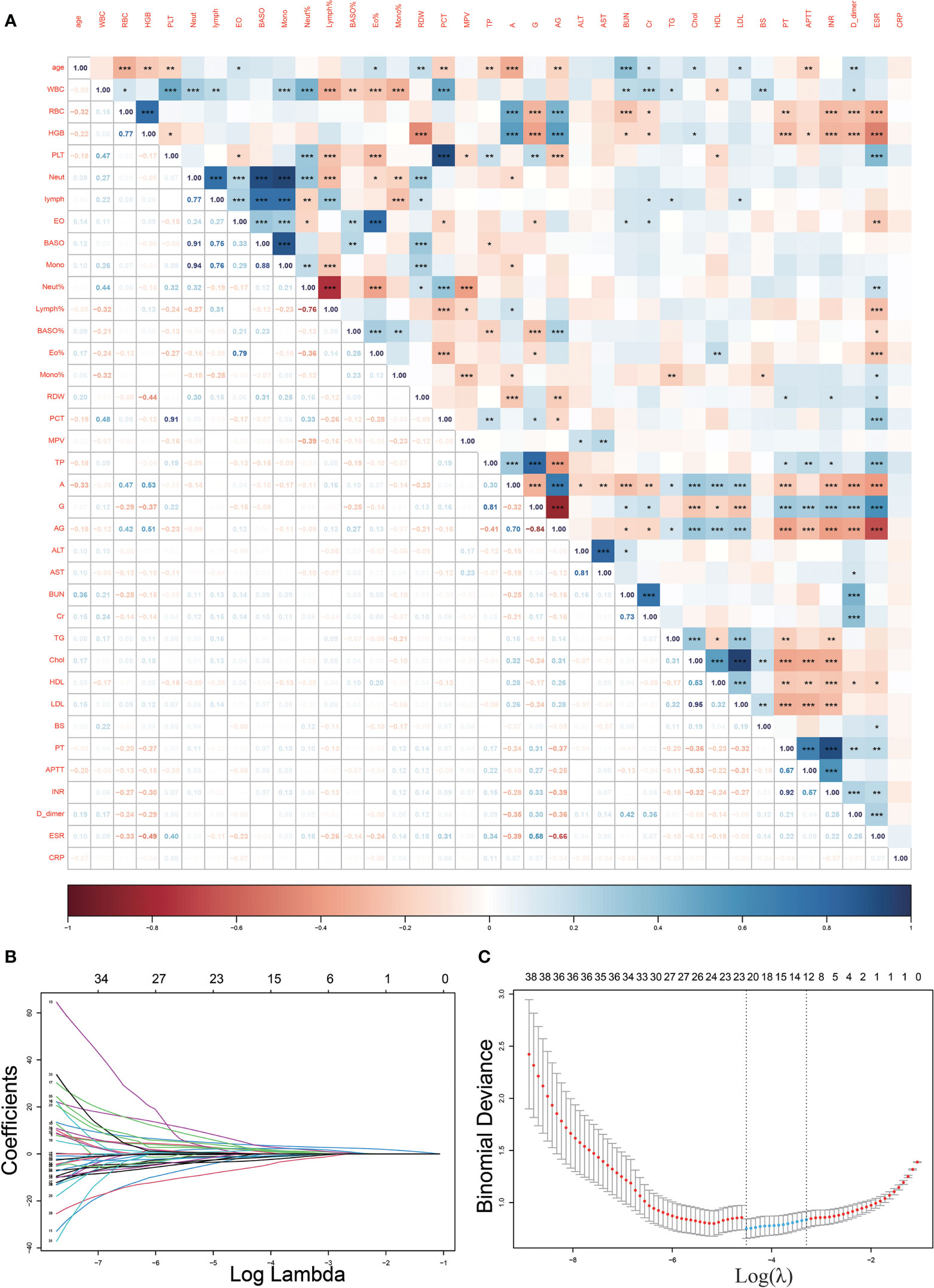
Figure 2 Heat map of correlations between variables and Lasso regression screening variables. (A), The heat map of correlations between continuous variables demonstrates the existence of covariance between many variables (*p<0.05, **p<0.01; ***p<0.001); (B, C), Lasso regression filtered out 12 variables from the 40.
3.4 Establishment of a predictive model for early diagnosis of STB and nomogram
We attempted to build logistic diagnostic models in the training set using twelve variables (TB.antibody, IGRAs, WBC, RBC, Lymph%, Mono%, RDW, A, AST, BUN, TG, PT) screened by Lasso regression, and we excluded variables that were not significant in the logistic regression model. We finally included seven variables, including TB.antibody, IGRAs, RBC, Mono%, RDW, AST, and BUN. We rebuilt the logistic regression model with these seven variables and drew a nomogram based on the logistic regression model that could be used in clinical practice (Figure 3).
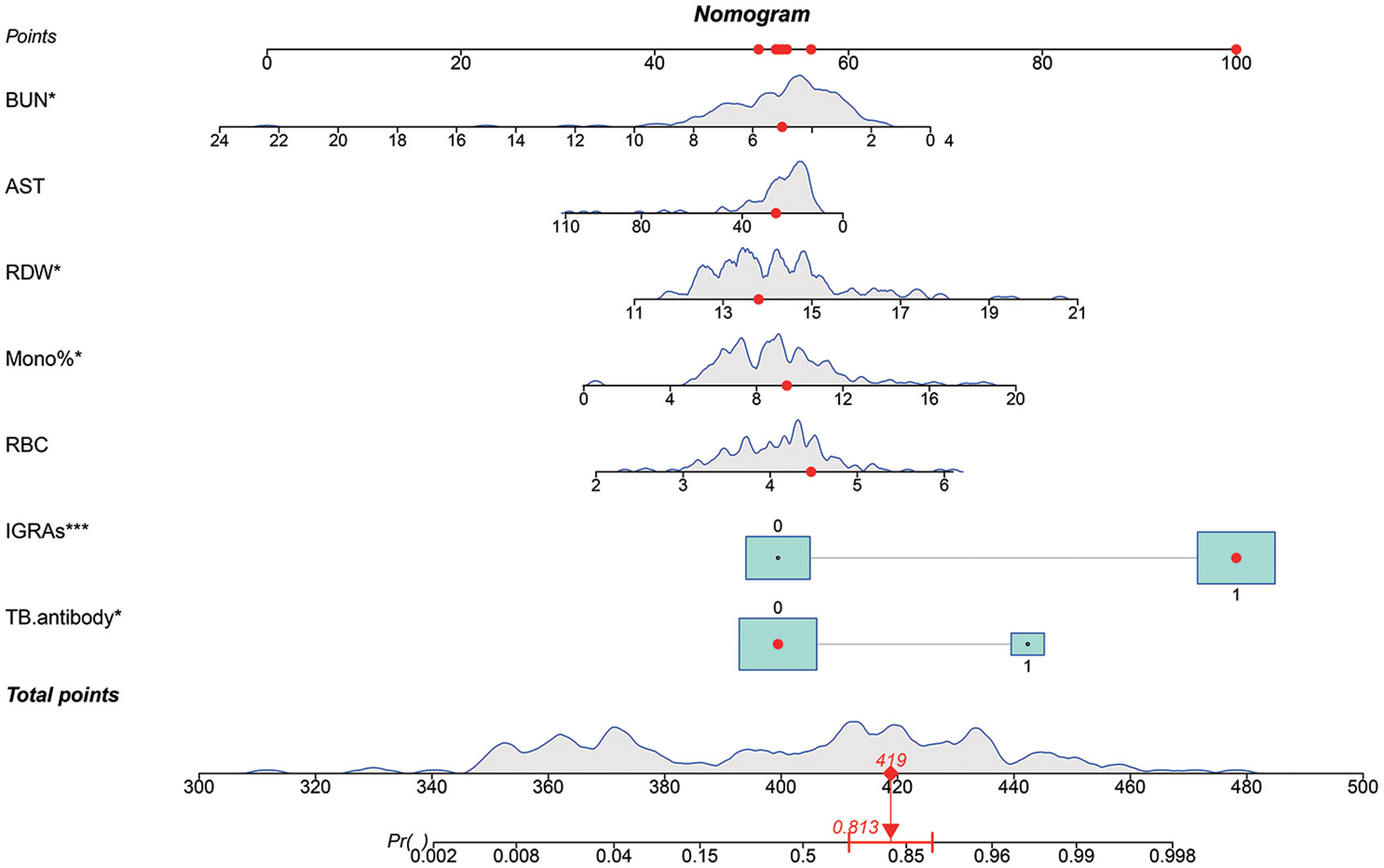
Figure 3 A predictive nomogram model for the early diagnosis of spinal tuberculosis. The red dots in the figure demonstrate the usage of the nomogram. A patient’s information was projected on the nomogram, and this patient had a final score of 419, corresponding to a probability of 0.813 (>0.5), so we judged this patient to have a final diagnosis of spinal tuberculosis (STB). The patient’s final diagnosis was consistent with our prediction.
3.5 Evaluation of a predictive model for early diagnosis of STB
We first plotted the calibration curve of the model in the training set (Figure 4A). We observed the model has a good fit based on the calibration curve. In addition, we plotted the ROC curve of the model for the training cohort, and its AUC was 0.9468 (Figure 4B). Since the model was fitted based on the training cohort, we used an additional validation set to further evaluate the predictive performance of the model. After plotting the ROC curve of the prediction model in the validation cohort, we found that the AUC of the prediction model was 0.9188 (Figure 4C).
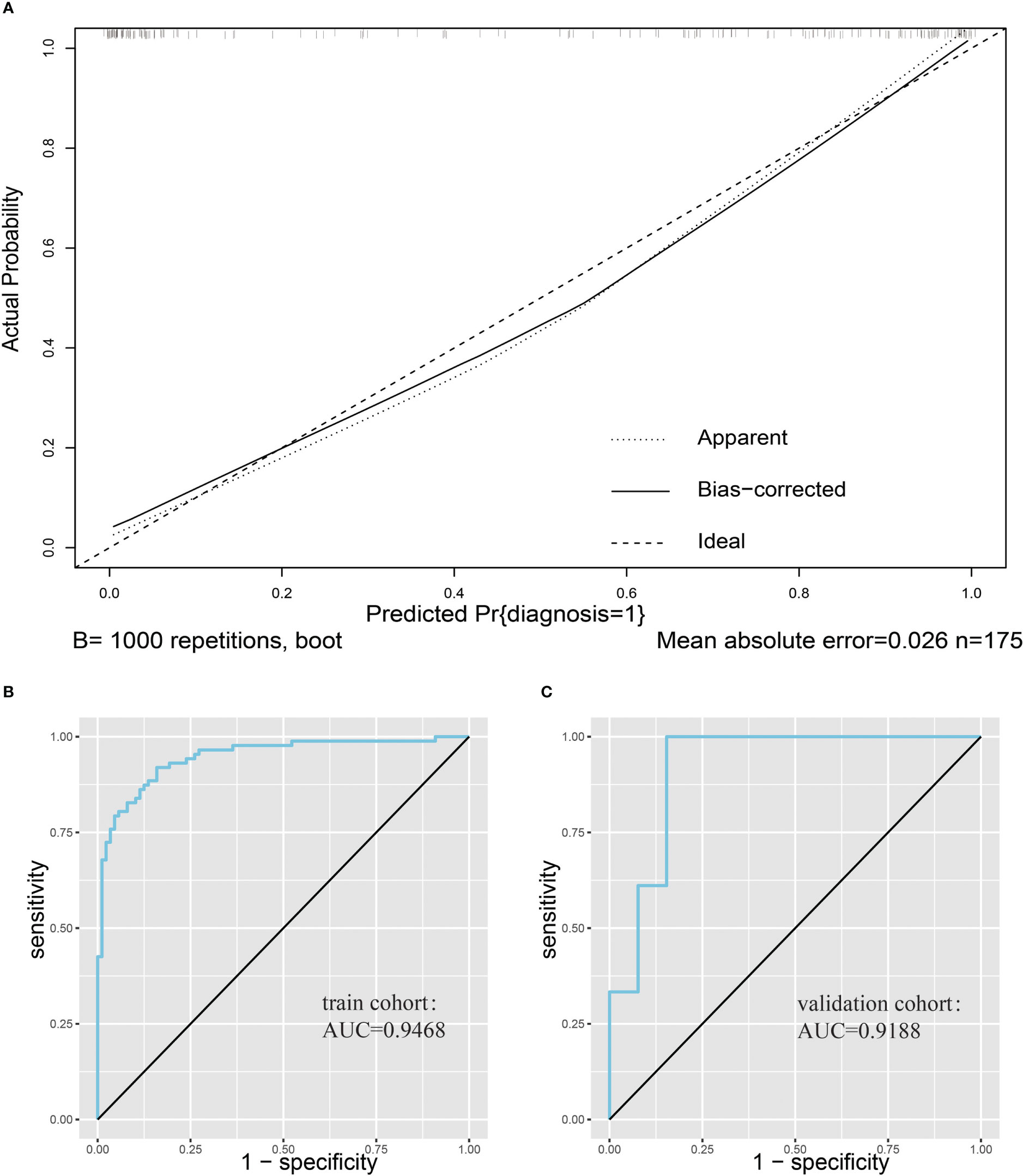
Figure 4 Evaluation of nomogram clinical prediction models. (A), Calibration curve of the model. (B), ROC curve of the model for the training cohort, and its AUC was 0.9468. (C), ROC curve of the model for the validation cohort, and its AUC was 0.9188.
4 Discussion
Early diagnosis of spinal tuberculosis plays an important role in reducing complications and speeding up the recovery of patients (Chen et al., 2016). Early diagnosis of STB can guide physicians to use anti-tuberculosis drugs more effectively. Currently, the early diagnosis of STB before surgery relies on imaging and IGRAs. It is difficult to distinguish STB from septic spinal infections and some spinal tumors, which also manifest as bone destruction (Liu et al., 2021). IGRAs have been shown to be highly sensitive for the diagnosis of spinal tuberculosis (Hu et al., 2022) However, IGRAs have a low specificity for the diagnosis of STB due to their inability to differentiate between active and latent TB. Spine surgeons lack a tool that can be used for early differential diagnosis of spinal tuberculosis, leading to missed and misdiagnosed cases of spinal tuberculosis. Underdiagnosis of spinal tuberculosis delays the initiation of antituberculosis treatment in patients with spinal tuberculosis, preventing the early initiation and regular administration of antituberculosis treatment, whereas misdiagnosis of spinal tuberculosis results in patients with NSTB taking large amounts of antituberculosis drugs that are unnecessary (Dunn and Ben Husien, 2018). Antituberculosis drugs have considerable adverse effects, including hepatotoxicity, allergic reactions, peripheral neuritis, optic nerve damage, and hearing loss (Xu et al., 2017). In addition, the misuse of antituberculosis treatment may lead to the emergence of drug-resistant TB, which has been defined by the World Health Organization as a public health crisis; the incidence of drug-resistant TB has continued to increase in recent years, making the prevention of drug-resistant TB an urgent priority (World Health Organization, ).
In this study, we enrolled patients with suspected spinal tuberculosis from three hospitals. After removing data containing missing values and excluding patients that did not meet the inclusion and diagnostic criteria, a total of 206 eligible patients with suspected spinal tuberculosis were included for analysis. The final diagnosis was determined by postoperative pathogenic microbiology or pathology, and a total of 105 STB cases and 101 NSTB cases were identified. There were no significant differences in age or sex between patients in the STB and NSTB groups. Further univariate analysis of the results of 38 routine laboratory tests in STB patients and NSTB patients demonstrated that there were statistically significant differences between the two groups in TB.antibody, IGRAs, RBC, mono%, lymph, Lymph%, A, AG, BUN, BS, ALT, TG, and HGB (p < 0.05). Nevertheless, by plotting the ROC curves and calculating the AUC, we found that, except for IGRAs, which had good performance in the diagnosis of STB (AUC = 0.8326), other variables did not play a significant role in the diagnosis of STB (AUC < 0.7). An increasing number of studies have shown that one indicator alone is often unable to lead to a valid and accurate diagnosis, and the use of multiple indicators is more widely applicable. To meet practical clinical needs and minimize test costs, combining multiple routine laboratory tests to improve the early diagnostic efficacy of spinal tuberculosis can be applied for patients with spinal tuberculosis in underserved and remote areas.
Thirty-eight routine laboratory test variables and 2 other variables, including the age and sex of the patients, were included in this study. The variables selected are generally available at most hospitals to ensure practical application of the predictive model. Correlation analysis identified a high correlation between many of the variables, and this could be observed on the correlation heatmap. The problem of multicollinearity among the characteristics could not be resolved in the stepwise regression local optimum estimation of logistic regression (Bayman and Dexter, 2021). Therefore, we used Lasso regression, a regularization method widely used for model improvement and variable selection, for variable selection to optimize our model (Ternès et al., 2016). When multicollinearity existed in the original variables, Lasso regression could effectively screen the variables with multicollinearity (Fernández-Delgado et al., 2019). Using Lasso regression, we identified 12 variables that could be used for inclusion in the subsequent model.
Logistic regression was used to construct a predictive model for spinal tuberculosis. Twelve variables identified by Lasso regression were included in the logistic regression equation, and variables that were not significant in the logistic regression model were eliminated. Finally, seven variables were retained, including TB.antibody, IGRAs, RBC, Mono%, RDW, AST, and BUN were retained, and the model was refitted with these variables. Column line plots were drawn for better applicability to clinical practice. To evaluate the predictive model, we plotted calibration curves and ROC curves in a validation cohort different from the training cohort. The calibration curves showed a good fit, and the prediction model achieved an AUC value of 0.9188 in the validation cohort. The final model had good diagnostic validity. Based on our findings, increased levels of RBC, RDW, and mono% in patients could indicate a greater likelihood of STB diagnosis, whereas elevated BUN and AST levels may suggest a higher probability of NSTB diagnosis. In previously published studies, elevated RDW and mono% were commonly observed in patients with TB (Baynes et al., 1986). Patients with chronic TB infection often suffer from malnutrition, resulting in the development of anemia (Cobelens and Kerkhoff, 2021). However, in our study, the differential diagnosis of STB was often made in patients with other septic spinal infections and spinal tumors, who also had a poor nutritional status. This resulted in elevated levels of BUN and AST in both diseases, making it difficult to distinguish between them. Further studies are needed to better understand the distinction between these diseases.
In published studies, we noted that Liyi Chen et al. (2022) also attempted early diagnosis of spinal tuberculosis by drawing columnar maps, but they included fewer variables and lacked specific indicators for the diagnosis of spinal tuberculosis (IGRAs, TB antibody), and they did not develop strict inclusion and diagnostic criteria. A prospective study published in 2022 also diagnosed STB by combining multiple indicators, but we observed that this study included diagnostic indicators such as Xpert, acid-fast staining, and other indicators that occur late in the patient’s hospitalization (Qi et al., 2022); therefore, this study did not focus on the early diagnosis of STB. In addition, a recent study focused on a deep learning model for early diagnosis of spinal tuberculosis using imaging data analysis to determine the early diagnosis of spinal tuberculosis (Li et al., 2022), whereas our study focused on routine laboratory findings. In a follow-up study, we plan to combine both imaging data and routine laboratory findings to further optimize the early diagnosis prediction model of spinal tuberculosis through imaging histology and machine learning.
To enhance the generalizability of our model, we incorporated two distinct methods for IGRAs, namely QuantiFERON-TB Gold In-Tube and T-SPOT(®).TB, both of which share a similar underlying principle. Moreover, previous studies have highlighted the potential utility of the TBAg/PHA ratio as a means of improving diagnostic accuracy in tuberculosis (Wang et al., 2016; Luo et al., 2020), with particular promise demonstrated in the diagnosis of extrapulmonary disease (Wang et al., 2019). Although we were unable to include this variable in the current analysis, we eagerly anticipate the opportunity to assess the diagnostic efficacy of the TBAg/PHA ratio in T-SPOT(®).TB for spinal tuberculosis in a larger cohort of patients.
This study was a retrospective study, and due to missing data, we excluded some cases and other factors, such as calcitoninogen and body mass index, that might have been meaningful. We intend to conduct further prospective studies informed by our present results which will include complete clinical data and patient imaging data to further improve the early diagnosis of spinal tuberculosis.
5 Conclusion
In this study, we developed a nomogram to predict the occurrence of spinal tuberculosis for early diagnosis. Our model contained seven conventional laboratory indicators, including TB.antibody, IGRAs, RBC, Mono%, RDW, AST, and BUN. We hope to use this model to improve the early diagnosis of STB, especially in hospitals in poor and remote areas where testing is limited.
Data availability statement
The raw data supporting the conclusions of this article will be made available by the authors, without undue reservation.
Ethics statement
The studies involving human participants were reviewed and approved by the Ethics Committee of Xiangya Hospital Central South University. The patients/participants provided their written informed consent to participate in this study.
Author contributions
XH and QG designed research, performed research, analyzed data, and wrote the paper. HZ, MT and SL developed the idea for the study. GZ, BT and DX collected the data. All authors contributed to the article and approved the submitted version.
Funding
This work was supported by National Natural Science Foundation of China(serial number: 82072460, 82170901); Natural Science Foundation of Hunan Province(serial number: 2020JJ4892, 2020JJ4908)
Acknowledgments
I would like to thank all the surgeons in the Department of Spine Surgery at Xiangya Hospital of Central South University for their help and guidance.
Conflict of interest
The authors declare that the research was conducted in the absence of any commercial or financial relationships that could be construed as a potential conflict of interest.
Publisher’s note
All claims expressed in this article are solely those of the authors and do not necessarily represent those of their affiliated organizations, or those of the publisher, the editors and the reviewers. Any product that may be evaluated in this article, or claim that may be made by its manufacturer, is not guaranteed or endorsed by the publisher.
Supplementary material
The Supplementary Material for this article can be found online at: https://www.frontiersin.org/articles/10.3389/fcimb.2023.1150632/full#supplementary-material
References
Bayman, E. O., Dexter, F. (2021). Multicollinearity in logistic regression models. Anesth. Analgesia. 133 (2), 362–365. doi: 10.1213/ane.0000000000005593
Baynes, R. D., Flax, H., Bothwell, T. H., Bezwoda, W. R., Atkinson, P., Mendelow, B. (1986). Red blood cell distribution width in the anemia secondary to tuberculosis. Am. J. Clin. Pathol. 85 (2), 226–229. doi: 10.1093/ajcp/85.2.226
Beltran, C. G. G., Venter, R., Mann, T. N., Davis, J. H., Kana, B. D., Walzl, G. (2022). Culture filtrate supplementation can be used to improve mycobacterium tuberculosis culture positivity for spinal tuberculosis diagnosis. Front. Cell. Infection Microbiol. 12. doi: 10.3389/fcimb.2022.1065893
Chen, C. H., Chen, Y. M., Lee, C. W., Chang, Y. J., Cheng, C. Y., Hung, J. K. (2016). Early diagnosis of spinal tuberculosis. J. Formosan Med. Assoc. = Taiwan yi zhi. 115 (10), 825–836. doi: 10.1016/j.jfma.2016.07.001
Chen, L., Liu, C., Liang, T., Ye, Z., Huang, S., Chen, J., et al. (2022). Mechanism of COVID-19-Related proteins in spinal tuberculosis: Immune dysregulation. Front. Immunol. 13. doi: 10.3389/fimmu.2022.882651
Cobelens, F., Kerkhoff, A. D. (2021). Tuberculosis and anemia-cause or effect? Environ. Health Prev. Med. 26 (1), 93. doi: 10.1186/s12199-021-01013-4
Dunn, R. N., Ben Husien, M. (2018). Spinal tuberculosis: review of current management. Bone Joint J. 100-b (4), 425–431. doi: 10.1302/0301-620x.100b4.Bjj-2017-1040.R1
Fernández-Delgado, M., Sirsat, M. S., Cernadas, E., Alawadi, S., Barro, S., Febrero-Bande, M. (2019). An extensive experimental survey of regression methods. Neural Networks Off. J. Int. Neural Network Society. 111, 11–34. doi: 10.1016/j.neunet.2018.12.010
Hamada, Y., Cirillo, D. M., Matteelli, A., Penn-Nicholson, A., Rangaka, M. X., Ruhwald, M. (2021). Tests for tuberculosis infection: landscape analysis. Eur. Respir. J. 58 (5), 2100167. doi: 10.1183/13993003.00167-2021
Held, M., Laubscher, M., Zar, H. J., Dunn, R. N. (2014). GeneXpert polymerase chain reaction for spinal tuberculosis: an accurate and rapid diagnostic test. Bone Joint J. 96-b (10), 1366–1369. doi: 10.1302/0301-620x.96b10.34048
Hristea, A., Constantinescu, R. V., Exergian, F., Arama, V., Besleaga, M., Tanasescu, R. (2008). Paraplegia due to non-osseous spinal tuberculosis: report of three cases and review of the literature. Int. J. Infect. Dis. IJID Off. Publ. Int. Soc. Infect. Diseases. 12 (4), 425–429. doi: 10.1016/j.ijid.2007.12.004
Hu, X., Zhang, H., Li, Y., Zhang, G., Tang, B., Xu, D., et al. (2022). Analysis of the diagnostic efficacy of the QuantiFERON-TB gold in-tube assay for preoperative differential diagnosis of spinal tuberculosis. Front. Cell. Infection Microbiol. 12. doi: 10.3389/fcimb.2022.983579
Khanna, K., Sabharwal, S. (2019a). Spinal tuberculosis: A comprehensive review for the modern spine surgeon. Spine J. Off. J. North Am. Spine Society. 19 (11), 1858–1870. doi: 10.1016/j.spinee.2019.05.002
Khanna, K., Sabharwal, S. (2019b). Spinal tuberculosis: a comprehensive review for the modern spine surgeon. Spine J. 19 (11), 1858–1870. doi: 10.1016/j.spinee.2019.05.002
Li, Y., Dong, W., Lan, T., Fan, J., Qin, S., Guo, A. (2020). Distribution of linezolid in tuberculosis lesions in patients with spinal multidrug-resistant tuberculosis. Antimicrobial Agents Chemotherapy. 64 (7), e00450–20. doi: 10.1128/aac.00450-20
Li, Z., Wu, F., Hong, F., Gai, X., Cao, W., Zhang, Z., et al. (2022). Computer-aided diagnosis of spinal tuberculosis from CT images based on deep learning with multimodal feature fusion. Front. Microbiol. 13. doi: 10.3389/fmicb.2022.823324
Liu, H., Li, Y., Yi, J., Zhou, W., Zhao, S., Yin, G. (2022). Neutrophil-lymphocyte ratio as a potential marker for differential diagnosis between spinal tuberculosis and pyogenic spinal infection. J. Orthopaedic Surg. Res. 17 (1), 357. doi: 10.1186/s13018-022-03250-x
Liu, X., Zheng, M., Sun, J., Cui, X. (2021). A diagnostic model for differentiating tuberculous spondylitis from pyogenic spondylitis on computed tomography images. Eur. Radiology. 31 (10), 7626–7636. doi: 10.1007/s00330-021-07812-1
Luo, Y., Tang, G., Lin, Q., Mao, L., Xue, Y., Yuan, X., et al. (2020). Combination of mean spot sizes of ESAT-6 spot-forming cells and modified tuberculosis-specific antigen/phytohemagglutinin ratio of T-SPOT.TB assay in distinguishing between active tuberculosis and latent tuberculosis infection. J. Infect. 81 (1), 81–89. doi: 10.1016/j.jinf.2020.04.038
Mann, T. N., Davis, J. H., Walzl, G., Beltran, C. G., du Toit, J., Lamberts, R. P., et al. (2021). Candidate biomarkers to distinguish spinal tuberculosis from mechanical back pain in a tuberculosis endemic setting. Front. Immunol. 12. doi: 10.3389/fimmu.2021.768040
Pai, M., Behr, M., Dowdy, D., Dheda, K, Divangahi, M, Boehme, CC, et al. (2016). Tuberculosis. Nat. Rev. Dis. Primers 2, 16076. doi: 10.1038/nrdp.2016.76
Qi, Y., Liu, Z., Liu, X., Fang, Z., Liu, Y., Li, F. (2022). Tuberculosis-specific Antigen/Phytohemagglutinin ratio combined with GeneXpert MTB/RIF for early diagnosis of spinal tuberculosis: A prospective cohort study. Front. Cell. Infection Microbiol. 12. doi: 10.3389/fcimb.2022.781315
Rule, R., Mitton, B., Govender, N. P., Hoffmann, D., Said, M. (2021). Spinal epidural abscess caused by aspergillus spp masquerading as spinal tuberculosis in a person with HIV. Lancet Infect. Diseases. 21 (11), e356–ee62. doi: 10.1016/s1473-3099(20)30979-8
Scorrano, G., Viva, S., Pinotti, T., Fabbri, P. F., Rickards, O., Macciardi, F. (2022). Bioarchaeological and palaeogenomic portrait of two pompeians that died during the eruption of Vesuvius in 79 AD. Sci. Rep. 12 (1), 6468. doi: 10.1038/s41598-022-10899-1
Ternès, N., Rotolo, F., Michiels, S. (2016). Empirical extensions of the lasso penalty to reduce the false discovery rate in high-dimensional cox regression models. Stat Med. 35 (15), 2561–2573. doi: 10.1002/sim.6927
Wang, F., Hou, H. Y., Wu, S. J., Zhu, Q., Huang, M., Yin, B., et al. (2016). Using the TBAg/PHA ratio in the T-SPOT(®).TB assay to distinguish TB disease from LTBI in an endemic area. Int. J. Tuberc Lung Dis. 20 (4), 487–493. doi: 10.5588/ijtld.15.0756
Wang, T., Tan, Y. J., Wu, S. J., Huang, M., Yin, B. T., Huang, J., et al. (2019). [The ratio of tuberculosis-specific antigen to phytohemagglutinin in T-SPOT assay in the diagnosis of active tuberculosis]. Zhonghua Jie He He Hu Xi Za Zhi. 42 (4), 262–267. doi: 10.3760/cma.j.issn.1001-0939.2019.04.003
World Health Organization Global tuberculosis report 2021. Available at: https://www.who.int/news-room/fact-sheets/detail/tuberculosis (Accessed 13 December 2022).
Xu, Y., Wu, J., Liao, S., Sun, Z. (2017). Treating tuberculosis with high doses of anti-TB drugs: Mechanisms and outcomes. Ann. Clin. Microbiol. Antimicrobials. 16 (1), 67. doi: 10.1186/s12941-017-0239-4
Keywords: spinal tuberculosis, early diagnosis, nomogram, predictive model, conventional laboratory indices
Citation: Hu X, Zhang G, Zhang H, Tang M, Liu S, Tang B, Xu D, Zhang C and Gao Q (2023) A predictive model for early clinical diagnosis of spinal tuberculosis based on conventional laboratory indices: A multicenter real-world study. Front. Cell. Infect. Microbiol. 13:1150632. doi: 10.3389/fcimb.2023.1150632
Received: 24 January 2023; Accepted: 14 March 2023;
Published: 24 March 2023.
Edited by:
Amit Singh, Central University of Punjab, IndiaReviewed by:
Changwu Wu, Leipzig University, GermanyLiyan Mao, Huazhong University of Science and Technology, China
Anand Kumar Maurya, All India Institute of Medical Sciences, Bhopal, India
Copyright © 2023 Hu, Zhang, Zhang, Tang, Liu, Tang, Xu, Zhang and Gao. This is an open-access article distributed under the terms of the Creative Commons Attribution License (CC BY). The use, distribution or reproduction in other forums is permitted, provided the original author(s) and the copyright owner(s) are credited and that the original publication in this journal is cited, in accordance with accepted academic practice. No use, distribution or reproduction is permitted which does not comply with these terms.
*Correspondence: Qile Gao, gaoql@csu.edu.cn