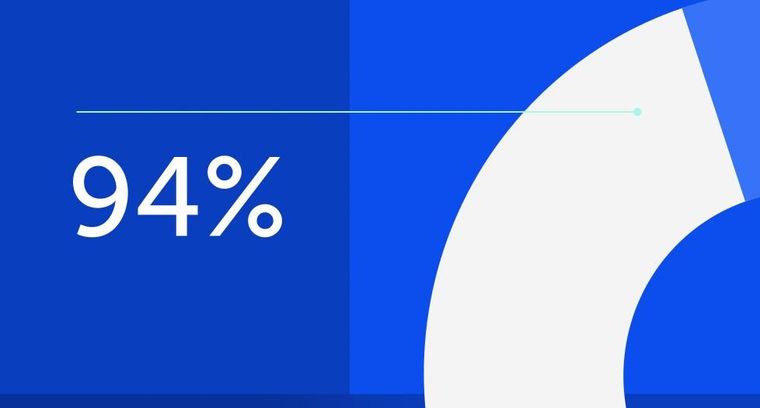
94% of researchers rate our articles as excellent or good
Learn more about the work of our research integrity team to safeguard the quality of each article we publish.
Find out more
ORIGINAL RESEARCH article
Front. Cell. Infect. Microbiol., 03 April 2023
Sec. Fungal Pathogenesis
Volume 13 - 2023 | https://doi.org/10.3389/fcimb.2023.1108235
This article is part of the Research TopicRising Stars in Fungal Pathogenesis: 2023View all 6 articles
Fungal infections are a major global health burden where Candida albicans is among the most common fungal pathogen in humans and is a common cause of invasive candidiasis. Fungal phenotypes, such as those related to morphology, proliferation and virulence are mainly driven by gene expression, which is primarily regulated by kinase signaling cascades. Serine-arginine (SR) protein kinases are highly conserved among eukaryotes and are involved in major transcriptional processes in human and S. cerevisiae. Candida albicans harbors two SR protein kinases, while Sky2 is important for metabolic adaptation, Sky1 has similar functions as in S. cerevisiae. To investigate the role of these SR kinases for the regulation of transcriptional responses in C. albicans, we performed RNA sequencing of sky1Δ and sky2Δ and integrated a comprehensive phosphoproteome dataset of these mutants. Using a Systems Biology approach, we study transcriptional regulation in the context of kinase signaling networks. Transcriptomic enrichment analysis indicates that pathways involved in the regulation of gene expression are downregulated and mitochondrial processes are upregulated in sky1Δ. In sky2Δ, primarily metabolic processes are affected, especially for arginine, and we observed that arginine-induced hyphae formation is impaired in sky2Δ. In addition, our analysis identifies several transcription factors as potential drivers of the transcriptional response. Among these, a core set is shared between both kinase knockouts, but it appears to regulate different subsets of target genes. To elucidate these diverse regulatory patterns, we created network modules by integrating the data of site-specific protein phosphorylation and gene expression with kinase-substrate predictions and protein-protein interactions. These integrated signaling modules reveal shared parts but also highlight specific patterns characteristic for each kinase. Interestingly, the modules contain many proteins involved in fungal morphogenesis and stress response. Accordingly, experimental phenotyping shows a higher resistance to Hygromycin B for sky1Δ. Thus, our study demonstrates that a combination of computational approaches with integration of experimental data can offer a new systems biological perspective on the complex network of signaling and transcription. With that, the investigation of the interface between signaling and transcriptional regulation in C. albicans provides a deeper insight into how cellular mechanisms can shape the phenotype.
Fungal infections constitute a major health burden worldwide with more than 150 million people suffering from serious fungal diseases. Candida albicans is one of the most common fungal pathogens of humans and is frequently found on the mucosal surfaces of the body. Invasive candidiasis poses a major threat with more than ~700,000 cases worldwide (Bongomin et al., 2017) and C. albicans is the most common cause of invasive candidiasis (Pfaller and Diekema, 2007) with very high mortality rates of 40 to 60% (Hsueh et al., 2009; Bassetti et al., 2019).
Gene expression plays a major role for the regulation of cellular processes shaping the phenotype by altering the morphology, growth and proliferation of the fungal cells which can lead to increased or decreased virulence or drug resistance of a fungal pathogen. Thus, detailed knowledge about the regulation of transcriptional processes is the key to understand how cellular functions shape the phenotype. Gene expression is one of the major downstream effectors of many signaling cascades, most of which are primarily built around central kinases which trigger cascading protein phosphorylations. These signaling cascades typically form complex networks, rather than linear pathways. Thus, to gain deeper insight into cellular functions, integrated methods of network analysis and large amount of data are required. This relates not only to the measurement of proteins, phosphorylation and transcripts levels, but also to information on how the components interact with each other.
Nowadays, high throughput technologies are becoming more available and affordable. In particular, mass spectrometry and Next Generation Sequencing (NGS) provide large scale profiles of the cellular transcriptome, proteome and phosphoproteome. Phosphoproteomics enables the characterization of proteins that are post-translationally modified by addition of a phosphate group and phosphopeptide enrichment methods can identify several thousands of phosphoproteins in one experiment (Zhou et al., 2010). To date, tens to hundreds of thousands of phosphosites of serine, threonine, or tyrosine residues have been identified in many organisms, including human (Ochoa et al., 2020), mouse (Huttlin et al., 2010; Qi et al., 2014) and yeast (Vlastaridis et al., 2017; Lanz et al., 2021). Several studies in fungi (Ramsubramaniam et al., 2014; Ren et al., 2016; Mattos et al., 2020) have exploited these technical possibilities to investigate the evolution of kinases and identify new substrates in different fungal species. most efforts focus on the study of established model organisms, while the characterization of the phosphoproteome of many fungi is still at an early stage. However, most efforts, focus on the study of established model organisms, while in many fungi, characterization of the phosphoproteome is still at an early stage. Nevertheless, it remains a major challenge to understand how this data translates into knowledge about the function of the cellular system. Cellular functions that shape fungal phenotypes are the product of a complex interplay between genes and proteins. Thus, to understand regulatory processes different cellular levels such as the phosphoproteome (delivering a view on signaling) and the transcriptome (capturing the effect of downstream gene expression) need to be measured in the same strain under the same conditions. Most important, but challenging, is the integration and subsequent analysis of the data in a common framework.
Serine-arginine (SR) protein kinases are highly conserved in eukaryotes (Giannakouros et al., 2011). They are characterized by phosphorylating SR proteins, which are enriched in serine/arginine recognition motifs at their N-terminus (Ghosh and Adams, 2011). In humans, SR proteins regulate major RNA related processes like mRNA export from the nucleus and both alternative and constitutive splicing (Zhou and Fu, 2013). In contrast, splicing plays only a minor role in the yeast S. cerevisiae but has been described to play a regulatory role during stress response in C. albicans (Sieber et al., 2018). However, it has been shown that the only SR kinase in S. cerevisiae, Sky1, phosphorylates the SR-like protein Npl3, which regulates pre-mRNA splicing and mRNA export from the nucleus (Moehle et al., 2012). In addition, Sky1 is important for polyamine transport and maintenance of ion homeostasis (Erez and Kahana, 2001; Forment et al., 2002). Recently, the function of SR kinases in C. albicans was described for the first time in human pathogenic fungi (Brandt et al., 2022). In this study, we performed a phylogenetic analysis which revealed that most common pathogenic Candida species possess two Sky kinases (Sky1 and Sky2), with the exception of C. glabrata, which contains only one SR protein kinase (Sky1 group)(Brandt et al., 2022). Furthermore, Sky1 and Sky2 have large sequence differences, indicating that these kinases have different functions in C. albicans. It has been shown that the SR-like protein kinase Sky1 in C. albicans appears to have a similar function as in S. cerevisiae (Brandt et al., 2022). In contrast, the SR-like protein kinase Sky2 is important for the metabolism of dipeptides and thus for the metabolic adaptation of the fungus (Brandt et al., 2022). Identifying the role of SR kinases in the human fungal pathogen Candida albicans could provide new insights into its virulence mechanisms.
Here, we set out to investigate the effects of SR-like kinase on transcriptional regulation in C. albicans and established integrated modules of Sky1 and Sky2 kinase signaling, focusing on downstream effects on gene expression. Therefore, we performed RNA-Seq to generate transcriptional profiles of sky1Δ and sky2Δ mutant strains and integrated data from a phosphoproteome study of these mutants, both comparing mutants with wild type (WT) controls. Subsequently, we performed pathway and transcription factor enrichment analysis to identify key components of transcriptional regulation. Next, we integrated computational predictions of kinases for the phosphoproteome data and further assembled information on protein-protein interactions to provide linking information between the signaling proteins and the transcription factors. Combining all data into a joint network we derived signaling modules for each mutant strain, which allow the analysis of transcriptomic and phosphoproteomic profiles within in this network context. This provides a detailed view on the interface between cellular signaling cascades and transcriptional regulation which offers the opportunity to investigate not only direct but also indirect effects of SR-like kinases in C. albicans. With that, we analyze specific and common functions of the two Sky kinases in a comparative manner, with focus on their influence on transcriptional regulation. We derive and discuss potential mechanisms and functional implications of various regulatory interactions. Here, we demonstrate how the combination of experimental data with network information can offer a broader perspective on C. albicans Sky kinases and can help to generate novel hypotheses about how signaling and transcription can shape the fungal phenotype.
The C. albicans strains used in this study are listed in Supplementary Table 1. All strains were stored as frozen stocks containing 20% glycerol at -80°C and sub-cultured on YPD agar plates (1% yeast extract, 2% peptone, 2% glucose, 2% agar) at 30°C for 2 days. Strains were routinely grown in YPD liquid medium at 30°C overnight with shaking at 180 rpm.
Sensitivity of the strains to the antibiotic Hygromycin B was tested by spot dilution assays on YPD agar plates. YPD overnight cultures of the wild type and mutant strains were centrifuged (4,000 × g, 5 min) and washed with dH2O. Strains were adjusted to an optical density of 600 nm (OD600) of 1.0. 5 µl of each 10-fold serial dilutions were spotted onto the YPD plates without or with Hygromycin B (250 µg/ml or 300 µg/ml) and incubated for 3 days at 37°C.
YPD overnight cultures of the wild type and mutant strains were centrifuged (4,000 × g, 5 min), washed with dH2O, adjusted to an OD600 of 0.5 and 5 µl of each cell suspension was spotted on solid arginine medium. The plates were incubated for 3 days at 37°C. Two different types of arginine media were used: 0.17% (w/v) yeast nitrogen base without ammonium sulfate and amino acids, 0.5% (w/v) ammonium sulfate, 1% (w/v) arginine (pH 6), and 0.17% (w/v) yeast nitrogen base without ammonium sulfate and amino acids, 10 mM Arginine (w/v), 0.2% glucose (w/v; pH 6).
YPD (1% yeast extract, 2% peptone, 2% glucose) overnight cultures of the C. albicans wild type as well as the sky1Δ and sky2Δ mutant strains were diluted in 50 ml fresh YPD medium with a starting OD600 of 0.3 and grown for 3 h at 37°C to logarithmic growth phase. Subsequently the strains were centrifuged (4000 × g, 5 min), washed with dH2O and were adjusted to an OD600 of 0.2 in 30 ml YPD medium and grown at 37°C for 4 h. Cells were collected by centrifugation and the cell pellets were shock frozen with liquid nitrogen. The cell pellets were resuspended in 400 µl AE buffer (50 mM sodium acetate, 10 mM EDTA) and transferred to screw cab tubes followed by adding 40 µl 10% SDS solution, 500 µl glass beads and 440 µl Phenol-Chloroform-Isoamylalkohol 125:24:1 (Ambion, acidic pH). Cells were mechanically disrupted on a FastPrep-24 cell-homogenizer (MP Biomedicals, Santa Ana, USA) with two 40 s runs at 6.0 m/s and with 5 min on ice incubation between each run. Samples were centrifuged at 12,000 g for 2 min and the upper phase was transferred into a new reaction tube and mixed with 1/10 volume 3 M sodium acetate (pH 5.3) and one volume 2-propanol. To precipitate the RNA, the samples were incubated for 30 min at -20°C and subsequently centrifuged for 10 min at 12,000 g. The pellets were washed twice with 100 µl 70% ethanol and resuspended in 100 µl RNase-free water. RNA quality and quantity were determined using the RNA Nano 6000 Assay Kit of the Bioanalyzer 2100 system (Agilent Technologies, CA, USA) and the NanoPhotometer® spectrophotometer (IMPLEN, CA, USA), respectively. RNA sequencing was performed by Novogene (Cambridge, United Kingdom) using the Illumina NovaSeq 6000 Sequencing System. The mRNA was enriched by a polyA capture followed by reverse transcription of cDNA to prepare an RNA library. Illumina PE150 technology was used for 150 bp paired-end sequencing.
After quality control of the libraries, all reads have been mapped against the C. albicans genome (Haplotype A chromosomes only, A22-s07-m01-r142 version, obtained from the Candida Genome Database (Skrzypek et al., 2017) with STAR (version 2.7.5b) (Dobin et al., 2013). Subsequently, aligned reads have been counted in a gene-wise manner using ‘featurecounts’ from the ‘Rsubread’ (version 2.0.1) package (Liao et al., 2014). Differential expression analysis has been performed based on generalized linear models comparing each mutant group with the control group as implemented in DESeq2 (version 1.26) (Love et al., 2014). Genes with an adjusted P-value < 0.05 (Benjamini and Hochberg, 1995) have been considered as significant and have been categorized as ‘low’ (absolute log2FC < 0.5) or ‘moderate to high’ (absolute log2FC ≥ 0.5) for further analyses. All transcriptomic analyses have been performed with the statistical software package R (version 3.6.3). Transcription factor enrichment was performed via the PathoYeastract+ website (Monteiro et al., 2020). Pathway analysis of at least moderately regulated genes were performed via the R-Package of g:Profiler (Kolberg et al., 2020).
A comprehensive repertoire of the C. albicans kinome has been compiled from literature (Lee et al., 2016) and was complemented by an automatic classification of kinases into superfamily, family and subfamily using Kinannote 1.0 (Goldberg et al., 2013). Phenotypic profiles for 143 C. albicans transcription factors were obtained from a published high-throughput knockout experiment (Homann et al., 2009). Furthermore, experimentally validated direct and indirect transcription regulatory associations of 127 transcription factors were extracted from PathoYeastract+ (Monteiro et al., 2020). Transcription regulatory genes from both sources were combined to obtain a comprehensive list of C. albicans transcription factors. Structure of the arginine pathway was obtained from KEGG (Okuda et al., 2008).
As basis for the construction of sky1Δ and sky2Δ modules, integrated C. albicans signaling networks were created combining kinase-substrate interaction (KSI) and protein-protein interaction data. Significant differentially phosphorylated kinases and transcription factors (absolute log2FC ≥ 1 and adjusted P-value < 0.05 obtained from phosphoproteomic datasets of sky1Δ and sky2Δ in YPD ((Brandt et al., 2022); PXD027612; Supplementary Figure 1A) were analyzed with the stand-alone batch predictor package of the Group-based Prediction System (GPS) (Wang et al., 2020). The high threshold option and the UniProt C. albicans reference proteome (UP000000559; downloaded August 2019) were used to predict site-specific phosphorylation for 617 different classifiers on the level of group, family and subfamily. To obtain kinase-specific information, the proteins of the C. albicans kinome were mapped to the corresponding classifiers, matching the kinase group, family and subfamily provided by the GPS prediction with that classified by Kinannote 1.0 (Goldberg et al., 2013). To remove low confidence predictions, only interaction with a GPS5 score greater than 80 were further used. As no information on experimentally validated KSI for C. albicans is available from databases so far, kinase-substrate interactions of S. cerevisiae obtained from major publicly available databases (dbPAF (Ullah et al., 2016), HPRD (Keshava Prasad et al., 2009), Phospho.Elm (Dinkel et al., 2011), PhosphoGRID (Oughtred et al., 2021), PhosphoSitePlus (Hornbeck et al., 2015), YeastKinome (Breitkreutz et al., 2010)) were transferred via the well-established interolog method as described in Remmele et al. (2015). Briefly, this method transfers interactions from template species S. cerevisiae to a target species (C. albicans) by linking two proteins in the target species by an interaction (A’ and B’) if the orthologous proteins in the template species are known to interact (A-B; Supplementary Figure 1B). For this, the orthology relation of proteins were extracted from the Ensembl Compara database (version 96) (Herrero et al., 2016) from the public ensemble MySql-Server (mysql-eg-publicsql.ebi.ac.uk:4157). Then the multiple sequence alignments of the respective Compara families were used to transfer the phosphorylation sites to orthologous proteins and sites in C. albicans. To derive a comprehensive protein-protein interaction network of C. albicans, experimentally validated protein-protein interactions of C. albicans were obtained from the 14 active partners of the International Molecular Exchange (IMEx) (Orchard et al., 2012) via PSICQUIC (searched on January, 2020) (Aranda et al., 2011). Additionally, all interactions were tested for support of domain-domain interactions. For that, the domains of the network interactors (A’, B’) were obtained from the UniProt website in January 2021 (UniProt, 2022). The information of known domain–domain interactions were downloaded from the database of three-interacting domains (3did) (Mosca et al., 2014) in January 2021 (Supplementary Figure 1B). This network was then filtered for interactions of differentially phosphorylated or expressed interactors which interact with kinases or transcription factors. From this filtered network we extracted the largest connected subnetwork for sky1Δ and sky2Δ (Supplementary Figure 1C).
To investigate the SKY1 and SKY2 knockout effects on gene regulation, we performed a genome wide transcription profiling of sky1Δ and sky2Δ mutant strains in comparison to the SC5314 wild type (WT) control (Figures 1A, B). The resulting expression profiles covered almost the entire C. albicans transcriptome with 99.2% (6,163/6,213) genes measured in at least one sample. Differential expression analysis yielded a total of 1,580 (25.4% of the entire transcriptome) differentially expressed genes (adjusted P-value < 0.05) for sky1Δ and 452 (7.3% of the entire transcriptome) differentially expressed genes for sky2Δ (adjusted P-value < 0.05). The analysis shows a largely symmetric effect on up- and downregulation for sky1Δ (51.4% up and 48.5% down) whereas in sky2Δ the majority of genes (63.9%) were downregulated (Figures 1A–C). Focusing on the set of genes with moderate to high expression changes (absolute log2FC ≥ 0.5 and adjusted P-Value < 0.05) the symmetric and asymmetric response patterns remain similar (Figures 1C). The relatively small number of differentially regulated genes shared between both strains points towards largely distinct effects of the kinases on transcriptional regulation (Figure 1D). The analysis of the directional effect of gene regulation reveals that most of the genes that are differentially expressed in both knockout strains, are regulated in the same direction, (Figure 1D) suggesting that Sky1 and Sky2 play a similar role in the regulation of these genes. Interestingly, we observed no transcriptional change of the other, intact, Sky kinase gene in both deletion strains compared to the wild type strain (expression of Sky2 in the sky1Δ mutant: log2FC = 0.001; adjusted P-value = 0.99 and expression of Sky1 in the sky2Δ strain: log2FC = 0.06; adjusted P-value = 0.74). This suggests that the deletion of one C. albicans Sky kinase does not lead to a compensatory transcriptional activation of the other Sky kinase gene.
Figure 1 Transcriptional profiles of sky1Δ and sky2Δ compared to wild type reveals distinct responses for each kinase mutant. Volcano plots comparing transcriptomic abundance log2FC changes (X-axis) and the adjusted P-values (Y-axis) for (A) sky1Δ and (B) sky2Δ versus wild type. RNA-Sequencing was performed after 4 hours growth of wild type, sky1Δ and sky2Δ in YPD medium. (C) Bar plot showing total number of differentially expressed (upper panel) and moderately to highly regulated genes (lower panel). Grouped bars represent upregulated (red), downregulated (blue) and total number of genes (grey) for sky1Δ and sky2Δ. (D) Venn diagrams of the total, downregulated and upregulated at least moderate differentially expressed genes (log2FC ≥0.5 and adjusted P-value < 0.05) of sky1Δ and sky2Δ.
The major key players in signaling and transcriptional regulation are kinases and transcription factors. In total, we found 71/282 (25.2%) transcription factors in sky1Δ to be differentially expressed (19 upregulated; 51 downregulated) whereas in sky2Δ 14 (5.0% of 282) transcription factors were differentially regulated (nine upregulated; five downregulated). Similar to the small overlap of all differentially regulated genes, only five transcription factors (EFH1, FLO8, SWC4, TUP1, ZSF1) were differentially expressed by both skyΔ mutant strains, among which only EFH1 show an expression log2FC change greater than 0.5 in both mutant strains (sky1Δ: 1.04; sky2Δ: 1.1). Since phosphorylations play a major role in the direct and indirect regulation of transcription factors we searched for differentially regulated kinases in sky1Δ and sky2Δ. Out of the 115 kinases in C. albicans were 29 kinases (25.2%) in sky1Δ and six kinases (5.2%) in sky2Δ differentially regulated compared to the wild type. Only two kinases (NIK1, HRR25) were differentially expressed in both knockout strains albeit only with a low expression change. This indicates, that both Sky kinases have only small similarities on transcriptional level regarding regulated kinases and transcription factors.
To functionally characterize the genome-wide transcriptional responses we performed pathway enrichment analyses based on the Gene Ontology (GO) categories, focusing again on genes with moderate to high effects. For sky1Δ this revealed an enrichment of mitochondrial respiratory associated categories like ‘mitochondrial respiratory chain complex assembly’, ‘mitochondrial intermembrane space’ and ‘organelle envelope lumen’ as well as RNA processing (‘U2 snRNP’) for upregulated genes (Figure 2A) and an enrichment of ‘regulation of gene expression’ and ‘nucleus localization’ for downregulated genes (Figure 2B). These processes are consistent with reported functions of Sky1 in S. cerevisiae where this kinase has been described to regulate various steps in RNA maturation and transport (Gilbert et al., 2001). In addition, a recent study has shown that the deletion of Sky1 in S. cerevisiae leads to mitochondrial defects (Hutchinson et al., 2022). In this light the significant upregulation of mitochondrial respiratory associated genes observed here indicates a similar mitochondrial involvement of Sky1 in C. albicans.
Figure 2 GO enrichment analysis reveals nuclear, mRNA processing and mitochondrial pathways for sky1Δ and glutamine and arginine metabolism pathways for sky2Δ. Bar plots show enriched biological processes (upper part; blue) and cellular components (lower part, brown) for (A) upregulated (moderate to high) as well as in (B) downregulated (moderate to high) genes. The X-axis represents the -log10 (adjusted P-value of enrichment) with the left side representing sky1Δ and the right side representing sky2Δ. For each GO category the total number of genes annotated in C albicans with this term is given in parentheses.
In contrast to that, we found an enrichment of metabolic and biosynthetic processes for arginine and glutamine for sky2Δ (Figure 2). For downregulated genes this relates primarily to catabolic processes (Figure 2B), whereas for upregulated genes primarily biosynthetic processes are enriched (Figure 2A) pointing towards a role of Sky2 in amino acid metabolism. Furthermore, the set of downregulated genes is primarily associated with the cell periphery (e.g. ‘hyphal cell wall’, ‘extracellular region’, ‘cell surface’, ‘cell periphery’). These results indicate that both kinases have different cellular functions in C. albicans. Similar to S. cerevisiae, Sky1 seems to be, mainly involved in mRNA processing as well as nuclear and mitochondrial related processes, whereas Sky2 seems plays a distinct role in the cell periphery and is involved in the transcriptional regulation of glutamine and arginine metabolism.
To further explore the role of Sky2 in amino acid sufficiency we focused on the differentially regulated genes in the glutamine and arginine metabolism pathways as provided by KEGG (Okuda et al., 2008). Interestingly, both skyΔ mutant strains showed a significant upregulation of the NADPH-dependent glutamate dehydrogenase (GDH3) which catalyzes the conversion of α-ketoglutarate and ammonium to glutamate. However, sky1Δ and sky2Δ mutants show different regulation of glutamate utilizing pathways like the biosynthetic and catabolic arginine pathway. So, sky2Δ upregulates most genes responsible for the conversion of glutamate to ornithine and further Arg4 which is the final step in the arginine biosynthesis pathway. This enzyme catalyzes the reversible breakdown of argininosuccinate to arginine and fumarate. Additionally, large parts of the arginine catabolic pathway with the initial conversion of arginine (Car1) to ornithine and further to proline, to glutamate (Put1, Put2) and to α-ketoglutarate as well as ammonium (Gdh2) are downregulated in sky2Δ. Since in this arginine catabolic pathway glutamate is used to fuel the TCA cycle (Silao et al., 2019), the sky2Δ strain seems to allocate glutamate for the generation of ornithine and arginine and instead of NADPH and ADP production. Sky1 has also been described in S. cerevisiae to be involved in the transport of polyamines and the maintenance of ion homeostasis (Erez and Kahana, 2001; Forment et al., 2002). Since ornithine is a precursor molecule for the polyamine production this could connect this intermediate of the arginine pathway with the known involvement of Sky1 orthologs in regulation of mRNA and polyamine transport. It has been shown that arginine and proline induce hyphae formation in C. albicans (Ghosh et al., 2009; Silao et al., 2019). As YPD contains arginine, we tested whether the sky2Δ mutant can filament on medium that contains arginine as the sole C- or N-source. We also included the wild-type strain SC5314, the sky1Δ mutant and the SKY1 and SKY2 complemented strains. On both media the wild type, sky1Δ mutant and the SKY1 complemented strain formed wrinkled colonies which indicate hyphae formation, whereas the sky2Δ mutant formed smooth colonies (Figure 3). The SKY2 complemented strain partially rescued the mutant phenotype on both media (Figure 3). A similar observation was made in our previous study (Brandt et al., 2022). As discussed there this indicates that one SKY2 allele is not sufficient to complement the phenotype of the wild-type strain (for detailed data see Supplementary Figure 3 in (Brandt et al., 2022)).
Figure 3 Arginine-induced wrinkle colony forming is impaired in the sky2Δ mutant. C. albicans strains were tested for arginine-induced filamentation on solid medium (0.17% YNB, 0.5% ammonium sulfate, 1% arginine [pH 6] and 0.17% YNB, 10 mM arginine, 0.2% glucose [pH 6]). The experiment was performed in duplicates and representative images were taken after 3 days of incubation at 37°C. The SKY2 complemented strain partially rescued the sky2Δ mutant phenotype. This was also shown in a previous study [(Brandt et al., 2022) for detailed data see Supplementary Figure 3 there].
To investigate the effects of sky1Δ and sky2Δ knockouts on protein phosphorylation in a network context we integrated previously published mass spectrometry phosphoproteome profiles of sky1Δ and sky2Δ mutants (Brandt et al., 2022). The experimental conditions (YPD medium; 4 h at 37°C) are concordant with that of our RNA sequencing experiment. The interpretation of phosphorylation events requires knowledge about the kinases potentially responsible for the phosphorylation of a specific protein. Data about such kinase-substrate relationships is sparse in databases, even for established model organisms and especially for C. albicans no experimental data seems to be available from databases so far. Therefore, we performed an in-silico analysis to predict candidate kinases for each regulated phosphorylation site in the phosphoproteome data using the motif-based approach GPS5 (Group based Prediction system) (Wang et al., 2020) with a high threshold setting to obtain only high confidence predictions (see Material and Methods and Supplementary Figure 1). With that we were able to assign a potential kinase to 55.4% of the sky1Δ and 33.8% of the sky2Δ proteins that were differentially phosphorylated. For S. cerevisiae several known kinase-substrate interactions have been experimentally identified and are available in databases. To further complement the set of motif-based predictions for sky1Δ and sky2Δ we transferred known kinase-substrate interactions of S. cerevisiae to C. albicans based on protein orthology information (see Materials and Methods). Apart from direct phosphorylation events, protein-protein interactions are an important part of signaling networks. Hence, we collected experimentally validated interactions of C. albicans from databases for the set of differentially expressed or phosphorylated proteins, focusing on interactions involving kinases and transcription factors. This yielded 85 interactions between 74 proteins for sky1Δ and 51 interactions between 50 proteins for sky2Δ.
Next, we intended to identify the transcription factors that are driving the observed transcriptional response. Those transcription factors may not be the direct targets of Sky1 and Sky2 and many of the phosphorylated transcription factors may be indirect phosphorylation targets acting downstream in the signaling cascade. Those transcription factors directly bind with the promoters of genes identified as the downstream targets in RNA-seq data. To this end, we performed a transcription factor enrichment analysis for the differentially regulated genes in sky1Δ and sky2Δ mutants with the PathoYeastract+ (Pathogenic Yeast Search for Transcriptional Regulators And Consensus Tracking) database for C. albicans (Monteiro et al., 2020), again focusing on genes with moderate to high expression change (absolute log2FC ≥ 0.5 and adjusted P-value < 0.05). In total, we detected a significant enrichment for 45 transcription factors in sky1Δ and 67 transcription factors in sky2Δ. To identify candidates with a potential impact on transcriptional response in sky1Δ and sky2Δ, we selected those transcription factors which are either differentially expressed or differentially phosphorylated compared to wild type. This revealed five transcription factors for sky1Δ (Ace2, Cup9, Efg1, Flo8, Wor1; Figure 4A) and eight transcription factors (Ace2, Efg1, Flo8, Mac1, Pho4, Sfl1, Sfl2, Zcf2) for sky2Δ (Figure 4B) which together regulate in sky1Δ the majority (68.7%; 314/457) and in sky2Δ almost all (95.2%; 139/146) genes with moderate to high expression changes. For sky1Δ two transcription factors were downregulated and one was upregulated in transcriptomics, whereas two showed an increased phosphorylation (Ace2: T241, S243, S565; Efg1: T391, T505). For sky2Δ four transcription factors were transcriptionally regulated with three downregulated and one upregulated while four (Efg1: S383; Ace2: S173, S233, S234; Sfl1: S641; Flo8: S575) showed decreased phosphorylation. This contrasts with sky1Δ, where all sites showed an increase in phosphorylation (Figure 4). There is evidence in literature that these candidate transcription factors directly or indirectly affect each other in C. albicans, either by transcriptional regulation of the corresponding genes (PathoYeastract+) (Monteiro et al., 2020) or by physical protein-protein interaction (IMEx) (Orchard et al., 2012). We compiled a detailed overview of the studies and experimental data supporting these interactions in Supplementary Table 2 and 3. With the integration of this information, regulatory modules of these transcription factors can be constructed as depicted in Figure 4. For example, Efg1, Flo8, Wor1 and Sfl2 have been described in literature to physically interact (Cao et al., 2006; Noffz et al., 2008; Alkafeef et al., 2018) and have been shown to play a role in hyphal morphogenesis (Stoldt et al., 1997; Cao et al., 2006; Zordan et al., 2007; Nobile et al., 2012; McCall et al., 2018). Here, the transcription factors Ace2, Efg1 and Flo8 are present in both modules as dominating players (based on the number of regulated genes and their location in the network context) For Example, Efg1 directly regulates Ace2, Flo8, Sfl2, Wor1 and Cup9 (Nobile et al., 2012; Hernday et al., 2013), while Flo8 has been shown to directly bind to the promotors of Efg1, Mac1, Cup9 and Pho4 (Znaidi et al., 2013; Fox et al., 2015; Polvi et al., 2019). Based on this data, this set of shared transcription factors has the potential to regulate most of the other enriched transcription factors (Monteiro et al., 2020) (Figure 4). Therefore, these transcription factors may be the leading drivers behind the observed transcriptional response.
Figure 4 Regulation and protein-protein interaction modules of enriched transcription factors point towards a few core regulators that are differentially phosphorylated or expressed in sky1Δ and sky2Δ. (A) Modules for sky1Δ and (B) sky2Δ enriched transcription factors (rectangles and phosphorylation sites (small circles) that are connected by simple edges representing a known direct regulation of the node, whereas double edges represent a known physical protein-protein interaction (PPI). Circle fill color intensity shows the strength of differential regulation or phosphorylation, where blue color show decreased, red increased and white no significant regulation (wild type vs skyΔ; P-value <0.05). Transcription factors are annotated with the relative frequency (percent) of regulated targets of the total significantly regulated genes.
Although these transcription factors together appear to drive large parts of the transcriptional response in both mutant strains the differentially regulated genes targeted by these transcription factors are quite different in sky1Δ and sky2Δ (Figure 5A). This indicates that in the two knockout strains the functionality of these three transcription factors might be modulated by other factors, so that they target different genes. There are several factors by which transcription factors could be influenced; e.g. by post-translational modifications or interacting proteins (Spitz and Furlong, 2012).
Figure 5 Core set of transcription factors shared by sky1Δ and sky2Δ show distinct subsets of regulated genes in sky1Δ and sky2Δ and exhibit different phosphorylation patterns and interactors. (A) Venn diagrams for shared transcription factors represents the number of moderately expressed genes that were identified as regulation targets by transcription factor enrichment analysis. (B) Transcription factor network of sky1Δ (left) and sky2Δ (right) with transcription factors (rectangles), potential effector proteins (circles) and phosphorylation sites (small circles) connected by double edges representing a known physical protein-protein interaction. Circle fill color intensity shows the strength of differential regulation or phosphorylation, where blue color shows decreased, red increased and white no significant regulation (wild type vs skyΔ, P-value <0.05).
The combination of transcriptomic and phosphoproteomic data with literature-derived information on protein-protein and regulatory interactions allows the investigation of individual proteins and phosphorylation events in the context of their interaction environment. With that it is possible to derive novel hypotheses about transcription factors and kinases and their cellular functions. Thus, to identify potential activity regulating factors in sky1Δ and sky2Δ, we explored the network around these core transcription factors focusing on protein interactors and phosphorylation events (Figure 5B). Ace2, Efg1 and Flo8 were differentially phosphorylated in both knockout strains although at different sites and in different directions. Furthermore, the network context provides evidence for several direct protein-protein interactions in C. albicans for Ace2 (with Cst20, She3, orf19.3626)(van Wijlick et al., 2016), Flo8 (with Mfg1, Efg1) (Cao et al., 2006; Ryan et al., 2012) and Efg1 (with Flo8, Czf1, Wor1) (Petrovska and Kumamoto, 2012) (see Figure 5B; Supplementary Table 3). As these interactors were differentially expressed in transcriptomics and/or differentially phosphorylated in the knockout strains phosphoproteomics, they are candidates to act as cofactors to fine-tune the target specificity of the transcription factors.
For example, it has been shown that the binding of Efg1 to its DNA target sequence is influenced by phosphorylation and by interactions with other transcription factors (Lassak et al., 2011). In the network modules (Figure 5B) we observe that the Efg1 interaction partner Czf1 (Giusani et al., 2002; Noffz et al., 2008; Petrovska and Kumamoto, 2012; Frazer et al., 2020) is more abundantly phosphorylated in sky2Δ and, in addition, Efg1 is differentially phosphorylated in sky1Δ and sky2Δ. Interestingly, it has been reported that the binding of Czf1 to Efg1 may antagonize it function to change filamentous growth in C. albicans (Giusani et al., 2002; Frazer et al., 2020). Here we observe that Czf1 is transcriptionally downregulated in the sky2Δ mutant, which might indicate that this antagonistic effect could be weakened in this knockout strain (Petrovska and Kumamoto, 2012). Furthermore, Flo8 is less abundantly phosphorylated in sky2Δ at site S575. Albeit this phosphorylation has not been described before, it has been reported, that phosphorylation of the closely located site T589 represses the activity of Flo8 and that dephosphorylation of this site releases that repression (Hollomon et al., 2022). It was reported that Efg1 and Flo8 cooperatively bind to a subset of key targets involved in morphogenesis (Ryan et al., 2012). Furthermore, Mfg1, which is less abundantly phosphorylated in sky2Δ but not in sky1Δ, has been shown interact directly with Flo8 in C. albicans (Ryan et al., 2012).
A central transcription factor in these modules is Ace2 which is not only differentially phosphorylated in both kinase knockouts, but also on different sites and in opposite direction (sky1Δ: T241, S243, S565; sky2Δ: S173, S233, S234; Figure 5B). These sites are all located in a set of disordered regions between the nuclear export signal and the zinc finger domains (Wakade and Krysan, 2021) with currently unknown effect on the protein. The Ace2 sites S565 and S234 are conserved in S. cerevisiae (T486; S259) where they have been shown to be phosphorylated by the yeast Cyclin-dependent kinase 1 (CDC28) (Holt et al., 2009), suggesting a conserved functional role for these phosphorylation sites. In C. albicans it has been shown that the phosphorylation of Ace2 by Cbk1 plays a role for hyphal morphogenesis and is required for hyphal formation under hypoxic conditions (Wakade et al., 2020). Furthermore, several relevant morphogenetic regulators (Efg1, Flo8, Wor1) in both modules have been shown to be directly regulated by Ace2 (Desai et al., 2015).
Altogether, this indicates, that the transcription factors Ace2, Efg1 and Flo8, are central players in the transcriptional response of both kinases but different regulation of these proteins by phosphorylation or interactions might alter their functionality. In addition all three transcription factors have been reported to be involved in several processes comprising a broader range of cell morphology (Kelly et al., 2004; Cao et al., 2006; Glazier, 2022) in C. albicans, supporting the notion that the changes induced by the knockout of SKY1 and SKY2 modulate parts of pathways that maintain hyphal development and morphology in different ways.
The observed transcriptional responses are regulated by transcription factors which are the ultimate effectors of the Sky kinases. Network analysis of phosphoproteome profiles can identify direct and indirect kinase substrates (such as transcription factors). These are not necessarily direct substrates of the Sky kinases but might rather be phosphorylated by other kinases acting downstream in the signaling cascades. To investigate the regulation of the transcriptional responses and elucidate the effects of Sky kinases knockouts, we constructed integrated network modules for both kinase mutants (Supplementary Figure 1). The sky1Δ signaling module (Figure 6A) consists of 51 site-specific direct phosphorylations and 18 experimentally validated protein-protein interactions between 44 proteins. Consistent with the overall direction of phosphorylation change in the sky1Δ phosphoproteomic analysis, 89.4% of targeted phosphorylation sites (42/47) display increased phosphorylation. The signaling backbone of this module consists of 22 kinases and 15 transcription factors. Around this core module we found seven additional proteins with experimentally validated interactions in C. albicans which could potentially play a modulating role in the signaling cascade (Figure 6A). The sky2Δ module (Figure 6B) contains 16 kinases and 14 transcription factors where most of the 35 phosphorylation sites (31 sites; 88.6%) show a decreased phosphorylation in the mutant (absolute log2FC> 1 and adjusted P-Value < 0.05). Both networks are dominated by a few kinases (orf19.4518, Rim11, Hog1; Figures 6A, B-a), with orf19.4518 and Rim11 shared by sky1Δ and sky2Δ, and Hog1 present only in the sky2Δ module. Except for Hog1, not much is known for these kinases, but all three have been associated with cellular responses to osmotic stress and cell morphology in C. albicans. For example, Hog1 has been described to be involved in osmotic, heavy metal, and core stress response and has a role in regulation of response to stress in C. albicans (Smith et al., 2004; Enjalbert et al., 2006; Alonso-Monge et al., 2009). In addition, Rim11 orthologs in S. cerevisiae are known to be involved in oxidative stress resistance, resistance to chemicals and filamentous growth (Frohlich et al., 2015; Helsen et al., 2020). Notably, one of the targets of the central kinase orf19.4518 is the site S81 of Hrk1 (Figures 6A, B-b) which shows a particularly high decrease (64 times) of phosphorylation in both Sky knockout strains. Hrk1 is a putative serine/threonine kinase with a predicted role in cellular ion homeostasis which has previously been described to have the same knockout phenotype as sky1Δ (Brandt et al., 2022). Based on this, it was suggested that Hrk1 is either directly phosphorylated by Sky1 or is an indirect target acting in the same pathway. Here, the proposed integrated modules indicate that Hrk1 is rather an indirect target of Sky1 and Sky2 which might instead be directly phosphorylated by the kinase orf19.4518.
Figure 6 Integrated signaling modules of sky1Δ and sky2Δ. Network modules of (A) sky1Δ and (B) sky2Δ with kinases (octagons) and transcription factors (rectangles), phosphorylation sites (small circles) and first shell of potential effector proteins (large circles). Border color shows strongest phosphorylation change with significantly decreased (blue) and significantly increased phosphorylation (red; wild type vs skyΔ, P-value <0.05). Big node fill color intensity shows the strength of differential transcriptional regulation, where blue color shows down regulation, red upregulation and white no significant regulation (wild type vs skyΔ, P-value <0.05). Small node (phosphorylation site) fill color represents the strength of differential phosphorylation, with blue color showing decreased, red increased and white no significant regulation (wild type vs skyΔ, P-value <0.05). Kinase-substrate interactions (KSI) are represented by small doted (motif based only) and long doted (conserved only) edges with arrow. KSI supported by domain-domain interactions are shown by a continuous edge while experimentally described interaction are represented by double edges.
Four transcription factors which have been detected in the transcription factor enrichment analysis (Figures 4, 5) as candidate drivers of the sky1Δ and sky2Δ transcriptional response are also present in the signaling network module. There, Efg1, Flo8 and Wor1 form an interaction complex (Figures 6A, B-c, Supplementary Table 2) (Cao et al., 2006; Noffz et al., 2008; Alkafeef et al., 2018). In addition Ace2 is integrated in the modules (Figures 6A, B-d) as part of the Regulation of Ace2 and Morphogenesis (RAM) pathway (Wakade and Krysan, 2021). Interestingly, these core transcription factors seem to be targeted by orf19.4518, Rim11 and Hog1 in different ways with phosphorylation effects in mostly opposite directions in sky1Δ and sky2Δ. Based on motif-based kinase substrate analyses, only Efg1 is phosphorylated by Rim11 in the sky1Δ module, whereas three transcription factors (Czf1: S297; Efg1: S383; Flo8: S575) are targeted by the kinases Hog1 and orf19.4518 in the in sky2Δ module. This indicates a differential adjustment of Rim11, orf19.4518 and Hog1 in sky1Δ compared to sky2Δ potentially leading to distinct downstream effects on the transcriptional responses.
For Ace2, the network modules depict the phosphorylation of the sites S565 (log2FC = 1.5 and adjusted P-value < 0.05) in sky1Δ and S234 (log2FC = -1.9 and adjusted P-value < 0.05) in sky2Δ which are both conserved in S. cerevisiae and are targeted there by the kinase Cdc28 (Figures 6A, B-f) (Koivomagi et al., 2011; Faustova et al., 2021). In addition to Ace2, our signaling modules integrate further interactors of the RAM pathway (Hym1, Sog2, Kic1, Cas4, Mob2, Cbk1, Fkh2, Bcr1; Figures 6A, B-e, 7B) (Wakade and Krysan, 2021). Several interactors of this pathway are present in both modules but with different changes in sky1Δ and sky2Δ. Some components (Kic1, Fkh2, Cas4) are transcriptionally downregulated in sky1Δ but none of the genes are transcriptionally regulated in sky2Δ. On the protein level a decreased phosphorylation of Kic1, Mob2 can be observed in sky1Δ (compared to the wild type), in contrast to an increased phosphorylation in sky2Δ (Figure 7B). Another phosphorylation target of Cdc28 in both modules is Mob2 site S97 (supported by motif-based kinase substrate analysis and domain domain-interaction). Phosphorylation of this site has previously been shown to be crucial for normal hyphal development on hyphae-inducing conditions in C. albicans (Gutierrez-Escribano et al., 2011). However, in contrast to the sky2Δ module, the sky1Δ module also contains three other potential substrates targeted by Cdc28 namely Swi6, Gin4 and orf19.3751 (Figure 6; supported by motif-based kinase substrate analysis and domain domain-interaction). Swi6, together with Swi4, is a core component of the conserved (S. cerevisiae) (Bahler, 2005) cell cycle box-binding factor (SBF) complex (Hussein et al., 2011) (Figures 6A–G), which plays an important role in cell proliferation, G1/S progression and may be linked to aspects of hyphal development. Furthermore, the phosphorylation of Swi6 site S209 by Cdc28 is also conserved in S. cerevisiae. Furthermore, in addition to Swi6, Swi4 is differentially phosphorylated only in sky1Δ (log2FC = 2.7 and adjusted P-value < 0.05) but not in sky2Δ, suggesting that sky1Δ and sky2Δ differentially fine-tune Cdc28 kinase activity to regulate the RAM pathway and SBF complex. Since Swi4 and Swi6, similar to Ace2, influence morphogenesis including hyphae differentiation in C. albicans (Hussein et al., 2011) this could explain the opposing roles of Sky1 and Sky2 in these cell processes through direct or indirect regulation of Cdc28 activity. In line with this, an analysis of the network modules in the context of knockout phenotypes (based on literature and databases)(Skrzypek et al., 2017) revealed that a large number of genes in the modules of sky1Δ and sky2Δ are associated with cell morphological functional categories. For example, 65.9% (29/44) in the sky1Δ and 40.0% (30/75) of the proteins in the sky2Δ module are connected to filamentous growth. Additionally, 72% of the sky1Δ proteins are associated with resistance to chemicals whereas only 30% of the sky2Δ proteins were associated with this knockout phenotype.
Figure 7 Deletion of SKY1 confers resistance to Hygromycin B and both Sky knockouts strains show distinct changes of the RAM-Pathway in transcriptomics and phosphoproteomics. (A) YPD overnight cultures of the strains were adjusted to an optical density (OD600) of 1.0 and 5 µl of serial 10-fold dilutions and were spotted on YPD agar plates without or with 250 µg/ml or 300 µg/ml Hygromycin B and incubated for 3 days at 37°C. The experiment was performed in duplicates and representative images are shown. (B) Overview of the components of the RAM pathway of sky1Δ (left) and sky2Δ (right) with kinases (octagons) and transcription factors (rectangles), phosphorylation sites (small circles) and first shell of potential effector proteins (large circles). Big node fill color intensity shows the strength of differential transcriptional regulation, where blue color shows down regulation, red upregulation and grey no significant regulation (wild type vs skyΔ, P-value <0.05). Small node fill color represents the strength of differential phosphorylation, with blue color showing decreased, red increased and grey no significant regulation (wild type vs skyΔ, P-value <0.05).
To test whether sky1Δ and sky2Δ differentially influence the function of the RAM pathway, we tested the susceptibility of both mutants for Hygromycin B. This aminoglycoside antibiotic inhibits the protein synthesis and translation of both prokaryotic and eukaryotic cells and it has been shown that knockout of genes of the RAM pathway (CBK1, HYM1, KIC1, MOB2, PAG1, SOG2) induces hypersensitivity (Song et al., 2008). The sky1Δ mutant was less susceptible to Hygromycin B compared to the wild type and the sky2Δ mutant (Figure 7A). As the sky1Δ signaling module demonstrates that parts of the RAM pathway are differentially phosphorylated and regulated in the sky1Δ mutant (Figure 7B), this might explain the observed phenotype on Hygromycin B.
Here we investigated the different functions of the C. albicans Sky1 and Sky2 kinases regarding transcriptional regulation, kinase signaling and phenotype within an integrated network context. Our analyses revealed, that sky1Δ and sky2Δ show distinct transcriptional responses and share only a small number of regulated genes. These seems to be regulated by a common set of core transcription factors that have the potential to regulate large but Sky kinase-specific subsets of target genes. However, these core transcription factors and their adjacent protein interactors show mostly opposite or different phosphorylation and expression status, indicating potential mechanisms to fine tune the regulation of their target genes. Additionally, transcriptomic enrichment in sky1Δ reveals functional categories similar to the known role of Sky1 orthologs in S. cerevisiae namely pre-mRNA splicing, mRNA export from the nucleus and mitochondrial respiratory chain association, further supportingthe proposed conserved role of this kinase in C. albicans. In contrast, Sky2 seems to have a different role as suggested by enrichment of genes in the cell periphery and glutamine family amino acid metabolic process, supporting the prior observed role in nitrogen compound metabolic processes. In particular, sky2Δ shows an upregulation of several proteins of the arginine biosynthesis pathways while a large part of the arginine catabolic pathway is downregulated. Experimental validation of these observations revealed that arginine-induced hyphae formation is impaired in the sky2Δ mutant. Furthermore, transcription factor enrichment and integrated analysis of kinase signaling networks point towards several key components in the SKY1 and SKY2 knockout response which are connected to fungal morphology, resistance to chemicals and stress for both C. albicans Sky kinases. Further experimental phenotyping delivered evidence that sky1Δ results in higher resistance to Hygromycin B confirming these observations. Altogether, our results consolidate the hypotheses that in C. albicans the Sky1 protein kinase is functionally similar to Sky1 in S. cerevisiae, whereas C. albicans Sky2 seems to have distinct functions regarding gene expression and signaling. These might be controlled by transcriptional and posttranslational regulation of key transcription factors and effector proteins. Our data and network offer a systems biological perspective of candida phosphorylation and thus can help to generate novel hypotheses and obtain deeper insights into mechanisms of complex signaling cascades and transcriptional regulation.
The data presented in the study are deposited in the Sequence Read Archive (SRA) at NCBI (https://www.ncbi.nlm.nih.gov/sra) under the accession number PRJNA941841.
CL, MD, TM analyzed the data. PB performed the wet lab experiments. CL, PB designed the figures. MD, TD, TM, SV discussed the results. MD, SV, TD supervised the project. CL, MD, PB wrote the manuscript in consultation with SV, TD, TM. All authors contributed to the article and approved the submitted version.
This study was funded by the German Research Foundation (DFG) through the TRR 124 FungiNet, “Pathogenic fungi and their human host: Networks of Interaction,” DFG project number 210879364, project C2 (to SV) and B2 (to TD and MD).
We thank Sara Giddins for her native speaker and language corrections.
The authors declare that the research was conducted in the absence of any commercial or financial relationships that could be construed as a potential conflict of interest.
All claims expressed in this article are solely those of the authors and do not necessarily represent those of their affiliated organizations, or those of the publisher, the editors and the reviewers. Any product that may be evaluated in this article, or claim that may be made by its manufacturer, is not guaranteed or endorsed by the publisher.
The Supplementary Material for this article can be found online at: https://www.frontiersin.org/articles/10.3389/fcimb.2023.1108235/full#supplementary-material
Alkafeef, S. S., Yu, C., Huang, L., Liu, H. (2018). Wor1 establishes opaque cell fate through inhibition of the general co-repressor Tup1 in candida albicans. PloS Genet. 14 (1), e1007176. doi: 10.1371/journal.pgen.1007176
Alonso-Monge, R., Carvaihlo, S., Nombela, C., Rial, E., Pla, J. (2009). The Hog1 MAP kinase controls respiratory metabolism in the fungal pathogen candida albicans. Microbiol. (Reading) 155 (Pt 2), 413–423. doi: 10.1099/mic.0.023309-0
Aranda, B., Blankenburg, H., Kerrien, S., Brinkman, F. S., Ceol, A., Chautard, E., et al. (2011). PSICQUIC and PSISCORE: accessing and scoring molecular interactions. Nat. Methods 8 (7), 528–529. doi: 10.1038/nmeth.1637
Bahler, J. (2005). Cell-cycle control of gene expression in budding and fission yeast. Annu. Rev. Genet. 39, 69–94. doi: 10.1146/annurev.genet.39.110304.095808
Bassetti, M., Giacobbe, D. R., Vena, A., Trucchi, C., Ansaldi, F., Antonelli, M., et al. (2019). Incidence and outcome of invasive candidiasis in intensive care units (ICUs) in Europe: results of the EUCANDICU project. Crit. Care 23 (1), 219. doi: 10.1186/s13054-019-2497-3
Benjamini, Y., Hochberg, Y. (1995). Controlling the false discovery rate - a practical and powerful approach to multiple testing. J. R. Stat. Soc. Ser. B-Statistical Method. 57 (1), 289–300. doi: 10.1111/j.2517-6161.1995.tb02031.x
Bongomin, F., Gago, S., Oladele, R. O., Denning, D. W. (2017). Global and multi-national prevalence of fungal diseases-estimate precision. J. Fungi (Basel) 3 (4). doi: 10.3390/jof3040057
Brandt, P., Gerwien, F., Wagner, L., Kruger, T., Ramirez-Zavala, B., Mirhakkak, M. H., et al. (2022). Candida albicans SR-like protein kinases regulate different cellular processes: Sky1 is involved in control of ion homeostasis, while Sky2 is important for dipeptide utilization. Front. Cell Infect. Microbiol. 12. doi: 10.3389/fcimb.2022.850531
Breitkreutz, A., Choi, H., Sharom, J. R., Boucher, L., Neduva, V., Larsen, B., et al. (2010). A global protein kinase and phosphatase interaction network in yeast. Science 328 (5981), 1043–1046. doi: 10.1126/science.1176495
Cao, F., Lane, S., Raniga, P. P., Lu, Y., Zhou, Z., Ramon, K., et al. (2006). The Flo8 transcription factor is essential for hyphal development and virulence in candida albicans. Mol. Biol. Cell 17 (1), 295–307. doi: 10.1091/mbc.e05-06-0502
Desai, P. R., van Wijlick, L., Kurtz, D., Juchimiuk, M., Ernst, J. F. (2015). Hypoxia and temperature regulated morphogenesis in candida albicans. PloS Genet. 11 (8), e1005447. doi: 10.1371/journal.pgen.1005447
Dinkel, H., Chica, C., Via, A., Gould, C. M., Jensen, L. J., Gibson, T. J., et al. (2011). Phospho.ELM: a database of phosphorylation sites–update 2011. Nucleic Acids Res. 39 (Database issue), D261–D267. doi: 10.1093/nar/gkq1104
Dobin, A., Davis, C. A., Schlesinger, F., Drenkow, J., Zaleski, C., Jha, S., et al. (2013). STAR: ultrafast universal RNA-seq aligner. Bioinformatics 29 (1), 15–21. doi: 10.1093/bioinformatics/bts635
Enjalbert, B., Smith, D. A., Cornell, M. J., Alam, I., Nicholls, S., Brown, A. J., et al. (2006). Role of the Hog1 stress-activated protein kinase in the global transcriptional response to stress in the fungal pathogen candida albicans. Mol. Biol. Cell 17 (2), 1018–1032. doi: 10.1091/mbc.e05-06-0501
Erez, O., Kahana, C. (2001). Screening for modulators of spermine tolerance identifies Sky1, the SR protein kinase of saccharomyces cerevisiae, as a regulator of polyamine transport and ion homeostasis. Mol. Cell Biol. 21 (1), 175–184. doi: 10.1128/MCB.21.1.175-184.2001
Faustova, I., Moll, K., Valk, E., Loog, M., Ord, M. (2021). Docking to a basic helix promotes specific phosphorylation by G1-Cdk1. Int. J. Mol. Sci. 22 (17). doi: 10.3390/ijms22179514
Forment, J., Mulet, J. M., Vicente, O., Serrano, R. (2002). The yeast SR protein kinase Sky1p modulates salt tolerance, membrane potential and the Trk1,2 potassium transporter. Biochim. Biophys. Acta 1565 (1), 36–40. doi: 10.1016/s0005-2736(02)00503-5
Fox, E. P., Bui, C. K., Nett, J. E., Hartooni, N., Mui, M. C., Andes, D. R., et al. (2015). An expanded regulatory network temporally controls candida albicans biofilm formation. Mol. Microbiol. 96 (6), 1226–1239. doi: 10.1111/mmi.13002
Frazer, C., Staples, M. I., Kim, Y., Hirakawa, M., Dowell, M. A., Johnson, N. V., et al. (2020). Epigenetic cell fate in candida albicans is controlled by transcription factor condensates acting at super-enhancer-like elements. Nat. Microbiol. 5 (11), 1374–1389. doi: 10.1038/s41564-020-0760-7
Frohlich, F., Petit, C., Kory, N., Christiano, R., Hannibal-Bach, H. K., Graham, M., et al. (2015). The GARP complex is required for cellular sphingolipid homeostasis. Elife 4. doi: 10.7554/eLife.08712
Ghosh, G., Adams, J. A. (2011). Phosphorylation mechanism and structure of serine-arginine protein kinases. FEBS J. 278 (4), 587–597. doi: 10.1111/j.1742-4658.2010.07992.x
Ghosh, S., Navarathna, D. H., Roberts, D. D., Cooper, J. T., Atkin, A. L., Petro, T. M., et al. (2009). Arginine-induced germ tube formation in candida albicans is essential for escape from murine macrophage line RAW 264.7. Infect. Immun. 77 (4), 1596–1605. doi: 10.1128/IAI.01452-08
Giannakouros, T., Nikolakaki, E., Mylonis, I., Georgatsou, E. (2011). Serine-arginine protein kinases: a small protein kinase family with a large cellular presence. FEBS J. 278 (4), 570–586. doi: 10.1111/j.1742-4658.2010.07987.x
Gilbert, W., Siebel, C. W., Guthrie, C. (2001). Phosphorylation by Sky1p promotes Npl3p shuttling and mRNA dissociation. RNA 7 (2), 302–313. doi: 10.1017/s1355838201002369
Giusani, A. D., Vinces, M., Kumamoto, C. A. (2002). Invasive filamentous growth of candida albicans is promoted by Czf1p-dependent relief of Efg1p-mediated repression. Genetics 160 (4), 1749–1753. doi: 10.1093/genetics/160.4.1749
Glazier, V. E. (2022). EFG1, everyone's favorite gene in candida albicans: A comprehensive literature review. Front. Cell Infect. Microbiol. 12. doi: 10.3389/fcimb.2022.855229
Goldberg, J. M., Griggs, A. D., Smith, J. L., Haas, B. J., Wortman, J. R., Zeng, Q. (2013). Kinannote, a computer program to identify and classify members of the eukaryotic protein kinase superfamily. Bioinformatics 29 (19), 2387–2394. doi: 10.1093/bioinformatics/btt419
Gutierrez-Escribano, P., Gonzalez-Novo, A., Suarez, M. B., Li, C. R., Wang, Y., de Aldana, C. R., et al. (2011). CDK-dependent phosphorylation of Mob2 is essential for hyphal development in candida albicans. Mol. Biol. Cell 22 (14), 2458–2469. doi: 10.1091/mbc.E11-03-0205
Helsen, J., Voordeckers, K., Vanderwaeren, L., Santermans, T., Tsontaki, M., Verstrepen, K. J., et al. (2020). Gene loss predictably drives evolutionary adaptation. Mol. Biol. Evol. 37 (10), 2989–3002. doi: 10.1093/molbev/msaa172
Hernday, A. D., Lohse, M. B., Fordyce, P. M., Nobile, C. J., DeRisi, J. L., Johnson, A. D. (2013). Structure of the transcriptional network controlling white-opaque switching in candida albicans. Mol. Microbiol. 90 (1), 22–35. doi: 10.1111/mmi.12329
Herrero, J., Muffato, M., Beal, K., Fitzgerald, S., Gordon, L., Pignatelli, M., et al. (2016). Ensembl comparative genomics resources. Database (Oxford) 2016. doi: 10.1093/database/baw053
Hollomon, J. M., Liu, Z., Rusin, S. F., Jenkins, N. P., Smith, A. K., Koeppen, K., et al. (2022). The candida albicans Cdk8-dependent phosphoproteome reveals repression of hyphal growth through a Flo8-dependent pathway. PloS Genet. 18 (1), e1009622. doi: 10.1371/journal.pgen.1009622
Holt, L. J., Tuch, B. B., Villen, J., Johnson, A. D., Gygi, S. P., Morgan, D. O. (2009). Global analysis of Cdk1 substrate phosphorylation sites provides insights into evolution. Science 325 (5948), 1682–1686. doi: 10.1126/science.1172867
Homann, O. R., Dea, J., Noble, S. M., Johnson, A. D. (2009). A phenotypic profile of the candida albicans regulatory network. PloS Genet. 5 (12), e1000783. doi: 10.1371/journal.pgen.1000783
Hornbeck, P. V., Zhang, B., Murray, B., Kornhauser, J. M., Latham, V., Skrzypek, E. (2015). PhosphoSitePlus 2014: mutations, PTMs and recalibrations. Nucleic Acids Res. 43 (Database issue), D512–D520. doi: 10.1093/nar/gku1267
Hsueh, P. R., Graybill, J. R., Playford, E. G., Watcharananan, S. P., Oh, M. D., Ja'alam, K., et al. (2009). Consensus statement on the management of invasive candidiasis in intensive care units in the Asia-pacific region. Int. J. Antimicrob. Agents 34 (3), 205–209. doi: 10.1016/j.ijantimicag.2009.03.014
Hussein, B., Huang, H., Glory, A., Osmani, A., Kaminskyj, S., Nantel, A., et al. (2011). G1/S transcription factor orthologues Swi4p and Swi6p are important but not essential for cell proliferation and influence hyphal development in the fungal pathogen candida albicans. Eukaryot Cell 10 (3), 384–397. doi: 10.1128/EC.00278-10
Hutchinson, K. M., Hunn, J. C., Reines, D. (2022). Nab3 nuclear granule accumulation is driven by respiratory capacity. Curr. Genet. 68 (5-6), 581–591. doi: 10.1007/s00294-022-01248-w
Huttlin, E. L., Jedrychowski, M. P., Elias, J. E., Goswami, T., Rad, R., Beausoleil, S. A., et al. (2010). A tissue-specific atlas of mouse protein phosphorylation and expression. Cell 143 (7), 1174–1189. doi: 10.1016/j.cell.2010.12.001
Kelly, M. T., MacCallum, D. M., Clancy, S. D., Odds, F. C., Brown, A. J., Butler, G. (2004). The candida albicans CaACE2 gene affects morphogenesis, adherence and virulence. Mol. Microbiol. 53 (3), 969–983. doi: 10.1111/j.1365-2958.2004.04185.x
Keshava Prasad, T. S., Goel, R., Kandasamy, K., Keerthikumar, S., Kumar, S., Mathivanan, S., et al. (2009). Human protein reference database–2009 update. Nucleic Acids Res. 37 (Database issue), D767–D772. doi: 10.1093/nar/gkn892
Koivomagi, M., Valk, E., Venta, R., Iofik, A., Lepiku, M., Morgan, D. O., et al. (2011). Dynamics of Cdk1 substrate specificity during the cell cycle. Mol. Cell 42 (5), 610–623. doi: 10.1016/j.molcel.2011.05.016
Kolberg, L., Raudvere, U., Kuzmin, I., Vilo, J., Peterson, H. (2020). gprofiler2 – an r package for gene list functional enrichment analysis and namespace conversion toolset g:Profiler. F1000Res 9, 709. doi: 10.12688/f1000research.24956.2
Lanz, M. C., Yugandhar, K., Gupta, S., Sanford, E. J., Faca, V. M., Vega, S., et al. (2021). In-depth and 3-dimensional exploration of the budding yeast phosphoproteome. EMBO Rep. 22 (2), e51121. doi: 10.15252/embr.202051121
Lassak, T., Schneider, E., Bussmann, M., Kurtz, D., Manak, J. R., Srikantha, T., et al. (2011). Target specificity of the candida albicans Efg1 regulator. Mol. Microbiol. 82 (3), 602–618. doi: 10.1111/j.1365-2958.2011.07837.x
Lee, K. T., So, Y. S., Yang, D. H., Jung, K. W., Choi, J., Lee, D. G., et al. (2016). Systematic functional analysis of kinases in the fungal pathogen cryptococcus neoformans. Nat. Commun. 7, 12766. doi: 10.1038/ncomms12766
Liao, Y., Smyth, G. K., Shi, W. (2014). featureCounts: an efficient general purpose program for assigning sequence reads to genomic features. Bioinformatics 30 (7), 923–930. doi: 10.1093/bioinformatics/btt656
Love, M. I., Huber, W., Anders, S. (2014). Moderated estimation of fold change and dispersion for RNA-seq data with DESeq2. Genome Biol. 15 (12), 550. doi: 10.1186/s13059-014-0550-8
Mattos, E. C., Silva, L. P., Valero, C., de Castro, P. A., Dos Reis, T. F., Ribeiro, L. F. C., et al. (2020). The aspergillus fumigatus phosphoproteome reveals roles of high-osmolarity glycerol mitogen-activated protein kinases in promoting cell wall damage and caspofungin tolerance. mBio 11 (1), e02962–02919. doi: 10.1128/mBio.02962-19
McCall, A. D., Kumar, R., Edgerton, M. (2018). Candida albicans Sfl1/Sfl2 regulatory network drives the formation of pathogenic microcolonies. PloS Pathog. 14 (9), e1007316. doi: 10.1371/journal.ppat.1007316
Moehle, E. A., Ryan, C. J., Krogan, N. J., Kress, T. L., Guthrie, C. (2012). The yeast SR-like protein Npl3 links chromatin modification to mRNA processing. PloS Genet. 8 (11), e1003101. doi: 10.1371/journal.pgen.1003101
Monteiro, P. T., Oliveira, J., Pais, P., Antunes, M., Palma, M., Cavalheiro, M., et al. (2020). YEASTRACT+: a portal for cross-species comparative genomics of transcription regulation in yeasts. Nucleic Acids Res. 48 (D1), D642–D649. doi: 10.1093/nar/gkz859
Mosca, R., Ceol, A., Stein, A., Olivella, R., Aloy, P. (2014). 3did: a catalog of domain-based interactions of known three-dimensional structure. Nucleic Acids Res. 42 (D1), D374–D379. doi: 10.1093/nar/gkt887
Nobile, C. J., Fox, E. P., Nett, J. E., Sorrells, T. R., Mitrovich, Q. M., Hernday, A. D., et al. (2012). A recently evolved transcriptional network controls biofilm development in candida albicans. Cell 148 (1-2), 126–138. doi: 10.1016/j.cell.2011.10.048
Noffz, C. S., Liedschulte, V., Lengeler, K., Ernst, J. F. (2008). Functional mapping of the candida albicans Efg1 regulator. Eukaryot Cell 7 (5), 881–893. doi: 10.1128/EC.00033-08
Ochoa, D., Jarnuczak, A. F., Vieitez, C., Gehre, M., Soucheray, M., Mateus, A., et al. (2020). The functional landscape of the human phosphoproteome. Nat. Biotechnol. 38 (3), 365–373. doi: 10.1038/s41587-019-0344-3
Okuda, S., Yamada, T., Hamajima, M., Itoh, M., Katayama, T., Bork, P., et al. (2008). KEGG atlas mapping for global analysis of metabolic pathways. Nucleic Acids Res. 36 (Web Server issue), W423–W426. doi: 10.1093/nar/gkn282
Orchard, S., Kerrien, S., Abbani, S., Aranda, B., Bhate, J., Bidwell, S., et al. (2012). Protein interaction data curation: the international molecular exchange (IMEx) consortium. Nat. Methods 9 (4), 345–350. doi: 10.1038/nmeth.1931
Oughtred, R., Rust, J., Chang, C., Breitkreutz, B. J., Stark, C., Willems, A., et al. (2021). The BioGRID database: A comprehensive biomedical resource of curated protein, genetic, and chemical interactions. Protein Sci. 30 (1), 187–200. doi: 10.1002/pro.3978
Petrovska, I., Kumamoto, C. A. (2012). Functional importance of the DNA binding activity of candida albicans Czf1p. PloS One 7 (6), e39624. doi: 10.1371/journal.pone.0039624
Pfaller, M. A., Diekema, D. J. (2007). Epidemiology of invasive candidiasis: a persistent public health problem. Clin. Microbiol. Rev. 20 (1), 133–163. doi: 10.1128/CMR.00029-06
Polvi, E. J., Veri, A. O., Liu, Z., Hossain, S., Hyde, S., Kim, S. H., et al. (2019). Functional divergence of a global regulatory complex governing fungal filamentation. PloS Genet. 15 (1), e1007901. doi: 10.1371/journal.pgen.1007901
Qi, L., Liu, Z., Wang, J., Cui, Y., Guo, Y., Zhou, T., et al. (2014). Systematic analysis of the phosphoproteome and kinase-substrate networks in the mouse testis. Mol. Cell Proteomics 13 (12), 3626–3638. doi: 10.1074/mcp.M114.039073
Ramsubramaniam, N., Harris, S. D., Marten, M. R. (2014). The phosphoproteome of aspergillus nidulans reveals functional association with cellular processes involved in morphology and secretion. Proteomics 14 (21-22), 2454–2459. doi: 10.1002/pmic.201400063
Remmele, C. W., Luther, C. H., Balkenhol, J., Dandekar, T., Muller, T., Dittrich, M. T. (2015). Integrated inference and evaluation of host-fungi interaction networks. Front. Microbiol. 6. doi: 10.3389/fmicb.2015.00764
Ren, S., Yang, M., Li, Y., Zhang, F., Chen, Z., Zhang, J., et al. (2016). Global phosphoproteomic analysis reveals the involvement of phosphorylation in aflatoxins biosynthesis in the pathogenic fungus aspergillus flavus. Sci. Rep. 6, 34078. doi: 10.1038/srep34078
Ryan, O., Shapiro, R. S., Kurat, C. F., Mayhew, D., Baryshnikova, A., Chin, B., et al. (2012). Global gene deletion analysis exploring yeast filamentous growth. Science 337 (6100), 1353–1356. doi: 10.1126/science.1224339
Sieber, P., Voigt, K., Kammer, P., Brunke, S., Schuster, S., Linde, J. (2018). Comparative study on alternative splicing in human fungal pathogens suggests its involvement during host invasion. Front. Microbiol. 9. doi: 10.3389/fmicb.2018.02313
Silao, F. G. S., Ward, M., Ryman, K., Wallstrom, A., Brindefalk, B., Udekwu, K., et al. (2019). Mitochondrial proline catabolism activates Ras1/cAMP/PKA-induced filamentation in candida albicans. PloS Genet. 15 (2), e1007976. doi: 10.1371/journal.pgen.1007976
Skrzypek, M. S., Binkley, J., Binkley, G., Miyasato, S. R., Simison, M., Sherlock, G. (2017). The candida genome database (CGD): incorporation of assembly 22, systematic identifiers and visualization of high throughput sequencing data. Nucleic Acids Res. 45 (D1), D592–D596. doi: 10.1093/nar/gkw924
Smith, D. A., Nicholls, S., Morgan, B. A., Brown, A. J., Quinn, J. (2004). A conserved stress-activated protein kinase regulates a core stress response in the human pathogen candida albicans. Mol. Biol. Cell 15 (9), 4179–4190. doi: 10.1091/mbc.e04-03-0181
Song, Y., Cheon, S. A., Lee, K. E., Lee, S. Y., Lee, B. K., Oh, D. B., et al. (2008). Role of the RAM network in cell polarity and hyphal morphogenesis in candida albicans. Mol. Biol. Cell 19 (12), 5456–5477. doi: 10.1091/mbc.E08-03-0272
Spitz, F., Furlong, E. E. (2012). Transcription factors: from enhancer binding to developmental control. Nat. Rev. Genet. 13 (9), 613–626. doi: 10.1038/nrg3207
Stoldt, V. R., Sonneborn, A., Leuker, C. E., Ernst, J. F. (1997). Efg1p, an essential regulator of morphogenesis of the human pathogen candida albicans, is a member of a conserved class of bHLH proteins regulating morphogenetic processes in fungi. EMBO J. 16 (8), 1982–1991. doi: 10.1093/emboj/16.8.1982
Ullah, S., Lin, S., Xu, Y., Deng, W., Ma, L., Zhang, Y., et al. (2016). dbPAF: an integrative database of protein phosphorylation in animals and fungi. Sci. Rep. 6, 23534. doi: 10.1038/srep23534
UniProt, C. (2022). UniProt: the universal protein knowledgebase in 2023. Nucleic Acids Res. 51 (D1), D523–D531. doi: 10.1093/nar/gkac1052
van Wijlick, L., Swidergall, M., Brandt, P., Ernst, J. F. (2016). Candida albicans responds to glycostructure damage by Ace2-mediated feedback regulation of Cek1 signaling. Mol. Microbiol. 102 (5), 827–849. doi: 10.1111/mmi.13494
Vlastaridis, P., Papakyriakou, A., Chaliotis, A., Stratikos, E., Oliver, S. G., Amoutzias, G. D. (2017). The pivotal role of protein phosphorylation in the control of yeast central metabolism. G3 (Bethesda) 7 (4), 1239–1249. doi: 10.1534/g3.116.037218
Wakade, R. S., Krysan, D. J. (2021). The Cbk1-Ace2 axis guides candida albicans from yeast to hyphae and back again. Curr. Genet. 67 (3), 461–469. doi: 10.1007/s00294-020-01152-1
Wakade, R. S., Ristow, L. C., Stamnes, M. A., Kumar, A., Krysan, D. J. (2020). The Ndr/LATS kinase Cbk1 regulates a specific subset of Ace2 functions and suppresses the hypha-to-Yeast transition in candida albicans. mBio 11 (4), e01900–01920. doi: 10.1128/mBio.01900-20
Wang, C., Xu, H., Lin, S., Deng, W., Zhou, J., Zhang, Y., et al. (2020). GPS 5.0: An update on the prediction of kinase-specific phosphorylation sites in proteins. Genomics Proteomics Bioinf. 18 (1), 72–80. doi: 10.1016/j.gpb.2020.01.001
Zhou, H., Albuquerque, C. P., Liang, J., Suhandynata, R. T., Weng, S. (2010). Quantitative phosphoproteomics: New technologies and applications in the DNA damage response. Cell Cycle 9 (17), 3479–3484. doi: 10.4161/cc.9.17.13152
Zhou, Z., Fu, X. D. (2013). Regulation of splicing by SR proteins and SR protein-specific kinases. Chromosoma 122 (3), 191–207. doi: 10.1007/s00412-013-0407-z
Znaidi, S., Nesseir, A., Chauvel, M., Rossignol, T., d'Enfert, C. (2013). A comprehensive functional portrait of two heat shock factor-type transcriptional regulators involved in candida albicans morphogenesis and virulence. PloS Pathog. 9 (8), e1003519. doi: 10.1371/journal.ppat.1003519
Keywords: sky kinases, kinase signaling, network analysis, Candida albicans, transcriptome, transcriptional regulation, phosphoproteome
Citation: Luther CH, Brandt P, Vylkova S, Dandekar T, Müller T and Dittrich M (2023) Integrated analysis of SR-like protein kinases Sky1 and Sky2 links signaling networks with transcriptional regulation in Candida albicans. Front. Cell. Infect. Microbiol. 13:1108235. doi: 10.3389/fcimb.2023.1108235
Received: 25 November 2022; Accepted: 01 March 2023;
Published: 03 April 2023.
Edited by:
James Kronstad, University of British Columbia, CanadaReviewed by:
Teresa OMeara, University of Michigan, United StatesCopyright © 2023 Luther, Brandt, Vylkova, Dandekar, Müller and Dittrich. This is an open-access article distributed under the terms of the Creative Commons Attribution License (CC BY). The use, distribution or reproduction in other forums is permitted, provided the original author(s) and the copyright owner(s) are credited and that the original publication in this journal is cited, in accordance with accepted academic practice. No use, distribution or reproduction is permitted which does not comply with these terms.
*Correspondence: Marcus Dittrich, bWFyY3VzLmRpdHRyaWNoQGJpb3plbnRydW0udW5pLXd1ZXJ6YnVyZy5kZQ==
Disclaimer: All claims expressed in this article are solely those of the authors and do not necessarily represent those of their affiliated organizations, or those of the publisher, the editors and the reviewers. Any product that may be evaluated in this article or claim that may be made by its manufacturer is not guaranteed or endorsed by the publisher.
Research integrity at Frontiers
Learn more about the work of our research integrity team to safeguard the quality of each article we publish.