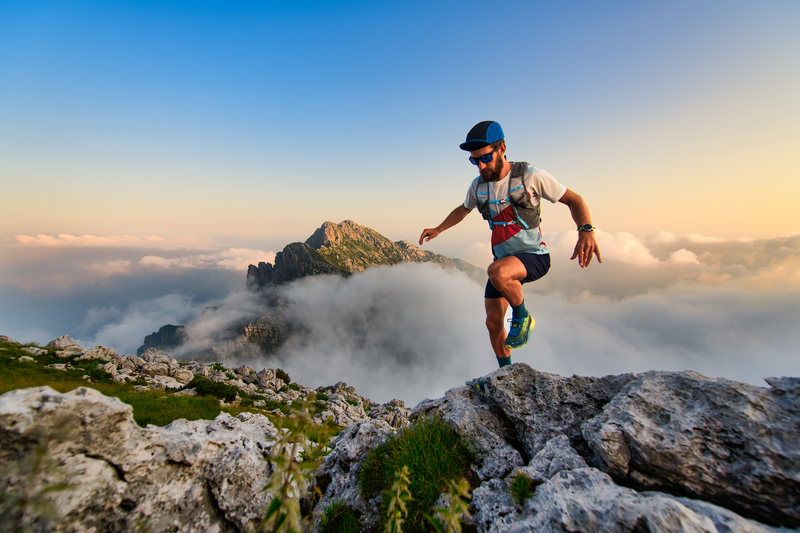
94% of researchers rate our articles as excellent or good
Learn more about the work of our research integrity team to safeguard the quality of each article we publish.
Find out more
REVIEW article
Front. Cell. Infect. Microbiol. , 10 February 2023
Sec. Clinical Microbiology
Volume 13 - 2023 | https://doi.org/10.3389/fcimb.2023.1108155
This article is part of the Research Topic Finding Potential Approaches for Tuberculosis Prevention and Treatment View all 9 articles
While early and precise diagnosis is the key to eliminating tuberculosis (TB), conventional methods using culture conversion or sputum smear microscopy have failed to meet demand. This is especially true in high-epidemic developing countries and during pandemic-associated social restrictions. Suboptimal biomarkers have restricted the improvement of TB management and eradication strategies. Therefore, the research and development of new affordable and accessible methods are required. Following the emergence of many high-throughput quantification TB studies, immunomics has the advantages of directly targeting responsive immune molecules and significantly simplifying workloads. In particular, immune profiling has been demonstrated to be a versatile tool that potentially unlocks many options for application in TB management. Herein, we review the current approaches for TB control with regard to the potentials and limitations of immunomics. Multiple directions are also proposed to hopefully unleash immunomics’ potential in TB research, not least in revealing representative immune biomarkers to correctly diagnose TB. The immune profiles of patients can be valuable covariates for model-informed precision dosing-based treatment monitoring, prediction of outcome, and the optimal dose prediction of anti-TB drugs.
Accounting for approximately 1.4 million fatal cases in 2021, tuberculosis (TB) is the 13th leading cause of death and the second most common life-threatening infection after coronavirus disease 2019 (COVID-19) (WHO, 2022). Knowing no place or age makes TB a significant threat to public health, especially in overpopulated or developing countries. Commonalities of 30 high-TB-burden countries and three global TB-watchlist countries (WHO, 2022) include the pressures of either overwhelming medical demand or financial instability. Therefore, a reliable, fast, and affordable medical approach to this curable and preventable disease is the key to controlling the TB epidemic.
The WHO’s End TB Strategy encompasses three pillars centralizing the patients, encouraging government-communities cooperation, and intensifying innovative research to eventually hit the goals of an 80% drop in TB incidence, a 90% drop in TB mortality, and catastrophic cost elimination for TB-affected households by 2030 in comparison to 2015. Reaching these milestones would have required a constant incidence decline of 4-5% per year by 2020 and a further drop of 10% by 2025 (WHO, 2022). However, the reality of current records fails to keep track of the End TB Strategy’s targets. Meanwhile, TB-related mortality reduction between 2015 and 2020 was only a quarter of the projected 2020 milestone of 32%. TB deaths increased in 2020, the first such occurrence for nine straight years. This resulted from the restriction of medical access during the COVID-19 pandemic. In particular, nearly half of TB cases were not diagnosed and treated (WHO, 2021b); undetected TB rose, which, with some lag-time, eventually caused an increase in TB death, community transmission, and the number of people developing TB (WHO, 2022). Hence, regarding the fact of our failure to reach the WHO’s target and the dominance of TB in developing countries, it is a must to optimize the existing tools and studies for the next generation to be predictable, fast, and affordable in giving access to all affected countries and populations, and helping to return to the expected trajectory of the global TB epidemic.
Culture conversion or sputum smear microscopy is still widely used regardless of their limitations for diagnosing and monitoring treatment responses in adults with pulmonary TB (PTB) (Yong et al., 2019; Zimmer et al., 2022). These methods require sputum samples, which are not promptly accessible from all populations, have long turnaround times, and are prone to contamination with poor specificity and sensitivity for outcome prediction (Yong et al., 2019; Zimmer et al., 2022). Furthermore, some patients face a risk of infection recurrence or have a higher risk of acquiring multi- or extensive drug-resistant TB (M/XDR-TB), especially those from low-income countries. Additionally, challenges regarding the accuracy of sputum-based diagnostics are often raised in cases of paucibacillary related to human immunodeficiency virus (HIV) and childhood TB (Newton et al., 2008; Cattamanchi et al., 2009). Hence, the WHO has endorsed several non-sputum-based TB diagnoses by utilizing the urine or blood of TB patients through nucleic acid amplification tests and loop-mediated isothermal amplification (LAMP) reaction, which have appeared as promising point-of-care (POC) tests for TB (Organization, 2020). Additionally, the tuberculin skin test (TST) and interferon-gamma release assays (IGRAs) have been widely utilized for latent tuberculosis infection (LTBI) detection in clinical practice (Zellweger et al., 2020), but neither of these effectively distinguish LTBI from active TB (Sharma et al., 2017). Even though IGRAs offer superior specificity, they are more expensive and associated with organizational and logistical challenges (Zellweger et al., 2020). Therefore, there is an urgent need to develop a novel, low-cost, non-sputum-based TB diagnostic test that is sensitive and specific, and employs readily available biological materials such as blood or urine (Goletti et al., 2016; Goletti et al., 2018).
The polymerase chain reaction (PCR)-based technique, namely GeneXpert MTB/RIF, recently developed to amplify the Mtb gene, represents a significant advancement in diagnosing TB (Zeka et al., 2011; Kwak et al., 2013) and detecting rifampicin resistance (Organization, 2020) within two hours. Sensitivity and specificity of PTB detection up to 79,5% and 100%, respectively, were reported by Kwak’s group when employing GeneXpert MTB/RIF assay in clinical practice (Kwak et al., 2013). Nevertheless, the utilization of the test limits the diagnosis of active PTB, not LTBI. Moreover, both PCR-based and sputum-based diagnostic systems exhibit inherent limitations in that they rarely detect extrapulmonary (EP)-TB in an efficient manner (Lee and Diseases, 2015). Therefore, an approach focused on the host biomarkers involved in host immune responses and pathological processes of LTBI, active TB, and EP-TB could be a better method for diagnosis and treatment monitoring.
As a predictable ending readout for various biological studies such as genomics, transcriptomics, proteomics, and metabolomics, omics sciences aim to quantify and demonstrate the biological profiles related to the structure, function, status, and dynamics of a cell, tissue, or organism (Vailati-Riboni et al., 2017). Immunomics is the study of immune responses after the interface of pathogen-derived proteins and the host immune system, focusing on multiple aspects of the immune molecules, such as their presence, variation, target, and function at a certain stage of a health condition (Doolan et al., 2014; De Sousa and Doolan, 2016; Yan et al., 2018). Immune profiling has provided valuable complementary information for genomics, transcriptomics, and proteomics (Figure 1). This advanced combination has provided an unprecedented opportunity to develop a rational immunomic approach to target antigen selections such as Plasmodium (Doolan, 2011; ENPICOM, 2020), COVID-19, and TB, which are all significant public health problems and pose a threat to complex genetic and immunological adaptations (De Sousa and Doolan, 2016; Yan et al., 2018).
Figure 1 The natural course of TB and potential points for biomarker-guided interventions. A better understanding of disease progression, accruable diagnosis, appropriate monitoring, and possible therapeutic interventions can be acquired by integrating demographic and conventional clinical data with high-throughput omics data. TB, tuberculosis.
TB has remained in the community (Barberis et al., 2017) due to the lack of highly effective biomarkers of the host which has restricted the improvement of diagnoses and treatment strategies. Several immune responses have been reported as a part of natural defense mechanisms against TB, such as interferon-γ (IFN-γ) production, CD4+ T cell or polyfunctional T cell responses, interactive immunogenic secretion, and regulation. Therefore, the evaluation of host immune responses by immune profiling, including cytokines, chemokines, the proteins associated with responses to inflammation or proteins released during TB evasion, tissue damage, and treatment prognosis, will shed light on TB diagnosis and the clinical management of individual patients (Walzl et al., 2011).
Moreover, besides being a supportive information channel, immune response profiling claims certain advantages compared to current gene-expression profiling and its precedent counterparts such as ELISA (Enzyme-Linked Immunosorbent Assay). By directly targeting expressive proteins, which are immune molecules, the approach bypasses the concern of relatively low transcriptional correlation (less than 0.3 among 1,066 genes in 23 human cell lines) (Gry et al., 2009) in genomic and transcriptomic studies. The unavoidable presence of fluctuated metabolites during translation and post-modification is another setback that immunomics scientists can generally ignore. Finally, by using advanced technology, high-throughput immune profiling requires much lower blood volume than DNA isolation does in genomic assay or ELISA (approximately 50 μL and hundreds μL, respectively) (Elshal and McCoy, 2006). It also shortens the running time significantly from days to hours, the turnaround time for laboratory diagnosis, and reduces the number of running plates several times compared to ELISA, all of which end up reducing the workload for blood collection and other clinical practices. For those reasons, high-throughput immune profiling can offer enormous potential as either a sufficient reference for TB management or an additional input for better and more precise patient stratification.
In this article, we summarize the current discovered immune markers and immune-centric approaches for TB investigation in the omics era. Furthermore, we propose a novel TB-management strategy through semi-automated therapeutic drug monitoring (TDM) utilizing model-informed precision dosing (MIPD).
In clinical care for TB, the demand for biomarkers is most critical for certain investigations, including the prediction of durable cure in active disease cases, disclosing reactivation risk, monitoring treatment, predicting outcome for latent Mtb-infected individuals, and vaccine efficacy prediction in case of patients suffering from other active diseases (Wallis et al., 2010). Furthermore, biomarkers can benefit candidate determination during drug discovery, accelerating dosage selection in preliminary clinical research and enriching the knowledge of TB pathogenesis (Wallis et al., 2010).
Infection and the host immune system have an intimate relationship as two facets of the same coin. When Mtb enters the host lung via inhalation and invades interstitial tissue, the immune response is inevitably activated, resulting in host profile alterations (Bulterys et al., 2019; Yong et al., 2019). Since they are easy to use, affordable, and practical for POC, pathogen-specific antibodies are most often utilized as the host biomarkers for serologic tests. The Mtb-specific antibodies have been extensively investigated for infection diagnosis and response prediction to anti-TB treatment, including IgA, IgG, and IgM antibodies to: mycobacterial secreted 38-kDa and cytosolic 16-kDa antigens (Raja et al., 2008; Ben-Selma et al., 2011); antibodies against culture filtrate protein 10 (CFP-10) and early secretory antigenic target 6 (ESAT-6) (Andersen et al., 2000; Arend et al., 2000); and antigen 60, heat shock protein, purified protein derivative, and lipid-derived antigens (Kumar Verma and Jain, 2007). Even though participants had smear-positive results, earlier investigations revealed a low sensitivity diagnosis, approximately 75% at best, by ELISA (Raja et al., 2008). The heterogeneous quality and quantity of host antibody responses could be revealed as the main reason, and probably arise at different stages of the disease or are regulated by multiple host factors. Recently, several promising candidates were reported to improve the sensitivity and specificity of TB diagnosing assays, some widely common, such as: serum IgG against lipoarabinomannan (LAM) (Ben Selma et al., 2010); RV1255c-E and RV0310c-E antigens (Luo et al., 2017); proline-proline-glutamic acid protein 17 (Abraham et al., 2018); and mycobacterial DNA binding protein 1 (Maekura et al., 2019).
Host immunity protection against Mtb is not fully understood, but it depends on various innate and adaptive immunological mechanisms. Additionally, cellular immune responses to Mtb-specific antigens have demonstrated higher constancy than antibody responses (Yong et al., 2019). In principle, T cell-induced immune responses play a crucial role in controlling and protecting against Mtb infection (Walzl et al., 2011). At certain differentiated stages, CD4+ and CD8+ T cells progress to central memory (TCM) and effector memory (TEM), which activate and modulate phagocytosis and produce molecules that can directly influence cytotoxic microbial infection. Based on these understandings, several attempts have been undertaken to characterize the T cell-functional signature correlated with different TB stages. In particular, CD69 or CD137, a co-stimulatory receptor, is responsible for T cell activation and proliferation, and the frequency of CD69+ and CD137+ has been reported to link with active TB (Nikolova et al., 2013; Yan et al., 2017). Another study by Ahmed et al. used two markers for immune activation, CD38, and maturation, CD27, on Mtb-specific CD4+ T cells to differentiate active TB and LTBI (Ahmed et al., 2018). According to their observations, CD38pos CD27low frequencies were increased in active TB whilst LTBI performed CD38neg CD27high profile.
Treatment efficacy can be monitored via promising surface markers such as T-cell activation (CD38, HLA-DR) and proliferation (Ki-67) (Adekambi et al., 2015) under phenotypic characteristic analysis on Mtb-specific T cells. After nine weeks of anti-TB therapy initiation, CD38, Ki67, and HLA-DR frequencies of T cells were remarkably decreased and associated with stable culture conversion time (Ahmed et al., 2018). The amount of TEM and TCM could also be exerted as a predictor for reoccurrence, in which after six months of anti-TB therapy initiation the cases with a substantial amount of TEM may result in relapse because of living Mtb persistence, while TCM may reflect the complete clearance of Mtb (Millington et al., 2010; Wang et al., 2010).
Various host cytokines and chemokines are released once Mtb-specific antigens encounter and stimulate the immune responses of an Mtb-infected individual (Baatjies et al., 2021). The intricate relationship between Mtb and the immune system is attributed to the production of various cytokines in response to Mtb infection (Etna et al., 2014) which could restrict bacterial growth and modulate inflammatory responses that contribute to the development of TB (Bai et al., 2018). Additionally, chemokines are supposed to be closely associated with the development and maintenance of quiescent granulomas as well as enrolling periphery cells to positioning within the granuloma (Kumar et al., 2019b). Diverse investigations have focused on cytokine and chemokine identification which can improve discrimination between LTBI and active TB, treatment monitoring, and outcome prediction (Table 1).
The most popular commercial immune-based diagnosis for TB in clinical practice is still IGRAs despite the fact that IGRAs do not discriminate between active TB and LTBI and are perhaps inefficient in high-burden conditions (Organization, 2011; Pai et al., 2014; Jacobs et al., 2016). Hence, numerous studies have been reported to identify several singleplex or complex accurate and sensitive cytokine-based biomarkers for serological tests. In particular, cytokine/chemokine-based biomarkers can be used for monitoring treatment response in TB patients. Another examination was performed with plasma chemokines of PTB, LTBI, and healthy control (HC) individuals (Kumar et al., 2019b) to determine mycobacterial burden and disease severity. This study exhibited that the PTB chemokine levels containing CCL1, CCL3, CXCL1, CXCL2, CXCL9, and CXCL10 are significantly higher compared to LTBI and HC individuals. Furthermore, Kumar et al. also found that the plasma levels of CCL1, CCL2, CXCL2, CXCL9, CXCL10, and CXCL11 are all remarkably reduced following anti-TB chemotherapy, suggesting that they can be added to host chemokine panels for treatment-response monitoring. A meta-analysis was performed to identify valuable cytokines for distinguishing LTBI and active TB (Wei et al., 2020). Fourteen studies with 982 participants evaluated AUC, sensitivity, and specificity to reveal potential immune markers. As a result, seven molecules were identified that can discriminate between individuals with LTBI and active TB, including IL-2 (AUC, 0.91; sensitivity, 0.87; specificity, 0.61), IP-10 (0.86, 0.77, and 0.73), IL-5 (0.85, 0.64, and 0.75), IL-13 (0.85, 0.75, and 0.71), IFN-γ (0.80, 0.67, and 0.75), IL-10 (0.80, 0.68, and 0.74), and TNF-α (0.78, 0.67, and 0.64). Notably, due to their low specificity, single cytokines barely exhibit adequate diagnostic performance to be used as biomarkers. Also, host immune signatures have been developed in primary care settings (Mutavhatsindi et al., 2021). However, there is insufficient evidence to support their validity in secondary care settings.
Primarily evidence revealed that the host immune response to Mtb antigens is suppressed in resistant TB, but inflammatory cytokines still serve a fundamental role in the persistence of pulmonary tissue damage (Fortes et al., 2005). Impaired Th1-related cytokine production (Lee et al., 2002; Fortes et al., 2005; Geffner et al., 2009), such as IFN-γ, and increased Th2-related cytokines (Geffner et al., 2009), such as IL-4, were observed early in blood samples collected from MDR- and drug susceptible TB (DS-TB) patients, which emphasizes the alteration of Th1/Th2 profile characteristics in the disease progression. Furthermore, at the initiation of treatment for MDR-TB patients, peripheral Th1 and Th2 cells are remarkably diminished in comparison with drug susceptible TB (DS-TB) and HCs. These observations suggested the inhibition of both Th1 and Th2 pathways were intervened in the host response to MDR-TB infection (Tan et al., 2012). Besides the decreased level of IFN-γ, it was also found that IL-2 cytokines are significantly diminished in MDR-TB blood, even though they can function as protective cytokines against MDR-TB infection. Notably, different cytokine profiles are characterized in each stage of MDR-TB patients, with significantly decreased levels of IFN-γ, IL-2, and IL-10 in early infection, while during progressive necrosis and fibrosis, IL-4, IL-6, and TNF-α are increased (Tan et al., 2012). These results show that MDR-TB infection is undoubtedly complicated and challenging to treat. Thus, it is necessary to undertake extensive examinations for MDR-TB cytokine profiles that can identify biomarkers for MDR-TB diagnosis and treatment monitoring.
There are fluctuating cytokine profiles that coincide during the treatment process from the transition between intensive TB treatment and the continuation phase (Riou et al., 2012). The data from the study of Riou and colleagues demonstrated that only plasma levels of IP-10 and vascular endothelial growth factor (VEGF) remarkably changed in response to anti-TB treatment (Riou et al., 2012). These results substantiated previous observations that the reduction of plasma IP-10 is associated with successful TB treatment (Azzurri et al., 2005; Djoba Siawaya et al., 2009); hence IP-10 could be utilized as a valuable indicator of clinical response and treatment success. Furthermore, an investigation was recently conducted that quantified serum inflammatory and anti-inflammatory cytokine concentrations and compared MDR-TB, DS-TB, and HC (Mensah et al., 2021). The results identified a low concentration of serum IFN-γ and a high level of IL-4 related to MDR-TB compared to DS-TB individuals. Moreover, in the measurement of proinflammatory and anti-inflammatory cytokines, Granzyme B, IFN-γ, IL-4, IL-6, and TNF-α showed the greatest promise to differentiate between DS-TB or MDR-TB and HC. A recent systemic review identified promising biomarkers for the effective assessment of TB therapy, indicating that TNF-α is the most valuable biomarker (Clifford et al., 2015). Moreover, the proportion of both IFN-γ and IL-2 was reported to increase during the treatment period, suggesting they may be common markers of immunological protection against TB. However, the cytokine profiles from reported studies that focused on treatment monitoring in TB are controversial due to the heterogeneity of study designs and small sample sizes (Clifford et al., 2015), so extensive investigation is required to predict any failure of first-line treatments and ensure the adequacy of LTBI.
Despite treatments initially seeming to be effective, TB relapse occasionally occurs after completing anti-TB therapy and clinical symptoms re-emerge (De Steenwinkel et al., 2013). Transition to a negative sputum culture is generally considered predictive for effective TB treatment after two months (Wallis et al., 2013). However, this predictive method has poor sensitivity, with only 40% predicting relapse and 57% predicting failure outcome (Horne et al., 2010). Recently, emerging studies indicated that the failures of the host immune system to restrain invading Mtb, including immune disorders or severe immunodeficiency, are supposed to be the leading cause of re-treated TB (Bai et al., 2018). Bai et al. observed that cytokines released from Th1 and Th2 cells dramatically altered and upregulated Th1/Th2 cytokine ratios in re-treated TB patients (Bai et al., 2018). Several serum markers, including IFN-γ, IL-2, IL-7, and soluble CD54, were shown remarkably enhanced and more sensitive compared with other cytokines. Hence, they might serve as helpful serum indicators to determine TB re-treatment response. Moreover, a study from Steenwinkel et al. compared plasma cytokine profiles of primary TB and re-occurrence TB, especially in patients who were treatment non-compliant (De Steenwinkel et al., 2013). Not only the Mtb load in infected organs, additionally, in the cases of HIV-infected individuals or patients suffering a long period of immunosuppressive therapy with TNF-α inhibitors, the reactivated risk of LTBI increased remarkably (Bruns et al., 2009; Getahun et al., 2010; Amelio et al., 2019). Hence, besides biomarkers that imply successful treatment during the therapeutic period, more specific indicators are required to predict relapse at the end of six-month treatment, which might significantly enhance clinical prognosis. Interestingly, an investigation from Ronacher et al. disclosed that the levels of IL-5 and MMP-2 decreased, while sIL-2Rα and CRP were highly elevated at diagnosis in relapse patients in comparison with failed and cured individuals (Ronacher et al., 2019).
A multi-omics approach has facilitated the identification of biomarkers and provided a profound understanding of the pathological processes in the field of TB (Jakhar et al., 2020; Pitaloka et al., 2022). A multi-omics approach can provide a holistic view of disease mechanisms and better predict disease outcomes (Hasin et al., 2017; Ntoumi et al., 2022). Therefore, multi-omics has been recognized as one of the crucial pillars for personalized medicine for TB. The integration of genomics, transcriptomics, proteomics, and metabolomics approaches to monitoring TB treatment has been suggested recently (Pitaloka et al., 2022). However, as TB is an infectious disease that triggers the inflammation agents during the disease phases, immunomics plays a key role in the multi-omics approach (Bonaguro et al., 2022). Transcriptomic, metabolic, epigenetic, and functional immune studies should be integrated and further analyzed with correspondence to clinical outcomes data (Lange et al., 2020; Ntoumi et al., 2022). Although the biomarkers found have not been further validated in a larger cohort, so far the identified biomarkers hold promise for treatment monitoring, disease prediction, and differential diagnosis of TB (Yong et al., 2019; Perumal et al., 2021; Pitaloka et al., 2022).
An immunomics approach has been widely investigated to differentiate between active TB, LTBI, and/or healthy people (Wei et al., 2020; Mutavhatsindi et al., 2021). Earlier publications found higher proportions of neutrophils and monocytes in active TB compared to non-active TB patients (Wang et al., 2015; La Manna et al., 2017; Estévez et al., 2020a). On the other hand, the proportions of T-helper (CD4+) cells and B cells were higher in non-active TB, suggesting that adaptive immune response is lower in active TB patients. However, as it appears more challenging, the immune expression profiles of those with LTBI largely overlapped with HC, making it difficult to distinguish separate clusters based on the 1,000 most variable genes (Bah et al., 2018). It was discovered that the type I and type II interferon signaling pathways’ upstream and downstream components are expressed more strongly in peripheral blood leukocytes from active TB patients (Berry et al., 2010; Zambuzi et al., 2016). Additionally, another study has shown that the expression of CD64, LTF, and Rab33A together formed biomarkers that can distinguish individuals with and without TB (Jacobsen et al., 2007). Furthermore, it has been widely recognized that IL-6 could be useful for monitoring the effectiveness of anti-TB drugs and, together with sCD14, used to distinguish the active state of the disease (Yong et al., 2019; Boni et al., 2022). Previous reports also showed that in active TB patients, plasma levels of sCD163 and TNF-α were linked with the severity of the condition and extent of lung damage (Zambuzi et al., 2016; De Andrade Júnior et al., 2008; De Steenwinkel et al., 2013). Another study also identified several increased immune markers were linked to active TB patients, reported as GM-CSF, IFN-α-2, IL-4, IL-5, IP-10, and MIP-1α (Zambuzi et al., 2016; Estévez et al., 2020b). Until now, the diagnosis confirmation of LTBI patients still followed the results of the IGRA test (Herrera et al., 2011). Nevertheless, several limitations, such as the large amount of blood needed, limited assay reproducibility, low sensitivity, unknown prognostic value, and slow sample processing, have limited IGRA usage for mass testing (Herrera et al., 2011; Kaul et al., 2022). A recent publication confirmed FCGR1B, GBP1, and GBP5 as the transcript signatures that distinguished between LTB1 and active TB, which could be helpful in screening LTBI on a mass scale (Kaul et al., 2022). Furthermore, they classified the LTBI into clusters following the IFN-γ values in TB1 and TB2 antigens using machine-learning cluster analysis. Such a clustering approach may help to identify which group would likely progress to become active TB, thus effectively targeting preventive therapy in this cluster. However, the implementation of immunomics to distinguish between active TB and LTBI remains challenging. Even though these biomarkers could be further used to monitor treatment, differentially diagnose, and predict disease progression, little to none of these markers have been validated in extensive prospective studies. The ratio among biomarkers might keep changing inconstantly throughout the treatment phase and be considered as a dynamic of biomarkers, another critical challenge to be addressed (Dheda et al., 2010). Therefore, utilization of homologous (e.g., gene experiment only) or heterogenous (e.g., cytokines and endogenous metabolites) biosignatures to distinguish disease diagnoses and treatment monitoring would be much more reliable and preferable, rather than the identification of single biomarkers.
Special or edge populations usually appear with physiological changes or system immaturities that may produce different immune cell markers (Ciabattini et al., 2018; Abu-Raya et al., 2020; Aulin et al., 2021). Pediatric, pregnant, elderly, and immunocompromised patients are recognized as populations that confound standard TB treatment strategies (Nahid et al., 2016). Determining the TB diagnosis in the pediatric population remains challenging with current routine clinical and laboratory tests (Gunasekera et al., 2022). Both the TST and IFN-γ fail to differentiate diagnoses of TB in pediatric patients. Hence, the WHO have promoted the pediatric TB scoring chart that was invented to help with assessment in limited-resource countries which might be biased (Van Rheenen and Health, 2002). Furthermore, treatment monitoring remains elusive in this population. Most of the time, a clinician only assesses successful treatment based on weight gain and loss of symptoms (Chiang et al., 2020). Although an immune markers-based test promises to solve this issue, no immune-diagnostic or monitoring test is well-developed and validated (Yong et al., 2019; Kumar et al., 2021a). A recent publication found that pediatric TB patients have 20% differentially expressed genes in comparison to adults (Bah et al., 2018). It is very well-known that childhood patients are characterized notably by the significant increase of IFN-regulated genes (Yoshida et al., 2022). Nonetheless, other regulated immune pathway genes may also be upregulated, including the elevation of alternative antimicrobial defense mechanisms (H2AFJ, HISTIH2BG, and CTSG) and multi-functional IL-27 that blocks IL-17 and IRF1 signaling (Bah et al., 2018). In addition to upregulated genes, pediatric TB is also characterized by downregulated genes, with pathways primarily engaged in stimulation of T or B cells (CD40L, CD7, ICOS, FCER2, PTPRCAP, and ADAM23), dendritic cell development (FLT3LG), alternative promoters of inflammation (CD248 and EDAR), and inhibition of neutrophil degranulation by ADORA3.
Due to their central role in promoting immune responses, monocytes and lymphocytes may reflect a person’s immune status during infection. In TB infection, Mtb will target the monocytes for their growth, while lymphocytes are principally responsible for Mtb clearance. Monocyte to lymphocyte ratio (ML ratio) has gained interest as a potential biomarker that can classify TB diagnosis even in special populations. A previous report investigating infants born to HIV-positive mothers revealed that the increased ML ratio in peripheral blood was associated with definite TB disease. Although the ML ratio could be a good predictor for TB disease, the ratio showed a modest role in predicting TB disease-free survival (Naranbhai et al., 2014). Another study reported that an extreme deviation of the ML ratio, either high or low (median of ML ratio: 0.36), was correlated with active TB disease. The authors also mentioned that patients with EP-TB and an age of >60 years were more likely to have severe ML ratio alterations (Wang et al., 2015). Since Mtb infection can interfere with the development of hematopoietic stem cells (HSCs), the ML ratio might be affected differently among people. For example, Mtb hinders HSCs engraftment and uniquely reorganizes HSCs (Khan et al., 2020); Mtb infection on HSCs could wreak havoc on the balance of myeloid and lymphoid lineages development in different people (Tamburini et al., 2021). This condition could return to normal with the administration of anti-TB therapy. On the contrary, an earlier study failed to distinguish between LTBI and active TB using the ML ratio (Buttle et al., 2021). Even though the ML ratio showed great sensitivity, the specificity was poor. However, this study discovered a higher ML ratio in males due to less effective immune responses in males. Therefore, the severity of TB disease might be more advanced in male patients. Another study confirmed this finding, showing that the ML ratio (median: 0.5) could distinguish patients with active TB from those of healthy volunteers (La Manna et al., 2017). Despite this, it failed to distinguish active TB from LTBI patients and cured TB patients. Apart from the ML ratio, the neutrophil to lymphocyte ratio (NL ratio) has recently been proposed as a biomarker to distinguish active TB, LTBI, cured TB, and HC. A recent report found that active TB patients appeared to have a high NL ratio compared to both HC and cured TB patients (La Manna et al., 2019). Among those with active TB, a higher neutrophil count and a lower lymphocyte count were identified. Nevertheless, this ratio was gradually decreased by TB treatment, showing the potential of this marker to monitor TB as well. Understanding these potential biomarkers to diagnose TB is considerably important to provide faster and easier confirmation of TB. Therefore, anti-TB drugs can be started immediately. The aforementioned biomarkers were routinely performed in hospital laboratory settings, even in low-resource countries. Although the precision remained inconclusive, these biomarkers may serve as an alternative in limited-resource settings because, as cytokine/chemokine secreting cells, the changes in the ML ratio may contribute to the variation of cytokine/chemokine composition. Further consideration related to other progressive infections possibly affecting the results should be assessed in the implementation of the markers.
Despite substantial differences in immune responses between adults and pediatric patients, some similarities can be observed. There is an increase of lymphocyte and a decrease of macrophage percentages in both adults and children with TB (Herrera et al., 2022). Nonetheless, the downregulation of IL1RN and IL1R2, which are involved in the generation of the adaptive immune response, is believed to cause disseminated infections in pediatric patients (Bah et al., 2018). Even so, the common immune pathways activated during TB infection are similar between TB adults and children regardless of the different clinical symptoms presented by these two groups (Berry et al., 2010). Several immune chemokines have also been reported to differentiate between active TB and non-TB in children, which showed higher baseline levels of CCL1, CXCL1, and CXCL10 (Kumar et al., 2021a). Those chemokines can decrease with treatment and are useful for treatment monitoring.
In adult TB patients, chemokines have been widely considered as potential diagnostic biomarkers. Indeed, CCL1, CCL3, CXCL1, CXCL10, and CXCL11 were associated with TB disease severity and positively correlated with bacteria load, while CCL1, CXCL9, CXCL10, and CXCL11 could discriminate PTB from LTBI infection in individuals (Kumar et al., 2019b). MCP-1 was also demonstrated to differentiate between PTB, endobronchial TB, and TB pleurisy (Xiong et al., 2016). Baseline levels of plasma CCL2, CCL3, CCL4, CXCL8, CXCL10, CX3CL1, and CXCL1 could predict unfavorable treatment outcomes in PTB (Kumar et al., 2021b). Nevertheless, pregnant women have different immune profiles compared to adults, and transient changes in immunity occur during pregnancy (Abu-Raya et al., 2020). A previous study found that pregnant women with LTBI had lower levels of various proinflammatory cytokines, such as IL-1β, IL-6, and IL-17A, and a higher level of IFN-γ, notably during the second trimester (Naik et al., 2021). These results suggested that latent TB during pregnancy is characterized by a distinct immune profile with high levels of IFN-γ but low levels of other immune markers known to play a role in TB. The mechanisms for this finding were unclear. Previous studies on the mechanisms of TB disease showed that IFN-γ could give negative feedback to IL-1β, IL-6, and IL-17A in some instances (Nandi and Behar, 2011; Eigenbrod et al., 2013), thus high levels of IFN-γ might be associated with a low level of other markers. Additionally, an increase in neutrophil levels was observed during pregnancy and associated with lower IL-6 and IL-17 in TB infection (Sacks et al., 1998; Nishida et al., 2007).
Furthermore, immune markers might be altered by several factors, including age. Studies of immune marker concentration for diagnosis or monitoring TB disease in either elderly or preterm neonates were hard to find. However, physiological changes and the complicated clinical conditions that commonly appear in this population should be noted (Ciabattini et al., 2018; Aulin et al., 2021). TB in the elderly is often associated with the reactivation of quiescent lesions (Caraux-Paz et al., 2021). These activated lesions might be attributable to changes in the immune system related to aging, notably the decline in the ability to reactivate acquired immunity or immune response expressions. Elevated plasma levels of IL-6, IL-1, and TNF-α have been observed in the elderly (Weiskopf et al., 2009; Martín et al., 2017). These markers were previously described as “inflammaging” and are considerable for biomarker-based therapeutic optimization strategies (Franceschi et al., 2018; Aulin et al., 2021). On the other hand, preterm infants have a notable reduction in IL-1β, IL-6, and TNF-α because their innate immune responses are amid the developing process (Delanghe and Speeckaert, 2015). In addition, within several days after birth, preterm and term infants were reported to have higher levels of CRP and PCT, likely related to birth stress (Sharma et al., 2012).
Since TB frequently co-exists in immune-compromised patients, people who live with HIV are the most vulnerable to infection and disease progression risk (Organization, 2015). The increased susceptibility to TB in HIV-infected individuals is likely due to the characteristic immunological effects of HIV that predominantly infect CD4+ T cells (Okoye and Picker, 2013; Geremew et al., 2020). Both CD4+ and CD8+ T cells have been implicated in the immune response against Mtb infection (Prezzemolo et al., 2014). However, animal studies have shown that CD4+ T cells are more critical than CD8+ T cells in the host’s immune response to fight TB (Lin and Flynn, 2015). Additionally, there is growing evidence that TNF-α-dependent macrophage apoptosis is reduced with HIV-TB co-infection (Patel et al., 2007; Walker et al., 2013). These findings indicate that not only is adaptive immune response altered in HIV subjects, but also the innate immune response. A further condition regarding HIV patients that may complicate TB treatment is tuberculosis-immune reconstitution inflammatory syndrome (TB-IRIS). TB-IRIS is an abnormal and excessive immune response against alive or dead Mtb that usually occurs in HIV-infected patients (Tadokera et al., 2011; Naidoo et al., 2012). This condition may appear after the initiation of antiretroviral therapy independently of an effective suppression of HIV viremia. TB-IRIS decreases the levels of several circulating inflammatory markers, including IL-6 and Th1/2/17 cytokines (IL-2, IL-3, IL-12, IL-15, and IL-17A) (Ravimohan et al., 2015). The cytokines stimulate T and natural killer (NK) cell responses to activate macrophages and control Mtb infection (Gonzalez‐Juarrero et al., 2005; Rausch et al., 2006; Méndez-Samperio, 2010). Therefore, low pre-ART levels of these cytokines in TB-IRIS patients may implicate abnormal innate immune responses and further hamper pathogen clearance. Measurement of immunologic profiles prior to and early after ART initiation for HIV patients is encouraged to predict the treatment response and guide the regimen changes in TB therapy.
Another concern regarding immunocompromised patients is diabetes mellitus (DM). It has been widely reported that DM and TB often appear together, especially in the aging population (Niazi and Kalra, 2012; Lin et al., 2015). DM is known as a risk factor for the conversion of LTBI into active TB (Hensel et al., 2016). The function of neutrophils, macrophages, dendritic cells (DC), NK cells, and other components of innate immunity, as the first-encounter immune responses, are compromised due to metabolic changes in DM patients (Martinez and Kornfeld, 2014; Yew et al., 2017). Hence, type 2 DM patients may be characterized by the decreased secretion of IL-1β, IL-12, IL-18, and IFN-γ, which increases their susceptibility (Stalenhoef et al., 2008). Additionally, DM patients also manifest impaired adaptive immune responses. Previous studies revealed that TB patients with DM had significantly lower frequencies of both myeloid DC and plasmacytoid DC compared with individuals with only TB (Ayelign et al., 2019). Hyperglycemia was reported to cause a lower DC and neutrophils count in TB patients with DM (Kumar Nathella and Babu, 2017; Raposo-Garcia et al., 2017). As an explanation, hyperglycemia may increase adhesion and integrin expression, reduce chemotaxis, defect phagocytes, and reduce microbicidal activity (Raposo-Garcia et al., 2017; Ayelign et al., 2019).
Furthermore, DM also may potentially influence and/or decrease the frequencies of Th1 and Th17 cells due to the increased frequencies of Th2 cells which produce IL-4 (Yamashiro et al., 2005; Kumar Nathella and Babu, 2017). Given all the aforementioned conditions, the incorporation of immunomics into clinical settings would bring more insights to guide safe and effective treatment for these special populations. It is also worth noting that Mtb-specific immune responses are probably not homogenous in all populations and might be influenced by the immunity alterations of each specific population. Hence, using generalized potential immune biomarkers to monitor and diagnose TB in these populations may not be appropriate.
Interindividual variability (IIV) has been recognized as one of the most important factors causing slow treatment response, treatment failure, and the development of drug resistance in TB treatment (Srivastava et al., 2011). Anti-TB drugs are widely known to exhibit wide IIV on exposure and maximum concentration (Cmax), challenging the finding of optimal dose levels for patients (Zuur et al., 2016; Sturkenboom et al., 2021). IIV affects both the pharmacokinetics (PK) and pharmacodynamics (PD) of the drugs, which is caused by multiple factors, including environment, genetics, demographics, concomitant medications, and comorbidities (Sturkenboom et al., 2021). It is well known that immune recognition, immune response, and immune regulation of Mtb determine the occurrence, development, and outcome of the disease. Even though the immune cells’ effects on the PK/PD of the anti-TB drugs has not been fully understood, the general impact of immune cells in altering the drug metabolism enzymes and transporters may be acknowledged (Christensen and Hermann, 2012). Like other infectious diseases, TB may activate the immunological response and release cytokines as a part of its pathophysiology (Dheda et al., 2010). Cytokines are a critical component of the immune response, which act as chemical mediators and bridge the physiological communication among cells throughout the body (Newton and Dixit, 2012). Given the importance of cytokines, understanding how they affect the expression of proteins that influence the outcome of drug therapies is essential. Previous reports demonstrated that cytokines regulate the expression and activity of drug-metabolizing enzymes and transporters, thereby affecting the PK of drugs (Christensen and Hermann, 2012; Sanz Codina and Zeitlinger, 2022). Cytochrome P-450 (CYP) enzymes are involved in the biotransformation of various endogenous as well as exogenous compounds (Tomaszewski et al., 2008). Most anti-TB drugs are metabolized by CYP enzymes (Horita and Doi, 2014). IFN-γ, produced by Th1 in response to TB infections, is known to downregulate the expression of CYP1A2, CYP2B6, CYP2C8, CYP2C9, and CYP3A4 (Wu and Lin et al., 2019). Rifampicin (RIF) is metabolized partially by CYP3A4, while CYP1A2 is needed for hydrazine, one of isoniazid (INH) metabolite, detoxification (Chen et al., 2006; Tostmann et al., 2008). The downregulation expression of these CYPs enzymes causes adverse drug reactions related to isoniazid (INH) and rifampicin (RIF). In addition, IFN-γ, IL-2, and IL-12 are associated with the increased expression of ABCB1 (encodes P-gp), which is responsible for the low systemic exposure of RIF. The low exposure of RIF as one of key drugs in TB treatment may contribute to the risk of obtaining drug-resistant TB. In addition, the reduced oral clearance of drugs with intermediate to high hepatic extraction ratios, which may affect low binding drugs like INH more, was observed in the patients with elevated proinflammatory cytokines (Mayo et al., 2000; Gausi et al., 2021). A summary of each immune marker and its association with PK parameters are given in Table 2.
However, it is worth mentioning that not only do immune profiles affect the PK of anti-TB drugs, but anti-TB drugs can also directly or indirectly regulate immune profiles (Park et al., 2021). Anti-TB drugs alter the immune system by indirectly affecting microbiota composition or directly affecting immune cell functions (Ubeda and Pamer, 2012; Moffatt et al., 2019). Thereby, the clinical outcomes and treatment response vary between patients. For instance, INH induces the apoptosis of activated CD4+ T cells and impairs the production of Mtb-specific IFN-γ and anti-CFP-10 antibody in latent TB patients (Tousif et al., 2014). Furthermore, the administration of RIF disturbs the cytokine cascade by suppressing IL-1β and TNF-α, and inducing IL-6 and IL-10 secretion (Ziglam et al., 2004). Other anti-TB drugs also are known to modulate immune profiles and are further summarized in Table 3. Considering the previous findings for both immune profiles and anti-TB drug association, comprehensive evaluation and understanding of TB pathophysiology, immune pathways, and PK profiles of anti-TB drugs should be carried out during biomarker validation for personalized medicine services in TB treatment.
Table 3 Anti-TB drugs modulation to immune profile" into "Anti-tuberculosis drugs modulation to immune profile.
To overcome IIV, TDM has been utilized to adjust the dose following patient’s PK/PD profile. This can reach well-defined PK/PD targets associated with the efficacy and toxicity of drugs (Alsultan and Peloquin, 2014; Lange et al., 2020). Most of the dose adjustments that have been done in clinical settings follow the PK/PD indices of specific anti-TB drugs (Alsultan and Peloquin, 2014; Sturkenboom et al., 2021). Nonetheless, substantially unpredictable variations in treatment response remain for some patients. Demographic characteristics, clinical conditions, and genetic variants of metabolic enzymes or transporters of anti-TB drugs are commonly identified as the source of IIV affecting the treatment response of patients. Apart from the previous findings, other sources of IIV, in relation to pathogen characteristics, a patient’s immune response, or the effects of inflammation on PK, should also be explored (Young et al., 2020; Aulin et al., 2021). In the near future, precision medicine for TB should not only depend on the pharmacological aspects of anti-TB drugs, but rather also focus on biomarker-based treatment decisions and monitoring (Alsultan and Peloquin, 2014; Aulin et al., 2021). Biomarkers hold the potential to inform treatment strategies and aid decisions throughout all phases of infection (Delhalle et al., 2018). However, the current application of biomarkers in TB treatment has primarily been introduced for diagnosis.
Recently, immune profile biomarkers have been of interest to quantify treatment efficacy in relation to treatment response due to the length of time needed for bacterial culture and the difficulty of sputum production (Thorsted et al., 2020; Mensah et al., 2021). During TB infection, the immune response is triggered by Mtb, producing several immune biomarkers. To guide and optimize TB treatment, immune biomarkers should ideally reflect the underlying pathogen load. In addition to the role of immune biomarkers for treatment monitoring and outcome prediction, the effect of immune biomarkers on IIV of anti-TB drugs PK also should be evaluated. It is widely known that infection induces inflammation effects on the absorption, distribution, metabolism, and elimination processes of drugs (Radke et al., 2017; Charlton and Thompson, 2019). Hence, immune biomarkers that capture infection-induced inflammation effects and predict IIV on anti-TB drug PK parameters are significantly relevant for further individualizing TB treatments.
Early and accurate detection of active TB is crucial to prevent transmission of TB, yet existing diagnostic procedures are insufficient. Despite the development of several immunoassays for determining antibodies or antigens in serum samples, only a few diagnostic methods are available (Kashyap et al., 2007). Typically, ELISA is the primary analytical tool to quantify mycobacterium-specific components (Kashyap et al., 2007; Reither et al., 2009), host antibody response (Ben Selma et al., 2010; Zhu et al., 2012), and cytokine/chemokine production (Hur et al., 2013; Indrati et al., 2022) for diagnosis and monitoring treatment (Kim et al., 2021). Kashyap et al. employed an indirect ELISA method to diagnose TB by monoclonal antibodies against Ag 85 complex, which yielded high sensitivity and specificity of up to 82% and 86%, respectively. Recently, a quantitative lateral flow assay was established for adjunct diagnostics and treatment monitoring of TB and COVID-19 (Pierneef et al., 2022). Analysis of seven host sera proteins exhibited potential in differentiation of LTBI and TB, HC and COVID-19 patients, and severe COVID-19 and TB (Pierneef et al., 2022). Additionally, there are several alternative approaches to detect TB in serum and urine, including dot-immunobinding assay (Bentley-Hibbert et al., 1999), nucleic acid amplification (D’Amato et al., 1995), the application of two-dimensional polyacrylamide gel electrophoresis (2DGE), and liquid chromatography-tandem mass spectrometry (LC-MS) (Kashyap et al., 2005).
Flow cytometry is an alternative approach frequently used to evaluate various cell surface markers and intracellular cytokine production, permitting the characterization of distinct immune cell types. Several analyses using this method manifest the possibility of distinguishing between active, LTBI, and uninfected patients (Harari et al., 2011; Pollock et al., 2013; Estévez et al., 2020a), and assessing treatment efficacy (Ahmed et al., 2018) as well as drug susceptibility (Hendon-Dunn et al., 2016).
More recently, multiplex bead array assays have emerged in analytes quantification (Elshal and McCoy, 2006) simultaneously due to their capability to provide a vast quantity of bio-information from a small sample size for a better prognosis, diagnosis, and treatment (Ahsan, 2021). The multiplex immunoassays make use of conventional immunoassay methods that capture circulating antibodies or proteins by using either antibodies or peptides/proteins as binder molecules (Ahsan, 2021). In suspension experiments, the targeted ligands are immobilized on color- or size-coded plastic microspheres, and flow cytometry is used to identify particular fluorescent signals (Ellington et al., 2010). Bead-based flow cytometry technologies that enable multianalyte measurements in clinical diagnostics are already widely accessible on commercial platforms (Spindel and Sapsford, 2014). By using multiplex methods, such as xMAP technology developed by Luminex or Bioplex, immunomics is by far strengthened in reducing technical burden. The Luminex xMAP system is one of the pioneers (Luminex Corp., USA). It has been employed in numerous recent studies to investigate and validate immune biomarkers for TB diagnosis (Phalane et al., 2013; Xiong et al., 2016; Kumar et al., 2021a; Mutavhatsindi et al., 2021), treatment monitoring (Kumar et al., 2020), and recurrence prediction (Sivro et al., 2017). Conversely, a conventional ELISA experiment is laborious and usually takes days to quantify multiple immune molecules. However, a multiplex system facilitates simultaneous evaluation and demonstrates correlation in data with ELISA within several hours (Wang et al., 2005). On another scale, Meso Scale Discovery (MSD), a global multiplex analysis to characterize innovative assays for biological molecules, is also worth considering. Sharing comparable specifications, both Luminex and MSD are applicable for multiple immune profile analyses (Chowdhury et al., 2009). However, while MSD has a wider dynamic range and sensitivity, Luminex’s strength is emphasized in its precision (Sourial et al., 2009; Günther et al., 2020), which may be more suitable for the considerable input of biomarkers across the wide range of personalized treatments. Therefore, despite the need for further validation, multiplex technology, especially Luminex, is taking steps to unlock complex cytokine networks and reveal new biosignatures for TB. The remarkable milestones of these TB-related immunological investigations are briefly depicted in Figure 2.
Figure 2 Representative immune biomarkers corresponding to TB diagnosis and management. The approach utilized for TB investigation has been gradually converted from conventional ELISA to high-throughput technology with multiple advantages. More and more immune biomarkers have been reported for different disease stages and types. Robust and affordable high-throughput technologies and data analysis facilitate the discovery of clinically relevant biomarkers via well-established study designs. (ELISA, Enzyme-linked immunoassay; xMAP, Multi-Analyte Profiling; LTBI, Latent TB infection; MDR-TB, Multidrug-Resistant Tuberculosis).
The immune system is a complicated and pervasive network that contains adaptive responses of numerous cell types and intracellular signals to recognize pathogens or stimuli and cross-communicate to perform protective functions (2013). With respect to this complexity and universality, there is no single golden standard for what to invest in among multi-omics layers. Whilst a single omics layer can only provide insight into a particular aspect, immunological studies tend to adopt different omics measurements to build rigorous profiles of samples at diverse levels, including genetics, epigenetics, transcriptomics, proteomics, metabolomics, and cellomics (Yu et al., 2019; Ota and Fuijo, 2021). In this part, we briefly discuss different technologies that can be applied in immunomics.
Genomics characterizes genetic diversity from single-nucleotide polymorphisms (SNPs) and insertion/deletion (InDels) to large-scale mutations that trigger polymorphism in the individual immune system (Netea et al., 2016). Popular techniques include PCR-based methods such as mixed-linker PCR (ML-PCR), fast ligation-mediated PCR (FliP), ligation-mediated PCR (LM-PCR), and DNA sequencing such as Next generation sequencing (NGS) and microarray.
Uninvolved in the changes in the nucleotide sequences, epigenomics evaluates chromosome accessibility, structure, and any modification of chromatin, DNA, or histone such as methylation and acetylation. The most commonly used techniques to capture chromatin phenomena are assayed for Transposase-Accessible Chromatin using sequencing (ATAC-seq), DNase-seq, and FAIRE-seq. By using the high throughput sequencing technique, both genomics and epigenomics facilitate huge reference genome databases for shotgun analysis with a static link to the organism. However, besides the need for high-performance machine installation, genome reconstruction by bioinformatic software also requires advanced training and troubleshooting, disregarding the fact that many sequenced elements’ activity cannot be fully identified (Chu et al., 2021; Ahamad et al., 2022).
Transcriptomics depicts RNA profiles under coding and non-coding transcripts of either a single cell or a bulk level. Unlike traditional PCR, which only amplifies a limited number of genes simultaneously, currently used transcriptomic techniques such as RNA-sequencing (RNA-seq) and microarray can quantify the entire transcriptome or the large-scale targeted analysis of most well-known genes. However, aside from handling errors during the long process of RNA extraction and sequencing, there is an undeniable disadvantage: RNA polymorphism is not always reflected in protein translation and functional expression (Chu et al., 2021; Ahamad et al., 2022).
While the genome is generally constant, proteome differs within cells and with time. Therefore, the large-scale study of proteins, proteomics, is more predictably complicated than other omics studies. In the immune system, immune molecules are often detected by either traditional immunofluorescent staining, 2DGE, or modern immunoassays such as ELISA, EMIT (enzyme multiplied immunoassay technique), and mass spectrometry-based approaches: LC-MS and MALDI-TOF (Matrix-Assisted Laser Desorption/Ionization-Time of Flight). Proteomics describes a direct link to organism phenotypes by surpassing the genomics-based approach regarding the correlation to functional expression. Furthermore, as the direct readout of the phenotype, metabolomics is suitable for quantifying metabolic responses to pathophysiological stimuli or genetic modification of a living organism. Metabolomics and its sub-field, lipidomics, can deal with complex metabolites at a high resolution to provide a reliable link between phenotypic characteristics and metabolic profiles. This technology is also helpful for discovering metabolism-centric biomarkers for TB (Long et al., 2022). However, the variation of metabolites is unavoidable, which may lead to several sampling artifacts and fluctuations (Chu et al., 2021; Ahamad et al., 2022). Additionally, metabolite and protein annotation is not always robust, the quantitative information is often limited, quality assurance/quality control (QA/QC) is troublesome, the cost is high, and the technical requirements are affordable only for specialized laboratories.
It has been highlighted that a single omics approach does not decently capture the whole biological process of TB and is unable to realize the true potential of omics for clinical research and implementation (Subramanian et al., 2020). The combination of transcriptomics and metabolomics to discriminate TB progressors improved the sensitivity of the test by up to 12% while still preserving the specificity value of 80% (Duffy et al., 2019) in comparison to using transcriptomic signatures alone. The upregulation levels of metabolite cortisol and the genes SOC1 and DDIT4 also were found to be associated with the immuno-metabolic pathways of TB progression.
Considering the drawbacks of previous omics studies and the need for direct references for TB clinical practice, immune profiling emerges as a promising solution that directly measures the immune response to pathogens, including all immune-related molecules and their regulators or targets. High-throughput technology, e.g., xMAP, is a game changer for immunologists (Figure 3). Immune molecule quantitative analysis not only ignores the transient nature of metabolites and the inconstant reflection from genes to proteins, but can also measure immune functioning during the development of therapies. It easily limits the waiting time for the clinical manifestations of toxicity, and overcomes the robustness problem of the former proteomics by integrating multiplex technology. Therefore, it increases the detectability and decreases the risk of unexpected metabolites and the number of subjects required in a trial.
Figure 3 An immune-centric approach for TB investigation in the omics era. Comparison of the high-throughput approach (left) and conventional assay (right) for immune profiling investigation in TB diagnosis and monitoring. Other matured omics technologies are capable of providing complementary information about the immune profiles of TB patients. LC-MS, liquid chromatography-mass spectrometry; TB, Tuberculosis.
However, immunomics creates a complicated scenario because it studies and manipulates the genes and proteins involved in immunologic functioning and the complex cellular microenvironment. The future of immunomics lies in the continued pursuit of validation of immunologic markers correlated with clinical outcomes, which is now limited, and many technologies are still in their infancy (Tremoulet and Albani, 2005; Chu et al., 2021; Ahamad et al., 2022). In other words, this is a chance to build up a platform worthy of investment, which only requires intense studies and improvement but has several advantages the previous one lack.
The concept of personalized medicine highlights the importance of a patient-tailored regimen by considering individual physiological and pathological factors. As has been stated earlier, one of the cores of personalized medicine is the translational process from the bench to bedside and vice versa (Hartl et al., 2021). As multi-omics appears to be one of the key components of translational personalized medicine, integrating multi-omics from early development (translational medicine) into late development (personalized medicine) is of the utmost importance (Shakhnovich, 2018; Hartl et al., 2021). Each omics discipline has different values for different clinical settings. Considering the nature of this infectious disease, which needs the optimal treatment at the earliest timing, immune profiling may be a game-changer in personalized medicine for TB (Di Martino et al., 2020; Wicha et al., 2021). The current approach to confirming TB diagnosis needs drug-susceptibility testing in the form of bacterial culture, and it has a long turnover time for results (Organization, 2020). The validated molecular biomarkers, especially the immune profile or a panel containing heterogeneous biomarkers, could be used to classify differential diagnoses of TB infection from the beginning of treatment, eventually saving much treatment cost and time (Yong et al., 2019). Furthermore, the markers can be utilized in treatment monitoring and predicting which patients have a higher risk of treatment failure (Duffy et al., 2019; Yong et al., 2019). Regimen changes and dose optimization could be done sooner rather than waiting for the radiological changes, acid-free bacilli staining, culture conversion, and evaluation. A strong point of immunomics is its small sample volume with no time-sensitive sampling needed. Thus, this approach is more convenient for patients and real clinical-setting practice. We have suggested a framework for facilitating the research and development of immune biomarkers (Figure 4). It is comparable with the study design and technical requirements of other functional omics platforms, such as proteomics, metabolomics, and lipidomics. Indeed, rapid diagnosis and excellence treatment monitoring are the keys to eradicating TB (Alffenaar et al., 2020). Previously, the WHO has endorsed a new target product profile with regards to a non-sputum-based test capable of detecting all forms of TB by identifying characteristic biomarkers or biosignatures (Organization, 2014). The POC biomarker test is intended to enable the diagnosis of PTB and EP-TB, as well as TB in pediatric.
Figure 4 Sample collection strategy of immunological biosignature discovery. The sample collection for immunological biosignature discovery should focus on three critical phases of tuberculosis (TB) treatment: 1) Baseline; 2) Evaluation; 3) Treatment completion. The samples taken in the baseline before TB treatment is started could be used as biomarker for diagnosis confirmation. Meanwhile, the samples collected in the evaluation phase could be used to identify biomarkers for treatment monitoring. Further, the biomarkers could predict the early bacterial conversion and/or adverse drug reaction potency. The samples taken during the treatment completion could be helpful to predict the successfulness of treatment and possibility of future recurrence. Contributions to each phase might be from either the same patients or different patients of a similar age and gender compared to the patients enrolled in other phases. DS, Drug Susceptibility; DR, Drug Resistance; TB, tuberculosis.
The COVID-19 pandemic has jeopardized TB treatment and further substantially changed the conventional research and development (R&D) approach in many aspects (Acosta et al., 2022; Petersen et al., 2022). Virtual utilization of advanced technology, data-based, data-sharing, and collaborative research are now preferable (Hartl et al., 2021; Acosta et al., 2022). The further integration of multi-omics and put artificial intelligence (AI)-based analysis have also shown great potential for digital biomarker endpoints and diagnoses. Considering all the opportunities to improve TB treatment strategies that have arisen during the COVID-19 era, several pitfalls challenge the success of the new current R&D approach (Acosta et al., 2022). It should be noted that biomedical data frequently have high numbers of missing data or clinical information to validate the findings. It is sometimes more appropriate to fill these gaps through statistical procedures, such as multiple imputations, even though, in some circumstances, it is possible to simply exclude patients with missing data prior to training. Nonetheless, this approach might further introduce bias into the analysis. Although large datasets are needed to train adequate model predictors, a data set with various covariates or features would result in better predictions, due to a more comprehensive evaluation of variable combinations. Additionally, longitudinal or repeated-sampling study designs also have certain advantages, such as increased statistical power and better PK-/PD-profile modeling. It should be noted that extensive data collection would be challenging at times and need multi-nation collaboration. Another foremost challenge to address is the geographical barrier in data collection, which limits the evaluation of potential interethnic diversity, particularly in genetics, gene expression, and PK-/PD-related profiles to anti-TB drugs (Olafuyi et al., 2021). Moreover, discovered biomarkers should also be validated with large populations. In a recent metabolomics study from Indonesia, TB-DM patients were further characterized by lower levels of glycine, serine, threonine, and homoserine compared to TB patients and HC (Vrieling et al., 2019). This result was consistent with their previous publication focused on other ethnicities. Thus, these markers could be considered as potential POC biomarkers tests to classify TB-DM patients. Just as importantly, the assimilation and collection of multi-modal data, including multi-omics, from collaborative research also needs the assurance of data safety. Hence, trusted central centers should be established to train the models, build safe data storage, ensure data standardization, and finally distribute the models to collaborators for knowledge transfer and data sharing.
Our recent review paper has proposed a novel strategy by incorporating semi-automated TDM using MIPD as a pillar of TB treatment (Jayanti et al., 2022). MIPD-based TDM has three approaches for its implementation, which incorporate a mechanistic approach, physiologically based pharmacokinetic modeling and simulation (PBPK), a data-driven approach (population PK), and a combination of both. Thus far, the population PK model is the most common tool used in MIPD-based TDM to identify the source of IIV in PK parameters through the exploration of different available covariates (Polasek et al., 2019). These models are helpful in quantifying the characteristics of biomarker emergence and decline, their connection with PK or drug exposure, and the measurement of specific sources of IIV and patient-associated predictors (Vinnard et al., 2017; Aulin et al., 2021). Another important potential use of pharmacometrics models for biomarkers is clinical outcome relationships, which may use the biomarker results as the PD target (Thorsted et al., 2020; Aulin et al., 2021). Since the MIPD-based TDM models may facilitate mechanism-based integration, model-based approaches play a pivotal role in reaching this aim.
We have developed an informatics framework for the MIPD-based TDM algorithm. The algorithm allows us to monitor anti-TB drug concentrations in patients and suggests the optimal dose initiation and adjustment following the model-based estimation PK profile. Established collaboration with other high-burden TB countries allows the transfer of knowledge and technology to attain TB eradication through personalized medicine implementation as the end goal of the partnership. Our current models have been developed explicitly following specific ethnicities (Cho et al., 2021; Soedarsono et al., 2022), to ensure the PK estimation and significant covariates found are more precise and accurate. Considering only PK for personalized medicine might not be appropriate. As is widely known, PD parameters are essential to provide precise dose predictions (Thorsted et al., 2020). Nonetheless, this raises concern as the majority of patients would most likely produce no sputum before or after treatment. A previous study also found that the culture and smear test as a prognostic treatment outcome evaluation showed poor performance (Horne et al., 2010). Hence, it is about time to find the surrogate markers that can indicate early drug efficacy and accurately predict relapse and/or treatment failure which would significantly reduce the cost of TB treatment. Furthermore, even though the non-linear mixed-effect model could handle the sparse sampling approach at random-post dose points, samples taken near Cmax or distribution time of the drugs would be preferable (Free et al., 2011). Our current proposed strategy is to incorporate the data reflecting the immune profiles of TB patients into our well-developed algorithm of MIPD-based TDM, which is further illustrated in Figure 5. In order to achieve the aforementioned aims for this approach, a small sample size will not be sufficient. Considering the dynamics and kinetics changes of biomarkers throughout the infection and treatment phases, the novel discovered immune and other molecular biomarkers should be further validated in a large-scale cohort with the prospective observational design (Aulin et al., 2021; Bonaguro et al., 2022; Sanz Codina and Zeitlinger, 2022).
Figure 5 Integration of immunomics biosignature into semi-automated therapeutic drug monitoring application. Immune profile quantification in relation to a patient’s outcome and medical records are used to: (1) validate representative markers for TB diagnosis, outcome prediction, or susceptibility; (2) differentiate diagnosis of TB patients and outcome predictions; (3) provide initial optimal dose; (4) provide additional solid input for treatment monitoring; and (5) semi-automated TDM under population PK model evaluation. The quantified immune profile from each patient will be taken as part of comprehensive covariates evaluation during population PK model development. Our current semi-automated TDM algorithm takes PK results into account for each specific drug, immune markers (as both covariates and PD marker), ethnic differences, and clinical symptoms to provide optimal dose suggestions. Furthermore, the result of immune markers also can be provided in the TDM report to support the monitoring and evaluation phases. TDM, therapeutic drug monitoring; TB, tuberculosis; PK, pharmacokinetics; PD, pharmacodynamics; CL, clearance; Vd, volume of distribution; Ka, absorption rate; Tv, typical value.
In conclusion, TB management is not a new issue, but it could take a long time to accomplish the End TB Strategy’s mission, especially in developing countries and due to the pandemic. COVID-19 has suspended the global progress of TB control, highlighting the lack of accessibility and adaptability of current TB diagnosis and therapies. Immune profiling is emphasized in this paper as both a self-sufficient and an additional instrument to improve the existing TB control strategies in an unbiased manner. Our reviews summarized the relevance of immune biomarkers in TB management and characterized the integration of immunomics into the current TB roadmap of research and development. High-throughput immune profiling benefits not only the validation of new immune biomarkers for TB diagnosis, but also the outcome prediction, treatment monitoring, and anti-TB drug dose optimization, which will speed up TB control globally, and ensure “the world without TB” will not be a far off future.
Conceptualization, Y-SC, NL, and J-GS; Writing—Original Draft Preparation, VT, LD, RJ, HT, TH, NL, and J-GS; Writing—Review & Editing, VT, LD, RJ, HT, NL, Y-SC, and J-GS; Visualization, VT, LD, and RJ; Project Administration, NL; Supervision, Y-SC and J-GS; Funding Acquisition, J-GS. All authors have read and agreed to the published version of the manuscript.
This work was supported by the National Research Foundation of Korea (NRF) grant (No.2018R1A5A2021242) funded by the Korean government (MSIT).
Figures 1–5 were created using BioRender.com.
The authors declare that the research was conducted in the absence of any commercial or financial relationships that could be construed as a potential conflict of interest.
All claims expressed in this article are solely those of the authors and do not necessarily represent those of their affiliated organizations, or those of the publisher, the editors and the reviewers. Any product that may be evaluated in this article, or claim that may be made by its manufacturer, is not guaranteed or endorsed by the publisher.
Abraham, P. R., Devalraju, K. P., Jha, V., Valluri, V. L., Mukhopadhyay, S. (2018). PPE17 (Rv1168c) protein of mycobacterium tuberculosis detects individuals with latent TB infection. J. PloS One 13, e0207787. doi: 10.1371/journal.pone.0207787
Abu-Raya, B., Michalski, C., Sadarangani, M., Lavoie, P. M. (2020). Maternal immunological adaptation during normal pregnancy. J. Frontier In Immunol. 11, 575197. doi: 10.3389/fimmu.2020.575197
Acosta, J. N., Falcone, G. J., Rajpurkar, P., Topol, E. J. (2022). Multimodal biomedical AI. J. Nat. Medecine 28, 1773–1784. doi: 10.1038/s41591-022-01981-2
Adekambi, T., Ibegbu, C. C., Cagle, S., Kalokhe, A. S., Wang, Y. F., Hu, Y. (2015). Biomarkers on patient T cells diagnose active tuberculosis and monitor treatment response. Clin. Invest. 125, 1827–1838. doi: 10.1172/JCI77990
Ahamad, N., Gupta, S., Parashar, D. J. (2022). Using omics to study leprosy, tuberculosis, and other mycobacterial diseases. J Frontiers in Cellular Infection Microbiology 158. doi: 10.3389/fcimb.2022.792617
Ahmed, M. I., Ntinginya, N. E., Kibiki, G., Mtafya, B. A., Semvua, H., Mpagama, S. (2018). Phenotypic changes on mycobacterium tuberculosis-specific CD4 T cells as surrogate markers for tuberculosis treatment efficacy. Front. Immunol. 9, 2247. doi: 10.3389/fimmu.2018.02247
Ahsan, H. (2021). Monoplex and multiplex immunoassays: Approval, advancements, and alternatives. Comp. Clin. Path 1-13. doi: 10.1007/s00580-021-03302-4
Alffenaar, J.-W. C., Gumbo, T., Dooley, K. E., Peloquin, C. A., Mcilleron, H., Zagorski, A. (2020). Integrating pharmacokinetics and pharmacodynamics in operational research to end tuberculosis. J Clinical Infectious Diseases 70, 1774–1780. doi: 10.1093/cid/ciz942
Alsultan, A., Peloquin, C. A. (2014). Therapeutic drug monitoring in the treatment of tuberculosis: an update. J. Drugs 74, 839–854. doi: 10.1007/s40265-014-0222-8
Amelio, P., Portevin, D., Hella, J., Reither, K., Kamwela, L., Lweno, O. (2019). HIV Infection functionally impairs mycobacterium tuberculosis-specific CD4 and CD8 T-cell responses. Virol 93, e01728–e01718. doi: 10.1128/JVI.01728-18
Andersen, P., Munk, M., Pollock, J., Doherty, T. (2000). Specific immune-based diagnosis of tuberculosis. Lancet 356, 1099–1104. doi: 10.1016/S0140-6736(00)02742-2
Arend, S. M., Andersen, P., Van Meijgaarden, K. E., Skjøt, R. L., Subronto, Y. W., Van Dissel, J. T., et al. (2000). Detection of active tuberculosis infection by T cell responses to early-secreted antigenic target 6-kDa protein and culture filtrate protein 10. J The Journal of infectious diseases 181, 1850–1854. doi: 10.1086/315448
Aulin, L. B., De Lange, D. W., Saleh, M. A., Van Der Graaf, P. H., Völler, S., Van Hasselt, J. C. (2021). Biomarker-guided individualization of antibiotic therapy. J Clinical Pharmacology Therapeutics 110, 346–360. doi: 10.1002/cpt.2194
(2013). Overview of the immune system. Immune System Res. Available at: https://www.niaid.nih.gov/research/immune-response-features.
Ayelign, B., Negash, M., Genetu, M., Wondmagegn, T., Shibabaw, T. (2019). Immunological impacts of diabetes on the susceptibility of mycobacterium tuberculosis. J Journal of immunology research. doi: 10.1155/2019/6196532
Azzurri, A., Sow, O. Y., Amedei, A., Bah, B., Diallo, S., Peri, G., et al. (2005). IFN-γ-inducible protein 10 and pentraxin 3 plasma levels are tools for monitoring inflammation and disease activity in mycobacterium tuberculosis infection. J Microbes Infection 7, 1–8. doi: 10.1016/j.micinf.2004.09.004
Baatjies, L., Loxton, A. G., Williams, M. J. (2021). Host and bacterial iron homeostasis, an underexplored area in tuberculosis biomarker research. J Frontiers in Immunology 12. doi: 10.3389/fimmu.2021.742059
Bah, S. Y., Forster, T., Dickinson, P., Kampmann, B., Ghazal, P. J. (2018). Meta-analysis identification of highly robust and differential immune-metabolic signatures of systemic host response to acute and latent tuberculosis in children and adults. J Frontiers in genetics 9, 457. doi: 10.3389/fgene.2018.00457
Bai, X.-J., Li, H.-M., Yang, Y.-R., Zhang, J.-X., Liang, Y., Wu, X.-Q. (2018). Cytokine and soluble adhesion molecule profiles and biomarkers for treatment monitoring in re-treated smear-positive patients with pulmonary tuberculosis. J Cytokine 108, 9–16. doi: 10.1016/j.cyto.2018.03.009
Barberis, I., Bragazzi, N. L., Galluzzo, L., Martini, M. J. (2017). The history of tuberculosis: from the first historical records to the isolation of koch's bacillus. J Journal of preventive medicine hygiene 58.
Basingnaa, A., Antwi-Baffour, S., Nkansah, D. O., Afutu, E., Owusu, E. J. (2018). Plasma levels of cytokines (IL-10, IFN-γ and TNF-α) in multidrug resistant tuberculosis and drug responsive tuberculosis patients in Ghana. J Diseases 7, 2. doi: 10.3390/diseases7010002
Ben-Selma, W., Harizi, H., Boukadida, J. (2011). Immunochromatographic IgG/IgM test for rapid diagnosis of active tuberculosis. 18, 2090–2094. doi: 10.1128/CVI.05166-11
Ben Selma, W., Harizi, H., Marzouk, M., Ben Kahla, I., Ben Lazreg, F., Ferjeni, A. (2010). Rapid detection of immunoglobulin G against mycobacterium tuberculosis antigens by two commercial ELISA kits. J Clinical Vaccine immunology 14, 841–846.
Bentley-Hibbert, S. I., Quan, X., Newman, T., Huygen, K., Godfrey, H. (1999). Pathophysiology of antigen 85 in patients with active tuberculosis: Antigen 85 circulates as complexes with fibronectin and immunoglobulin G. J Infection Immunity 67, 581–588. doi: 10.1128/IAI.67.2.581-588.1999
Berry, M. P., Graham, C. M., Mcnab, F. W., Xu, Z., Bloch, S. A., Oni, T., et al. (2010). An interferon-inducible neutrophil-driven blood transcriptional signature in human tuberculosis. J Nature 466, 973–977. doi: 10.1038/nature09247
Bonaguro, L., Schulte-Schrepping, J., Ulas, T., Aschenbrenner, A. C., Beyer, M., Schultze, J. L. (2022). A guide to systems-level immunomics. J Nature Immunology 1–12. doi: 10.1038/s41590-022-01309-9
Boni, F. G., Hamdi, I., Koundi, L. M., Shrestha, K., Xie, J. (2022). Cytokine storm in tuberculosis and IL-6 involvement. J Infection, Genetics Evolution 97, 105166. doi: 10.1016/j.meegid.2021.105166
Bruns, H., Meinken, C., Schauenberg, P., Härter, G., Kern, P., Modlin, R. L., et al. (2009). Anti-TNF immunotherapy reduces CD8+ T cell–mediated antimicrobial activity against mycobacterium tuberculosis in humans. J The Journal of clinical investigation 119, 1167–1177. doi: 10.1172/JCI38482
Bulterys, M. A., Wagner, B., Redard-Jacot, M., Suresh, A., Pollock, N. R., Moreau, E., et al. (2019). Point-of-care urine LAM tests for tuberculosis diagnosis: A status update. J Journal of clinical medicine 9, 111. doi: 10.3390/jcm9010111
Buttle, T. S., Hummerstone, C. Y., Billahalli, T., Ward, R. J., Barnes, K. E., Marshall, N. J., et al. (2021). The monocyte-to-lymphocyte ratio: Sex-specific differences in the tuberculosis disease spectrum, diagnostic indices and defining normal ranges. J PloS one 16. doi: 10.1371/journal.pone.0247745
Caraux-Paz, P., Diamantis, S., De Wazières, B., Gallien, S. J. (2021). Tuberculosis in the elderly. J Journal of Clinical Medicine 10, 5888. doi: 10.3390/jcm10245888
Cattamanchi, A., Dowdy, D. W., Davis, J. L., Worodria, W., Yoo, S., Joloba, M., et al. (2009). Sensitivity of direct versus concentrated sputum smear microscopy in HIV-infected patients suspected of having pulmonary tuberculosis. J BMC Infectious Diseases 9, 1–9.
Chen, J., Raymond, K., Antimicrobials (2006). Roles of rifampicin in drug-drug interactions: Underlying molecular mechanisms involving the nuclear pregnane X receptor. J Annals of Clinical Microbiology Antimicrobials 5, 1–11. doi: 10.1186/1476-0711-5-3
Chiang, S. S., Park, S., White, E. I., Friedman, J. F., Cruz, A. T., Del Castillo, H., et al. (2020). Using changes in weight-for-age z score to predict effectiveness of childhood tuberculosis therapy. J Journal of the Pediatric Infectious Diseases Society 9, 150–158. doi: 10.1093/jpids/piy138
Cho, Y. S., Jang, T. W., Kim, H. J., Oh, J. Y., Lee, H. K., Park, H. K., et al. (2021). Isoniazid population pharmacokinetics and dose recommendation for Korean patients with tuberculosis based on target attainment analysis. J The Journal of Clinical Pharmacology 61, 1567–1578. doi: 10.1002/jcph.1931
Chowdhury, F., Williams, A., Johnson, P. J. (2009). Validation and comparison of two multiplex technologies, luminex® and mesoscale discovery, for human cytokine profiling. J Journal of immunological methods 340, 55–64. doi: 10.1016/j.jim.2008.10.002
Christensen, H., Hermann, M. J. (2012). Immunological response as a source to variability in drug metabolism and transport. J Frontiers in pharmacology 3, 8. doi: 10.3389/fphar.2012.00008
Chu, X., Zhang, B., Koeken, V. A., Gupta, M. K., Li, Y. (2021). Multi-omics approaches in immunological research. J Frontiers in Immunology 2312. doi: 10.3389/fimmu.2021.668045
Ciabattini, A., Nardini, C., Santoro, F., Garagnani, P., Franceschi, C., Medaglini, D. (2018). “Vaccination in the elderly: The challenge of immune changes with aging,” in Seminars in immunology (Elsevier), 40, 83–94.doi: 10.1016/j.smim.2018.10.010
Clifford, V., Zufferey, C., Street, A., Denholm, J., Tebruegge, M., Curtis, N. (2015). Cytokines for monitoring anti-tuberculous therapy: A systematic review. J Tuberculosis 95, 217–228. doi: 10.1016/j.tube.2015.01.003
D'amato, R. F., Wallman, A. A., Hochstein, L. H., Colaninno, P. M., Scardamaglia, M., Ardila, E., et al. (1995). Rapid diagnosis of pulmonary tuberculosis by using Roche AMPLICOR mycobacterium tuberculosis PCR test. J Journal of Clinical Microbiology 33, 1832–1834. doi: 10.1128/jcm.33.7.1832-1834.1995
De Andrade Júnior, D. R., dos Santos, S. A., de Castro, I., de Andrade, D. R. (2008). Correlation between serum tumor necrosis factor alpha levels and clinical severity of tuberculosis. 12, 226–233. doi: 10.1590/S1413-86702008000300013
Delanghe, J. R., Speeckaert, M. M. (2015). Translational research and biomarkers in neonatal sepsis. J Clinica chimica acta 451, 46–64. doi: 10.1016/j.cca.2015.01.031
Delhalle, S., Bode, S. F., Balling, R., Ollert, M., He, F. Q., Applications (2018). A roadmap towards personalized immunology. J NPJ systems biology applications 4, 1–14. doi: 10.1038/s41540-017-0045-9
De Sousa, K. P., Doolan, D. L. (2016). Immunomics: A 21st century approach to vaccine development for complex pathogens. J Parasitology 143, 236–244. doi: 10.1017/S0031182015001079
De Steenwinkel, J. E., De Knegt, G. J., Marian, T., Verbrugh, H. A., Hernandez-Pando, R., Leenen, P. J., et al. (2013). Relapse of tuberculosis versus primary tuberculosis; course, pathogenesis and therapy in mice. J Tuberculosis 93, 213–221. doi: 10.1016/j.tube.2012.11.006
Dheda, K., Schwander, S. K., Zhu, B., Van Zyl-Smit, R. N., Zhang, Y. (2010). The immunology of tuberculosis: From bench to bedside. J Respirology 15, 433–450. doi: 10.1111/j.1440-1843.2010.01739.x
Díaz, A., Bongiovanni, B., D’attilio, L., Santucci, N., Dídoli, G., Fernández, R. D. V., et al. (2017). The clinical recovery of tuberculosis patients undergoing specific treatment is associated with changes in the immune and neuroendocrine responses. J Pathogens Disease 75. doi: 10.1093/femspd/ftx087
Di Martino, M. T., Meschini, S., Scotlandi, K., Riganti, C., De Smaele, E., Zazzeroni, F., et al. (2020). From single gene analysis to single cell profiling: A new era for precision medicine. BioMed. Cent. doi: 10.1186/s13046-020-01549-3
Djoba Siawaya, J., Beyers, N., Van Helden, P., Walzl, G. (2009). Differential cytokine secretion and early treatment response in patients with pulmonary tuberculosis. J Clinical Experimental Immunology 156, 69–77. doi: 10.1111/j.1365-2249.2009.03875.x
Doolan, D. L. (2011). Plasmodium immunomics. J International journal for parasitology 41, 3–20. doi: 10.1016/j.ijpara.2010.08.002
Doolan, D. L., Apte, S. H., Proietti, C. (2014). Genome-based vaccine design: The promise for malaria and other infectious diseases. J International journal for parasitology 44, 901–913. doi: 10.1016/j.ijpara.2014.07.010
Duffy, F. J., Weiner Iii, J., Hansen, S., Tabb, D. L., Suliman, S., Thompson, E., et al. (2019). Immunometabolic signatures predict risk of progression to active tuberculosis and disease outcome. J Frontiers in immunology 10, 527. doi: 10.3389/fimmu.2019.00527
Eigenbrod, T., Bode, K. A., Dalpke, A. (2013). Early inhibition of IL-1β expression by IFN-γ is mediated by impaired binding of NF-κB to the IL-1β promoter but is independent of nitric oxide. J The Journal of Immunology 190, 6533–6541. doi: 10.4049/jimmunol.1300324
Ellington, A. A., Kullo, I. J., Bailey, K. R., Klee, G. G. (2010). Antibody-based protein multiplex platforms: Technical and operational challenges. J Clinical chemistry 56, 186–193. doi: 10.1373/clinchem.2009.127514
Elshal, M. F., Mccoy, J. (2006). Multiplex bead array assays: Performance evaluation and comparison of sensitivity to ELISA. J Methods 38, 317–323. doi: 10.1016/j.ymeth.2005.11.010
Estévez, O., Anibarro, L., Garet, E., Martínez, A., Pena, A., Barcia, L. (2020a). Multi-parameter flow cytometry immunophenotyping distinguishes different stages of tuberculosis infection. J Journal of Infection 81, 57–71. doi: 10.1016/j.jinf.2020.03.064
Estévez, O., Anibarro, L., Garet, E., Pallares, Á., Pena, A., Villaverde, C. (2020b). Identification of candidate host serum and saliva biomarkers for a better diagnosis of active and latent tuberculosis infection. J PloS one 15. doi: 10.1371/journal.pone.0235859
Etna, M. P., Giacomini, E., Severa, M., Coccia, E. M. (2014). “Pro-and anti-inflammatory cytokines in tuberculosis: A two-edged sword in TB pathogenesis,” in Seminars in immunology (Elsevier), 543–551. doi: 10.1016/j.smim.2014.09.011
Ferrian, S., Manca, C., Lubbe, S., Conradie, F., Ismail, N., Kaplan, G. (2017). A combination of baseline plasma immune markers can predict therapeutic response in multidrug resistant tuberculosis. J PloS one 12. doi: 10.1371/journal.pone.0176660
Fortes, A., Pereira, K., Antas, P., Franken, C., Dalcolmo, M., Ribeiro-Carvalho, M. (2005). Detection of in vitro interferon-γ and serum tumour necrosis factor-α in multidrug-resistant tuberculosis patients. J Clinical Experimental Immunology 141, 541–548. doi: 10.1111/j.1365-2249.2005.02872.x
Franceschi, C., Garagnani, P., Parini, P., Giuliani, C., Santoro, A. (2018). Inflammaging: A new immune–metabolic viewpoint for age-related diseases. J Nature Reviews Endocrinology 14, 576–590. doi: 10.1038/s41574-018-0059-4
Free, E. E., Bergesch, B. A., Arthur Peloquin, C. (2011). Population pharmacokinetics and its role in anti-tuberculosis drug development and optimization of treatment. J Current pharmaceutical design 17, 2889–2899. doi: 10.2174/138161211797470246
Fukutomi, Y., Maeda, Y., Makino, M. (2011). Apoptosis-inducing activity of clofazimine in macrophages. J Antimicrobial agents chemotherapy 55, 4000–4005. doi: 10.1128/AAC.00434-11
Gausi, K., Wiesner, L., Norman, J., Wallis, C. L., Onyango-Makumbi, C., Chipato, T., et al. (2021). Pharmacokinetics and drug-drug interactions of isoniazid and efavirenz in pregnant women living with HIV in high TB incidence settings: Importance of genotyping. J Clinical Pharmacology Therapeutics 109, 1034–1044. doi: 10.1002/cpt.2044
Geffner, L., Yokobori, N., Basile, J., Schierloh, P., Balboa, L., Romero, M. M. (2009). Patients with multidrug-resistant tuberculosis display impaired Th1 responses and enhanced regulatory T-cell levels in response to an outbreak of multidrug-resistant mycobacterium tuberculosis m and Ra strains. J Infection immunity 77, 5025–5034. doi: 10.1128/IAI.00224-09
Geremew, D., Melku, M., Endalamaw, A., Woldu, B., Fasil, A., Negash, M. (2020). Tuberculosis and its association with CD4+ T cell count among adult HIV positive patients in Ethiopian settings: A systematic review and meta-analysis. J BMC infectious diseases 20, 1–10. doi: 10.1186/s12879-020-05040-4
Getahun, H., Gunneberg, C., Granich, R., Nunn, P. (2010). HIV Infection–associated tuberculosis: The epidemiology and the response. J Clinical Infectious Diseases 50, S201–S207. doi: 10.1086/651492
Giraud-Gatineau, A., Coya, J. M., Maure, A., Biton, A., Thomson, M., Bernard, E. M. (2020). The antibiotic bedaquiline activates host macrophage innate immune resistance to bacterial infection. J Elife 9. doi: 10.7554/eLife.55692
Goletti, D., Lee, M. R., Wang, J. Y., Walter, N., Ottenhoff, T. H. (2018). Update on tuberculosis biomarkers: From correlates of risk, to correlates of active disease and of cure from disease. J Respirology 23, 455–466. doi: 10.1111/resp.13272
Goletti, D., Petruccioli, E., Joosten, S. A., Ottenhoff, T. H. (2016). Tuberculosis biomarkers: From diagnosis to protection. J Infectious disease reports 8, 6568. doi: 10.4081/idr.2016.6568
Gonzalez-Juarrero, M., Hattle, J. M., Izzo, A., Junqueira-Kipnis, A. P., Shim, T. S., Trapnell, B. C., et al. (2005). Disruption of granulocyte macrophage-colony stimulating factor production in the lungs severely affects the ability of mice to control mycobacterium tuberculosis infection. J Journal of leukocyte biology 77, 914–922. doi: 10.1189/jlb.1204723
Gry, M., Rimini, R., Strömberg, S., Asplund, A., Pontén, F., Uhlén, M. (2009). Correlations between RNA and protein expression profiles in 23 human cell lines. J BMC genomics 10, 1–14. doi: 10.1186/1471-2164-10-365
Gunasekera, K. S., Vonasek, B., Oliwa, J., Triasih, R., Lancioni, C., Graham, S. M. (2022). Diagnostic challenges in childhood pulmonary tuberculosis–optimizing the clinical approach. J Pathogens 11, 382. doi: 10.3390/pathogens11040382
Günther, A., Becker, M., Göpfert, J., Joos, T., Schneiderhan-Marra, N. (2020). Comparison of bead-based fluorescence versus planar electrochemiluminescence multiplex immunoassays for measuring cytokines in human plasma. J Frontiers in immunology 11, 572634. doi: 10.3389/fimmu.2020.572634
Gupta, S., Grieco, M. H., Siegel, I. J. (1975). Suppression of T-lymphocyte rosettes by rifampin: Studies in normals and patients with tuberculosis. J Annals of internal medicine 82, 484–488. doi: 10.7326/0003-4819-82-4-484
Harari, A., Rozot, V., Enders, F. B., Perreau, M., Stalder, J. M., Nicod, L. P. (2011). Dominant TNF-α+ mycobacterium tuberculosis–specific CD4+ T cell responses discriminate between latent infection and active disease. J Nature medicine 17, 372–376. doi: 10.1038/nm.2299
Hartl, D., De Luca, V., Kostikova, A., Laramie, J., Kennedy, S., Ferrero, E. (2021). Translational precision medicine: An industry perspective. J Journal of translational medicine 19, 1–14. doi: 10.1186/s12967-021-02910-6
Hasin, Y., Seldin, M., Lusis, A. J. (2017). Multi-omics approaches to disease. 18, 1–15. doi: 10.1186/s13059-017-1215-1
Hendon-Dunn, C. L., Doris, K. S., Thomas, S. R., Allnutt, J. C., Marriott, A. A. N., Hatch, K. A. (2016). A flow cytometry method for rapidly assessing mycobacterium tuberculosis responses to antibiotics with different modes of action. J Antimicrobial agents chemotherapy 60, 3869–3883. doi: 10.1128/AAC.02712-15
Hensel, R., Kempker, R., Tapia, J., Oladele, A., Blumberg, H., Magee, M. J. (2016). Increased risk of latent tuberculous infection among persons with pre-diabetes and diabetes mellitus. J The International Journal of Tuberculosis Lung Disease 20, 71–78. doi: 10.5588/ijtld.15.0457
Herrera, M. T., Guzmán-Beltrán, S., Bobadilla, K., Santos-Mendoza, T., Flores-Valdez, M. A., Gutiérrez-González, L. H. (2022). Human pulmonary tuberculosis: Understanding the immune response in the bronchoalveolar system. J Biomolecules 12, 1148. doi: 10.3390/biom12081148
Herrera, V., Perry, S., Parsonnet, J., Banaei, N. J. (2011). Clinical application and limitations of interferon-γ release assays for the diagnosis of latent tuberculosis infection. J Clinical infectious diseases 52, 1031–1037. doi: 10.1093/cid/cir068
Horita, Y., Doi, N. (2014). Comparative study of the effects of antituberculosis drugs and antiretroviral drugs on cytochrome P450 3A4 and p-glycoprotein. J Antimicrobial agents chemotherapy 58, 3168–31761. doi: 10.1128/AAC.02278-13
Horne, D. J., Royce, S. E., Gooze, L., Narita, M., Hopewell, P. C., Nahid, P. (2010). Sputum monitoring during tuberculosis treatment for predicting outcome: Systematic review and meta-analysis. J The Lancet infectious diseases 10, 387–394. doi: 10.1016/S1473-3099(10)70071-2
Hur, Y.-G., Gorak-Stolinska, P., Ben-Smith, A., Lalor, M. K., Chaguluka, S., Dacombe, R. (2013). Combination of cytokine responses indicative of latent TB and active TB in Malawian adults. J PloS one 8. doi: 10.1371/journal.pone.0079742
Indrati, A. R., Sumarpo, A., Atmadja, P., Wisesa, R. R., Ghozali, M., Judistiani, R. T. D. (2022). Exploring alternative cytokines as potential biomarkers for latent tuberculosis infection in pregnant women. J Plos one 17. doi: 10.1371/journal.pone.0270552
Jacobsen, M., Repsilber, D., Gutschmidt, A., Neher, A., Feldmann, K., Mollenkopf, H. J. (2007). Candidate biomarkers for discrimination between infection and disease caused by mycobacterium tuberculosis. J Journal of molecular medicine 85, 613–621. doi: 10.1007/s00109-007-0157-6
Jacobs, R., Malherbe, S., Loxton, A. G., Stanley, K., Van Der Spuy, G., Walzl, G. (2016). Identification of novel host biomarkers in plasma as candidates for the immunodiagnosis of tuberculosis disease and monitoring of tuberculosis treatment response. J Oncotarget 7, 57581. doi: 10.18632/oncotarget.11420
Jakhar, S., Bitzer, A. A., Stromberg, L. R., Mukundan, H. J. (2020). Pediatric tuberculosis: The impact of “omics“ on diagnostics development. J International journal of molecular sciences 21, 6979. doi: 10.3390/ijms21196979
Jayanti, R. P., Long, N. P., Phat, N. K., Cho, Y.-S., Shin, J.-G. (2022). Semi-automated therapeutic drug monitoring as a pillar toward personalized medicine for tuberculosis management. J Pharmaceutics 14, 990. doi: 10.3390/pharmaceutics14050990
Kashyap, R. S., Dobos, K. M., Belisle, J. T., Purohit, H. J., Chandak, N. H., Taori, G. M. (2005). Demonstration of components of antigen 85 complex in cerebrospinal fluid of tuberculous meningitis patients. J Clinical Vaccine Immunology 12, 752–758. doi: 10.1128/CDLI.12.6.752-758.2005
Kashyap, R. S., Rajan, A. N., Ramteke, S. S., Agrawal, V. S., Kelkar, S. S., Purohit, H. J. (2007). Diagnosis of tuberculosis in an Indian population by an indirect ELISA protocol based on detection of antigen 85 complex: A prospective cohort study. J BMC infectious diseases 7, 1–6. doi: 10.1186/1471-2334-7-74
Kaul, S., Nair, V., Birla, S., Dhawan, S., Rathore, S., Khanna, V., et al. (2022). Latent tuberculosis infection diagnosis among household contacts in a high tuberculosis-burden area: A comparison between transcript signature and interferon gamma release assay. J Microbiology Spectrum 10. doi: 10.1128/spectrum.02445-21
Khan, N., Downey, J., Sanz, J., Kaufmann, E., Blankenhaus, B., Pacis, A., et al. (2020). M. tuberculosis reprograms hematopoietic stem cells to limit myelopoiesis and impair trained immunity. J Cell 183, 752–770. e22. doi: 10.1016/j.cell.2020.09.062
Kim, J., Tran, V. T., Oh, S., Jang, M., Lee, D. K., Hong, J. C., et al. (2021). Clinical trial: Magnetoplasmonic ELISA for urine-based active tuberculosis detection and anti-tuberculosis therapy monitoring. J ACS central science 7, 1898–1907. doi: 10.1021/acscentsci.1c00948
Kumar, N. P., Hissar, S., Thiruvengadam, K., Banurekha, V. V., Balaji, S., Elilarasi, S. (2021a). Plasma chemokines as immune biomarkers for diagnosis of pediatric tuberculosis. J Immunology 21, 1–11. doi: 10.1186/s12879-021-06749-6
Kumar, N. P., Moideen, K., Banurekha, V. V., Nair, D., Babu, S. (2019a). “Plasma proinflammatory cytokines are markers of disease severity and bacterial burden in pulmonary tuberculosis,” in Open forum infectious diseases (US: Oxford University Press), ofz257. doi: 10.1093/ofid/ofz257
Kumar, N. P., Moideen, K., Nancy, A., Viswanathan, V., Shruthi, B. S., Sivakumar, S. (2019b). Plasma chemokines are biomarkers of disease severity, higher bacterial burden and delayed sputum culture conversion in pulmonary tuberculosis. J Clinical Infectious Diseases 9, 1–8. doi: 10.1038/s41598-019-54803-w
Kumar, N. P., Moideen, K., Nancy, A., Viswanathan, V., Shruthi, B. S., Sivakumar, S. (2020). Heterogeneity in the cytokine profile of tuberculosis–diabetes co-morbidity. J Cytokine 125, 154824. doi: 10.1016/j.cyto.2019.154824
Kumar, N. P., Moideen, K., Nancy, A., Viswanathan, V., Thiruvengadam, K., Nair, D. (2021b). Plasma chemokines are baseline predictors of unfavorable treatment outcomes in pulmonary tuberculosis. J Scientific Reports 73, e3419–e3427. doi: 10.1093/cid/ciaa1104
Kumar Nathella, P., Babu, S. (2017). Influence of diabetes mellitus on immunity to human tuberculosis. 152, 13–24. doi: 10.1111/imm.12762
Kumar Verma, R., Jain, A. (2007). Retracted: Antibodies to mycobacterial antigens for diagnosis of tuberculosis. J FEMS Immunology Medical Microbiology 51, 453–461. doi: 10.1111/j.1574-695X.2007.00302.x
Kwak, N., Choi, S. M., Lee, J., Park, Y. S., Lee, C.-H., Lee, S.-M., et al. (2013). Diagnostic accuracy and turnaround time of the xpert MTB/RIF assay in routine clinical practice. J PloS one 8. doi: 10.1371/journal.pone.0077456
La Manna, M. P., Orlando, V., Dieli, F., Di Carlo, P., Cascio, A., Cuzzi, G., et al. (2017). Quantitative and qualitative profiles of circulating monocytes may help identifying tuberculosis infection and disease stages. J PloS one 12. doi: 10.1371/journal.pone.0171358
La Manna, M. P., Orlando, V., Li Donni, P., Sireci, G., Di Carlo, P., Cascio, A., et al. (2018). Identification of plasma biomarkers for discrimination between tuberculosis infection/disease and pulmonary non tuberculosis disease. J PLoS One 13. doi: 10.1371/journal.pone.0192664
La Manna, M. P., Orlando, V., Paraboschi, E. M., Tamburini, B., Di Carlo, P., Cascio, A. (2019). Mycobacterium tuberculosis drives expansion of low-density neutrophils equipped with regulatory activities. J Frontiers in Immunology 10, 2761. doi: 10.3389/fimmu.2019.02761
Lange, C., Aarnoutse, R., Chesov, D., Van Crevel, R., Gillespie, S. H., Grobbel, H.-P. (2020). Perspective for precision medicine for tuberculosis. J Frontiers in immunology 11, 566608. doi: 10.3389/fimmu.2020.566608
Lee, J. Y., Diseases, R. (2015). Diagnosis and treatment of extrapulmonary tuberculosis. J Tuberculosis respiratory diseases 78, 47–55. doi: 10.4046/trd.2015.78.2.47
Lee, J.-S., Song, C.-H., Kim, C.-H., Kong, S.-J., Shon, M.-H., Kim, H.-J. (2002). Profiles of IFN-γ and its regulatory cytokines (IL-12, IL-18 and IL-10) in peripheral blood mononuclear cells from patients with multidrug-resistant tuberculosis. J Clinical Experimental Immunology 128, 516–524. doi: 10.1046/j.1365-2249.2002.01858.x
Lin, Y.-H., Chen, C.-P., Chen, P.-Y., Huang, J.-C., Ho, C., Weng, H.-H., et al. (2015). Screening for pulmonary tuberculosis in type 2 diabetes elderly: A cross-sectional study in a community hospital. 15, 1–8. doi: 10.1186/1471-2458-15-3
Lin, P. L., Flynn, J. L. (2015). “CD8 T cells and mycobacterium tuberculosis infection,” in Seminars in immunopathology (Springer), 239–249. doi: 10.1007/s00281-015-0490-8
Liu, Q. Y., Han, F., Pan, L. P., Jia, H. Y., Li, Q., Zhang, Z. D. (2018). Inflammation responses in patients with pulmonary tuberculosis in an intensive care unit. J Experimental therapeutic medicine 15, 2719–2726. doi: 10.3892/etm.2018.5775
Long, N. P., Anh, N. K., Yen, N. T. H., Phat, N. K., Park, S., Thu, V. T. A. (2022). Comprehensive lipid and lipid-related gene investigations of host immune responses to characterize metabolism-centric biomarkers for pulmonary tuberculosis. J Scientific reports 12, 1–14. doi: 10.1038/s41598-022-17521-4
Luo, J., Zhang, M., Yan, B., Li, F., Guan, S., Chang, K. (2019). Diagnostic performance of plasma cytokine biosignature combination and MCP-1 as individual biomarkers for differentiating stages mycobacterium tuberculosis infection. J Journal of Infection 78, 281–291. doi: 10.1016/j.jinf.2018.10.017
Luo, L., Zhu, L., Yue, J., Liu, J., Liu, G., Zhang, X. (2017). Antigens Rv0310c and Rv1255c are promising novel biomarkers for the diagnosis of mycobacterium tuberculosis infection. J Emerging Microbes Infections 6, 1–8. doi: 10.1038/emi.2017.54
Maekura, R., Kitada, S., Osada-Oka, M., Tateishi, Y., Ozeki, Y., Fujicawa, T. (2019). Serum antibody profiles in individuals with latent mycobacterium tuberculosis infection. J Microbiology immunology 63, 130–138. doi: 10.1111/1348-0421.12674
Manca, C., Koo, M.-S., Peixoto, B., Fallows, D., Kaplan, G., Subbian, S. (2013). Host targeted activity of pyrazinamide in mycobacterium tuberculosis infection. J PloS one 8. doi: 10.1371/journal.pone.0074082
Martinez, N., Kornfeld, H. (2014). Diabetes and immunity to tuberculosis. J European journal of immunology 44, 617–626. doi: 10.1002/eji.201344301
Martín, S., Pérez, A., Aldecoa, C. (2017). Sepsis and immunosenescence in the elderly patient: a review. J Frontiers in medicine 4, 20. doi: 10.3389/fmed.2017.00020
Mayo, P. R., Skeith, K., Russell, A. S., Jamali, F. (2000). Decreased dromotropic response to verapamil despite pronounced increased drug concentration in rheumatoid arthritis. J British journal of clinical pharmacology, 50, 605–613. doi: 10.1046/j.1365-2125.2000.00314.x
Méndez-Samperio, P. (2010). Role of interleukin-12 family cytokines in the cellular response to mycobacterial disease. J International Journal of Infectious Diseases 14, e366–e371. doi: 10.1016/j.ijid.2009.06.022
Mensah, G. I., Boakye, A. N., Basingnaa, A., Owusu, E., Antwi-Baffour, S., Ofori, M. F. (2021). Identification of serum cytokine biomarkers associated with multidrug resistant tuberculosis (MDR-TB). J Immuno 1, 400–409. doi: 10.3390/immuno1040028
Millington, K. A., Gooding, S., Hinks, T. S., Reynolds, D., Lalvani, A. (2010). Mycobacterium tuberculosis-specific cellular immune profiles suggest bacillary persistence decades after spontaneous cure in untreated tuberculosis. J The Journal of infectious diseases, 202, 1685–16849. doi: 10.1086/656772
Mlambo, G., Sigola, L. (2003). Rifampicin and dexamethasone have similar effects on macrophage phagocytosis of zymosan, but differ in their effects on nitrite and TNF-α production. J International immunopharmacology 3, 513–522. doi: 10.1016/S1567-5769(03)00022-5
Moffatt, J. H., Harper, M., Boyce, J. D. (2019). Mechanisms of polymyxin resistance. J Polymyxin Antibiotics: From Laboratory Bench to Bedside 55–71. doi: 10.1007/978-3-030-16373-0_5
Moideen, K., Kumar, N. P., Bethunaickan, R., Banurekha, V. V., Nair, D., Babu, S. (2020). Heightened systemic levels of anti-inflammatory cytokines in pulmonary tuberculosis and alterations following anti-tuberculosis treatment. J Cytokine 127, 154929. doi: 10.1016/j.cyto.2019.154929
Mutavhatsindi, H., Van Der Spuy, G. D., Malherbe, S. T., Sutherland, J. S., Geluk, A., Mayanja-Kizza, H. (2021). Validation and optimization of host immunological bio-signatures for a point-of-care test for TB disease. J Frontiers in immunology 12, 607827. doi: 10.3389/fimmu.2021.607827
Nahid, P., Dorman, S. E., Alipanah, N., Barry, P. M., Brozek, J. L., Cattamanchi, A. (2016). Official American thoracic society/centers for disease control and prevention/infectious diseases society of America clinical practice guidelines: Treatment of drug-susceptible tuberculosis. J Clinical Infectious Diseases 63, e147–e195. doi: 10.1093/cid/ciw376
Naidoo, K., Yende-Zuma, N., Padayatchi, N., Naidoo, K., Jithoo, N., Nair, G. (2012). The immune reconstitution inflammatory syndrome after antiretroviral therapy initiation in patients with tuberculosis: Findings from the SAPiT trial. J Annals of internal medicine 157, 313–324. doi: 10.7326/0003-4819-157-5-201209040-00004
Naik, S., Alexander, M., Kumar, P., Kulkarni, V., Deshpande, P., Yadana, S. (2021). Systemic inflammation in pregnant women with latent tuberculosis infection. J Frontiers in immunology, 11, 587617. doi: 10.3389/fimmu.2020.587617
Nandi, B., Behar, S. M. (2011). Regulation of neutrophils by interferon-γ limits lung inflammation during tuberculosis infection. J Journal of Experimental Medicine 208, 2251–2262. doi: 10.1084/jem.20110919
Naranbhai, V., Kim, S., Fletcher, H., Cotton, M. F., Violari, A., Mitchell, C. (2014). The association between the ratio of monocytes: Lymphocytes at age 3 months and risk of tuberculosis (TB) in the first two years of life. J BMC medicine 12, 1–6. doi: 10.1186/s12916-014-0120-7
Netea, M. G., Joosten, L. A., Li, Y., Kumar, V., Oosting, M., Smeekens, S., et al. (2016). Understanding human immune function using the resources from the human functional genomics project. J Nature medicine 22, 831–833. doi: 10.1038/nm.4140
Newton, S. M., Brent, A. J., Anderson, S., Whittaker, E., Kampmann, B. (2008). Paediatric tuberculosis. J The Lancet infectious diseases 8, 498–510. doi: 10.1016/S1473-3099(08)70182-8
Newton, K., Dixit, V. (2012). Signaling in innate immunity and inflammation. J Cold Spring Harbor perspectives in biology 4, a006049. doi: 10.1101/cshperspect.a006049
Niazi, A. K., Kalra, S. (2012). Diabetes and tuberculosis: a review of the role of optimal glycemic control. J Journal of Diabetes Metabolic Disorders 11, 1–4. doi: 10.1186/2251-6581-11-28
Nikolova, M., Markova, R., Drenska, R., Muhtarova, M., Todorova, Y., Dimitrov, V. (2013). Antigen-specific CD4-and CD8-positive signatures in different phases of mycobacterium tuberculosis infection. J Diagnostic microbiology infectious disease 75, 277–281. doi: 10.1016/j.diagmicrobio.2012.11.023
Nishida, N., Huang, Z.-L., Mikuni, N., Miura, Y., Urade, Y., Hashimoto, N. (2007). Deep brain stimulation of the posterior hypothalamus activates the histaminergic system to exert antiepileptic effect in rat pentylenetetrazol model. J Experimental neurology 205, 132–144. doi: 10.1016/j.expneurol.2007.01.021
Ntoumi, F., Petersen, E., Mwaba, P., Aklillu, E., Mfinanga, S., Yeboah-Manu, D., et al. (2022). Blue skies research is essential for ending the tuberculosis pandemic and advancing a personalized medicine approach for holistic management of respiratory tract infections. J International Journal of Infectious Diseases 124, S69–S74. doi: 10.1016/j.ijid.2022.03.012
Okoye, A. A., Picker, L. (2013). CD 4+ T-cell depletion in HIV infection: mechanisms of immunological failure. J Immunological reviews 25454–64. doi: 10.1111/imr.12066
Olafuyi, O., Parekh, N., Wright, J., Koenig, J. (2021). Inter-ethnic differences in pharmacokinetics–is there more that unites than divides? J Pharmacology research perspectives 9. doi: 10.1002/prp2.890
ORGANIZATION, W. H (2011). Use of tuberculosis interferon-gamma release assays (IGRAs) in low-and middle-income countries: Policy statement.
ORGANIZATION, W. H (2014). Meeting report: High-priority target product profiles for new tuberculosis diagnostics: Report of a consensus meeting 11–16.
ORGANIZATION, W. H (2015). Guideline on when to start antiretroviral therapy and on pre-exposure prophylaxis for HIV World Health Organization.
ORGANIZATION, W. H (2020). WHO consolidated guidelines on tuberculosis. module 3: Diagnosis-rapid diagnostics for tuberculosis detection, world health organization.
Ota, M., Fujio, K. (2021). Multi-omics approach to precision medicine for immune-mediated diseases. J Inflammation Regeneration 41, 1–6. doi: 10.1186/s41232-021-00173-8
Pai, M., Denkinger, C. M., Kik, S. V., Rangaka, M. X., Zwerling, A., Oxlade, O., et al. (2014). Gamma interferon release assays for detection of mycobacterium tuberculosis infection. J Clinical microbiology reviews 27, 3–20. doi: 10.1128/CMR.00034-13
Park, H.-E., Lee, W., Shin, M.-K., Shin, S. (2021). Understanding the reciprocal interplay between antibiotics and host immune system: How can we improve the anti-mycobacterial activity of current drugs to better control tuberculosis? J Frontiers in Immunology 12, 2539. doi: 10.3389/fimmu.2021.703060
Patel, N. R., Zhu, J., Tachado, S. D., Zhang, J., Wan, Z., Saukkonen, J. (2007). HIV Impairs TNF-α mediated macrophage apoptotic response to mycobacterium tuberculosis. J The Journal of Immunology 179, 6973–6980. doi: 10.4049/jimmunol.179.10.6973
Perumal, P., Abdullatif, M. B., Garlant, H. N., Honeyborne, I., Lipman, M., Mchugh, T. D. (2021). Validation of differentially expressed immune biomarkers in latent and active tuberculosis by real-time PCR. J Frontiers in immunology 3834. doi: 10.3389/fimmu.2020.612564
Petersen, E., Al-Abri, S., Chakaya, J., Goletti, D., Parolina, L., Wejse, C. (2022). World TB day 2022: Revamping and reshaping global TB control programs by advancing lessons learnt from the COVID-19 pandemic. J International Journal of Infectious Diseases. doi: 10.1016/j.ijid.2022.02.057
Phalane, K. G., Kriel, M., Loxton, A. G., Menezes, A., Stanley, K., Van Der Spuy, G. D. (2013). Differential expression of host biomarkers in saliva and serum samples from individuals with suspected pulmonary tuberculosis J Mediators of inflammation. doi: 10.1155/2013/981984
Pierneef, L., Van Hooij, A., De Jong, D., Fat, E. M., Van Meijgaarden, K. E., Petruccioli, E. (2022). Host biomarker-based quantitative rapid tests for detection and treatment monitoring of tuberculosis and COVID-19. J Iscience 105873. doi: 10.1016/j.isci.2022.105873
Pitaloka, D. A. E., Syamsunarno, M., Abdulah, R., Chaidir, L., Resistance, D. (2022). Omics biomarkers for monitoring tuberculosis treatment: A mini-review of recent insights and future approaches. J Infection Drug Resistance 15, 2703.
Polasek, T. M., Rostami-Hodjegan, A., Yim, D.-S., Jamei, M., Lee, H., Kimko, H., et al. (2019). “What does it take to make model-informed precision dosing common practice?,” in Report from the 1st Asian symposium on precision dosing (Busan, South Korea: Springer). doi: 10.1208/s12248-018-0286-6
Pollock, K. M., Whitworth, H. S., Montamat-Sicotte, D. J., Grass, L., Cooke, G. S., Kapembwa, M. S., et al. (2013). T-Cell immunophenotyping distinguishes active from latent tuberculosis. J The Journal of infectious diseases 208, 952–968. doi: 10.1093/infdis/jit265
Prezzemolo, T., Guggino, G., La Manna, M. P., Di Liberto, D., Dieli, F., Caccamo, N. (2014). Functional signatures of human CD4 and CD8 T cell responses to mycobacterium tuberculosis. J Frontiers in immunology 5, 180. doi: 10.3389/fimmu.2014.00180
Radke, C., Horn, D., Lanckohr, C., Ellger, B., Meyer, M., Eissing, T., et al. (2017). Development of a physiologically based pharmacokinetic modelling approach to predict the pharmacokinetics of vancomycin in critically ill septic patients. J Clinical pharmacokinetics, 56, 759–779. doi: 10.1007/s40262-016-0475-3
Raja, A., Ranganathan, U. D., Bethunaickan, R. (2008). Improved diagnosis of pulmonary tuberculosis by detection of antibodies against multiple mycobacterium tuberculosis antigens. J Diagnostic microbiology infectious disease 60, 361–368. doi: 10.1016/j.diagmicrobio.2007.11.006
Rambaran, S., Naidoo, K., Lewis, L., Hassan-Moosa, R., Govender, D., Samsunder, N. (2021). Effect of inflammatory cytokines/chemokines on pulmonary tuberculosis culture conversion and disease severity in HIV-infected and-uninfected individuals from south Africa. J Frontiers in immunology 12, 641065. doi: 10.3389/fimmu.2021.641065
Raposo-Garcia, S., Guerra-Laso, J. M., Garcia-Garcia, S., Juan-Garcia, J., Lopez-Fidalgo, E., Diez-Tascon (2017). Immunological response to mycobacterium tuberculosis infection in blood from type 2 diabetes patients. J Immunology letters 186, 41–45. doi: 10.1016/j.imlet.2017.03.017
Rausch, A., Heßmann, M., Hölscher, A., Schreiber, T., Bulfone-Paus, S., Ehlers, S. (2006). Interleukin-15 mediates protection against experimental tuberculosis: A role for NKG2D-dependent effector mechanisms of CD8+ T cells. J European journal of immunology 36, 1156–1167. doi: 10.1002/eji.200535290
Ravimohan, S., Tamuhla, N., Steenhoff, A. P., Letlhogile, R., Nfanyana, K., Bellamy, S. L. (2015). Immunological profiling of tuberculosis-associated immune reconstitution inflammatory syndrome and non-immune reconstitution inflammatory syndrome death in HIV-infected adults with pulmonary tuberculosis starting antiretroviral therapy: a prospective observational cohort study. J The Lancet Infectious diseases 15, 429–438. doi: 10.1016/S1473-3099(15)70008-3
Reither, K., Saathoff, E., Jung, J., Minja, L. T., Kroidl, I., Saad, E., et al. (2009). Low sensitivity of a urine LAM-ELISA in the diagnosis of pulmonary tuberculosis. J BMC infectious diseases 9, 1–10. doi: 10.1186/1471-2334-9-141
Riou, C., Perez Peixoto, B., Roberts, L., Ronacher, K., Walzl, G., Manca, C. (2012). Effect of standard tuberculosis treatment on plasma cytokine levels in patients with active pulmonary tuberculosis. J PloS one 7. doi: 10.1371/journal.pone.0036886
Ronacher, K., Chegou, N. N., Kleynhans, L., Siawaya, J. F. D., Du Plessis, N., Loxton, A. G., et al. (2019). Distinct serum biosignatures are associated with different tuberculosis treatment outcomes. J Tuberculosis 118, 101859. doi: 10.1016/j.tube.2019.101859
Sacks, G. P., Studena, K., Sargent, I. L., Redman, C. W. (1998). Normal pregnancy and preeclampsia both produce inflammatory changes in peripheral blood leukocytes akin to those of sepsis. J American journal of obstetrics gynecology 179, 80–86. doi: 10.1016/S0002-9378(98)70254-6
Sanz Codina, M., Zeitlinger, M. (2022). Biomarkers predicting tissue pharmacokinetics of antimicrobials in sepsis: A review. J Clinical Pharmacokinetics 1–25. doi: 10.1007/s40262-021-01102-1
Shakhnovich, V. (2018). It's time to reverse our thinking: the reverse translation research paradigm. J Clinical translational science 11, 98. doi: 10.1111/cts.12538
Sharma, A. A., Jen, R., Butler, A., Lavoie, P. (2012). The developing human preterm neonatal immune system: A case for more research in this area. J Clinical Immunology 145, 61–68. doi: 10.1016/j.clim.2012.08.006
Sharma, S. K., Vashishtha, R., Chauhan, L., Sreenivas, V., Seth, D. (2017). Comparison of TST and IGRA in diagnosis of latent tuberculosis infection in a high TB-burden setting. J PloS one 12. doi: 10.1371/journal.pone.0169539
Sigal, G., Segal, M., Mathew, A., Jarlsberg, L., Wang, M., Barbero, S., et al. (2017). Biomarkers of tuberculosis severity and treatment effect: A directed screen of 70 host markers in a randomized clinical trial. J EBioMedicine 25, 112–121. doi: 10.1016/j.ebiom.2017.10.018
Sivro, A., Mckinnon, L. R., Yende-Zuma, N., Gengiah, S., Samsunder, N., Abdool Karim, S. S. (2017). Plasma cytokine predictors of tuberculosis recurrence in antiretroviral-treated human immunodeficiency virus-infected individuals from Durban, south Africa. J Clinical Infectious Diseases 65, 819–826. doi: 10.1093/cid/cix357
Soedarsono, S., Jayanti, R. P., Mertaniasih, N. M., Kusmiati, T., Permatasari, A., Indrawanto, D. W. (2022). Development of population pharmacokinetics model of isoniazid in Indonesian patients with tuberculosis. J International Journal of Infectious Diseases 117, 8–14. doi: 10.1016/j.ijid.2022.01.003
Sourial, S., Marcusson-Ståhl, M., Cederbrant, K. (2009). Meso scale discovery and luminex comparative analysis of calbindin D28K. J Journal of Biomedicine Biotechnology. doi: 10.1155/2009/187426
Spindel, S., Sapsford, K. E. (2014). Evaluation of optical detection platforms for multiplexed detection of proteins and the need for point-of-care biosensors for clinical use. J Sensors 14, 22313–22341. doi: 10.3390/s141222313
Srivastava, S., Pasipanodya, J. G., Meek, C., Leff, R., Gumbo, T. (2011). Multidrug-resistant tuberculosis not due to noncompliance but to between-patient pharmacokinetic variability. J The Journal of infectious diseases 204, 1951–1959. doi: 10.1093/infdis/jir658
Stalenhoef, J. E., Alisjahbana, B., Nelwan, E., Van Der Ven-Jongekrijg, J., Ottenhoff, T., Van Der Meer, J. (2008). The role of interferon-gamma in the increased tuberculosis risk in type 2 diabetes mellitus. J European Journal of Clinical Microbiology Infectious Diseases 27, 97–103. doi: 10.1007/s10096-007-0395-0
Sturkenboom, M. G., Märtson, A.-G., Svensson, E. M., Sloan, D. J., Dooley, K. E., Van Den Elsen, S. H. (2021). Population pharmacokinetics and Bayesian dose adjustment to advance TDM of anti-TB drugs. J Clinical pharmacokinetics 60, 685–710. doi: 10.1007/s40262-021-00997-0
Subramanian, I., Verma, S., Kumar, S., Jere, A., Anamika, K. (2020). Multi-omics data integration, interpretation, and its application. J Bioinformatics biology insights 14. doi: 10.1177/1177932219899051
Tadokera, R., Meintjes, G., Skolimowska, K. H., Wilkinson, K. A., Matthews, K., Seldon, R. (2011). Hypercytokinaemia accompanies HIV–tuberculosis immune reconstitution inflammatory syndrome. J European Respiratory Journal 37, 1248–1259. doi: 10.1183/09031936.00091010
Tamburini, B., Badami, G. D., Azgomi, M. S., Dieli, F., La Manna, M. P., Caccamo, N. (2021). Role of hematopoietic cells in mycobacterium tuberculosis infection. J Tuberculosis 130, 102109. doi: 10.1016/j.tube.2021.102109
Tan, Q., Xie, W., Min, R., Dai, G., Xu, C.-C., Pan, H., et al. (2012). Characterization of Th1-and Th2-type immune response in human multidrug-resistant tuberculosis. J European journal of clinical microbiology infectious diseases 31, 1233–1242. doi: 10.1007/s10096-011-1434-4
Thorsted, A., Nielsen, E. I., Friberg, L. E. (2020). Pharmacodynamics of immune response biomarkers of interest for evaluation of treatment effects in bacterial infections. J International journal of antimicrobial agents 56, 106059. doi: 10.1016/j.ijantimicag.2020.106059
Tomaszewski, P., Kubiak-Tomaszewska, G., Pachecka, J. (2008). Ccytochrome p450 polymorphism ń molecular, metabolic, and pharmacogenetic aspects. ii. participation of cyp isoenzymes in the metabolism of endogenous substances and drugs. Acta Poloniae Pharmaceutica Drug Research (Polish Pharmaceutical Society)
Tostmann, A., Boeree, M. J., Aarnoutse, R. E., De Lange, W. C., Van Der Ven, A. J., Dekhuijzen, R. (2008). Antituberculosis drug-induced hepatotoxicity: Concise up-to-date review. J Journal of gastroenterology hepatology 23, 192–202. doi: 10.1111/j.1440-1746.2007.05207.x
Tousif, S., Singh, D. K., Ahmad, S., Moodley, P., Bhattacharyya, M., Van Kaer, L., et al. (2014). Isoniazid induces apoptosis of activated CD4+ T cells. J Journal of Biological Chemistry 289, 30190–30195. doi: 10.1074/jbc.C114.598946
Tremoulet, A. H., Albani, S. (2005). Immunomics in clinical development: Bridging the gap. J Expert Review of Clinical Immunology 1, 3–6. doi: 10.1586/1744666X.1.1.3
Ubeda, C., Pamer, E. G. (2012). Antibiotics, microbiota, and immune defense. J Trends in immunology 33, 459–466. doi: 10.1016/j.it.2012.05.003
Urbanova, M., Gajdosova, M., Steinhart, M., Vetchy, D., Brus, J. (2016). Molecular-level control of ciclopirox olamine release from poly (ethylene oxide)-based mucoadhesive buccal films: Exploration of structure–property relationships with solid-state NMR. J Molecular Pharmaceutics 13, 1551–1563. doi: 10.1021/acs.molpharmaceut.6b00035
Vailati-Riboni, M., Palombo, V., Loor, J. (2017). “What are omics sciences?,” in Periparturient diseases of dairy cows (Springer). doi: 10.1007/978-3-319-43033-1_1
Van Rheenen, P. (2002). The use of the paediatric tuberculosis score chart in an HIV-endemic area. J Tropical Medicine International Health 7, 435–441. doi: 10.1046/j.1365-3156.2002.00882.x
Vinnard, C., Ravimohan, S., Tamuhla, N., Pasipanodya, J., Srivastava, S., Modongo, C., et al. (2017). Pyrazinamide clearance is impaired among HIV/tuberculosis patients with high levels of systemic immune activation. J PLoS One 12. doi: 10.1371/journal.pone.0187624
Vrieling, F., Alisjahbana, B., Sahiratmadja, E., Van Crevel, R., Harms, A. C., Hankemeier, T. (2019). Plasma metabolomics in tuberculosis patients with and without concurrent type 2 diabetes at diagnosis and during antibiotic treatment. J Scientific reports 9, 1–12. doi: 10.1038/s41598-019-54983-5
Walker, N. F., Meintjes, G., Wilkinson, R. (2013). HIV-1 and the immune response to TB. 8, 57–80. doi: 10.2217/fvl.12.123
Wallis, R. S., Pai, M., Menzies, D., Doherty, T. M., Walzl, G., Perkins, M. D., et al. (2010). Biomarkers and diagnostics for tuberculosis: Progress, needs, and translation into practice. J The lancet 375, 1920–1937. doi: 10.1016/S0140-6736(10)60359-5
Wallis, R. S., Wang, C., Meyer, D., Thomas, N. (2013). Month 2 culture status and treatment duration as predictors of tuberculosis relapse risk in a meta-regression model. J PloS one 8. doi: /10.1371/journal.pone.0071116
Walzl, G., Ronacher, K., Hanekom, W., Scriba, T. J., Zumla, A. (2011). Immunological biomarkers of tuberculosis. J Nature Reviews Immunology 11, 343–354. doi: 10.1038/nri2960
Wang, X., Cao, Z., Jiang, J., Niu, H., Dong, M., Tong, A., et al. (2010). Association of mycobacterial antigen-specific CD4+ memory T cell subsets with outcome of pulmonary tuberculosis. 60, 133–139. doi: 10.1016/j.jinf.2009.10.048
Wang, K., Wadhwa, P. D., Culhane, J. F., Nelson, E. L. (2005). Validation and comparison of luminex multiplex cytokine analysis kits with ELISA: Determinations of a panel of nine cytokines in clinical sample culture supernatants. Journal of reproductive immunology 66, 175–191. doi: 10.1016/j.jri.2005.03.005
Wang, J., Yin, Y., Wang, X., Pei, H., Kuai, S., Gu, L., et al. (2015). Ratio of monocytes to lymphocytes in peripheral blood in patients diagnosed with active tuberculosis. J Brazilian Journal of Infectious Diseases 19, 125–131. doi: 10.1016/j.bjid.2014.10.008
Wei, Z., Li, Y., Wei, C., Li, Y., Xu, H., Wu, Y., et al. (2020). The meta-analysis for ideal cytokines to distinguish the latent and active TB infection. J BMC Pulmonary Medicine 20, 1–12. doi: 10.1186/s12890-020-01280-x
Weiskopf, D., Weinberger, B., Grubeck-Loebenstein, B. (2009). The aging of the immune system. J Transplant international 22, 1041–1050. doi: 10.1111/j.1432-2277.2009.00927.x
WHO (2014). The end TB strategy (Geneva, Switzerland: WHO). Available at: https://www.who.int/publications/i/item/WHO-HTM-TB-2015.19.
WHO (2021a) Global tuberculosis report. Available at: https://www.who.int/teams/global-tuberculosis-programme/tb-reports.
WHO (2021b). Tuberculosis deaths rise for the first time in more than a decade due to the COVID-19 pandemic (Geneva, Switzerland: WHO). Available at: https://www.who.int/news/item/14-10-2021-tuberculosis-deaths-rise-for-the-first-time-in-more-than-a-decade-due-to-the-covid-19-pandemic.
WHO (2022). Global tuberculosis report 2022 (Geneva, Switzerland: WHO). Available at: https://www.who.int/teams/global-tuberculosis-programme/tb-reports/global-tuberculosis-report-2022.
Wicha, S. G., Märtson, A. G., Nielsen, E. I., Koch, B. C., Friberg, L. E., Alffenaar, J. W., et al. (2021). From therapeutic drug monitoring to model-informed precision dosing for antibiotics. J Clinical Pharmacology Therapeutics 109, 928–941. doi: 10.1002/cpt.2202
Wilson, L., Jordan, M. (2004). New microtubule/tubulin-targeted anticancer drugs and novel chemotherapeutic strategies. J Journal of chemotherapy 16, 83–85. doi: 10.1179/joc.2004.16.Supplement-1.83
Wu, K.-C., Lin, C.-J. (2019). The regulation of drug-metabolizing enzymes and membrane transporters by inflammation: Evidences in inflammatory diseases and age-related disorders. J journal of food drug analysis 27, 48–59. doi: 10.1016/j.jfda.2018.11.005
Xiong, W., Dong, H., Wang, J., Zou, X., Wen, Q., Luo, W., et al. (2016). Analysis of plasma cytokine and chemokine profiles in patients with and without tuberculosis by liquid array-based multiplexed immunoassays. J PLoS One 11. doi: 10.1371/journal.pone.0148885
Yamashiro, S., Kawakami, K., Uezu, K., Kinjo, T., Miyagi, K., Nakamura, K., et al. (2005). Lower expression of Th1-related cytokines and inducible nitric oxide synthase in mice with streptozotocin-induced diabetes mellitus infected with mycobacterium tuberculosis. J Clinical Experimental Immunology 139, 57–64. doi: 10.1111/j.1365-2249.2005.02677.x
Yan, S., Nagle, D. G., Zhou, Y., Zhang, W. (2018). “Application of systems biology in the research of TCM formulae,” in Systems biology and its application in TCM formulas research (Elsevier) 31–67. doi: 10.1016/B978-0-12-812744-5.00003-5
Yan, Z. H., Zheng, X. F., Yi, L., Wang, J., Wang, X. J., Wei, P. J., et al. (2017). CD 137 is a useful marker for identifying CD 4+ T cell responses to mycobacterium tuberculosis. J Scandinavian Journal of Immunology 85, 372–380. doi: 10.1111/sji.12541
Yao, X., Liu, Y., Liu, Y., Liu, W., Ye, Z., Zheng, C. (2017). Multiplex analysis of plasma cytokines/chemokines showing different immune responses in active TB patients, latent TB infection and healthy participants. J Tuberculosis 107, 88–94. doi: 10.1016/j.tube.2017.07.013
Yew, W. W., Leung, C. C., Zhang, Y. (2017). Oxidative stress and TB outcomes in patients with diabetes mellitus? J Journal of Antimicrobial Chemotherapy 72, 1552–1555. doi: 10.1093/jac/dkx046
Yong, Y. K., Tan, H. Y., Saeidi, A., Wong, W. F., Vignesh, R., Velu, V., et al. (2019). Immune biomarkers for diagnosis and treatment monitoring of tuberculosis: Current developments and future prospects. J Frontiers in microbiology 10, 2789. doi: 10.3389/fmicb.2019.02789
Yoshida, M., Worlock, K. B., Huang, N., Lindeboom, R. G., Butler, C. R., Kumasaka, N. (2022). Local and systemic responses to SARS-CoV-2 infection in children and adults. J Nature 602, 321–327. doi: 10.1038/s41586-021-04345-x
Young, C., Walzl, G., Du Plessis, N. (2020). Therapeutic host-directed strategies to improve outcome in tuberculosis. J Mucosal immunology 13, 190–204. doi: 10.1038/s41385-019-0226-5
Yu, J., Peng, J., Chi, H. (2019). Systems immunology: Integrating multi-omics data to infer regulatory networks and hidden drivers of immunity. J Current opinion in systems biology 15, 19–29. doi: 10.1016/j.coisb.2019.03.003
Zambuzi, F. A., Cardoso-Silva, P. M., Espindola, M. S., Soares, L. S., Galvão-Lima, L. J., Brauer, V. S. (2016). Identification of promising plasma immune biomarkers to differentiate active pulmonary tuberculosis. J Cytokine 88, 99–107. doi: 10.1016/j.cyto.2016.08.030
Zeka, A. N., Tasbakan, S., Cavusoglu, C. (2011). Evaluation of the GeneXpert MTB/RIF assay for rapid diagnosis of tuberculosis and detection of rifampin resistance in pulmonary and extrapulmonary specimens. J Journal of clinical microbiology 49, 4138–4141. doi: 10.1128/JCM.05434-11
Zellweger, J.-P., Sotgiu, G., Corradi, M., Durando, P. (2020). The diagnosis of latent tuberculosis infection (LTBI): Currently available tests, future developments, and perspectives to eliminate tuberculosis (TB). J La Medicina del Lavoro 111, 170. doi: 10.23749/mdl.v111i3.9983
Zhao, Y., Yang, X., Zhang, X., Yu, Q., Zhao, P., Wang, J., et al. (2018). IP-10 and RANTES as biomarkers for pulmonary tuberculosis diagnosis and monitoring. J Tuberculosis 111, 45–53. doi: 10.1016/j.tube.2018.05.004
Zhu, C., Liu, J., Ling, Y., Yang, H., Liu, Z., Zheng, R., et al. (2012). Evaluation of the clinical value of ELISA based on MPT64 antibody aptamer for serological diagnosis of pulmonary tuberculosis. J BMC infectious diseases 12, 1–8. doi: 10.1186/1471-2334-12-96
Ziglam, H., Daniels, I., Finch, R. (2004). Immunomodulating activity of rifampicin. J Journal of chemotherapy 16, 357–361. doi: 10.1179/joc.2004.16.4.357
Zimmer, A. J., Lainati, F., Aguilera Vasquez, N., Chedid, C., Mcgrath, S., Benedetti, A. (2022). Biomarkers that correlate with active pulmonary tuberculosis treatment response: A systematic review and meta-analysis. J Journal of clinical microbiology 60, e01859–e01821. doi: 10.1128/jcm.01859-21
Keywords: tuberculosis, immunomics, multi-omics, personalized medicine, biomarkers, model-informed precision dosing
Citation: Thu VTA, Dat LD, Jayanti RP, Trinh HKT, Hung TM, Cho Y-S, Long NP and Shin J-G (2023) Advancing personalized medicine for tuberculosis through the application of immune profiling. Front. Cell. Infect. Microbiol. 13:1108155. doi: 10.3389/fcimb.2023.1108155
Received: 25 November 2022; Accepted: 17 January 2023;
Published: 10 February 2023.
Edited by:
Bindu Singh, Texas Biomedical Research Institute, United StatesReviewed by:
Jian Xu, Capital Medical University, ChinaCopyright © 2023 Thu, Dat, Jayanti, Trinh, Hung, Cho, Long and Shin. This is an open-access article distributed under the terms of the Creative Commons Attribution License (CC BY). The use, distribution or reproduction in other forums is permitted, provided the original author(s) and the copyright owner(s) are credited and that the original publication in this journal is cited, in accordance with accepted academic practice. No use, distribution or reproduction is permitted which does not comply with these terms.
*Correspondence: Jae-Gook Shin, cGhzaGluamdAaW5qZS5hYy5rcg==; Nguyen Phuoc Long, cGh1b2Nsb25nQGluamUuYWMua3I=
†These authors have contributed equally to this work
Disclaimer: All claims expressed in this article are solely those of the authors and do not necessarily represent those of their affiliated organizations, or those of the publisher, the editors and the reviewers. Any product that may be evaluated in this article or claim that may be made by its manufacturer is not guaranteed or endorsed by the publisher.
Research integrity at Frontiers
Learn more about the work of our research integrity team to safeguard the quality of each article we publish.