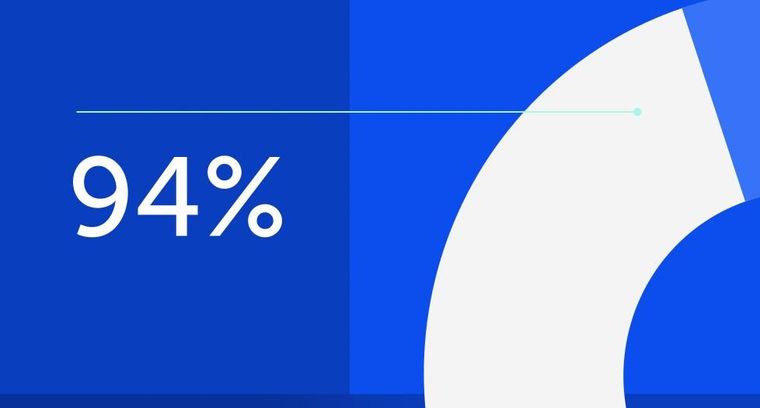
94% of researchers rate our articles as excellent or good
Learn more about the work of our research integrity team to safeguard the quality of each article we publish.
Find out more
ORIGINAL RESEARCH article
Front. Cell. Infect. Microbiol., 05 October 2022
Sec. Clinical Microbiology
Volume 12 - 2022 | https://doi.org/10.3389/fcimb.2022.1010771
This article is part of the Research TopicDesign and development of new therapeutics against infectious diseases using computational and experimental approachesView all 13 articles
Multi-drug resistant tuberculosis still remains a major public health crisis globally. With the emergence of newer active tuberculosis disease, the requirement of prolonged treatment time and adherence to therapy till its completion necessitates the search of newer therapeutics, targeting human host factors. The current work utilized statistical meta-analysis of human gene transcriptomes of active pulmonary tuberculosis disease obtained from six public datasets. The meta-analysis resulted in the identification of 2038 significantly differentially expressed genes (DEGs) in the active tuberculosis disease. The gene ontology (GO) analysis revealed that these genes were major contributors in immune responses. The pathway enrichment analyses identified from various human canonical pathways are related to other infectious diseases. In addition, the comparison of the DEGs with the tuberculosis genome wide association study (GWAS) datasets revealed the presence of few genetic variants in their proximity. The analysis of protein interaction networks (human and Mycobacterium tuberculosis) and host directed drug-target interaction network led to new candidate drug targets for drug repurposing studies. The current work sheds light on host genes and pathways enriched in active tuberculosis disease and suggest potential drug repurposing targets for host-directed therapies.
Tuberculosis (TB) is an infectious disease which remained throughout human history. Mycobacterium tuberculosis (Mtb) is the main causative agent of TB. Around 10% of individuals develop TB when exposed to Mtb and 5% of the infected individuals develop TB within 1-2 years while the remaining 5% develop the disease at any other time (Frieden et al., 2003). Active TB has higher burden of TB when compared to latent TB (Lee, 2016). Individuals with compromised immune systems, such as people with HIV, diabetes or people with constant tobacco use are at high risk of falling ill.
TB is the 13th leading cause of death globally in 2020. Around 86% of new cases reported around the world in 2020 were contributed majorly by China, Indonesia, the Philippines, Pakistan, Nigeria, Bangladesh and South Africa with India leading the list (WHO, 2021). Over the period of time Mtb has adopted newer subversion strategies to successfully evade the host immune system enabling it to reside in the host resulting in latent or active disease manifestation (Behar et al., 2010; Ernst, 2018).
The infection results in a complex dynamics between the host and pathogen triggering various immune signalling cascades and cross-talks between molecular components (Casadevall and Pirofski, 2000). Several contributing factors associated with the disease were identified through genetic and biochemical experimental studies. The recent surge of omics data has further aided in understanding of factors influencing predisposition of the disease and markers associated with the disease severity.
With increased availability of gene expression data, studies based on TB blood transcriptomics offers a robust approach to study the immunology of TB. The comparative studied of healthy and TB cohorts shed light on differentially expressed genes (DEGs) and also allow observations of such DEG upon vaccine/drug treatment. Further the DEG analysis also aids in understanding of regulatory mechanisms contributing to the functional consequences.
In the current study, a statistical meta-analysis was carried out using whole blood expression profiles from infected TB patients to identify key human transcriptomics signatures characteristic of the disease. The study also utilized host genetic disease association and drug-repurposing analyses to further to prioritize the results. In addition, the gene ontology and pathway-based annotations identified genes and pathways significantly altered in the diseased condition.
The whole-blood microarray gene expression profiles of patients with active pulmonary tuberculosis and healthy cohorts were retrieved from NCBI GEO (Barrett et al., 2013). The datasets were further filtered based on the following conditions: (i) The expression profiles were from human patients affected by tuberculosis undergoing no prior treatment, (ii) Only samples from active tuberculosis patients and control groups were considered, (iii) The datasets should include both healthy controls and patient group, (iv) The patient or the control group should not be infected with any other secondary diseases, (v) The patient and control group should include more than 5 samples each.
The background corrected files were processed using limma package in R (Ritchie et al., 2015). The data were quantile normalized, log transformed and missing values were removed. The probe identifiers were converted to Entrez gene IDs. If multiple probes are mapped to a single gene, then the average expression value of the probes were used for the gene. Post normalization, individual datasets were subjected to Principal Component Analysis (PCA). PCA was carried out to observe a distinct separation between the active and control samples.
Statistical meta-analysis was carried out using NetworkAnalyst (Zhou et al., 2019). NetworkAnalyst interprets gene expression data including meta-analysis, tissue specific PPI networks, gene regulatory networks, gene co-expression networks along with networks for toxicogenomics and pharmacogenomics studies. The pre-processed expression values were used as input for the web tool. Differential expression analysis for each dataset was performed using limma with false discovery rate (FDR) cutoff of 0.05. The batch effects were adjusted using ComBat method. The corrected datasets were merged and statistical meta-analysis was carried out using INMEX. The combined effect size method for meta-analysis was used to generate the results. The random effect model which encloses cross-study heterogeneity was used for meta-analysis. Differentially expressed genes (DEG) were obtained using FDR cutoff of 0.01 in the meta-analysis. The DEGs with absolute combined effect size > 1.5 were chosen for genetic variant analyses and drug interaction analyses.
The strength of the results obtained from meta-analysis was further validated by comparing the genes expressed in latent and control samples from the same datasets. Partial Least Square Discriminant Analysis (PLS-DA) was applied to the DEGs. Significant model was selected by 7-fold cross validation. The model performance was evaluated using the area under the Receiver Operating Characteristic (ROC) curve (AUC). All the above validation process was carried out using mixOmics package in R (Rohart et al., 2017).
Gene and pathway enrichment analysis was carried out using DAVID web server to identify significantly enriched Gene ontology (GO) biological processes (BPs) and KEGG pathways, which were ranked based on the hypergeometric test with FDR cutoff of 0.05 (Sherman et al., 2022). DAVID web server offers functional annotation and enrichment analyses of gene lists provided by the user.
A comprehensive human protein-protein interaction network (hPPI) was constructed. High confidence, experimentally verified interactions extracted from STRING database was used for the construction (Szklarczyk et al., 2021). The STRING database integrates known and predicted associations between proteins encompassing physical interactions and functional associations. Similarly, the pathogen proteins interacting with host DEG were mined from various literature sources (Rapanoel et al., 2013; Penn et al., 2018; Augenstreich and Briken, 2020; Verma et al., 2022). These data were used to construct human protein – Mtb protein interaction network (hmPPI).
The highly interconnected components of the hPPI and hmPPI were identified using the Cytoscape plugin CytoHubba. CytoHubba is a user-friendly interface to explore important nodes in biological networks using various topological metrics. The hub genes of the networks were identified using the topological metrics degree and Maximal Clique Centrality (MCC) (Chin et al., 2014).
The drug compounds interacting with DEG were retrieved from DrugBank Version 5 (Wishart et al., 2018). DrugBank is a comprehensive database which holds information about drugs, their mechanisms, interactions and targets. Drugs with experimental or clinical evidence for direct interactions with the protein were selected. Drugs with pharmacological actions as the same direction of the DEG and drugs with unknown pharmacological actions were excluded. Only DEGs with a combined effect size greater than 1.5 were considered for the analysis.
The genetic differences between tuberculosis-affected and healthy individuals can give a mechanistic insight about the disease and functional implication of the affected gene. The single nucleotide polymorphisms (SNPs) proximal to the DEG were obtained from GRASP database (P-value < 5e-8) (Leslie et al., 2014). GRASP database encloses deeply extracted and annotated database of genome-wide association studies (GWAS) results enclosing more than 6.2 million SNP-phenotype association. Similarly, the regulatory SNP were retrieved from Slidebase database using the enhancer regions of the DEG (Ienasescu et al., 2016). SlideBase offers a new way of selecting genes, promoters, enhancers and microRNAs that are preferentially expressed/used in a specified set of cells/tissues. The genomic regions in linkage disequilibrium (LD) with the SNP were collected from SNAP (Johnson et al., 2008). The association between a gene and its corresponding SNP was prioritized based on the overlap between the genomic location of the DEG or its enhancer and LD region of a SNP. The query tool SNAP enables the identification of single-nucleotide polymorphisms (SNPs) and annotate nearby SNPs in linkage disequilibrium (proxies) based on HapMap project results.
The work plan and approaches implemented in the current study is illustrated in Figure 1.
The database querying and filtering identified around 149 GEO microarray datasets for TB-related host response at the time of study (June 2022). Further, filtering based on the study inclusion criteria, a total of six datasets enclosing control and active TB samples were selected for next set analyses (Table 1).
The results of PCA indicated that the samples were clustered based on the observations of the study. However, after batch correction the samples were clustered based on the disease condition as active TB and control. We also observed a few samples outside the clusters before and after the batch correction procedure (Figure 2).
Figure 2 Principal Component Analysis (PCA) plots showing the separation control and active samples across all datasets used in the study. (A) GSE19435 (B) GSE19439 (C) GSE19444 (D) GSE54992 (E) GSE62525 (F) GSE152532.
When the datasets DEG were compared, we identified genes regulated in the same direction. The meta-analysis identified a total of 2038 DEG of which 861 genes were up-regulated and 1177 genes were down-regulated (S. Table 1). Further analysis identified a total of 113 genes (up-regulated – 24 and down-regulated- 89) with absolute combined effect size as a reference for the log2 fold change (logFC) greater than 1.5 (Table 2). S1PR1 ranks first among the up-regulated genes. S1PR1 expression is associated with lymphocyte recirculation. Similarly, FCGR1B is the top-ranking gene which is down-regulated in the active TB. To assess the results obtained from the meta-analysis, we validated the 113 genes with logFC > 1.5 in three datasets GSE19444 (Illumina), GSE54992 (Affimetrix) and GSE62525 (Phalanx) from different platforms. The PLS-DA models showed good sensitivity (above 85%) and specificity (above 83%) in all three datasets. The control, active and latent TB samples formed three different clusters marking clear differentiation (Figure 3). The ROC plot for the models suggest that the PLS-DA model can distinguish active tuberculosis samples from both latent and control groups with high true positive and low false positive rate (Figure 4). These measures show that DEG can act as biomarkers for the detection of active TB cases.
Figure 3 Partial least squares-discriminate analysis (PLS-DA) plots showing differentiation between the control and TB samples.
Figure 4 Receiver operating characteristic (ROC) and Area under curve (AUC) from the PLS-DA on the 113 DEGs data.
The functional GO was carried out for the up and down regulated DEG identified by the meta-analysis (Table 3). The GO analysis identified that up-regulated DEG significantly involved in cellular components (CCs) were nucleoplasm, nucleus and cytosol. For GO BP analysis, the DEG showed involvement in mRNA splicing, via spliceosome, cytoplasmic translation and rRNA processing. Similarly for GO molecular function (MF) analyses, the DEGs were majorly enriched in RNA binding, protein binding and ATP binding. The GO CC analysis of down-regulated DEGs showed involvement in extracellular exosome, cytosol and lysosome. The GO BP analysis identified involvement in defense response to virus, innate immune response and response to virus. The GO MF analysis showed enriched functions such as protein binding, protease binding and MHC class I protein binding.
The pathway enrichment analysis implemented using DAVID identified various dysregulated pathways mediated by the DEGs (Table 4). The up-regulated DEGs showed enrichment of pathways involved in Spliceosome, Ribosome and Nucleocytoplasmic transport. We also observed pathways overlapping with other infectious diseases such as Herpes simplex virus 1 infection and Coronavirus disease - COVID-19.
The down-regulated DEGs showed involvement in NOD-like receptor signaling pathway, Lysosome and other infectious disease pathways such as Influenza A, Salmonella infection, Hepatitis C and Epstein-Barr virus infection. We also observed the presence of Tuberculosis pathway in the list. The presence of tuberculosis pathway in our analysis indicated that the genes identified in the meta-analysis demonstrate their significant association with the disease.
Identifying the physical interactions between the proteins will provide clues to combat infection. The mapping of 2038 DEGs along with their partners resulted in a network enclosing 325 nodes and 1460 edges (Figure 5). The average number of neighbours in the hPPI was 15. Around 323 genes showed direct interactions with their partners. The top ten hub genes which showed overlaps in degree and MCC measures are RPL10A, RPS4X, RPS16, RPS23, RPS3, RPS13, RPL7A, RPL4, RPS5 and RPS6. All the identified hub genes were ribosomal proteins involved in RNA binding.
Figure 5 Protein-protein interaction network (hPPI) of the 2038 DEGs in the meta-analysis. The up-regulated genes are colored in red and down-regulated genes are colored green. The edges are represented as orange lines.
The hmPPI interaction network enclosed 99 nodes and established 66 connections with an average of 1 connection between the neighbours (Figure 6). Due to the availability of limited Mtb-host protein-protein interactions we did not observe any hub genes based on the topological metrics.
Figure 6 Mtb-host protein-protein interaction network (hmPPI). The Mtb proteins are colored green and human proteins are colored in orange. The edges are represented as blue lines.
The DEGs from the study were queried against DrugBank to mine drugs targeting genes which may be used for repurposing against TB. A total of 22 drugs targeting 8 DEGs were obtained after the screening process (Table 5). Among them each compound showed association with at least one target gene with few exceptions such as ABCB1 which showed interaction with 14 drugs. Human immunoglobulin G (DB00028) acting on C5 and FCGR1B were also observed. FYN kinase targeted by the Fostamatinib was one among the up-regulated genes.
The detection of drug targets which has human genetic support by its involvement in the disease pathology may aid in success of the treatment against the disease by preventing late stage clinical failures. The evidence of involvement in the disease by the 2038 DEGs was retrieved from genome-wide association study (GWAS) datasets. A total of 483 TB-related SNPs were obtained from GRASP database. An overlap between the LD region with a SNP and the DEG location or the enhancer region suggest strong association between the SNP and that particular gene. A total of 33 genes showed association with TB-related SNPs (Table 6).
The meta-analysis of transcriptomes in this study identified S1PR1 as an up-regulated gene with highest fold change. S1PR1, by the detection of its ligand S1P in the blood and lymph, is crucial for naive lymphocytes to access the circulatory system. S1P-S1PR1 signaling is crucial for regulating immune cell development and function. S1P-S1PR1 signaling is needed for mature thymocytes to leave the thymus and for T/B cells to leave secondary lymphoid organs and enter the blood or lymph in both homeostatic and pathological situations (Sinha et al., 2009; Zachariah and Cyster, 2010; Allende et al., 2010; Zhang et al., 2012). S1PR1 analog therapy raises IL-6 and lowers IL-10, but it can’t stop the mycobacterial infection inside the cell (Arish and Naz, 2022). Consequently, the diminished expression of S1PR1 causes retention of naïve T cells in lymphoid tissues (Skon et al., 2013). Similarly, FCGR1B is the top ranking gene with highest fold change and is down-regulated in the active TB condition. The FCGR1B gene is a member of immunoglobulin G, which binds directly with pathogens and neutralizes them. Changes in the Fc gamma receptors affect the response of a host to infection (Song et al., 2017). The FCGR1B gene aids in the host’s immune response during a mycobacterial infection. According to Maertzdorf’s research, people with TB and LTBI had more DEGs than uninfected individuals (Maertzdorf et al., 2011). In a different study, Satproedprai et al. found that the overexpression of FCGR1B in response to bacterial infection caused a humoral immune response and contributed to the development of lung inflammation (Satproedprai et al., 2015). Our results showed downregulation of FCGR1B, probably as a result of active TB.
The enrichment of spliceosomes and lysosomes in GO, indicates its crucial role in active TB infection. The functions of spliceosomes are also regulated differently in infected macrophages. The lysosomes protect against Mtb by controlling how Mtb moves through the lysosomes and stopping it from spreading in cells. Pre-mRNA splicing plays a crucial role in regulating gene expression and protein diversity. The Serine/Arginine rich (SR) proteins are the major components contributing to the selective splicing mechanism. The disruption in the RNA splicing mechanism can lead to crosstalk in the intricate network interactions. The Mtb infection alters the patterns of alternate splicing within the macrophages by affecting the expression of SR proteins (Zhang et al., 2018).
The evolution of Mtb infection has proven its ability to successfully gain access to host cellular components needed for its survival before the initiation of innate antimicrobial response. The process is accomplished by altering various immune response elements such as interferon (IFN)-induced transmembrane (IFITM) gene family members. The IFITM members receive signals for their activation through type I and II IFN stimulation to preclude the establishment of productive infection. Ranjbar et al. show that IFITM proteins inhibit Mtb intracellular growth, indicating that they may contribute to host defense against intracellular bacterial infection (Ranjbar et al., 2015). The IFITMs act on host membrane fluidity at the sites of viral entry by preventing the formation of viral fusion pore. In addition, they increase the trafficking of trapped viruses to the lysosome for its degradation. However, Mtb alters this phagocytic mechanism by switching off the acidification of the phagosomes mediated by the IFTIM family members. One such example is the vacuolar ATPase, a mediator of endosomal acidification which is excluded from Mtb-containing phagosome by Mtb’s bacterial tyrosine phosphatase (Ranjbar et al., 2015).
In addition, pathways related to immune mediated cascades such as T cell receptor signaling pathway, Th17 cell differentiation and NF-kappa B signaling pathway were also observed (Urdahl et al., 2011). The order of appearance of T cell receptor signaling pathway down the list indicate that the onset of genes contributing to this pathways are delayed. Nuclear factor-kappa B (NFκB) pathway mediates pro-inflammatory responses which are required by the host to control of many microbial pathogens. The activation of NFκB has proven to increase the viability of intracellular Mtb in human macrophages by preventing apoptosis and autophagy (Bai et al., 2013). The lysosomal and pathogenic pathways indicate the dominance of these pathways used by the pathogens to avoid lysosomal targeting. They function by actively manipulating the host vesicular trafficking and reside in a vacuoles altered from the default lysosomal trafficking (Sachdeva and Sundaramurthy, 2020). The overlap of infectious disease pathways signals the usage of similar players for evading the infection and using host counterparts to reproduce.
The PPI networks reveal the involvement of major players contributing to the infection. The hPPI network identified ten hub genes which play crucial role in the infection. All these identified hub genes were ribosomal proteins involved in RNA binding. These RNA-binding proteins play critical roles in co- and post-translational regulation. Due to the distinct differences in ribosome structure between Mtb and the host, the ribosome is a multiprotein complex, and the protein-protein interactions of its subunits may be an appealing target for novel antibiotics (Lin et al., 2012). Earlier report based on microarray expression analysis such as Wang et al., also reported up-regulation of 22 unique ribosomal proteins in tuberculosis infection (Wang et al., 2003). In the current work we identified the involvement of 10 (RPL10A, RPS4X, RPS16, RPS23, RPS3, RPS13, RPL7A, RPL4, RPS5 and RPS6) unique ribosomal proteins in the active disease stage with high degree of connectedness. However, the significance of these genes is unclear and requires future studies. The hmPPI network showed MAT2A of the host protein interacted with ten Mtb proteins. MAT2A catalyse the conversion of L-methionine to S-adenosyl-L-methionine in cysteine and methionine metabolism. The Mtb protein partners also perform similar function, for example MetK which is a methionine adenosyltransferanse (Wang et al., 2003).
The meta-analysis identified the involvement of various kinases in active TB. The drug bank list also narrowed down few drugs acting on kinases. Various classes of tyrosine kinase inhibitors exhibit distinct mechanism of action to inhibit phagocytosis of tubercle bacilli in dose and time-dependent manner. Early studies have proven that tyrosine kinase inhibitors including Dasatinib, Bosutinib, Imatinib, Nilotinib, Ponatinib, Nintedanib, Fostamatinib and Tirbanibulin reduce the growth of intracellular Mtb. The ligation of complement receptors by Mtb plays a major role in stimulation of tyrosine phosphorylation (Schlesinger and DesJardin, 2022). Focusing on drugs targeting these proteins can act as a starting point for the development of host mediated drug repurposing studies. The genetic-variant analysis recognized genes contributing to drug resistance such as ABCB1 (Pontual et al., 2017) and susceptibility to latent tuberculosis such as SP110 and OAS1 (Chang et al., 2018; Leisching et al., 2019). Analyzing the genetic variants of the tuberculosis patients before starting any treatment regimens is highly suggested to prevent late stage failures.
The current study focuses on meta-analysis and highlights host genes and pathways crucial for tuberculosis disease. The DEGs identified in the current work shed light on promising drug targets for host-directed repurposing therapies. The work also suggests considering the genetic variants associated with the TB-related genes to enhance the success rate of therapies in individuals affected with tuberculosis. Future studies assessing the behavior of the identified DEGs during and after the treatment can ascertain their involvement in the disease pathogenesis and progression.
The datasets presented in this study can be found in online repositories. The names of the repository/repositories and accession number(s) can be found in the article/Supplementary Material.
Collected data, Implemented the analysis and Manuscript writing: NP. Conceived and designed the analysis: MA. All authors contributed to the article and approved the submitted version.
This research did not receive any specific grant from funding agencies in the public, commercial, or not-for-profit sectors.
We would like to thank Vellore Institute of Technology (VIT) for providing computational facility.
The authors declare that the research was conducted in the absence of any commercial or financial relationships that could be construed as a potential conflict of interest.
All claims expressed in this article are solely those of the authors and do not necessarily represent those of their affiliated organizations, or those of the publisher, the editors and the reviewers. Any product that may be evaluated in this article, or claim that may be made by its manufacturer, is not guaranteed or endorsed by the publisher.
The Supplementary Material for this article can be found online at: https://www.frontiersin.org/articles/10.3389/fcimb.2022.1010771/full#supplementary-material
Supplementary Table 1 | List of 2038 DEGs identified by meta-analysis.
Allende, M. L., Tuymetova, G., Lee, B. G., Bonifacino, E., Wu, Y. P., Proia, R. L. (2010). S1P1 receptor directs the release of immature b cells from bone marrow into blood. J. Exp. Med. 207 (5), 1113–1124. doi: 10.1084/jem.20092210
Arish, M., Naz, F. (2022). Sphingosine-1-phosphate receptors 2 and 3 reprogram resting human macrophages into M1 phenotype following mycobacteria infection. Curr. Res. Immunol. 3, 110–117. doi: 10.1016/j.crimmu.2022.05.004
Augenstreich, J., Briken, V. (2020). Host cell targets of released lipid and secreted protein effectors of mycobacterium tuberculosis. Front. Cell. Infection Microbiol. 10. doi: 10.3389/fcimb.2020.595029
Bai, X., Feldman, N. E., Chmura, K., Ovrutsky, A. R., Su, W. L., Griffin, L., et al. (2013). Inhibition of nuclear factor-kappa b activation decreases survival of Mycobacterium tuberculosis in human macrophages. PLoS One 8 (4), e61925. doi: 10.1371/journal.pone.0061925
Barrett, T., Wilhite, S. E., Ledoux, P., Evangelista, C., Kim, I. F., Tomashevsky, M., et al. (2013). NCBI GEO: archive for functional genomics data sets–update. Nucleic Acids Res. 41 (Database issue), D991–D995. doi: 10.1093/nar/gks1193
Behar, S. M., Divangahi, M., Remold, H. G. (2010). Evasion of innate immunity by Mycobacterium tuberculosis: is death an exit strategy? Nat. Rev. Microbiol. 8 (9), 668–674. doi: 10.1038/nrmicro2387
Casadevall, A., Pirofski, L.a. (2000). Host-pathogen interactions: Basic concepts of microbial commensalism, colonization, infection, and disease. Infect. Immun. 68 (12), 6511–6518. doi: 10.1128/IAI.68.12.6511-6518.2000
Chang, S. Y., Chen, M. L., Lee, M. R., Liang, Y. C., Lu, T. P., Wang, J. Y., et al. (2018). SP110 polymorphisms are genetic markers for vulnerability to latent and active tuberculosis infection in Taiwan. Dis. Markers 2018, 4687380. doi: 10.1155/2018/4687380
Chin, C. H., Chen, S. H., Wu, H. H., Ho, C. W., Ko, M. T., Lin, C. Y. (2014). cytoHubba: identifying hub objects and sub-networks from complex interactome. BMC Syst. Biol. 8 (4), S11. doi: 10.1186/1752-0509-8-S4-S11
Ernst, J. D. (2018). Mechanisms of m. tuberculosis immune evasion as challenges to TB vaccine design. Cell Host Microbe 24 (1), 34–42. doi: 10.1016/j.chom.2018.06.004
Frieden, T. R., Sterling, T. R., Munsiff, S. S., Watt, C. J., Dye, C. (2003). Tuberculosis. Lancet 362 (9387), 887–899. doi: 10.1016/S0140-6736(03)14333-4
Ienasescu, H., Li, K., Andersson, R., Vitezic, M., Rennie, S., Chen, Y., et al. (2016). On-the-fly selection of cell-specific enhancers, genes, miRNAs and proteins across the human body using SlideBase. Database (Oxford) 2016, baw144. doi: 10.1093/database/baw144
Johnson, A. D., Handsaker, R. E., Pulit, S. L., Nizzari, M. M., O’Donnell, C. J., de Bakker, P. I. W. (2008). SNAP: a web-based tool for identification and annotation of proxy SNPs using HapMap. Bioinformatics 24 (24), 2938–2939. doi: 10.1093/bioinformatics/btn564
Lee, S. H. (2016). Tuberculosis infection and latent tuberculosis. Tuberc Respir. Dis. (Seoul) 79 (4), 201–206. doi: 10.4046/trd.2016.79.4.201
Leisching, G., Cole, V., Ali, A. T., Baker, B. (2019). OAS1, OAS2 and OAS3 restrict intracellular m. tb replication and enhance cytokine secretion. Int. J. Infect. Dis. 80S, S77–S84. doi: 10.1016/j.ijid.2019.02.029
Leslie, R., O’Donnell, C. J., Johnson, A. D. (2014). GRASP: analysis of genotype-phenotype results from 1390 genome-wide association studies and corresponding open access database. Bioinformatics 30 (12), i185–i194. doi: 10.1093/bioinformatics/btu273
Lin, Y., Li, Y., Zhu, Y., Zhang, J., Li, Y., Liu, X., et al. (2012). Identification of antituberculosis agents that target ribosomal protein interactions using a yeast two-hybrid system. Proc. Natl. Acad. Sci. U.S.A. 109 (43), 17412–17417. doi: 10.1073/pnas.1110271109
Maertzdorf, J., Repsilber, D., Parida, S. K., Stanley, K., Roberts, T., Black, G., et al. (2011). Human gene expression profiles of susceptibility and resistance in tuberculosis. Genes Immun. 12 (1), 15–22. doi: 10.1038/gene.2010.51
Penn, B. H., Netter, Z., Johnson, J. R., Von Dollen, J., Jang, G. M., Johnson, T., et al. (2018). An Mtb-human protein-protein interaction map identifies a switch between host antiviral and antibacterial responses. Mol. Cell. 71 (4), 637–648.e5. doi: 10.1016/j.molcel.2018.07.010
Pontual, Y., Pacheco, V. S. S., Monteiro, S. P., Quintana, M. S. B., Costa, M. J. M., Rolla, V. C., et al. (2017). ABCB1 gene polymorphism associated with clinical factors can predict drug-resistant tuberculosis. Clin. Sci. (Lond) 131 (15), 1831–1840. doi: 10.1042/CS20170277
Ranjbar, S., Haridas, V., Jasenosky, L. D., Falvo, J. V., Goldfeld, A. E. (2015). A role for IFITM proteins in restriction of Mycobacterium tuberculosis infection. Cell Rep. 13 (5), 874–883. doi: 10.1016/j.celrep.2015.09.048
Rapanoel, H. A., Mazandu, G. K., Mulder, N. J. (2013). Predicting and analyzing interactions between Mycobacterium tuberculosis and its human host. PLoS One 8 (7), e67472. doi: 10.1371/journal.pone.0067472
Ritchie, M. E., Phipson, B., Wu, D., Hu, Y., Law, C. W., Shi, W., et al. (2015). Limma powers differential expression analyses for RNA-sequencing and microarray studies. Nucleic Acids Res. 43 (7), e47. doi: 10.1093/nar/gkv007
Rohart, F., Gautier, B., Singh, A., Cao, K. A. L. (2017). mixOmics: An r package for ‘omics feature selection and multiple data integration. PLoS Comput. Biol. 13 (11), e1005752. doi: 10.1371/journal.pcbi.1005752
Sachdeva, K., Sundaramurthy, V. (2020). The interplay of host lysosomes and intracellular pathogens. Front. Cell Infect. Microbiol. 10, 595502. doi: 10.3389/fcimb.2020.595502
Satproedprai, N., Wichukchinda, N., Suphankong, S., Inunchot, W., Kuntima, T., Kumpeerasart, S., et al. (2015). Diagnostic value of blood gene expression signatures in active tuberculosis in thais: a pilot study. Genes Immun. 16 (4), 253–260. doi: 10.1038/gene.2015.4
Schlesinger, L. S., DesJardin, L. E. (2022). Tuberculosis: The microbe host interface (London: Taylor & Francis), 292 p.
Sherman, B. T., Hao, M., Qiu, J., Jiao, X., Baseler, M. W., Lane, H. C., et al. (2022). DAVID: a web server for functional enrichment analysis and functional annotation of gene lists (2021 update). Nucleic Acids Res. 50 (W1), W216–W221. doi: 10.1093/nar/gkac194
Sinha, R. K., Park, C., Hwang, I. Y., Davis, M. D., Kehrl, J. H. (2009). B lymphocytes exit lymph nodes through cortical lymphatic sinusoids by a mechanism independent of sphingosine-1-phosphate-mediated chemotaxis. Immunity 30 (3), 434–446. doi: 10.1016/j.immuni.2008.12.018
Skon, C. N., Lee, J. Y., Anderson, K. G., Masopust, D., Hogquist, K. A., Jameson, S. C. (2013). Transcriptional downregulation of S1pr1 is required for establishment of resident memory CD8+ T cells. Nat. Immunol. 14 (12), 1285–1293. doi: 10.1038/ni.2745
Song, F., Qian, Y., Peng, X., Li, X., Xing, P., Ye, D., et al. (2017). The frontline of immune response in peripheral blood. PLoS One 12 (8), e0182294. doi: 10.1371/journal.pone.0182294
Szklarczyk, D., Gable, A. L., Nastou, K. C., Lyon, D., Kirsch, R., Pyysalo, S., et al. (2021). The STRING database in 2021: customizable protein–protein networks, and functional characterization of user-uploaded gene/measurement sets. Nucleic Acids Res. 49 (D1), D605–D612. doi: 10.1093/nar/gkab835
Urdahl, K., Shafiani, S., Ernst, J. (2011). Initiation and regulation of T-cell responses in tuberculosis. Mucosal Immunol. 4 (3), 288–293. doi: 10.1038/mi.2011.10
Verma, R. N., MdZ, M., GP, S., Subbarao, N. (2022). Identification of key proteins in host–pathogeninteractions between Mycobacterium tuberculosis and homo sapiens: A systematic network theoretical approach. Healthcare Analytics 2, 100052. doi: 10.1016/j.health.2022.100052
Wang, J. P., Rought, S. E., Corbeil, J., Guiney, D. G. (2003). Gene expression profiling detects patterns of human macrophage responses following Mycobacterium tuberculosis infection. FEMS Immunol. Med. Microbiol. 39 (2), 163–172. doi: 10.1016/S0928-8244(03)00223-2
WHO (2021). Tuberculosis. Available at: https://www.who.int/news-room/fact-sheets/detail/tuberculosis.
Wishart, D. S., Feunang, Y. D., Guo, A. C., Lo, E. J., Marcu, A., Grant, J. R., et al. (2018). DrugBank 5.0: a major update to the DrugBank database for 2018. Nucleic Acids Res. 46 (D1), D1074–D1082. doi: 10.1093/nar/gkx1037
Zachariah, M. A., Cyster, J. G. (2010). Neural crest-derived pericytes promote egress of mature thymocytes at the corticomedullary junction. Science 328 (5982), 1129–1135. doi: 10.1126/science.1188222
Zhang, W., Niu, C., Fu, R. Y., Peng, Z. Y. (2018). Mycobacterium tuberculosis H37Rv infection regulates alternative splicing in macrophages. Bioengineered 9 (1), 203–208. doi: 10.1080/21655979.2017.1387692
Zhang, L., Orban, M., Lorenz, M., Barocke, V., Braun, D., Urtz, N., et al. (2012). A novel role of sphingosine 1-phosphate receptor S1pr1 in mouse thrombopoiesis. J. Exp. Med. 209 (12), 2165–2181. doi: 10.1084/jem.20121090
Keywords: tuberculosis, meta-analysis, gene ontology, pathway enrichment, genetic variants, drug repurposing
Citation: Ponnusamy N and Arumugam M (2022) Meta-analysis of active tuberculosis gene expression ascertains host directed drug targets. Front. Cell. Infect. Microbiol. 12:1010771. doi: 10.3389/fcimb.2022.1010771
Received: 03 August 2022; Accepted: 21 September 2022;
Published: 05 October 2022.
Edited by:
Parth Sarthi Sen Gupta, Indian Institute of Science Education and Research Berhampur (IISER), IndiaReviewed by:
Michal Letek, Universidad de León, SpainCopyright © 2022 Ponnusamy and Arumugam. This is an open-access article distributed under the terms of the Creative Commons Attribution License (CC BY). The use, distribution or reproduction in other forums is permitted, provided the original author(s) and the copyright owner(s) are credited and that the original publication in this journal is cited, in accordance with accepted academic practice. No use, distribution or reproduction is permitted which does not comply with these terms.
*Correspondence: Mohanapriya Arumugam, bW9oYW5hcHJpeWFhQHZpdC5hYy5pbg==
†ORCID: Nirmaladevi Ponnusamy, orcid.org/0000-0001-9282-3642
Mohanapriya Arumugam, orcid.org/0000-0002-1647-3182
Disclaimer: All claims expressed in this article are solely those of the authors and do not necessarily represent those of their affiliated organizations, or those of the publisher, the editors and the reviewers. Any product that may be evaluated in this article or claim that may be made by its manufacturer is not guaranteed or endorsed by the publisher.
Research integrity at Frontiers
Learn more about the work of our research integrity team to safeguard the quality of each article we publish.