- 1Department of Biochemistry and Biophysics, Texas A&M University, College Station, TX, United States
- 2Lyme Disease Biobank, Portland, OR, United States
- 3Department of Veterinary Pathobiology, School of Veterinary Medicine and Biomedical Sciences, Texas A&M University, College Station, TX, United States
Lyme disease (LD), the leading tick-borne disease in the Northern hemisphere, is caused by spirochetes of several genospecies of the Borreliella burgdorferi sensu lato complex. LD is a multi-systemic and highly debilitating illness that is notoriously challenging to diagnose. The main drawbacks of the two-tiered serology, the only approved diagnostic test in the United States, include poor sensitivity, background seropositivity, and cross-reactivity. Recently, Raman spectroscopy (RS) was examined for its LD diagnostic utility by our earlier proof-of-concept study. The previous investigation analyzed the blood from mice that were infected with 297 and B31 strains of Borreliella burgdorferi sensu stricto (s.s.). The selected strains represented two out of the three major clades of B. burgdorferi s.s. isolates found in the United States. The obtained results were encouraging and prompted us to further investigate the RS diagnostic capacity for LD in this study. The present investigation has analyzed blood of mice infected with European genospecies, Borreliella afzelii or Borreliella garinii, or B. burgdorferi N40, a strain of the third major class of B. burgdorferi s.s. in the United States. Moreover, 90 human serum samples that originated from LD-confirmed, LD-negative, and LD-probable human patients were also analyzed by RS. The overall results demonstrated that blood samples from Borreliella-infected mice were identified with 96% accuracy, 94% sensitivity, and 100% specificity. Furthermore, human blood samples were analyzed with 88% accuracy, 85% sensitivity, and 90% specificity. Together, the current data indicate that RS should be further explored as a potential diagnostic test for LD patients.
Introduction
Lyme disease (LD) is an emerging infectious disease, which was first recognized in the 1970s near Lyme, Connecticut (Steere et al., 1977). LD is caused by the spirochetal bacterium of the Borreliella burgdorferi sensu lato (B. burgdorferi s.l.) complex. It should be mentioned that, based on a number of molecular and phenotypic characteristics, the old genus name of the complex genospecies, Borrelia, was recently changed (although not without some scientific debate (Adeolu and Gupta, 2014; Barbour et al., 2017; Margos et al., 2017a; Margos et al., 2017b; Margos et al., 2018; Gupta, 2019; Margos et al., 2020; Barbour and Gupta, 2021)) to Borreliella in order to distinguish between B. burgdorferi s.l. and relapsing fever Borrelia spirochetes. The enzootic cycle of the LD spirochete is maintained by Ixodes ticks and mammalian reservoirs (Steere et al., 2016; Stone et al., 2017). The ixodid ticks, which are responsible for transmission of the LD pathogen in the United States, are Ixodes scapularis and Ixodes pacificus, whereas in Europe and Asia these are, respectively, Ixodes ricinus and Ixodes persulcatus (Rand et al., 2003).
The three main genospecies of B. burgdorferi s.l., Borreliella afzelii (B. afzelii), B. burgdorferi sensu stricto (B. burgdorferi s.s.), and Borreliella garinii (B. garinii), account for the majority of LD cases in the world (Baranton et al., 1992; Steere et al., 2016). Infection with B. burgdorferi s.s., the causative genospecies of LD in both the United States and Europe, often results in arthritis. In contrast, B. afzelii and B. garinii, which are other pathogenic genospecies found in Europe, predominantly cause dermatological and neurological symptoms, respectively (Van Dam et al., 1993; Steere, 2001; Steere et al., 2016).
LD is a complex, multi-systemic, and often slowly progressing illness, which is notoriously difficult to diagnose. With the exception of erythema migrans (EM), the pathognomonic but not-always-present skin lesion, other symptoms of early-stage LD are non-specific (Steere et al., 2016). During the early disseminated stage, LD patients may also develop lymphocytic meningitis, facial palsy, radiculoneuritis, and/or carditis (Pachner and Steere, 1985; Steere et al., 2016). The late disseminated stage of LD is characterized by arthritis and presents itself several months after the appearance of EM in untreated patients (Steere et al., 1987). Arthritis is less common among LD patients in Europe, where dermatological and neurological symptoms are dominant (Herzer, 1991).
LD is managed by treatment with oral antimicrobial agents over 10-28 days. In the United States, patients are treated with doxycycline, amoxicillin, or cefuroxime axetil, while azithromycin is used as the second-line antimicrobial agent (Lantos et al., 2021). For more advanced LD symptoms, like neuropathy and facial palsy, a 14-to-21-day course of intravenous ceftriaxone, cefotaxime, or penicillin is recommended. Non-steroidal anti-inflammatory drugs (NSAIDs) can be given with the antibiotics to manage the arthritis (Pachner et al., 1995; Arvikar and Steere, 2015). In some patients, arthritis, fatigue, and other symptoms can persist long after the conclusion of treatment (Shor et al., 2019).
To date, the only Food and Drug Administration-approved diagnostic tests for LD in the United States are based on serology. They are composed of highly sensitive (ELISA) and highly specific (Western blot) assays (the original technology) or two enzyme immunoassays (EIAs; the modified system) (Branda et al., 2011; Branda et al., 2017; Pegalajar-Jurado et al., 2018). However, this diagnostic system has numerous drawbacks. In addition to background seropositivity and cross-reactivity, the test sensitivity may significantly vary. In early-stage LD, for example, sensitivity for patients presenting with EM is only between 29% and 40%, though this number does increase as the infection progresses (>90%) (Hilton et al., 1999; Bacon et al., 2003; Aguero-Rosenfeld et al., 2005; Wormser et al., 2006; Molins et al., 2014; Lager et al., 2019; Horn et al., 2020). In contrast to the two-tiered system, other available diagnostic modalities (e.g., PCR, culture) are not recommended by the United States Centers for Disease Control and Prevention due to their inferior performance (Li et al., 2011; Moore et al., 2016; Lantos et al., 2021). For example, PCR was demonstrated to have a very low sensitivity (Alby and Capraro, 2015; Waddell et al., 2016; Nigrovic et al., 2019). Bacterial culture, which is considered the “gold” standard for many bacterial diseases, is time-consuming and is not often rewarding in a clinical setting (Steere, 2001; Steere et al., 2016).
Raman spectroscopy (RS) was recently explored for its diagnostic utility of LD (Farber et al., 2021). In our early proof-of-concept study, C3H mice, which is a common experimental mouse model of LD, were infected with wild-type strains of B. burgdorferi s.s., 297 or B31, or an infectious but attenuated (unable to establish persistent infection) B31-derived mutant, ΔvlsE. The 297 and B31 strains represented two (RST1 and RST2, respectively) out of the three major classes of B. burgdorferi s.s. isolated across the United States (Liveris et al., 1996; Liveris et al., 1999). Blood samples drawn prior to and weekly after mouse infection for eight consecutive weeks were analyzed by a confocal RS (Farber et al., 2021). The overall results demonstrated that RS was a promising method for detecting active and past B. burgdorferi infection in the blood with an average true positive rate being 86-89%. The present report, which is an expansion of our previous study, has included blood samples from C3H mice infected with either B. afzelii or B. garinii, the pathogenic European genospecies, or B. burgdorferi N40, a strain of the third major clade of B. burgdorferi s.s. (RST3) in the United States. Moreover, this study involved 90 serum samples from the Lyme Disease Biobank (LDB) that originated from LD-confirmed, LD-negative, and LD-probable human patients.
Methods
Bacteria and culture conditions
Borreliella strains were grown in Barbour-Stoenner-Kelly II medium supplemented with 6% rabbit serum (referred to here as BSK-II medium; Gemini Bio-Products, CA, USA) under 2.5% CO2 at 35°C. To culture animal tissues, the antimicrobial cocktail composed of 0.02 mg/mL phosphomycin, 0.05 mg/mL rifampicin, and 2.5 mg/mL amphotericin B was added to BSK-II medium in order to prevent bacterial and fungal contamination.
Mouse infection and blood sampling
A total of 20 male C3H/HeJ (C3H) mice of 4-6 weeks of age were purchased from the Jackson Laboratories (ME, USA). The mice were randomly split into four groups of five and, after a short adaption period, three groups were needle inoculated with 1.1x106 cells of B. afzelii strain PGau (referred to here as B. afzelii PGau; group A), B. garinii subsp. bavariensis PBi (referred to here as B. garinii PBi; group B), or B. burgdorferi s.s. strain N40 (referred to here as B. burgdorferi N40; group C) per animal. Five other C3H mice (group D) remained uninfected and served as an age- and sex-matched control. Each mouse group was caged separately. To verify the infection in the challenged animals, their blood and other tissues were harvested and cultured in BSK-II medium as described (Rogovskyy et al., 2016). Specifically, 50 μl of blood was sampled from each mouse via maxillary bleed at day 7 postinfection (pi). Biopsies of ear pinnae from the B. afzelii PGau- and B. garinii PBi-infected mice were harvested at day 21 pi. Ear tissues of B. burgdorferi N40-challenged animals were sampled at 28 pi. At day 56 pi, bladder, ear pinnae, heart, and tibiotarsal joints were also harvested and cultured in BSK-II medium as detailed (Rogovskyy et al., 2016). The presence or absence of viable spirochetes was confirmed by weekly observing the cultures via dark-field microscopy for four weeks. At days 0, 3 (except for B. afzelii PGau), 7, and weekly onwards until day 56 pi, 50 μl of blood was collected from each animal via cheek bleed. Individual blood samples were placed in sterile Eppendorf tubes, allowed to be clotted, and then stored at −80°C until RS analysis.
Human blood samples
Human whole blood samples (collected in EDTA tubes) were acquired from the Lyme Disease Biobank (LDB) (Horn et al., 2020). LDB is a repository of well-characterized samples, which was specifically established to facilitate research on LD and other medically important tick-borne diseases. For this study, a total of 90 blood specimens were obtained from LDB. Of them, 45 blood samples (referred to here as C samples) were acquired from LD patients, individuals enrolled with signs and symptoms of early LD, whose diagnosis was confirmed by the two-tiered testing algorithm, two positive ELISAs with EM > 5 cm, IgG seroconversion at the second draw, LD-positive culture and/or PCR (Table S1). The 30 serology-negative endemic control (EC) samples were obtained from healthy individuals without a history of LD or other tick-borne infections, who tested negative by all serologic tests. The 15 LD probable (P) samples were acquired from LD patients, who had been clinically diagnosed with LD (all developed EM lesions of > 5 cm) but were negative by the two-tiered testing algorithm (Table S1) (Horn et al., 2020).
Raman spectroscopy
To prepare them for RS, blood samples were melted by hand and then vortexed before 50 µL were spread in a thin layer on a foil-wrapped microscope slide. The aluminum foil was flattened with a razor blade before wrapping. Samples were dried for 30 minutes in a fume hood before scanning. Spectra were obtained using a home-built confocal Raman microscope with a 785 nm continuous-wave laser (Necsel, NJ, USA) with a laser power of ~8.0 mW. The laser beam was guided into the inverted microscope (Nikon TE-2000 U) via a set of mirrors and passed through a 50/50 beam splitter. The laser was focused on the dried blood surface through a 20X Nikon objective lens (NA = 0.45). The scattered light was directed through an LP02-785RE-25 long-pass filter (Semrock, NY, USA) to filter Rayleigh scattering, then passed to an IsoPlane SCT 320 spectrograph (Princeton Instruments, NJ, USA) equipped with a 600 groove/mm grating blazed at 750 nm. The light was then sent to a PIXIS:400BR CCD (Princeton Instruments). The sample was moved relative to the incident laser beam using an H117P2TE (Prior, MA, USA) motorized stage, controlled by a Prior Proscan II. A total of 19,235 Raman spectra were obtained throughout the experiment (spectral acquisition time was 30 s). During preprocessing, the spectra’s baseline was flattened and the area under the curve was normalized. The following spectra were what the partial least squares discriminant analysis (PLS-DA) model analyzed.
Statistical analysis
Analysis of variance (ANOVA) was performed in MATLAB or the MATLAB add-on PLS_Toolbox (Eigenvector Research, Inc., WA, USA). Raw spectra were first averaged in groups of five to reduce noise. These averaged spectra were then baseline-corrected using the Automatic Weighted Least Squares algorithm with a 6th order polynomial then normalized to the total area under the curve of the spectrum.
Partial least squares-discriminant analysis (PLS-DA) was used to differentiate between timepoints after infection for each genospecies and the uninfected control. Then, 45 binary models per genospecies/uninfected control were generated with the exception of B. afzelii PGau because day 3 postinfection samples were not available. Binary models were also constructed comparing each time point for each of the genospecies to all the control spectra. The best performing model was then recorded, based on the highest Matthew’s Correlation Coefficient (MCC), which has been found to be a more robust way of evaluating binary models than either F1 score or accuracy because it takes into account all four values in the confusion matrix (Chicco and Jurman, 2020; Yao and Shepperd, 2020; Chicco et al., 2021). In order to determine whether the peaks were significantly different, Kruskal-Wallis one-way ANOVA was conducted on the peaks used by the PLS-DA model as shown by the loading plots from the PLS_toolbox. Differences were considered statistically significant at a P value of 0.05. Post-hoc Tukey HSD tests were performed to generate 95% confidence intervals.
Results
Mouse infection
Although all 15 blood samples taken at day 7 pi were culture-negative, the successful infection of three groups of C3H mice (A, B, and C) was confirmed by culture-positive results of ear pina biopsies harvested at day 21 (B. afzelii PGau and B. garinii PBi) or 28 (B. burgdorferi N40) pi. The presence of long-term infection was verified by positive cultures of mouse tissues harvested at day 56 pi for all B. afzelii PGau- and B. burgdorferi N40-infected mice (Table 1). However, a long-term infection by B. garinii PBi was only detected in two out of 5 mice as shown by positive cultures of their ear tissues. The lack of culture-detectable B. garinii PBi may suggest that the three mice had either cleared the infection, or the numbers of spirochetes were low (under the threshold of detection by culture) at the time the mouse tissues were collected.
The analysis of mouse blood by Raman spectroscopy
Overall, the Raman spectra collected from blood of mice infected with B. afzelii PGau, B. garinii PBi, or B. burgdorferi N40 exhibited vibrational bands that could be assigned to proteins, heme, sugars, carotenoids, and other biomolecules (Figure 1 and Table 2). Regardless of the Borreliella genospecies and time points the blood was collected at, only small changes in the relative intensities of the vibrational bands were observed.
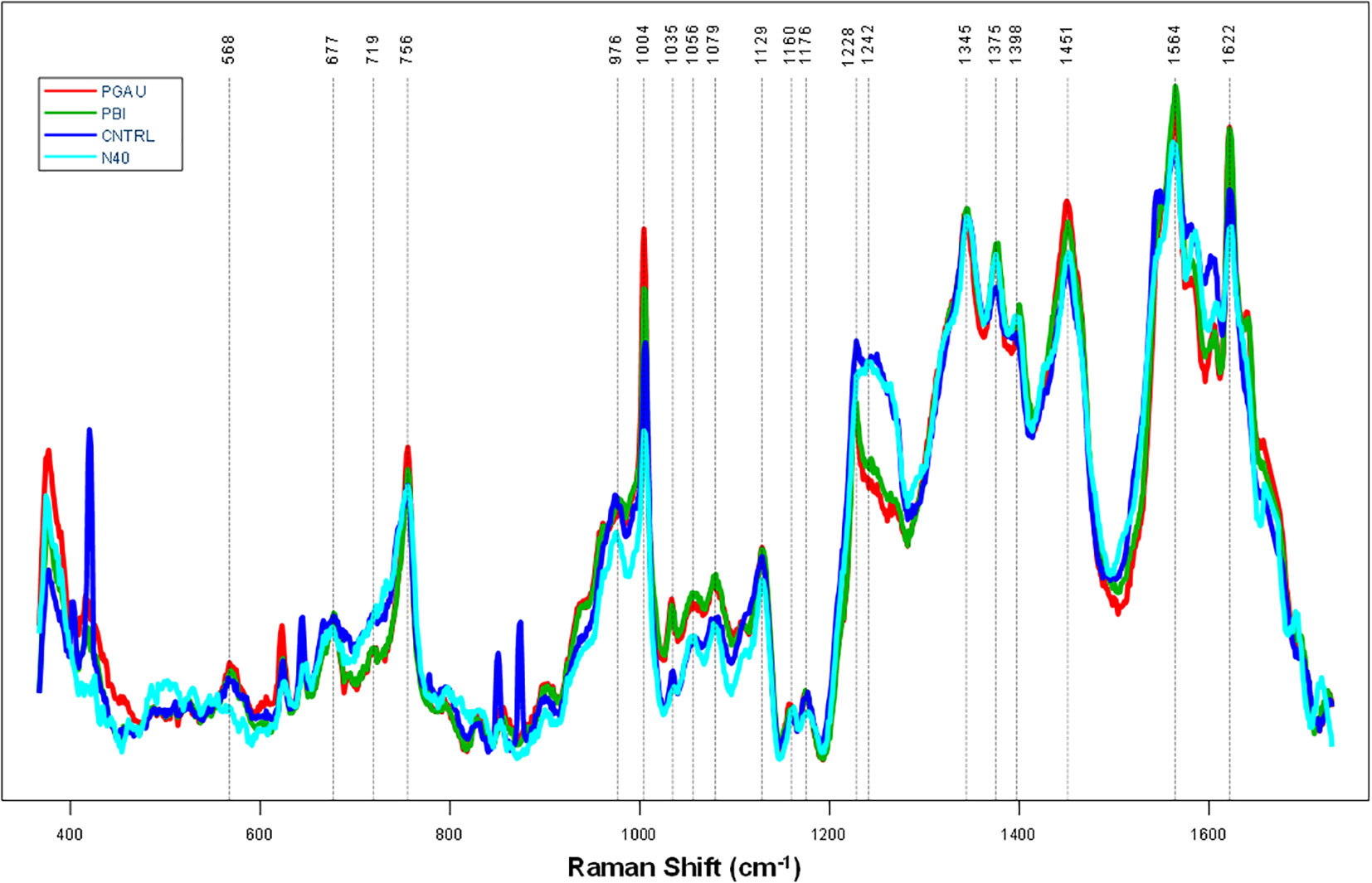
Figure 1 The average preprocessed (Automatic Whitaker Filter baselined and area normalized) Raman spectra for blood samples taken from Borreliella-infected C3H mice at day 56 postinfection. C3H mice (5 animals per group) were infected with B afzelii PGau (PGAU), B garinii PBi (PBI), or B burgdorferi N40 (N40). One group of mice remained uninfected and served as a control (CNTR).
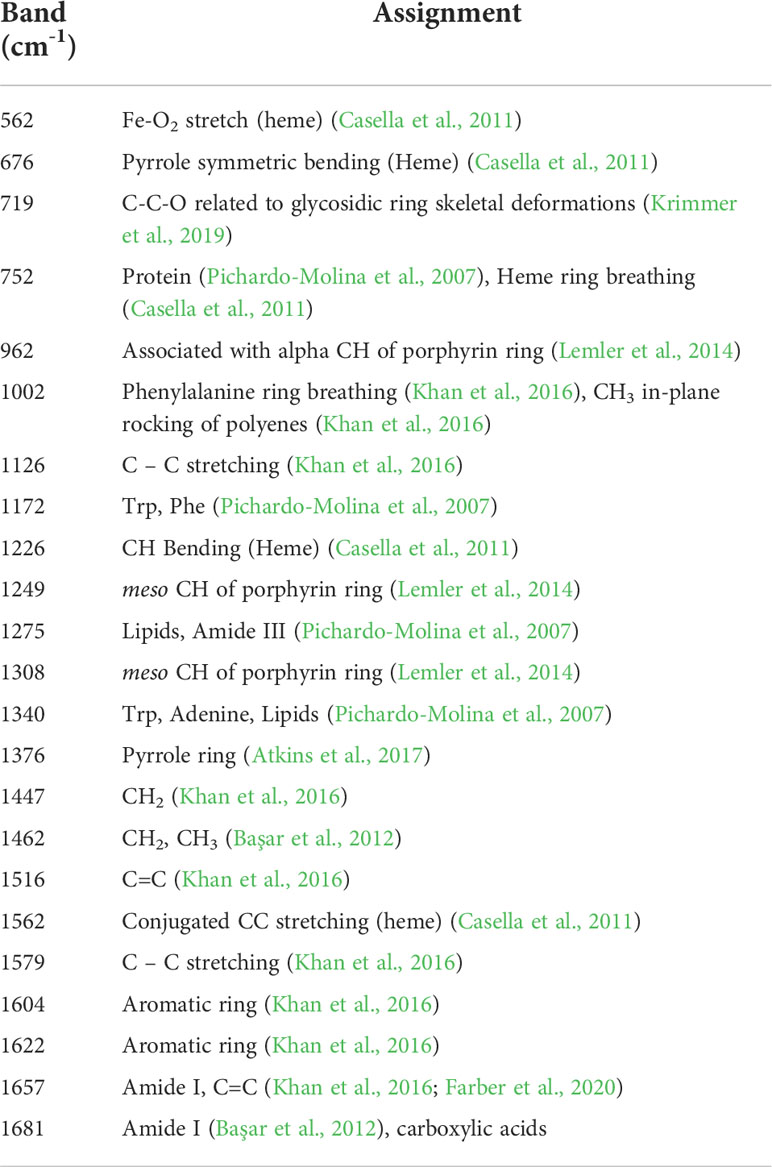
Table 2 Assignments of vibrational bands for the Raman spectra acquired from blood samples of Borreliella-infected C3H mice.
By using our previously developed PLS-DA method (Farber et al., 2021), the prediction accuracy for the collected spectra was determined. As demonstrated by the MCC (Figure 2), the ability of the model to detect B. garinii PBi infection in C3H mice at day 7 pi was only 46.8%. However, the MCC increased with progression of the mouse infection, reaching 86.1% at day 56 pi (Figure 2). Similar results were obtained for the accuracy of detection of mouse infection with B. afzelii PGau. Specifically, the diagnostic ability of the model increased from 58% for day 7 to 78% for day 35 pi. Surprisingly, there was a drastic decrease in the detection accuracy for day 42 pi (67%), whereas the MCC for days 49 and 56 pi was 87% (Figure 2). In contrast, the model, which was used to differentiate between the control blood from uninfected mice and the blood sampled from B. burgdorferi N40-infected animals, showed no clear trend with MCC values being consistently high for all time points, including day 0 (pre-infection).
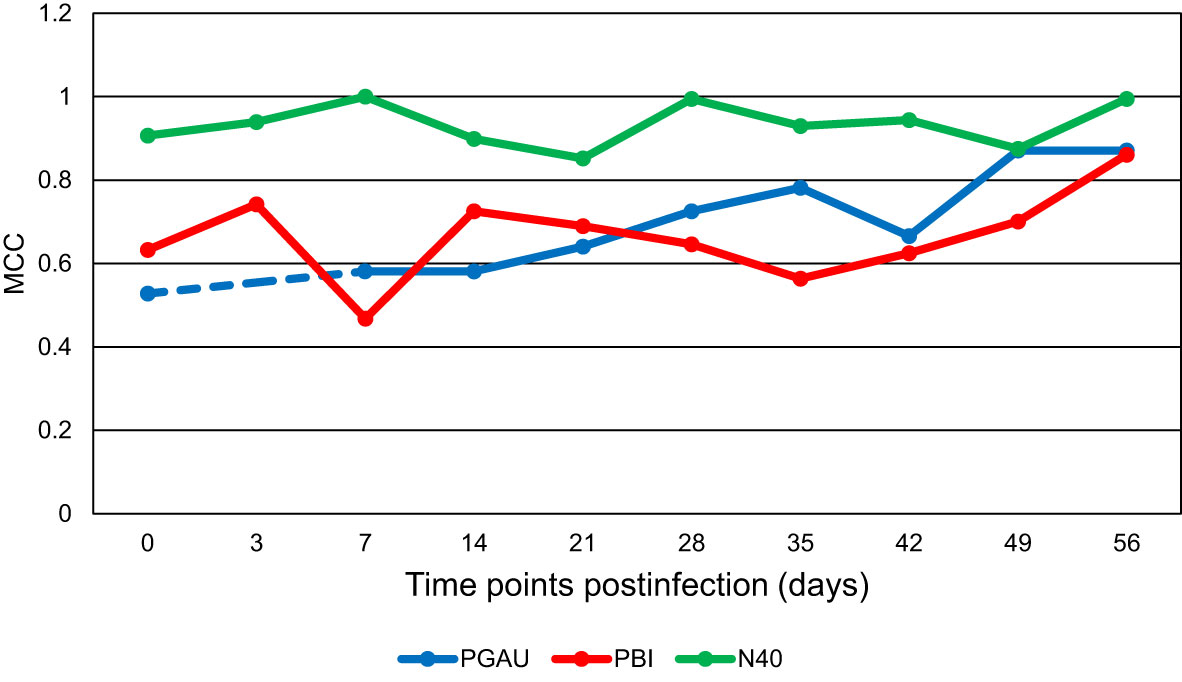
Figure 2 The Matthew’s Correlation Coefficient (MCC) for the binary model comparing the uninfected control to each of the three Borreliella genospecies over time. Mouse blood was sampled from C3H mice prior to their infection and postinfection with B afzelii PGau (PGAU), B garinii PBi (PBI), or B burgdorferi N40 (N40). The blood was also collected from age- and sex-matched uninfected (control) C3H mice. The dashed line indicates that the data are missing.
The acquired RS data were also combined to generate the binary model that would differentiate between the blood from all Borreliella-infected mice and the blood of uninfected control animals for each time point (Figure 3). The result showed that the only model with high predictability was the model for day 56 pi (MCC of 89%). The MCC values for the models of the other time points were consistently low (MCC of 34-58%). For the day 56 model, the odds ratio was calculated to be 3069.3 (z=5.584, p<0.0001) with the accuracy, sensitivity, and specificity being 96%, 94% and 100%, respectively. Similarly, as calculated by F1, the models of the earlier time points demonstrated a low diagnostic capacity (51-70%), whereas the day 56 model more accurately differentiated all Borreliella-infected mice from the respective uninfected controls (87%; Figure 4).
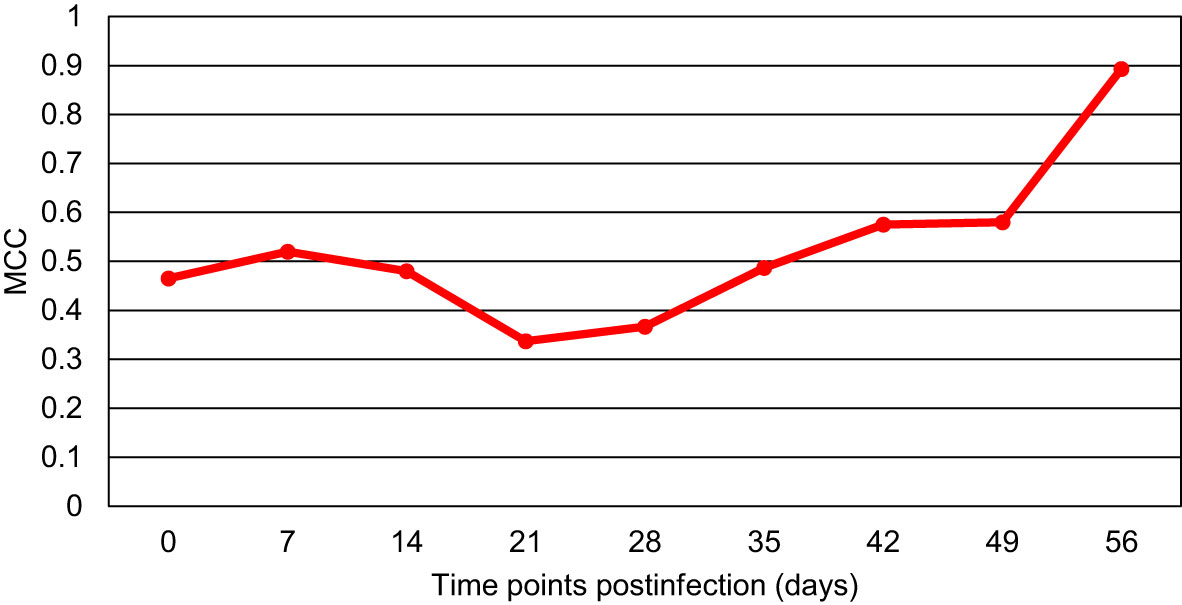
Figure 3 The Matthew’s Correlation Coefficient (MCC) for the binary model comparing blood samples of all Borreliella-infected mice to blood samples of all uninfected controls for each time point. Mouse blood was sampled from C3H mice prior to their infection and postinfection with B afzelii Pgau (PGAU), B garinii PBi (PBI), or B burgdorferi N40 (N40). The blood was also collected from age- and sex-matched uninfected (control) C3H mice.
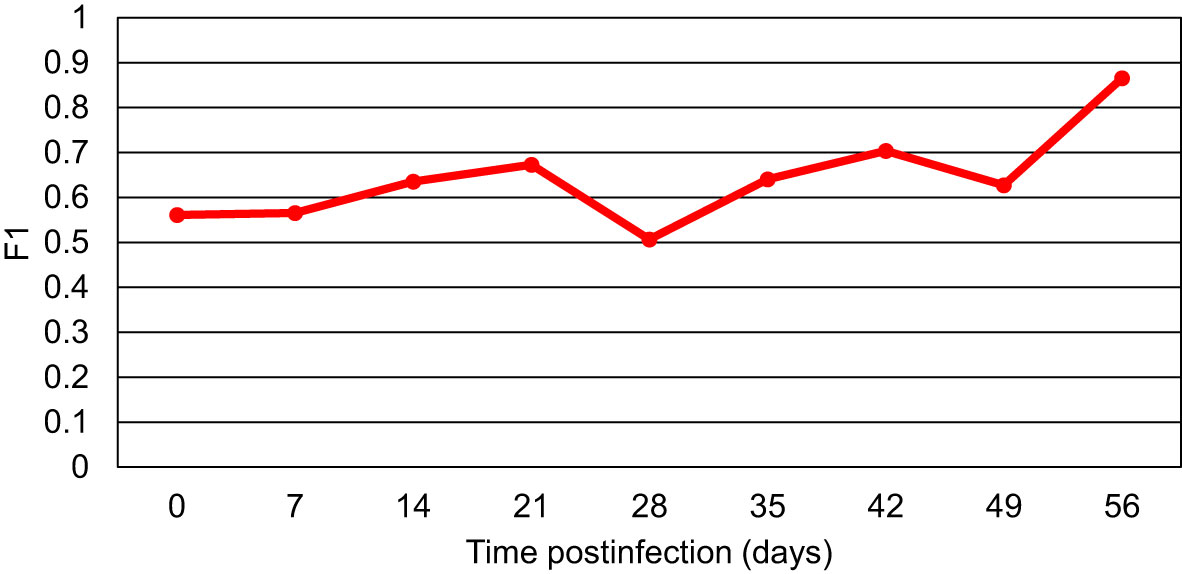
Figure 4 The F1 scores for the models comparing blood samples of all Borreliella-infected mice to blood samples of all uninfected controls for each time point. Mouse blood was sampled from C3H mice prior to their infection and postinfection with B afzelii Pgau (PGAU), B garinii PBi (PBI), or B burgdorferi N40 (N40). The blood was also collected from age- and sex-matched uninfected (control) C3H mice.
In order to examine the capacity of RS to differentiate different timepoints of mouse infection, the Raman spectra acquired from weekly blood samples were compared within each genospecies. The overall results demonstrated that MCC ranges were 66-100%, 68-100%, and 80-100% for B. afzelii PGau, B. garinii PBi, and B. burgdorferi N40, respectively (Tables 3-5). The frequency at which the timepoints were differentiated with the highest accuracy (MCC of 100%) was much higher for blood samples obtained from B. burgdorferi N40- or B. garinii PBi-infected mice (53% and 31%, respectively). In contrast, when weekly samples from B. afzelii PGau-infected animals were compared, only one comparison (day 0 vs 42 pi) had an MCC of 100%. However, the MCC values of 90-99% were more frequently observed for infection with B. afzelii PGau (58%; Table 3) than that of B. garinii PBi (42%; Table 4) or B. burgdorferi N40 (33%; Table 5). Overall, the timepoints of mouse infection were differentiated with the highest accuracy (MCC of 90-100%) for B. burgdorferi N40 (87%) followed by B. garinii PBi (73%) and B. afzelii PGau (61%).
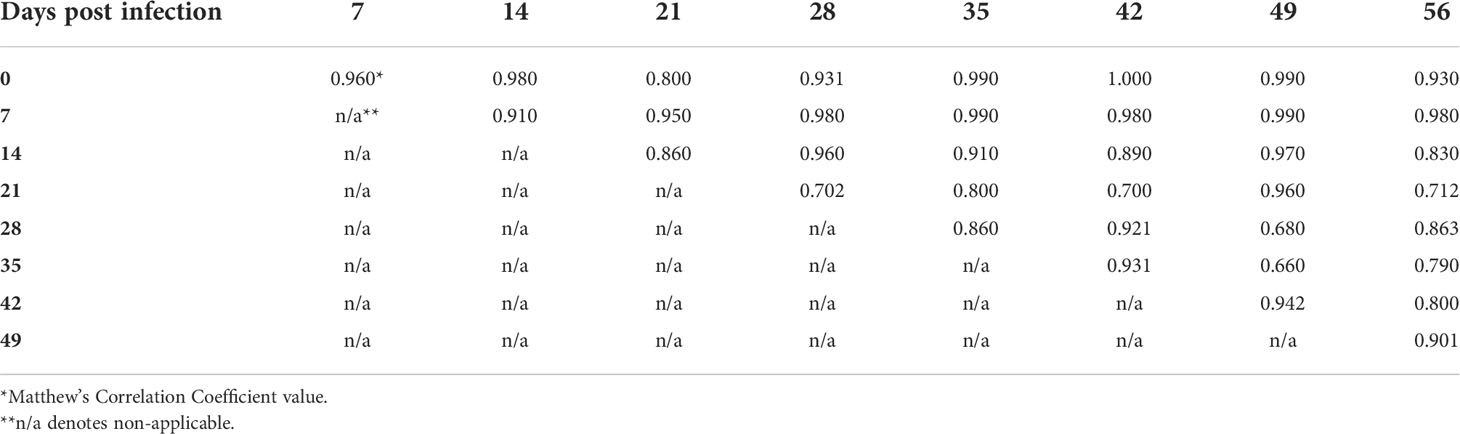
Table 3 The accuracy of identification of B. afzelii PGau infection in C3H mice at different time points postinfection as measured by the Matthew’s Correlation Coefficient.
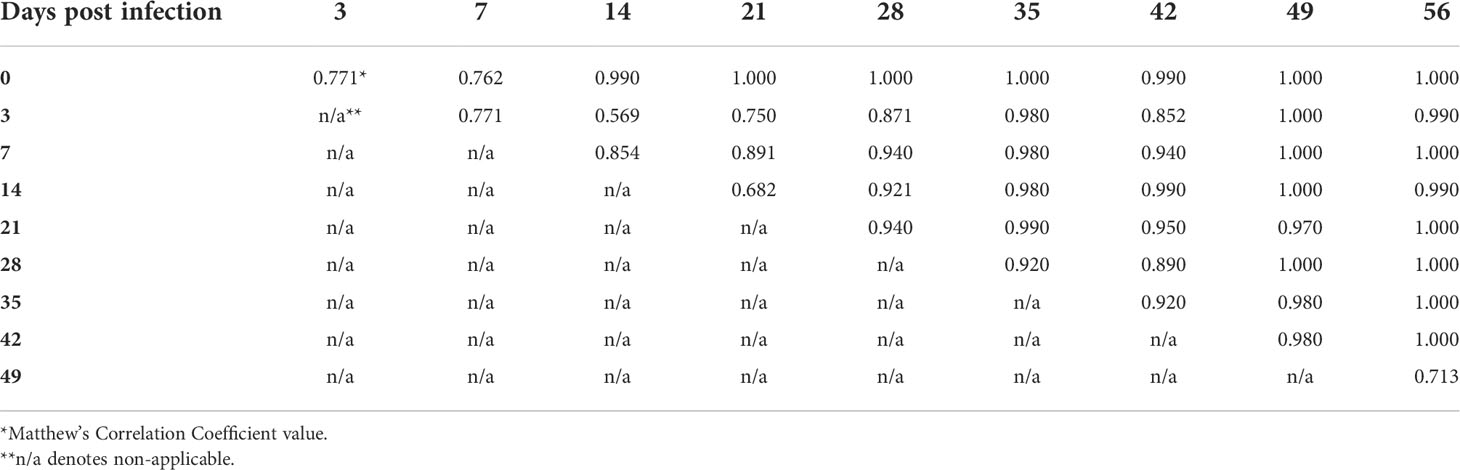
Table 4 The accuracy of identification of B. garinii PBi infection in C3H mice at different time points postinfection as measured by the Matthew’s Correlation Coefficient.
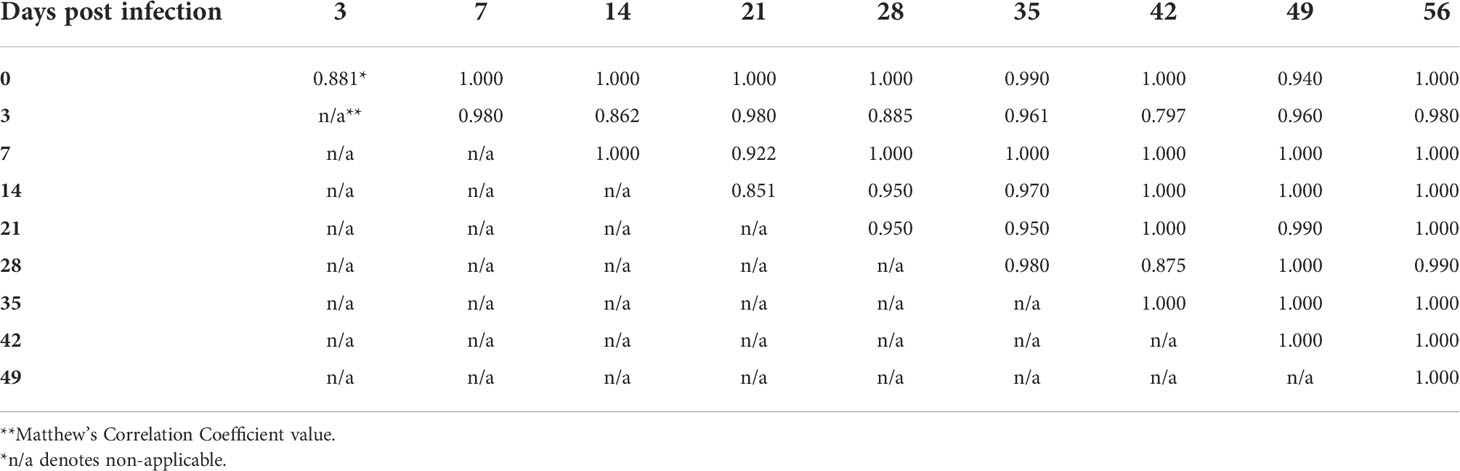
Table 5 The accuracy of identification of B. burgdorferi N40 infection in C3H mice at different time points postinfection as measured by the Matthew’s Correlation Coefficient.
The analysis of human blood by Raman spectroscopy
To further explore the diagnostic capacity of RS, human blood samples acquired from LDB were analyzed (Horn et al., 2020). A total of 45 C, 30 EC, and 15 P samples were included in this study. As a result of the RS analysis, 8198 spectra were collected from the 90 human blood samples. In order to determine the capacity of RS to differentiate between the C and EC samples, the respective spectra were analyzed and compared by our previously developed PLS-DA model (Farber et al., 2021). The results demonstrated that the PLS-DA model identified the healthy individuals (the EC samples) and LD patients (the C samples) with the accuracy of 74% and 77%, respectively (Figure 5). To increase the PLS-DA accuracy, signal-to-noise quality of spectra was improved by averaging the spectra. When 9 or 11 spectra were averaged together in one group, the accuracy of identifying blood samples of the healthy individuals and LD patients increased to 85% of and 90%, respectively (Figure 5). The improved PLS-DA model was further applied to analyze the 15 P samples (the patients who were clinically diagnosed with EM > 5 cm but tested negative by the two-tiered testing algorithm). Based on their matching scores, samples P11, P12, and P13 were assigned to the C sample group with a high probability (91%, 98%, and 87%, respectively) (Table 6). In contrast, samples P1-P5 had low MCC values (0-1%) and, therefore, were assigned to the EC sample group (Table 6). Samples P6-P10 could be assigned to the C sample group with MCC values of 66-79%. The MCC values of P14 and P15 were 33% and 44%, respectively, making their group assignment undetermined (Table 6).
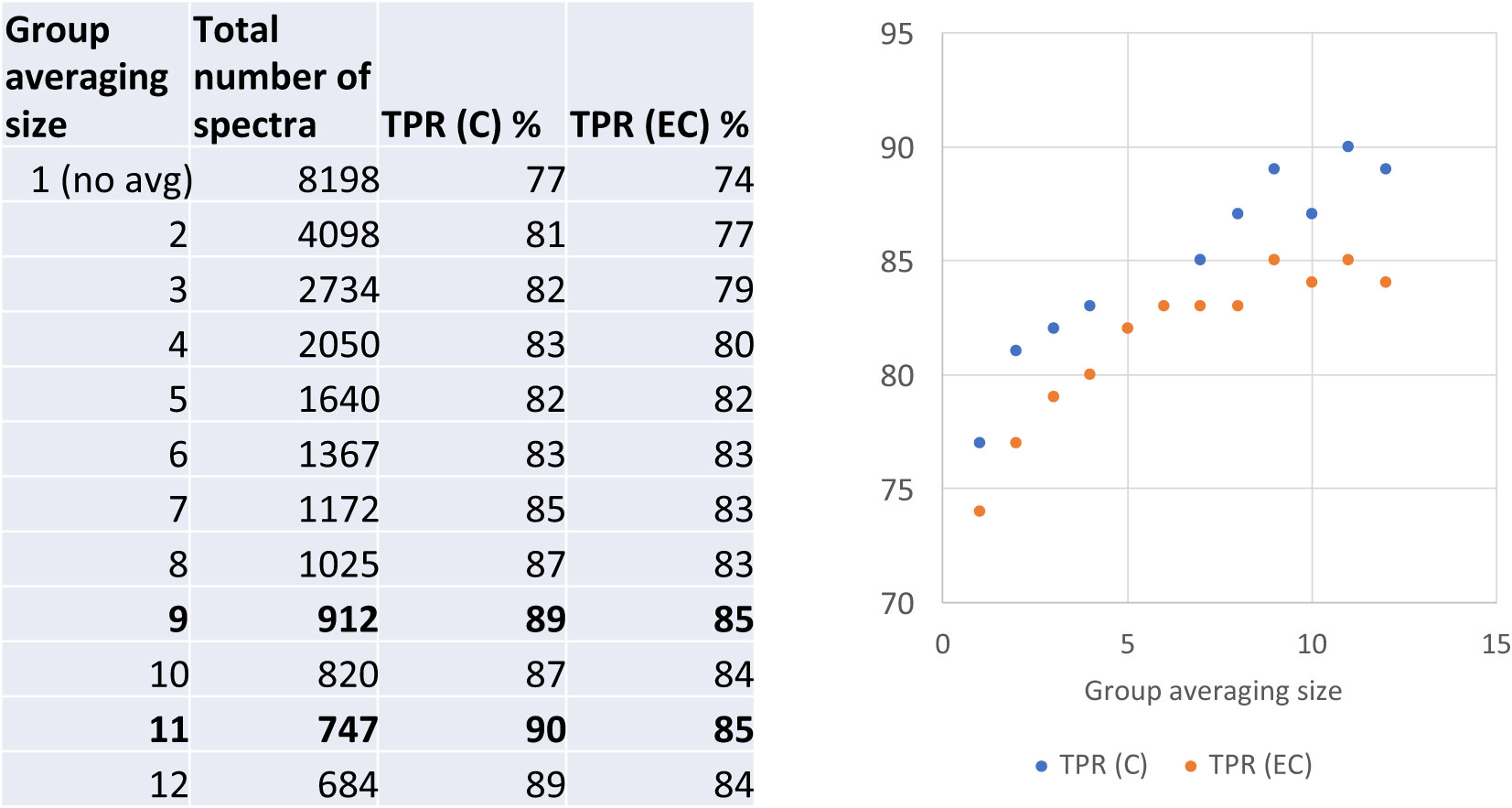
Figure 5 The accuracy of differentiation between serologically negative (EC) and LD-confirmed (C) groups of human blood samples when averaged in different sized groups. TPR denotes a true positive rate.
After all models were created, ANOVA was performed on the pre-processed spectra in order to detect any significant differences in the intensity of Raman scattering for various peaks. Specifically, the analysis involved a total of 15 peaks, which were most used by each model. To differentiate between the three Borreliella genospecies, loading plots outputted by the PLS-DA were utilized, and the following peaks were identified: 420, 643, 745, 874, 973, 1008, 1249, 1351, 1394, 1426, 1454, 1540, 1592, 1627, and 1782 cm-1. Tables S2 through S5 show the results of the ANOVA and post-hoc Tukey HSD test (significant vs non-significant) for each peak and model tested. The ANOVA results demonstrated that each generated model had at least 9 significantly different peaks, and that, for four models, significant difference was observed for all 15 peaks (Tables S2-S5). Together, the results indicated that the models generated in this study were statistically significant.
Discussion
The results of the present study were consistent with our previous findings, confirming that RS coupled with chemometrics could identify Borreliella-induced infection in C3H mice with high accuracy (Farber et al., 2021). Although RS was previously shown to differentiate the blood from Borreliella-infected mice from pre-infection samples after the infection had been cleared (Farber et al., 2021), it is still possible that low numbers of wild-type spirochetes could have been present in the mouse blood samples tested in the current study. Overall, the present data suggested that RS had sufficient discriminating capacity to differentiate between early and late stages (timepoints) of LD infection in the mouse model. The combined results of our studies also showed that mouse infection by a representative strain (297, B31, or N40) of the three major clades of B. burgdorferi s.s. (RST1, RST2, and RST3, respectively) in the United States (Liveris et al., 1996; Liveris et al., 1999) could be equally detected by RS. In addition to B. burgdorferi s.s., the current data demonstrated that RS could also identify mouse infection of additional genospecies of B. burgdorferi s.l., B. afzelii and B. garinii, though the accuracy of their detection was only high for the late stage (day 56 pi). The reason for the latter is not obvious and requires further investigation. Overall, blood sampled from Borreliella-infected mice was detected with 96% accuracy, 94% sensitivity, and 100% specificity. Similarly, human blood samples were analyzed with 88% accuracy, 85% sensitivity, and 90% specificity. The observed differences were most likely due to variability that human samples inevitably introduced (e.g., bacterial strain, infectious dose, stage of infection, patient immune status, treatment) as opposed to samples that were generated from inbred mice under the well-controlled study. This human sample variability may also account for different MCC values obtained from the P samples. Collectively, the present results indicate that RS could be further explored as a follow-up assay for those patients, who are clinically diagnosed as LD-positive (due to their erythema migrans of > 5 cm) and yet test negative by the existing two-tier serologic testing.
In addition to our published study (Farber et al., 2021), two other subsequent investigations that had also examined the RS capacity for LD diagnostics were recently published (Senger et al., 2022; Tabb et al., 2022). One study compared Raman spectra acquired from urine samples of 30 serology-confirmed LD patients against urine spectra from 235 LD-negative (healthy) individuals, 362 patients with end-stage kidney disease, and 17 bladder cancer patients (Senger et al., 2022). As a result, the study demonstrated that RS could distinguish the LD-confirmed patients from the other groups with 87-88% accuracy, 83-87% sensitivity, and 87-90% specificity (Senger et al., 2022). These assay characteristics were almost identical to those of the present RS analysis of the human blood samples.
The other recent investigation has examined the capacity of surface enhanced Raman scattering (referred to here as SERS) to directly detect Borreliella spirochetes in serum samples of LD pediatric patients (Tabb et al., 2022). Outer surface protein A (OspA), a surface lipoprotein whose function is to mediate the attachment of spirochetes to the tick midgut (Fingerle et al., 1995; Schwan et al., 1995), was chosen as a target of DNA aptamers. By testing 24 LD-negative and 23 LD-positive samples, this approach showed higher accuracy (94%), sensitivity (91%) and selectivity (96%) than the presently and previously utilized indirect RS-based detection methods (Senger et al., 2022). However, the results of this OspA aptamer-based SERS are quite surprising. The seminal study clearly demonstrated that OspA is not expressed during mammalian (mouse) infection (Ohnishi et al., 2001). Thus, in sera of LD patients, the chosen OspA target is either absent, or at best is present at very low levels. The latter was only shown by a single study that involved sera from a very limited number of patients (n=3) (Cheung et al., 2015). Furthermore, in addition to its complicated and time consuming sample preparation (Tabb et al., 2022), it is unknown how serum handling, freeze-thaw, and long-term storage affect the stability of peptide targets for SERS (Dufresne et al., 2017).
Overall, there are two overarching caveats of the present and above-mentioned studies (Farber et al., 2021; Senger et al., 2022; Tabb et al., 2022). First, the clinical samples have not been tested in a blind manner. Second, the studies did not include samples from LD-negative patients, who would be diagnosed with other (tick-borne) infectious diseases. An adequate evaluation of RS specificity is, therefore, highly warranted. To achieve this, future studies should include blood samples both from mice experimentally infected with various pathogens and humans with confirmed diagnosis of the respective diseases.
It should be noted that the current study has other limitations. First, due to a large scale of work, the RS data were collected by different persons, which unavoidably introduced some variability. To minimize the latter, the process of RS scanning could be automated (Lopez-Reyes and Rull Pérez, 2017; O’dwyer et al., 2021). Second, as with many biological materials, the Raman spectra collected from the blood were relatively noisy, most likely due to such factors as fluorescence and chemical complexity of biological samples (Lin et al., 2012; Leon Bejarano et al., 2017; Barton and Hennelly, 2018; Jahn et al., 2021). Although the inter-scanner variability and noise were reduced through the averaging of spectra, the issue still persisted in the present study. To further reduce the noise, vials composed of quartz could be used for the direct scanning of blood. A quartz vial would allow for a thicker layer of blood compared to the foil-wrapped slide used in the present study and would avoid the issue of interference. It was shown that quartz does not exhibit Raman fluorescence or heavy Raman scattering beyond 500 cm-1 Raman shift (Tuschel, 2016). Third, some peaks may have hindered an accurate identification of component compounds. For example, the peak at 1657 cm-1 could represent vibrations from an amide bond, characteristic of all proteins, or an alkene group in unsaturated fatty acids (Khan et al., 2016; Farber et al., 2020; Farber et al., 2021). To accurately identify compounds and their functional groups, high performance liquid chromatography (HPLC) could potentially be applied (Dou et al., 2021; Higgins et al., 2022). Previous utilization of HPLC coupled to mass spectrometry has successfully identified changes in the metabolic profile of the blood from patients diagnosed with early-stage LD (Molins et al., 2015). A vast majority of the molecular species identified in LD patients’ blood were lipids or their derivatives (e.g., cholesterol, cholesteryl acetate, diacylglycerol, phospholipids, sphingolipids, triglycerides) (Molins et al., 2015).
Together, the results of the current and other studies (Farber et al., 2021; Senger et al., 2022; Tabb et al., 2022) are highly encouraging, as they consistently suggested that RS could potentially be utilized as a diagnostic test for LD patients. The transitioning from using a large RS instrument (confocal Raman spectrometer) used in this study to a hand-held, easy-to-operate Raman spectrometer would be another next step towards utilization of RS for LD diagnostics. Given the portability and easy sample preparation process (the spreading of blood onto a foil-wrapped microscope slide), the testing of blood samples by RS may ultimately be deployed in medically underserved areas that often lack access to diagnostic laboratories.
Data availability statement
The raw data supporting the conclusions of this article will be made available by the authors, without undue reservation.
Ethics statement
The mouse experimental procedures were approved by the Institutional Animal Care and Use Committee of Texas A&M University. All experiments were performed in accordance with Public Health Service (PHS) Policy on Humane Care and Use of Laboratory Animals (2002), Guide for the Care and Use of Agricultural Animals in Research and Teaching (2010), and Guide for the Care and Use of Laboratory Animals (2011). Institutional review board (IRB) approval was obtained for each site through the LDB sponsor protocol (Advarra IRB protocol Pro00012408) or the site’s local IRB.
Author contributions
NG was involved in data collection, analysis, writing, and project management. TD was involved in data analysis. RM was involved in the collection of spectra from human blood. SH and KM were involved in data collection. EH provided resources and was involved in the study design and edited the manuscript. AR concepted and developed the study and wrote the manuscript. DK concepted and developed the study, oversaw the project, and edited the manuscript. All authors contributed to the article and approved the submitted version.
Funding
Funding for this study was provided by the 2020 Bay Area Lyme Foundation’s Emerging Leader Fund, 2022 Bay Area Lyme Foundation award, and the Texas A&M AgriLife Research Insect Vector Disease Seed Grant.
Acknowledgments
The authors would like to thank Maliha Batool for her help with the mouse experiments, and Bridget Bedore, Natalia Ayala, Stephen Parlamas and Dillon Humpal for their assistance with the acquisition of Raman spectra. We are grateful to Troy Bankhead, Jenifer Coburn, Richard Marconi, Ulrike Munderloh, and Jon Skare for providing the bacterial strains for this study.
Conflict of interest
The authors declare that the research was conducted in the absence of any commercial or financial relationships that could be construed as a potential conflict of interest.
Publisher’s note
All claims expressed in this article are solely those of the authors and do not necessarily represent those of their affiliated organizations, or those of the publisher, the editors and the reviewers. Any product that may be evaluated in this article, or claim that may be made by its manufacturer, is not guaranteed or endorsed by the publisher.
Supplementary material
The Supplementary Material for this article can be found online at: https://www.frontiersin.org/articles/10.3389/fcimb.2022.1006134/full#supplementary-material
References
Adeolu, M., Gupta, R. S. (2014). A phylogenomic and molecular marker based proposal for the division of the genus Borrelia into two genera: The emended genus Borrelia containing only the members of the relapsing fever Borrelia, and the genus Borreliella gen. nov. containing the members of the Lyme disease Borrelia (Borrelia burgdorferi sensu lato complex). Antonie Van Leeuwenhoek 105, 1049–1072. doi: 10.1007/s10482-014-0164-x
Aguero-Rosenfeld, M. E., Wang, G., Schwartz, I., Wormser, G. P. (2005). Diagnosis of Lyme borreliosis. Clin. Microbiol. Rev. 18, 484–509. doi: 10.1128/CMR.18.3.484-509.2005
Alby, K., Capraro, G. A. (2015). Alternatives to serologic testing for diagnosis of Lyme disease. Clin. Lab. Med. 35, 815–825. doi: 10.1016/j.cll.2015.07.005
Arvikar, S. L., Steere, A. C. (2015). Diagnosis and treatment of Lyme arthritis. Infect. Dis. Clin. North Am. 29, 269–280. doi: 10.1016/j.idc.2015.02.004
Atkins, C. G., Buckley, K., Blades, M. W., Turner, R. F. B. (2017). Raman spectroscopy of blood and blood components. Appl. Spectrosc. 71, 767–793. doi: 10.1177/0003702816686593
Bacon, R. M., Biggerstaff, B. J., Schriefer, M. E., Gilmore, R. D., Jr., Philipp, M. T., Steere, A. C., et al. (2003). Serodiagnosis of Lyme disease by kinetic enzyme-linked immunosorbent assay using recombinant VlsE1 or peptide antigens of Borrelia burgdorferi compared with 2-tiered testing using whole-cell lysates. J. Infect. Dis. 187, 1187–1199. doi: 10.1086/374395
Baranton, G., Postic, D., Saint Girons, I., Boerlin, P., Piffaretti, J.-C., Assous, M., et al. (1992). Delineation of Borrelia burgdorferi sensu stricto, Borrelia garinii sp. nov., and group VS461 associated with Lyme borreliosis. Int. J. Syst. Evol. Microbiol. 42, 378–383. doi: 10.1099/00207713-42-3-378
Barbour, A. G., Adeolu, M., Gupta, R. S. (2017). Division of the genus Borrelia into two genera (corresponding to Lyme disease and relapsing fever groups) reflects their genetic and phenotypic distinctiveness and will lead to a better understanding of these two groups of microbes (Margos et al., (2016) there is inadequate evidence to support the division of the genus Borrelia. int. j. syst. evol. microbiol. doi: 10.1099/ijsem.0.001717). Int. J. Syst. Evol. Microbiol. 67, 2058–2067. doi: 10.1099/ijsem.0.001717)
Barbour, A. G., Gupta, R. S. (2021). The family Borreliaceae (Spirochaetales), a diverse group in two genera of tick-borne spirochetes of mammals, birds, and reptiles. J. Med. Entomol. 58, 1513–1524. doi: 10.1093/jme/tjab055
Barton, S. J., Hennelly, B. M. (2018). “Signal to noise ratio of raman spectra of biological samples,” in SPIE photonics Europe (Strousbourg, France: SPIE).
Başar, G., Parlatan, U., Şeninak, Ş., Günel, T., Benian, A., Kalelioğlu, I. (2012). Investigation of preeclampsia using raman spectroscopy. J. Spectrosc. 27, 239–252. doi: 10.1155/2012/376793
Branda, J. A., Linskey, K., Kim, Y. A., Steere, A. C., Ferraro, M. J. (2011). Two-tiered antibody testing for Lyme disease with use of 2 enzyme immunoassays, a whole-cell sonicate enzyme immunoassay followed by a VlsE C6 peptide enzyme immunoassay. Clin. Infect. Dis. 53, 541–547. doi: 10.1093/cid/cir464
Branda, J. A., Strle, K., Nigrovic, L. E., Lantos, P. M., Lepore, T. J., Damle, N. S., et al. (2017). Evaluation of modified 2-tiered serodiagnostic testing algorithms for early Lyme disease. Clin. Infect. Dis. 64, 1074–1080. doi: 10.1093/cid/cix043
Casella, M., Lucotti, A., Tommasini, M., Bedoni, M., Forvi, E., Gramatica, F., et al. (2011). Raman and SERS recognition of β-carotene and haemoglobin fingerprints in human whole blood. Spectrochim. Acta Part A: Mol. Biomol. Spectrosc. 79, 915–919. doi: 10.1016/j.saa.2011.03.048
Cheung, C. S., Anderson, K. W., Benitez, K. Y., Soloski, M. J., Aucott, J. N., Phinney, K. W., et al. (2015). Quantification of Borrelia burgdorferi membrane proteins in human serum: A new concept for detection of bacterial infection. Anal. Chem. 87, 11383–11388. doi: 10.1021/acs.analchem.5b02803
Chicco, D., Jurman, G. (2020). The advantages of the matthews correlation coefficient (MCC) over F1 score and accuracy in binary classification evaluation. BMC Genomics 21, 6. doi: 10.1186/s12864-019-6413-7
Chicco, D., Starovoitov, V., Jurman, G. (2021). The benefits of the matthews correlation coefficient (MCC) over the diagnostic odds ratio (DOR) in binary classification assessment. IEEE Access 9, 47112–47124. doi: 10.1109/ACCESS.2021.3068614
Dou, T., Sanchez, L., Irigoyen, S., Goff, N., Niraula, P., Mandadi, K., et al. (2021). Biochemical origin of raman-based diagnostics of huanglongbing in grapefruit trees. Front. Plant Sci. 12. doi: 10.3389/fpls.2021.680991
Dufresne, J., Hoang, T., Ajambo, J., Florentinus-Mefailoski, A., Bowden, P., Marshall, J. (2017). Freeze-dried plasma proteins are stable at room temperature for at least 1 year. Clin. Proteomics 14, 35. doi: 10.1186/s12014-017-9170-0
Farber, C., Morey, R., Krimmer, M., Kurouski, D., Rogovskyy, A. S. (2021). Exploring a possibility of using raman spectroscopy for detection of Lyme disease. J. Biophotonics 14, e202000477. doi: 10.1002/jbio.202000477
Farber, C., Sanchez, L., Rizevsky, S., Ermolenkov, A., Mccutchen, B., Cason, J., et al. (2020). Raman spectroscopy enables non-invasive identification of peanut genotypes and value-added traits. Sci. Rep. 10, 7730. doi: 10.1038/s41598-020-64730-w
Fingerle, V., Hauser, U., Liegl, G., Petko, B., Preac-Mursic, V., Wilske, B. (1995). Expression of outer surface proteins A and C of Borrelia burgdorferi in Ixodes ricinus. J. Clin. Microbiol. 33, 1867–1869. doi: 10.1128/jcm.33.7.1867-1869.1995
Gupta, R. S. (2019). Distinction between Borrelia and Borreliella is more robustly supported by molecular and phenotypic characteristics than all other neighbouring prokaryotic genera: Response to margos’ et al. “The genus Borrelia reloaded” (PLoS ONE 13(12): e0208432). PloS One 14, e0221397. doi: 10.1371/journal.pone.0221397
Herzer, P. (1991). Joint manifestations of Lyme borreliosis in Europe. Scand. J. Infect. Dis. Suppl. 77, 55–63.
Higgins, S., Jessup, R., Kurouski, D. (2022). Raman spectroscopy enables highly accurate differentiation between young male and female hemp plants. Planta 255, 85. doi: 10.1007/s00425-022-03865-8
Hilton, E., Devoti, J., Benach, J. L., Halluska, M. L., White, D. J., Paxton, H., et al. (1999). Seroprevalence and seroconversion for tick-borne diseases in a high-risk population in the northeast United States. Am. J. Med. 106, 404–409. doi: 10.1016/S0002-9343(99)00046-7
Horn, E. J., Dempsey, G., Schotthoefer, A. M., Prisco, U. L., Mcardle, M., Gervasi, S. S., et al. (2020). The Lyme disease biobank: Characterization of 550 patient and control samples from the East coast and upper Midwest of the united states. J. Clin. Microbiol. 58(6):e00032. doi: 10.1128/JCM.00032-20
Jahn, I. J., Grjasnow, A., John, H., Weber, K., Popp, J., Hauswald, W. (2021). Noise sources and requirements for confocal raman spectrometers in biosensor applications. Sensors 21. doi: 10.3390/s21155067
Khan, S., Ullah, R., Saleem, M., Bilal, M., Rashid, R., Khan, I., et al. (2016). Raman spectroscopic analysis of dengue virus infection in human blood sera. Optik 127, 2086–2088. doi: 10.1016/j.ijleo.2015.11.060
Krimmer, M., Farber, C., Kurouski, D. (2019). Rapid and noninvasive typing and assessment of nutrient content of maize kernels using a handheld raman spectrometer. ACS Omega 4, 16330–16335. doi: 10.1021/acsomega.9b01661
Lager, M., Dessau, R. B., Wilhelmsson, P., Nyman, D., Jensen, G. F., Matussek, A., et al. (2019). Serological diagnostics of Lyme borreliosis: Comparison of assays in twelve clinical laboratories in northern Europe. Eur. J. Clin. Microbiol. Infect. Dis. 38, 1933–1945. doi: 10.1007/s10096-019-03631-x
Lantos, P. M., Rumbaugh, J., Bockenstedt, L. K., Falck-Ytter, Y. T., Aguero-Rosenfeld, M. E., Auwaerter, P. G., et al. (2021). Clinical practice guidelines by the infectious diseases society of America (IDSA), American academy of neurology (AAN), and American college of rheumatology (ACR): 2020 guidelines for the prevention, diagnosis and treatment of Lyme disease. Clin. Infect. Dis. 72, e1–e48. doi: 10.1093/cid/ciab049
Lemler, P., Premasiri, W. R., Delmonaco, A., Ziegler, L. D. (2014). NIR raman spectra of whole human blood: Effects of laser-induced and in vitro hemoglobin denaturation. Anal. Bioanal Chem. 406, 193–200. doi: 10.1007/s00216-013-7427-7
Leon Bejarano, F., Ramirez-Elias, M. G., Mendez, M., Dorantes Méndez, G., Rodríguez-Aranda, M., Alba, A. (2017). Denoising of raman spectroscopy for biological samples based on empirical mode decomposition. Int. J. Modern Phys. C 28.
Li, X., Mchugh, G. A., Damle, N., Sikand, V. K., Glickstein, L., Steere, A. C. (2011). Burden and viability of Borrelia burgdorferi in skin and joints of patients with erythema migrans or Lyme arthritis. Arthritis Rheum. 63, 2238–2247. doi: 10.1002/art.30384
Lin, J., Chen, R., Feng, S., Pan, J., Li, B., Chen, G., et al. (2012). Surface-enhanced raman scattering spectroscopy for potential noninvasive nasopharyngeal cancer detection. J. Raman Spectrosc. 43, 497–502. doi: 10.1002/jrs.3072
Liveris, D., Varde, S., Iyer, R., Koenig, S., Bittker, S., Cooper, D., et al. (1999). Genetic diversity of Borrelia burgdorferi in Lyme disease patients as determined by culture versus direct PCR with clinical specimens. J. Clin. Microbiol. 37, 565–569. doi: 10.1128/JCM.37.3.565-569.1999
Liveris, D., Wormser, G. P., Nowakowski, J., Nadelman, R., Bittker, S., Cooper, D., et al. (1996). Molecular typing of Borrelia burgdorferi from Lyme disease patients by PCR-restriction fragment length polymorphism analysis. J. Clin. Microbiol. 34, 1306–1309. doi: 10.1128/jcm.34.5.1306-1309.1996
Lopez-Reyes, G., Rull Pérez, F. (2017). A method for the automated raman spectra acquisition. J. Raman Spectrosc. 48, 1654–1664. doi: 10.1002/jrs.5185
Margos, G., Fingerle, V., Oskam, C., Stevenson, B., Gofton, A. (2020). Comment on: Gupta 2019, distinction between Borrelia and Borreliella is more robustly supported by molecular and phenotypic characteristics than all other neighbouring prokaryotic genera: Response to margos’ et al. “The genus Borrelia reloaded” (PLoS one 13(12): e0208432). Tick Tick Borne Dis. 11, 101320. doi: 10.1016/j.ttbdis.2019.101320
Margos, G., Gofton, A., Wibberg, D., Dangel, A., Marosevic, D., Loh, S. M., et al. (2018). The genus Borrelia reloaded. PloS One 13, e0208432. doi: 10.1371/journal.pone.0208432
Margos, G., Marosevic, D., Cutler, S., Derdakova, M., Diuk-Wasser, M., Emler, S., et al. (2017a). Corrigendum: There is inadequate evidence to support the division of the genus Borrelia. Int. J. Syst. Evol. Microbiol. 67, 2073. doi: 10.1099/ijsem.0.002100
Margos, G., Marosevic, D., Cutler, S., Derdakova, M., Diuk-Wasser, M., Emler, S., et al. (2017b). There is inadequate evidence to support the division of the genus Borrelia. Int. J. Syst. Evol. Microbiol. 67, 1081–1084. doi: 10.1099/ijsem.0.001717
Molins, C. R., Ashton, L. V., Wormser, G. P., Hess, A. M., Delorey, M. J., Mahapatra, S., et al. (2015). Development of a metabolic biosignature for detection of early Lyme disease. Clin. Infect. Dis. 60, 1767–1775. doi: 10.1093/cid/civ185
Molins, C. R., Sexton, C., Young, J. W., Ashton, L. V., Pappert, R., Beard, C. B., et al. (2014). Collection and characterization of samples for establishment of a serum repository for Lyme disease diagnostic test development and evaluation. J. Clin. Microbiol. 52, 3755–3762. doi: 10.1128/JCM.01409-14
Moore, A., Nelson, C., Molins, C., Mead, P., Schriefer, M. (2016). Current guidelines, common clinical pitfalls, and future directions for laboratory diagnosis of Lyme disease, united states. Emerg. Infect. Dis. 22, 1169–1177. doi: 10.3201/eid2207.151694
Nigrovic, L. E., Lewander, D. P., Balamuth, F., Neville, D. N., Levas, M. N., Bennett, J. E., et al. (2019). The Lyme disease polymerase chain reaction test has low sensitivity. Vector Borne Zoonotic Dis. 20, 310–313. doi: 10.1089/vbz.2019.2547
O’dwyer, K., Domijan, K., Dignam, A., Butler, M., Hennelly, B. M. (2021). Automated raman micro-spectroscopy of epithelial cell nuclei for high-throughput classification. Cancers 13. doi: 10.3390/cancers13194767
Ohnishi, J., Piesman, J., De Silva, A. M. (2001). Antigenic and genetic heterogeneity of Borrelia burgdorferi populations transmitted by ticks. Proc. Natl. Acad. Sci. U.S.A. 98, 670–675. doi: 10.1073/pnas.98.2.670
Pachner, A. R., Delaney, E., O’neill, T. (1995). Neuroborreliosis in the nonhuman primate: Borrelia burgdorferi persists in the central nervous system. Ann. Neurol. 38, 667–669. doi: 10.1002/ana.410380417
Pachner, A. R., Steere, A. C. (1985). The triad of neurologic manifestations of Lyme disease. Neurology 35, 47. doi: 10.1212/WNL.35.1.47
Pegalajar-Jurado, A., Schriefer, M. E., Welch, R. J., Couturier, M. R., Mackenzie, T., Clark, R. J., et al. (2018). Evaluation of modified two-tiered testing algorithms for Lyme disease laboratory diagnosis using well-characterized serum samples. J. Clin. Microbiol. 56, e01943–e01917. doi: 10.1128/JCM.01943-17
Pichardo-Molina, J. L., Frausto-Reyes, C., Barbosa-García, O., Huerta-Franco, R., González-Trujillo, J. L., Ramírez-Alvarado, C. A., et al. (2007). Raman spectroscopy and multivariate analysis of serum samples from breast cancer patients. Lasers Med. Sci. 22, 229–236. doi: 10.1007/s10103-006-0432-8
Rand, P. W., Lubelczyk, C., Lavigne, G. R., Elias, S., Holman, M. S., Lacombe, E. H., et al. (2003). Deer density and the abundance of Ixodes scapularis (Acari: Ixodidae). J. Med. Entomol. 40, 179–184. doi: 10.1603/0022-2585-40.2.179
Rogovskyy, A. S., Gillis David, C., Ionov, Y., Gerasimov, E., Zelikovsky, A. (2016). Antibody response to Lyme disease spirochetes in the context of VlsE-mediated immune evasion. Infect. Immun. 85, e00890–e00816.
Schwan, T. G., Piesman, J., Golde, W. T., Dolan, M. C., Rosa, P. A. (1995). Induction of an outer surface protein on Borrelia burgdorferi during tick feeding. Proc. Natl. Acad. Sci. U.S.A. 92, 2909–2913. doi: 10.1073/pnas.92.7.2909
Senger, R. S., Sayed Issa, A., Agnor, B., Talty, J., Hollis, A., Robertson, J. L. (2022). Disease-associated multimolecular signature in the urine of patients with Lyme disease detected using raman spectroscopy and chemometrics. Appl. Spectrosc. 76, 284–299. doi: 10.1177/00037028211061769
Shor, S., Green, C., Szantyr, B., Phillips, S., Liegner, K., Burrascano, J. J., Jr., et al. (2019). Chronic Lyme disease: an evidence-based definition by the ILADS working group. Antibiot. (Basel Switzerland) 8, 269. doi: 10.3390/antibiotics8040269
Steere, A. C., Malawista, S. E., Snydman, D. R., Shope, R. E., Andiman, W. A., Ross, M. R., et al. (1977). An epidemic of oligoarticular arthritis in children and adults in three Connecticut communities. Arthritis Rheum. 20, 7–17. doi: 10.1002/art.1780200102
Steere, A. C., Schoen, R. T., Taylor, E. (1987). The clinical evolution of Lyme arthritis. Ann. Intern. Med. 107, 725–731. doi: 10.7326/0003-4819-107-5-725
Steere, A. C., Strle, F., Wormser, G. P., Hu, L. T., Branda, J. A., Hovius, J. W. R., et al. (2016). Lyme Borreliosis. Nat. Rev. Dis. Primers 2, 16090–16090. doi: 10.1038/nrdp.2016.90
Stone, B. L., Tourand, Y., Brissette, C. A. (2017). Brave new worlds: the expanding universe of Lyme disease. Vector Borne Zoonotic Dis. 17, 619–629. doi: 10.1089/vbz.2017.2127
Tabb, J. S., Rapoport, E., Han, I., Lombardi, J., Green, O. (2022). An antigen-targeting assay for Lyme disease: combining aptamers and SERS to detect the OspA protein. Nanomedicine 41, 102528. doi: 10.1016/j.nano.2022.102528
Tuschel, D. (2016). Selecting an excitation wavelength for raman spectroscopy. Spectroscopy 31, 14–23.
Van Dam, A. P., Kuiper, H., Vos, K., Widjojokusumo, A., De Jongh, B. M., Spanjaard, L., et al. (1993). Different genospecies of Borrelia burgdorferi are associated with distinct clinical manifestations of Lyme borreliosis. Clin. Infect. Dis. 17, 708–717. doi: 10.1093/clinids/17.4.708
Waddell, L. A., Greig, J., Mascarenhas, M., Harding, S., Lindsay, R., Ogden, N. (2016). The accuracy of diagnostic tests for Lyme disease in humans, a systematic review and meta-analysis of north American research. PloS One 11, e0168613. doi: 10.1371/journal.pone.0168613
Wormser, G. P., Dattwyler, R. J., Shapiro, E. D., Halperin, J. J., Steere, A. C., Klempner, M. S., et al. (2006). The clinical assessment, treatment, and prevention of Lyme disease, human granulocytic anaplasmosis, and babesiosis: clinical practice guidelines by the infectious diseases society of America. Clin. Infect. Dis. 43, 1089–1134. doi: 10.1086/508667
Keywords: Raman spectroscopy, Lyme borreliosis, Borrelia, PLS-DA, diagnostics, test
Citation: Goff NK, Dou T, Higgins S, Horn EJ, Morey R, McClellan K, Kurouski D and Rogovskyy AS (2022) Testing Raman spectroscopy as a diagnostic approach for Lyme disease patients. Front. Cell. Infect. Microbiol. 12:1006134. doi: 10.3389/fcimb.2022.1006134
Received: 29 July 2022; Accepted: 05 September 2022;
Published: 27 October 2022.
Edited by:
Shahid Karim, University of Southern Mississippi, United StatesReviewed by:
Jon Blevins, University of Arkansas for Medical Sciences, United StatesDeepak Kumar, University of Southern Mississippi, United States
Khemraj Budachetri, Eurofins BPT (Biopharma Product testing), United States
Copyright © 2022 Goff, Dou, Higgins, Horn, Morey, McClellan, Kurouski and Rogovskyy. This is an open-access article distributed under the terms of the Creative Commons Attribution License (CC BY). The use, distribution or reproduction in other forums is permitted, provided the original author(s) and the copyright owner(s) are credited and that the original publication in this journal is cited, in accordance with accepted academic practice. No use, distribution or reproduction is permitted which does not comply with these terms.
*Correspondence: Dmitry Kurouski, ZGt1cm91c2tpQHRhbXUuZWR1; Artem S. Rogovskyy, YXJvZ292c2t5eUB0YW11LmVkdQ==