- 1Qingdao Eye Hospital of Shandong First Medical University, Qingdao, China
- 2State Key Laboratory Cultivation Base, Shandong Provincial Key Laboratory of Ophthalmology, Shandong Eye Institute, Shandong First Medical University & Shandong Academy of Medical Sciences, Qingdao, China
- 3Qingdao University Medical College, Qingdao, China
- 4Department of Medical Oncology, The Affiliated Hospital of Qingdao University, Qingdao, China
The ocular surface possesses its own bacterial microbiota. Once given a chance, opportunistic pathogens within ocular microbiota may lead to corneal infection like bacterial keratitis (BK). To reveal the possible factor that makes people vulnerable to BK from the perspective of ocular bacterial microbiota, as well as to compare diagnostic information provided by high-throughput 16S rDNA sequencing and bacterial culture, 20 patients with BK and 42 healthy volunteers were included. Conjunctival swabs and corneal scrapings collected from the diseased eyes of BK patients were subjected for both high-throughput 16S rDNA sequencing and bacterial culture. Conjunctival swabs collected from the normal eyes of BK patients and healthy volunteers were sent only for sequencing. For identifying the pathogens causing BK, high-throughput 16S rDNA sequencing presented a higher positive rate than bacterial culture (98.04% vs. 17.50%), with 92.11% reaching the genus level (including 10.53% down to the species level). However, none of the sequencing results was consistent with the cultural results. The sequencing technique appears to challenge culture, and could be a complement for pathogen identification. Moreover, compared to the eyes of healthy subjects, the ocular microbiota of three sample groups from BK patients contained significantly less Actinobacteria and Corynebacteria (determinate beneficial symbiotic bacteria), but significantly more Gammaproteobacteria, Pseudomonas, Bacteroides, and Escherichia-Shigella (common ocular pathogenic bacteria). Therefore, it is speculated that the imbalance of protective and aggressive bacteria in the ocular microbiota of healthy people may trigger susceptibility to BK. Based on this speculation, it seems promising to prevent and treat infectious oculopathy through regulating ocular microbiota.
Introduction
The ocular surface possesses its own bacterial microbiota, which contains both probiotics and opportunistic organisms (Huang et al., 2016; St Leger et al., 2017). Once given a chance, such as ocular trauma, opportunistic pathogens within ocular microbiota may lead to devastating corneal infection like bacterial keratitis (BK) (Chidambaram et al., 2018). BK accounts for over 90% of microbial keratitis in temperate regions (Ong and Corbett, 2015). Under a conservative estimate, there are 1.5–2.0 million cases of monocular blindness annually associated with this disease (Shah et al., 2011; Ong and Corbett, 2015; Bograd et al., 2019)
Bacterial culture is considered as the gold standard for etiological diagnosis of BK (Sharma, 2012; Ren et al., 2020). However, this technique is becoming limited in the current diagnostic application for its frequent unsatisfying positive rates (Ren et al., 2020). Culture lacks specificity with its positivity for the healthy ocular surface being as high as 42% to 80% (Willcox, 2013; Huang et al., 2016; Bograd et al., 2019). Sometimes the culture results of specimens from BK patients do not concur with the microscopic examination results of stained smears (Eguchi et al., 2017). Thus, the positive results from bacterial culture may be attributed to certain symbiotic bacteria on the ocular surface that are easy to grow in the culture medium, rather than the exactly real pathogens. A therapeutic schedule of BK usually depends on the antibiotic susceptibility and pathogenicity of pathogenic bacteria, which requires accurate identification of specific pathogens (Bourcier et al., 2003).
The Human Microbiome Project was initiated by the National Institutes of Health in 2007 to identify the microorganisms that reside normally on the healthy human body and to ultimately characterize changes associated with disease states (Integrative HMPRNC, 2019). The investigate interactions between the gut microbiota and ocular pathology and their implications for progression of disease, namely “gut-eye” axis, has been focusing on for a long time (Kugadas et al., 2017; Floyd and Grant, 2020). However, the breakthrough related to the reciprocity between ocular microbiota and eye were not available until St. Leger et al. isolated Corynebacterium mastitidis, a type of ocular probiotics, on bacterial culture for the first time in 2017 (St Leger et al., 2017). Thereupon, for healthy people, whether ocular microbiota generally maintains a dynamic equilibrium status and whether dysbiosis of ocular microbiota can facilitate oculopathy become necessary to be explored, especially through new technologies and approaches.
Since the 16S rDNA gene not only has highly conserved structure and function but also can reflect the differences among different bacterial genera, it is generally used as a unique barcode for bacterial identification (Wang et al., 2007). High-throughput 16S rDNA sequencing has opened a new avenue for thorough comprehension of the human bacterial microbiome, for it can exhibit the relative content of almost all bacterial genera in a sample. In this study, from the perspective of ocular bacterial microbiota, the diagnostic information within the ocular bacterial microbiome for identifying causative bacteria of BK was disclosed using this sequencing technique, and the susceptibility factor of BK was revealed.
Materials and Methods
Ethics Approval
This research was approved by the Ethics Committee of Qingdao Eye Hospital and registered on Chinese Clinical Trial Registry (ChiCTR 1900023651). All procedures complied with the tenets of the Declaration of Helsinki. Informed consent was obtained from all participants.
Inclusion Criteria
Subject recruitment lasted for one whole year to eliminate seasonal effects. Twenty patients, 10 males and 10 females, who were diagnosed as BK following the Bacterial Keratitis Preferred Practice Pattern from the American Academy of Ophthalmology (Lin et al., 2019) were included, with an average age of 50 years (range, 24 to 80 years). Patients with any recent diagnosis and treatment in other eye care institutions, recent usage of eyedrops by themselves, concurrent eye diseases, and any previous oculopathy or ophthalmic/corneal refractive surgery were excluded. Based on the stratified sampling, 42 healthy volunteers with a similar gender and age composition were enrolled as controls.
Sample Collection
In a disinfected operating room utilizing a laminar flow system (conforming to GB50333-2013-I standard of China), all participants lay supine for collecting corneal scrapings and/or conjunctival samples. Eye lids were wiped using Iodophor, and the remaining facial area was covered with a sterile surgical drape. The palpebral, bulbar, and fornical conjunctiva of the affected and normal eyes of each patient as well as the randomly chosen eye of each healthy control was rubbed lightly using a sterile swab soaked with oxybuprocaine hydrochloride eye drops for preparation of conjunctival samples. Then, after a drop of oxybuprocaine hydrochloride eye drops was dripped to the diseased eyes, the corneal lesions were scraped using an ophthalmic microsurgical knife (Cat. No. MR-G137A, Suzhou Mingren Medical Equipment Co., Suzhou, China) under a microscope.
To avoid potential contamination, after intraday sampling, three sterile swabs exposed to laminar flow for 3 minutes and all remaining oxybuprocaine hydrochloride eye drops were sent for DNA extraction and high-throughput 16S rDNA sequencing. Intraday samples from subjects were kept in reserve so long as aforementioned environmental samples generated negative results.
Bacterial Culture
Samples were streaked on blood agar medium (Cat. No. 16, Autobio, Zhengzhou, China) at 37°C using the quad plate streaking method, with daily observation. Once macroscopic colonies appeared in the medium, they were stained with Gram staining and verified according to morphology under a microscope by two experienced technicians. Then the colonies were sent into MicroScan WalkAway 96Plus (Siemens, Germany) to identify the genus or species. If no colony formed within 14 days, the culture was reported to be negative.
High-Throughput 16S rDNA Sequencing
DNA Extraction
Total genomic DNA was extracted from samples using the DNA Extraction Kit (Cat. No. D3096-100T, Omega Bio-tek, Norcross, GA, USA), after which all DNA samples were delivered to OE Biotech (Qingdao, China) for high-throughput 16S rDNA sequencing. All the sequencing and analytic process was subjected to the standard operating procedure of OE Biotech. More experimental details about sequencing are available at https://www.qdoebiotech.com.
DNA Amplification
After the quantity and quality of DNA were assessed by NanoDrop and agarose gel, samples were loaded into 0.6% agarose gel and subjected to 120V constant voltage electrophoresis for 15 minutes. A total of 50 ng DNA without degradation or with slight degradation were used for PCR amplification (Cat. No. 580BR10905, Bio-rad, Hercules, CA, USA) after being diluted to 1 ng/μl as template, with primers and Takara Ex Taq (Cat. No. RR001Q, Takara, Shiga, Japan), following instructions. V3-V4 variable regions of 16S rRNA were amplified with universal primers 343F (5’- TACGGRAGGCAGCAG -3’) and 798R (5’- AGGGTATCTAATCCT-3’).
Library Construction
Amplicon quality was evaluated using gel electrophoresis, refined with AMPure XP beads (Agencourt), and enlarged for another round of PCR using primers 343F and 798R. After purification with AMPure XP beads again, the amplicon was quantified using Qubit dsDNA assay kit (Cat. No. Q32852, Life Technologies, Carlsbad, CA, USA) to become the final amplicon. Equal amounts of refined amplicon were pooled for subsequent high-throughput sequencing (Illumina miseq pe300).
Bioinformatic Analysis
Raw sequencing data were stored as FASTQ format. Paired-end reads were operated using Trimmomatic software (version 0.53) to discover and cut out ambiguous bases (N). Trimmomatic software also cut off inferior quality sequences with an average quality score below 20 through the sliding window trimming approach. Paired-end reads were assembled using FLASH software (version 1.2.11) after trimming. Parameters of the assembly were set as 10 bp of minimal overlapping, 20% of maximum mismatch rate, and 200 bp of maximum overlapping. Moreover, sequences were further denoised by quitting reads with ambiguous, homologous sequences or those below 200 bp. Reads with 75% of bases beyond Q20 were reserved, while those having chimera were removed. The two processes were performed using QIIME software (version 1.8.0). Clean reads were further subjected to primer sequences removal and clustering to form operational taxonomic units (OTUs) with 97% similarity cut out using Vsearch software (version 2.4.2). All representative reads of every OTU were chosen using QIIME package. All representative reads were annotated and blasted against Silva database (version 123) and Greengens database using RDP classifier (confidence threshold was 70%).
Using the statistical software R (corrplot package), a predicted interaction network of top 30 bacterial genera was established based on Spearman correlation coefficient. The bacterial genera with |SpearmanCoef| > 0.8 and p < 0.01 were highlighted.
Parameters of Microbial Community
Alpha diversities were used to delineate within-community characteristics. Good’s coverage index reflected the depth of sequencing. The closer the index was to 1, it meant that the depth of sequencing had basically covered all species in the sample. Observed species parametrized the actual number of observed OTUs. Chao1 index estimated how many kinds of OTUs actually presented in the community (Hill et al., 2003). Both Shannon Wiener index and Simpson’s index of diversity (1-D) are estimators of species richness and evenness, but the former was more sensitive to species richness, whereas the latter was more sensitive to evenness (Hill et al., 2003; Kim et al., 2017). The larger the value of phylogenetic diversity index represented the microbial community was constituted by species that had farther relationship of evolution between each other (Faith and Baker, 2007). Moreover, beta diversities were used to reveal between-community characteristics in order to compare the differences among the diseased eyes and normal eyes of BK patients and the eyes of healthy volunteers.
Results
Overview of the Study
With high-throughput 16S rDNA sequencing, 98.04% of specimens (100/102) presented positive results, including 19 conjunctival swabs (95%, 19/20) and 19 corneal scrapings (95%, 19/20) from the diseased eyes, 20 conjunctival swabs (100%, 20/20) from the healthy eyes of BK patients, and 42 conjunctival swabs (100%, 42/42) from the eyes of healthy volunteers. The amount of OTUs in each sample ranged from 10 to 3666. The content and the representative sequence of each OTU in each sample are shown in Supplementary Data 1, 2. The relative bacterial compositions of top 30 families and genera for each sample are presented in Figure 1.
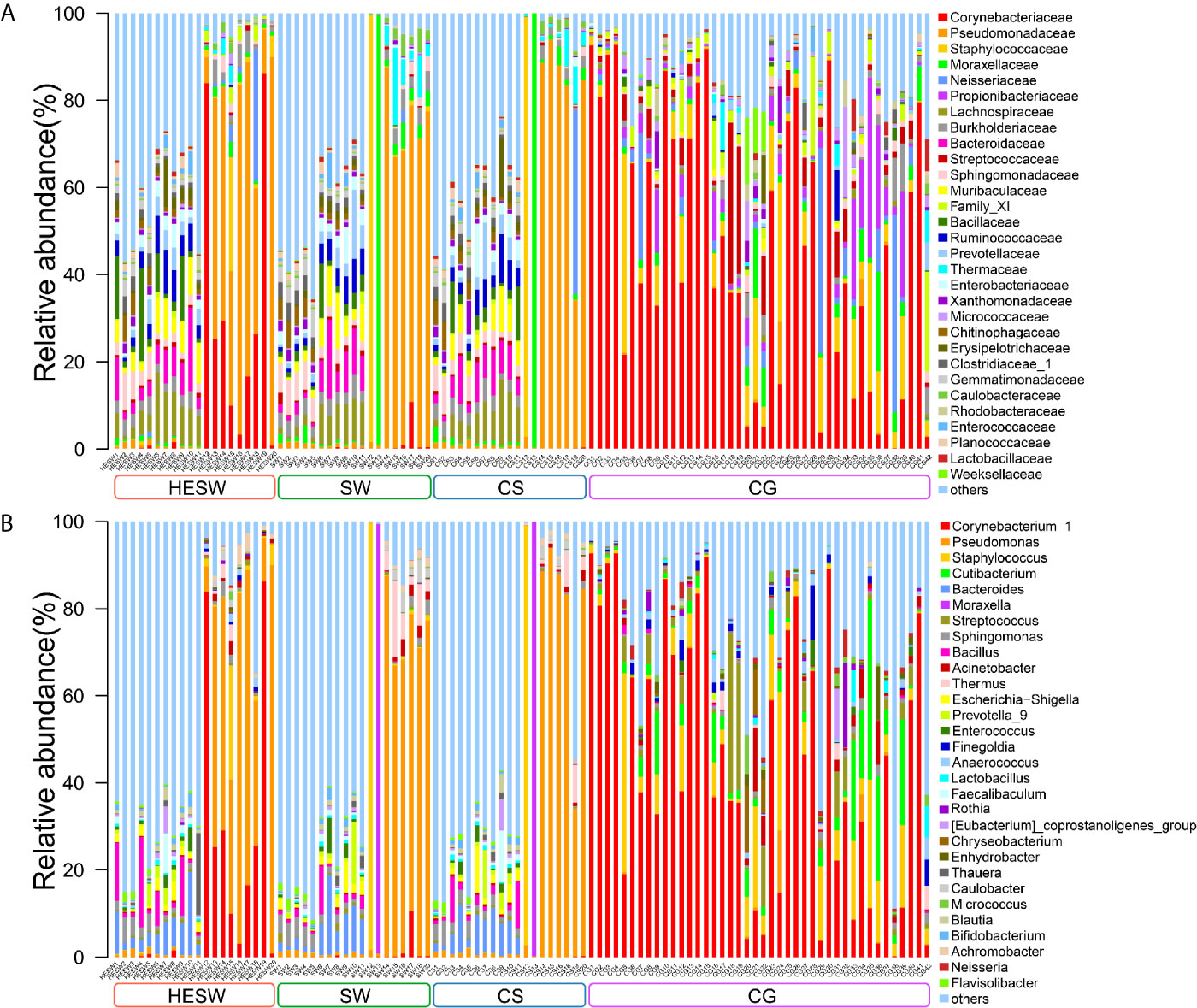
Figure 1 The relative composition of ocular microbiota. Each bar represents a sample of conjunctival microbiota, various color portions represent different genera, and the length of a colored portion represents the relative content of a family (A) or genus (B). Among the 102 samples, 100 positive samples were obtained, including 20 conjunctival swabs from the healthy eyes of patients with bacterial keratitis (BK) (group HESW), 19 conjunctival swabs from the diseased eyes of BK patients (group SW), 19 corneal scrapings from the diseased eyes of BK patients (group CS), and 42 conjunctival swabs from the healthy volunteers (group CG). Different samples possess diverse ocular surface microbiotas.
On the other hand, the results of bacterial culture were positive in 17.5% of samples (7/40) from the diseased eyes, including 2 conjunctival swabs and 5 corneal scrapings. In total, 4 bacterial genera were found (Table 1).
Identification of the Pathogenic Bacteria
All high-throughput 16S rDNA sequencing reports disclosed 3 results at the family level, 31 results at the genus level, and 4 results at the species level. Meanwhile, bacterial culture exposed 1 result at the genus level and 6 results at the species level. However, none of the sequencing results was consistent with the culture results (Table 1). The positive rate of high-throughput 16S rDNA sequencing was higher than bacterial culture (98.04% vs. 17.5%), but the sequencing presented a lower percentage of results at the genus level (92.11% vs. 100%).
Using high-throughput 16S rDNA sequencing, as shown in Table 1, 18 patients obtained positive results from both conjunctival swabs and corneal scrapings of the diseased eyes. The 18 pairs of results can be analyzed according to two hypotheses. If the genus with the highest relative content was hypothesized as the result for identifying pathogens, the sequencing results from conjunctival swabs and corneal scrapings were consistent in eight of 18 patients (44.44%). If the genus with the highest relative content was deemed the causative bacterium as long as it occupied more than 50% of the content and other conditions were deemed multiple bacterial co-infections, all results (100%, 18/18) from conjunctival swabs and corneal scrapings were consistent. Thus, in the latter circumstance, conjunctival swabs seemed to be an alternative noninvasive approach to etiological examination of BK.
Community Structural Alterations
Due to the similar environmental stress, the right and left eyes have no difference in the structure of microbiota (Cavuoto et al., 2018). If an infection in one eye would not lead to an alteration of the bacterial community of the other eye, the microbiota of the healthy eyes of BK patients exactly reflected the status before BK occurred. Based on this hypothesis, the comparison between the conjunctival swabs from the healthy eyes of BK patients and the normal volunteers could reveal the susceptibility factor of BK from the perspective of ocular microbiota.
With respect to alpha diversities (Figure 2), the healthy eyes of BK patients were significantly different from the healthy eyes of controls in Good’s coverage index (P=0.00145), observed species (P=0.044), Chao1 index (P=0.0276), Shannon Wiener index (P=0.039), Simpson’s index (P=0.025), and phylogenetic diversity index (P=0.012). All were of pairwise comparisons using Wilcoxon rank sum test. For beta diversity (Figure 3), the PCoA plots (Weighted Unifrac algorithm, P=0.001, R2 = 1, F. Model=13.823, total SumsOfSqs=12.1678, and total Df=99) showed significant difference between the healthy eyes from the patients and the controls. On the whole, the sort and richness of ocular bacterial microbiota of the healthy eyes of BK patients had significant difference from the eyes of healthy people.
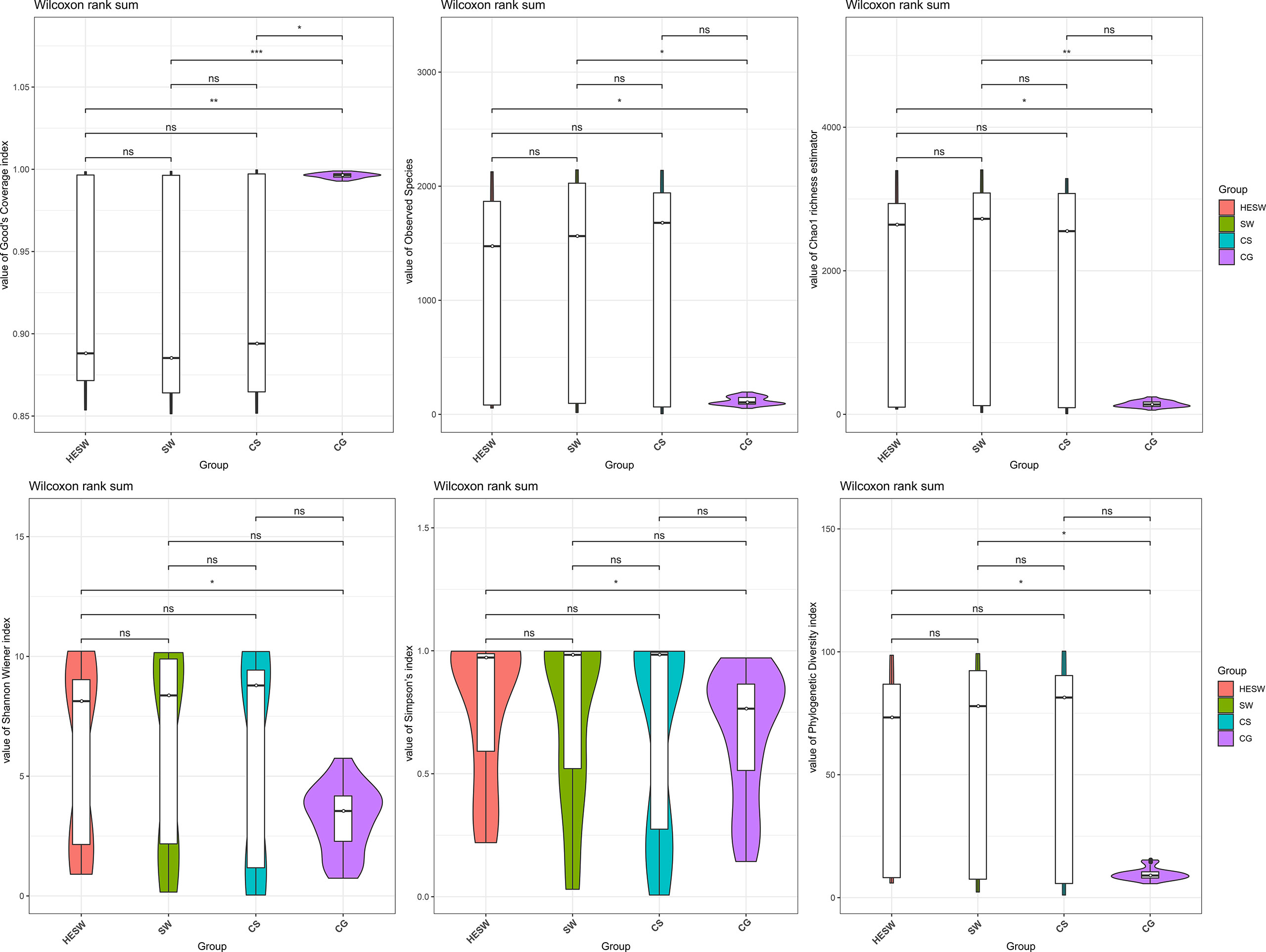
Figure 2 On the dimensionality of alpha diversities, six indices all suggest the sort and richness of ocular bacterial microbiota from the healthy eyes of BK patients had significant difference from the eyes of healthy people. Alpha diversity indices in this study includes Good’s coverage index, Observed species, Chao1 index, Shannon Wiener index, Simpson’s index, and phylogenetic diversity index. (HESW, conjunctival swabs from the healthy eyes of BK patients; SW, conjunctival swabs from the diseased eyes of BK patients; CS, corneal scrapings from the diseased eyes of BK patients; CG, conjunctival swabs from healthy volunteers; ns, no significant difference; *P < 0.05; **P < 0.01; ***P < 0.001).
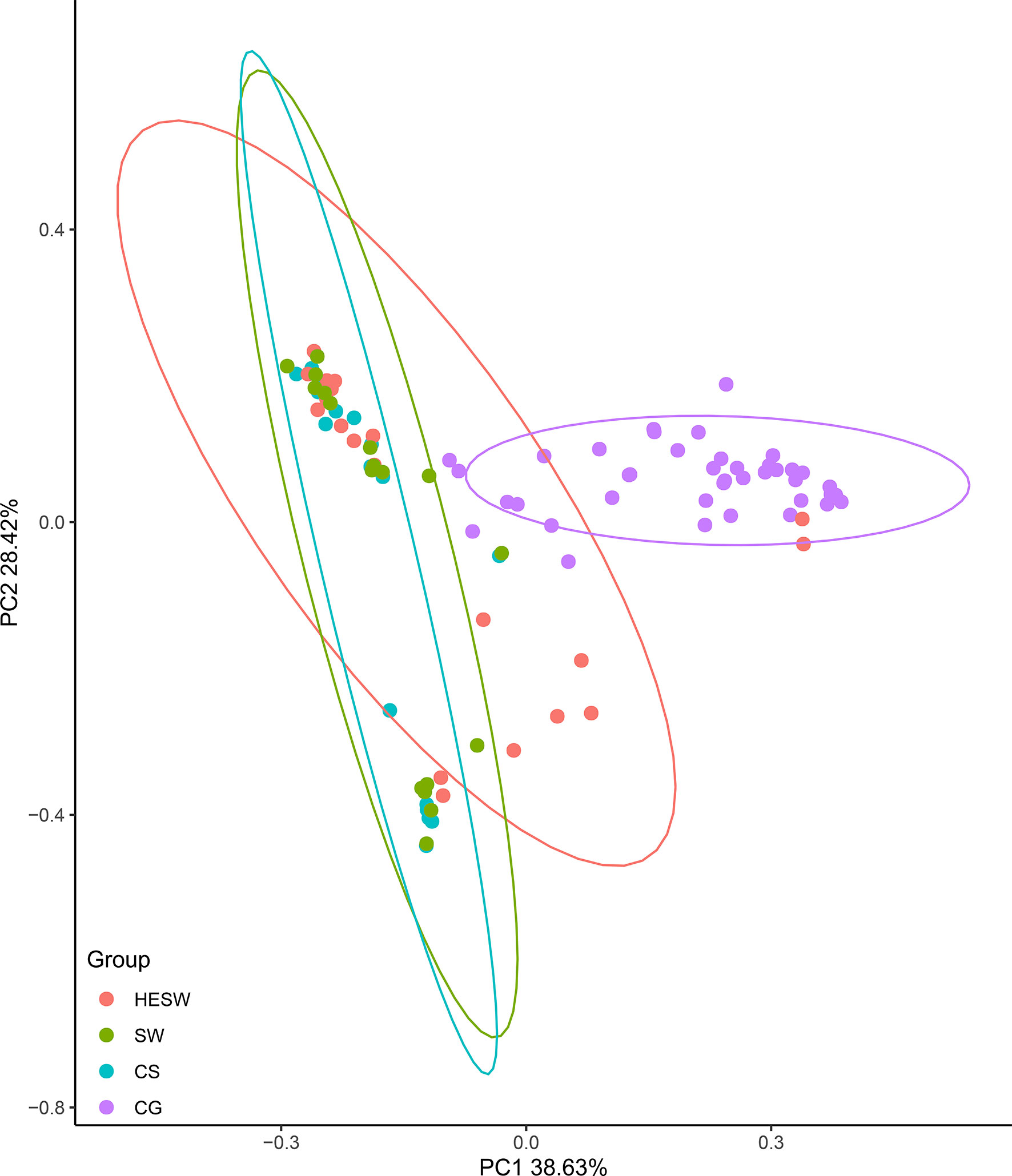
Figure 3 On the dimensionality of beta diversities, PCoA plots suggest the sort and richness of ocular bacterial microbiota from the healthy eyes of BK patients had significant difference from the eyes of healthy people. Two-dimensional PCoA plots with confidence ellipse (Weighted Unifrac algorithm) show the beta diversities of each sample. The abscissa (PC1) and the ordinate (PC2) are the two principal co-ordinates which have the largest interpretation of the difference between samples. The percentage on abscissa (or ordinate) represents the explanatory degree to the difference among samples. The CG group had significant difference from the HESW group (HESW, conjunctival swabs from the healthy eyes of BK patients; SW, conjunctival swabs from the diseased eyes of BK patients; CS, corneal scrapings from the diseased eyes of BK patients; CG, conjunctival swabs from the healthy volunteers; BK, bacterial keratitis).
Figure 4 shows the top 10 taxa with significant difference (p values were shown in Table S1) among four groups at the levels of class, order, family, and genus. Compared to the healthy subjects, the ocular microbiota of the healthy eyes of BK patients possessed less Actinobacteria, but more Gammaproteobacteria, Bacteroidia, Clostridia, Alphaproteobacteria, Deltaproteobacteria, Gemmatimonadetes, Thermoleophilia, Acidimicrobiia, and Mollicutes at the class level; less Corynebacteriales, Lactobacillales, Propionibacteriales, and Micrococcales, but more Pseudomonadales, Clostridiales, Bacteroidales, Sphingomonadales, Rhizobiales, and Chitinophagales at the order level; less Corynebacteriaceae, Propionibacteriaceae, Streptococcaceae, and Family XI, but more Pseudomonadaceae, Lachnospiraceae, Burkholderiaceae, Bacteroidaceae, Sphingomonadaceae, and Muribaculaceae at the family level; less Corynebacterium, Cutibacterium, Streptococcus, Finegoldia, and Anaerococcus, but more Pseudomonas, Bacteroides, Sphingomonas, Bacillus, and Escherichia-Shigella at the genus level.
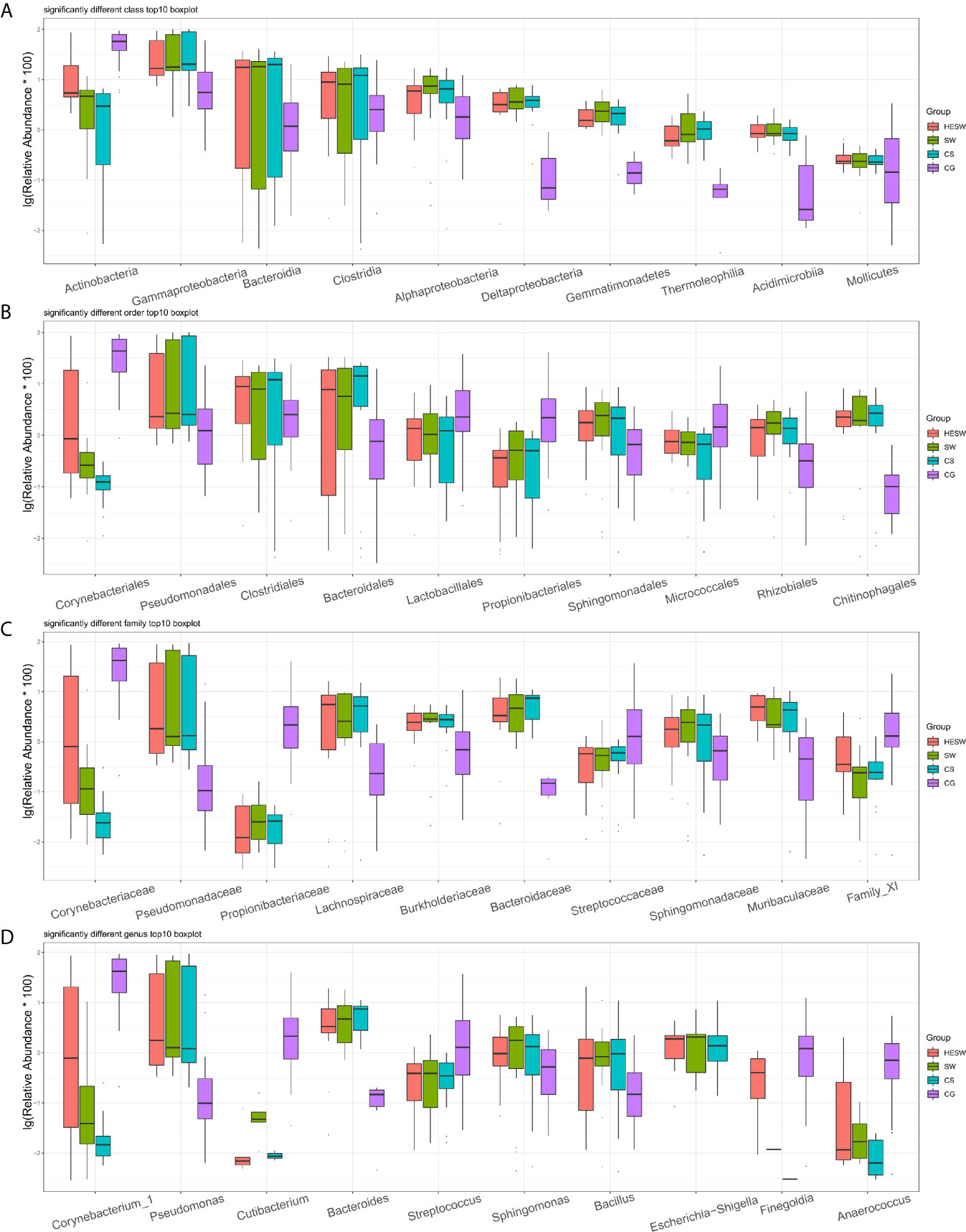
Figure 4 Top 10 taxa with significant difference at the levels of class, order, family, and genus. (A) At the class level, Actinobacteria significantly decreased and Gammaproteobacteria significantly increased in BK patients’ ocular microbiota. (B–D) At the levels of order, family, and genus, Corynebacter significantly decreased, while Pseudomonas, Bacteroides, and Escherichia-Shigella significantly increased in BK patients’ ocular microbiota (HESW, conjunctival swabs from the healthy eyes of BK patients; SW, conjunctival swabs from the diseased eyes of BK patients; CS, corneal scrapings from the diseased eyes of BK patients).
Based on PICRUSt (Langille et al., 2013), the ocular microbiota of three sample groups from BK patients were found to possess significantly different composition of bacterial gene functions, compared to the eyes of healthy subjects. Compared to the healthy subjects, the ocular microbiota of BK patients presented significantly more gene related to metabolism, cellular processes, human diseases, organismal systems, environmental information processing, and genetic information processing (Figure 5). Namely, the ocular microbiota of BK patients appeared more active and aggressive.
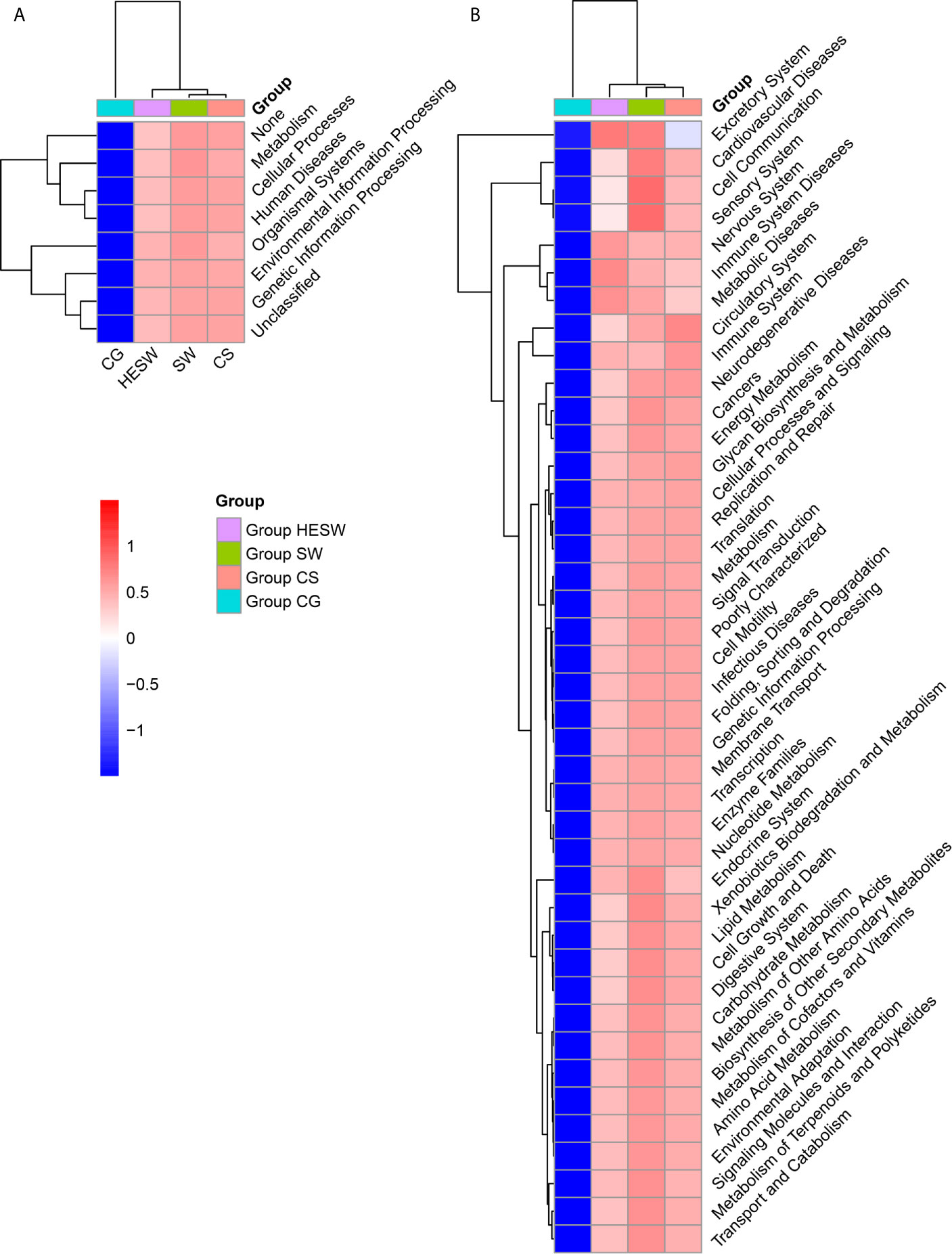
Figure 5 The ocular microbiota of BK patients appeared more active and aggressive. At L1 (A) and L2 (B) level, two-dimensional heat map showing rank normalized abundances (scaled between 0 and 1) of differentially abundant bacterial gene functions determined by Kruskal-Wallis test and KEGG. (HESW, conjunctival swabs from the healthy eyes of BK patients; SW, conjunctival swabs from the diseased eyes of BK patients; CS, corneal scrapings from the diseased eyes of BK patients).
A Predicted Interaction Network of Ocular Bacterial Microbiota at the Genus Level
Based on Spearman correlation coefficient (|SpearmanCoef|> 0.8 and P <0.01) of the top 50 bacterial genera, Figure S1 reveals a predicted higher-order interaction network at the genus level for ocular bacterial microbiota. This network was not verified in vitro, but it still may help to enlighten further investigations.
Discussion
High-Throughput 16S rDNA Sequencing Challenges Bacterial Culture in Identifying the Pathogenic Bacteria of BK
Despite the worldwide recognition of culture in identifying microorganisms responsible for corneal infection (Austin et al., 2017; Eguchi et al., 2017), it has been facing an increasing challenge. According to our previous studies, microbiota abounding on the ocular surface of both healthy people and patients with non-infective eye disease undermines the reliability of microbial culture (Huang et al., 2016; Dong et al., 2019; Ge et al., 2019; Wang et al., 2019; Ren et al., 2020). High-throughput internal transcribed spacer (the unique barcode for fungal identification) sequencing also presents advantages over fungal culture for fungal identification in fungal keratitis (Ren et al., 2020). The inconformity of results between culture and high-throughput 16S rDNA sequencing seems to be within expect, but it does not mean high-throughput sequencing technology could supersede microbial culture, for sequencing has deficiencies like PCR bias, insufficient coverage for microorganisms of public sequence databases, and incapability to distinguish resident and transient microorganisms, living microorganisms and simply DNA signatures (Nilsson et al., 2008; Shivaji et al., 2019). Furthermore, high-throughput 16S rDNA sequencing technology cannot test antibiotic susceptibility, which is important for treating BK. Methodologically, high-throughput 16S rDNA sequencing requires amplification step, however, culture have no need for amplification. Therefore, the amplification step also may account for the discrepancy in the numbers/types of revealed organisms. After weighing the pros and cons in this study, high-throughput 16S rDNA sequencing was considered to be an optional supplement for identification of bacterial pathogens in the diagnosis of BK.
It is hard to determine which result we should believe when divergent results are generated after sequencing and culture. In the current study, high-throughput 16S rDNA sequencing showed that the causative genus occupied more than 50% of ocular bacterial microbiota only when it was identified as Staphylococcus, Moraxella, and Pseudomonas, while in other situations it usually occupied less than 15% (scilicet multiple bacterial co-infections). This phenomenon was not in accordance with traditional cognition for infectious disease – a certain causative genus wildly grows and occupies absolute quantitative superiority. It seems that the causative genus of BK, along with its antibiotic resistance, should be comprehended anew.
One of the possible new understandings for BK may be similar to the revolution of comprehensions for bacterial vaginosis (BV). BV was first described to be caused by Haemophilus vaginalis or Gardnerella vaginalis (Ledger, 1993). Then it was considered to be related to a number of potential microbial pathogens, singly and in combinations (Onderdonk et al., 2016). Finally, BV was attributed to the reduction of Lactobacillus and the overgrowth of anaerobic bacteria (Redelinghuys et al., 2020). This eventuality reminds us of the probability of regulating one certain species or a category of species, instead of concentrating on the causative species, in the treatment of BK.
The Imbalance of Protective and Aggressive Bacteria in the Ocular Microbiota for Healthy People Triggers Susceptibility to BK
The ocular microbiota, which comprised both probiotic and pathogenic bacteria (Figure 1), and various host cells were disclosed to process a complicated interaction network in our study (Figure S1). The ocular surface microenvironment might be explained by the Yin-Yang balance, which is a basic theory in the traditional Chinese medicine, as well as a philosophical term. Therein Yin is on behalf of the inhibitory and repressive factors, whereas Yang represents the aggressive and active factors (Yan et al., 2020). Normally, Yin and Yang maintain a dynamic equilibrium status. However, Yin-Yang imbalances enable people to develop certain disease. Accordingly, in this study, Yin stood for probiotics such as Corynebacterium and Actinobacteria, for Corynebacterium mastiditis (St Leger et al., 2017) has been previously demonstrated to elicit IL-17 response from γδ T cells in the ocular mucosa to resist bacterial and fungal infections on the murine ocular surface, and Actinobacteria (Valliappan et al., 2014; Dalitz et al., 2017) has proved to synthesize a series of secondary metabolites, which have antimicrobial, anti-viral, antiparasitic, antioxidant, anticancer, and neurological activities. Yin also represented a bunch of nonpathogenic bacteria with undiscovered benefits. On the other hand, Yang stood for a series of pathogenic bacteria, such as Pseudomonas, Bacteroides, Streptococcus, and Escherichia-Shigella. The ocular microbiota of BK patients was found to be composed of fewer protective bacteria but more aggressive bacteria than that of healthy people. Namely, the Yin-Yang imbalance made the cornea vulnerable.
It is worth noting that Yang does not mean relevant elements are always detrimental in Chinese philosophy. It is also crucial for the complete wholeness of the dynamic equilibrium status. In addition, a Yang element in one certain field can be the Yin in other fields. For example, Staphylococcus epidermidis is not only an opportunistic pathogen on the skin but also can produce staphylococcal lipoteichoic acid through Toll-like receptor 3 to impact keratinocytes, eventually inhibiting inflammation after skin injury (Lai et al., 2009). Corynebacterium mastiditis is an important pathogen of mastitis, but it can protect the cornea against infection (St Leger et al., 2017)
Moreover, the same inducement can cause diverse types of infection. For instance, corneal trauma may lead to BK, fungal keratitis or Acanthamoeba keratitis (Mascarenhas et al., 2014). In addition, lack of the same beneficial bacterial genus can make people vulnerable to diverse diseases. For example, the murine corneas lacking Corynebacterium mastiditis were observed to be liable to Candida albicans and Pseudomonas aeruginosa infections (St Leger et al., 2017). Similarly, our previous study on the human ocular microbiota showed a lower relative abundance of Corynebacterium in eyes with fungal keratitis when compared with the normal eyes (Ge et al., 2019). That is, the susceptibility factors of BK may not be on account of the fluctuation of content for simply a certain bacterial genus, but the holistic Yin-Yang balance.
High-throughput sequencing technology provides a holistic horizon for the ocular microbiota. However, the network within the microbiota community and between the microbiota and host cells needs to be eventually verified based on culture technology. Microbiota information combined with other omics technologies, such as metagenomics, metatranscriptomics, metaproteomics and metabolomics, can predict suitable conditions for culture of hard-to-culture microorganisms (Vilanova and Porcar, 2016). Therefore, the combination of cultivation-dependent and cultivation-independent approaches could be helpful. Ziesack et al. (2019) have illuminated the interaction in a conglomerate composed of four bacterial species and have artificially regulated the microbiota structure based on bacterial metabolites. When the network relationship is thoroughly deciphered, the prevention and treatment of oculopathy through regulation of ocular microbiota becomes promising.
Shivaji et al. (2021) recently published similar study. Some of their patients had received recently antibiotic treatment, and a few healthy controls had undergone photorefractive keratectomy. Despite the difference in the inclusion criteria, our study both reported that Actinobacteria was significantly reduced in the ocular microbiota of diseased eyes of BK patients when compared with healthy subjects on examinations of conjunctival swabs and corneal scrapings. Compared with their study, this presented study involved additional conjunctival swabs from the normal eyes of BK patients. This may bridge the ocular microbiota before the occurrence and during the course of BK for BK patients. As a consequence, we focused more on the normal eyes of patients and healthy volunteers for exploration of ocular microbiota. Based on the deductive alteration of ocular microbiota before and after the development of BK, we deduced for the first time that the imbalance of protective and aggressive bacteria in the ocular microbiota may make eyes vulnerable to BK.
This study has limitations. Although volunteers had been recruited for a long period of time, the amount of participants was still limited. The abundances of bacteria cannot be quantitative analyzed because the process of high-throughput sequencing involved amplification steps. Furthermore, the reason for the imbalance of ocular microbiota was not ascertained. Since similar microbiotas can generate different interrelations and functions (Heintz-Buschart et al., 2016), further investigations on the regulation of the structure of ocular microbiota, metabolism, and antibiotic resistance would be made based on multi-omics technology. Besides, aberrations in the gut microbiota have been provided to be associated with ocular diseases in both human and animal studies (Floyd and Grant, 2020), however, this study did not explore the interaction between gut microbiota and BK.
Conclusion
High-throughput 16S rDNA sequencing challenged bacterial culture and could be a complement in identifying bacterial pathogens in corneas. Moreover, this study suggests the imbalance of protective and aggressive bacteria of ocular microbiota may trigger susceptibility to BK in humans. Regulation of ocular microbiota seems to be promising for prevention and treatment of ocular disease.
Data Availability Statement
The datasets presented in this study can be found in online repositories. The names of the repository/repositories and accession number(s) can be found below: NCBI BioProject, accession no: PRJNA692666.
Ethics Statement
The studies involving human participants were reviewed and approved by the Ethics Committee of Shandong Eye Institute. The patients/participants provided their written informed consent to participate in this study.
Author Contributions
ZR contributed to literature search, data analysis and drafting of the manuscript. QL contributed to the conjunctival swab collection and patient management. WL contributed to the design of this research and revision of the manuscript. XW contributed to literature search and data analysis. YD contributed to the clinical diagnosis. YH contributed to the design of this research, literature search, data analysis and revision of the manuscript. All authors contributed to the article and approved the submitted version.
Funding
This research was supported by the National Natural Science Foundation of China (grant number 81970788), the Key Science and Technology Innovation Project of Shandong Province (grant number 2018CXGC1205), and the Taishan Scholar Program (grant number ts20190983).
Conflict of Interest
All authors declare that the research was conducted in the absence of any commercial or financial relationships that could be construed as a potential conflict of interest.
Acknowledgments
All authors thank Ping Lin for her linguistic and editorial assistance, Huabo Chen for his operation of bacterial culture, Yuqian Wang for her assistance of sample collection and Qingdao OeBiotech. Co., Ltd. for high-throughput sequencing.
Supplementary Material
The Supplementary Material for this article can be found online at: https://www.frontiersin.org/articles/10.3389/fcimb.2021.645907/full#supplementary-material
References
Austin, A., Lietman, T., Rose-Nussbaumer, J. (2017). Update on the Management of Infectious Keratitis. Ophthalmology 124, 1678–1689. doi: 10.1016/j.ophtha.2017.05.012
Bograd, A., Seiler, T., Droz, S., Zimmerli, S., Fruh, B., Tappeiner, C. (2019). Bacterial and Fungal Keratitis: A Retrospective Analysis At a University Hospital in Switzerland. Klin. Monbl. Augenheilkd. 236, 358–365. doi: 10.1055/a-0774-7756
Bourcier, T., Thomas, F., Borderie, V., Chaumeil, C., Laroche, L. (2003). Bacterial Keratitis: Predisposing Factors, Clinical and Microbiological Review of 300 Cases. Br. J. Ophthalmol. 87, 834–838. doi: 10.1136/bjo.87.7.834
Cavuoto, K. M., Banerjee, S., Miller, D., Galor, A. (2018). Composition and Comparison of the Ocular Surface Microbiome in Infants and Older Children. Transl. Vis. Sci. Technol. 7, 16. doi: 10.1167/tvst.7.6.16
Chidambaram, J. D., Venkatesh Prajna, N., Srikanthi, P., Lanjewar, S., Shah, M., Elakkiya, S., et al. (2018). Epidemiology, Risk Factors, and Clinical Outcomes in Severe Microbial Keratitis in South India. Ophthal. Epidemiol. 25, 297–305. doi: 10.1080/09286586.2018.1454964
Dalitz, C. A., Porsani, M. V., Figel, I. C., Pimentel, I. C., Dalzoto, P. R. (2017). Potential for Biocontrol of Melanized Fungi by Actinobacteria Isolated From Intertidal Region of Ilha do Mel, Parana, Brazil. Braz. J. Microbiol. 48, 32–36. doi: 10.1016/j.bjm.2016.09.010
Dong, X., Wang, Y., Wang, W., Lin, P., Huang, Y. (2019). Composition and Diversity of Bacterial Community on the Ocular Surface of Patients With Meibomian Gland Dysfunction. Invest. Ophthalmol. Vis. Sci. 60, 4774–4783. doi: 10.1167/iovs.19-27719
Eguchi, H., Hotta, F., Kuwahara, T., Imaohji, H., Miyazaki, C., Hirose, M., et al. (2017). Diagnostic Approach to Ocular Infections Using Various Techniques From Conventional Culture to Next-Generation Sequencing Analysis. Cornea 36, S46–S52. doi: 10.1097/ICO.0000000000001338
Faith, D. P., Baker, A. M. (2007). Phylogenetic Diversity (PD) and Biodiversity Conservation: Some Bioinformatics Challenges. Evol. Bioinform. Online 17, 121–128.
Floyd, J. L., Grant, M. B. (2020). The Gut-Eye Axis: Lessons Learned From Murine Models. Ophthalmol. Ther. 9, 499–513. doi: 10.1007/s40123-020-00278-2
Ge, C., Wei, C., Yang, B.-X., Cheng, J., Huang, Y.-S. (2019). Conjunctival Microbiome Changes Associated With Fungal Keratitis: Metagenomic Analysis. Int. J. Of Ophthalmol. 12, 194–200. doi: 10.18240/ijo.2019.02.02
Heintz-Buschart, A., May, P., Laczny, C. C., Lebrun, L. A., Bellora, C., Krishna, A., et al. (2016). Integrated Multi-Omics of the Human Gut Microbiome in a Case Study of Familial Type 1 Diabetes. Nat. Microbiol. 2, 16180. doi: 10.1038/nmicrobiol.2016.180
Hill, T. C. J., Walsh, K. A., Harris, J. A., Moffett, B. F. (2003). Using Ecological Diversity Measures With Bacterial Communities. FEMS Microbiol. Ecol. 43, 1–11. doi: 10.1111/j.1574-6941.2003.tb01040.x
Huang, Y., Yang, B., Li, W. (2016). Defining the Normal Core Microbiome of Conjunctival Microbial Communities. Clin. Microbiol. Infect. 22, 643 e647–643 e612. doi: 10.1016/j.cmi.2016.04.008
Integrative HMPRNC (2019). The Integrative Human Microbiome Project. Nature 569, 641–648. doi: 10.1038/s41586-019-1238-8
Kim, B. R., Shin, J., Guevarra, R., Lee, J. H., Kim, D. W., Seol, K. H., et al. (2017). Deciphering Diversity Indices for a Better Understanding of Microbial Communities. J. Microbiol. Biotechnol. 27, 2089–2093. doi: 10.4014/jmb.1709.09027
Kugadas, A., Wright, Q., Geddes-McAlister, J., Gadjeva, M. (2017). Role of Microbiota in Strengthening Ocular Mucosal Barrier Function Through Secretory Iga. Invest. Ophthalmol. Vis. Sci. 58, 4593–4600. doi: 10.1167/iovs.17-22119
Lai, Y., Di Nardo, A., Nakatsuji, T., Leichtle, A., Yang, Y., Cogen, A. L., et al. (2009). Commensal Bacteria Regulate Toll-like Receptor 3-Dependent Inflammation After Skin Injury. Nat. Med. 15, 1377–1382. doi: 10.1038/nm.2062
Langille, M. G., Zaneveld, J., Caporaso, J. G., McDonald, D., Knights, D., Reyes, J. A., et al. (2013). Predictive Functional Profiling of Microbial Communities Using 16S rRNA Marker Gene Sequences. Nat. Biotechnol. 31, 814–821. doi: 10.1038/nbt.2676
Ledger, W. J. (1993). Historical Review of the Treatment of Bacterial Vaginosis. Am. J. Obstet Gynecol. 169, 474–478. doi: 10.1016/0002-9378(93)90345-J
Lin, A., Rhee, M. K., Akpek, E. K., Amescua, G., Farid, M., Garcia-Ferrer, F. J., et al. (2019). Bacterial Keratitis Preferred Practice Pattern(R). Ophthalmology 126, P1–P55. doi: 10.1016/j.ophtha.2018.10.018
Mascarenhas, J., Lalitha, P., Prajna, N. V., Srinivasan, M., Das, M., D’Silva, S. S., et al. (2014). Acanthamoeba, Fungal, and Bacterial Keratitis: A Comparison of Risk Factors and Clinical Features. Am. J. Of Ophthalmol. 157, 56–62. doi: 10.1016/j.ajo.2013.08.032
Nilsson, R. H., Kristiansson, E., Ryberg, M., Hallenberg, N., Larsson, K.-H. (2008). Intraspecific ITS Variability in the Kingdom Fungi as Expressed in the International Sequence Databases and Its Implications for Molecular Species Identification. Evol. Bioinform. Online 26, 193–201. doi: 10.4137/EBO.S653
Onderdonk, A. B., Delaney, M. L., Fichorova, R. N. (2016). The Human Microbiome During Bacterial Vaginosis. Clin. Microbiol. Rev. 29, 223–238. doi: 10.1128/CMR.00075-15
Ong, H. S., Corbett, M. C. (2015). Corneal Infections in the 21st Century. Postgrad. Med. J. 91, 565–571. doi: 10.1136/postgradmedj-2015-133323
Redelinghuys, M. J., Geldenhuys, J., Jung, H., Kock, M. M. (2020). Bacterial Vaginosis: Current Diagnostic Avenues and Future Opportunities. Front. Cell Infect. Microbiol. 10, 354. doi: 10.3389/fcimb.2020.00354
Ren, Z., Liu, Q., Wang, Y., Dong, Y., Huang, Y. (2020). Diagnostic Information Profiling and Evaluation of Causative Fungi of Fungal Keratitis Using High-Throughput Internal Transcribed Spacer Sequencing. Sci. Rep. 10, 1640. doi: 10.1038/s41598-020-58245-7
Shah, A., Sachdev, A., Coggon, D., Hossain, P. (2011). Geographic Variations in Microbial Keratitis: An Analysis of the Peer-Reviewed Literature. Br. J. Ophthalmol. 95, 762–767. doi: 10.1136/bjo.2009.169607
Sharma, S. (2012). Diagnosis of Infectious Diseases of the Eye. Eye (Lond) 26, 177–184. doi: 10.1038/eye.2011.275
Shivaji, S., Jayasudha, R., Chakravarthy, S. K., SaiAbhilash, C. R., Sai Prashanthi, G., Sharma, S., et al. (2021). Alterations in the Conjunctival Surface Bacterial Microbiome in Bacterial Keratitis Patients. Exp. Eye Res. 203, 108418. doi: 10.1016/j.exer.2020.108418
Shivaji, S., Jayasudha, R., Sai Prashanthi, G., Kalyana Chakravarthy, S., Sharma, S. (2019). The Human Ocular Surface Fungal Microbiome. Invest. Ophthalmol. Visual Sci. 60, 451–459. doi: 10.1167/iovs.18-26076
St Leger, A. J., Desai, J. V., Drummond, R. A., Kugadas, A., Almaghrabi, F., Silver, P., et al. (2017). An Ocular Commensal Protects Against Corneal Infection by Driving an Interleukin-17 Response From Mucosal Gammadelta T Cells. Immunity 47, 148–158.e145. doi: 10.1016/j.immuni.2017.06.014
Valliappan, K., Sun, W., Li, Z. (2014). Marine Actinobacteria Associated With Marine Organisms and Their Potentials in Producing Pharmaceutical Natural Products. Appl. Microbiol. Biotechnol. 98, 7365–7377. doi: 10.1007/s00253-014-5954-6
Vilanova, C., Porcar, M. (2016). Are Multi-Omics Enough? Nat. Microbiol. 1, 16101. doi: 10.1038/nmicrobiol.2016.101
Wang, Y., Chen, H., Xia, T., Huang, Y. (2019). Characterization of Fungal Microbiota on Normal Ocular Surface of Humans. Clin. Microbiol. Infect. 26(1), 123.e9–123.e13. doi: 10.1016/j.cmi.2019.05.011
Wang, Q., Garrity, G. M., Tiedje, J. M., Cole, J. R. (2007). Naive Bayesian Classifier for Rapid Assignment of rRNA Sequences Into the New Bacterial Taxonomy. Appl. Environ. Microbiol. 73, 5261–5267. doi: 10.1128/AEM.00062-07
Willcox, M. D. (2013). Characterization of the Normal Microbiota of the Ocular Surface. Exp. Eye Res. 117, 99–105. doi: 10.1016/j.exer.2013.06.003
Yan, C., Luo, Z., Li, W., Li, X., Dallmann, R., Kurihara, H., et al. (2020). Disturbed Yin-Yang Balance: Stress Increases the Susceptibility to Primary and Recurrent Infections of Herpes Simplex Virus Type 1. Acta Pharm. Sin. B. 10, 383–398. doi: 10.1016/j.apsb.2019.06.005
Keywords: ocular microbiome, bacterial keratitis, causative bacteria, latent susceptibility, dysbiosis, next-generation sequencing
Citation: Ren Z, Liu Q, Li W, Wu X, Dong Y and Huang Y (2021) Profiling of Diagnostic Information of and Latent Susceptibility to Bacterial Keratitis From the Perspective of Ocular Bacterial Microbiota. Front. Cell. Infect. Microbiol. 11:645907. doi: 10.3389/fcimb.2021.645907
Received: 24 December 2020; Accepted: 23 April 2021;
Published: 13 May 2021.
Edited by:
Jerome Ozkan, University of New South Wales, AustraliaReviewed by:
Mihaela Gadjeva, Harvard Medical School, United StatesJudith Flanagan, University of New South Wales, Australia
Copyright © 2021 Ren, Liu, Li, Wu, Dong and Huang. This is an open-access article distributed under the terms of the Creative Commons Attribution License (CC BY). The use, distribution or reproduction in other forums is permitted, provided the original author(s) and the copyright owner(s) are credited and that the original publication in this journal is cited, in accordance with accepted academic practice. No use, distribution or reproduction is permitted which does not comply with these terms.
*Correspondence: Yusen Huang, aHVhbmdfeXVzZW5AMTI2LmNvbQ==