- 1Key Laboratory of Tropical Translational Medicine of Ministry of Education and School of Tropical Medicine and Laboratory Medicine, Hainan Medical University, Haikou, China
- 2CAS Center for Excellence in Biotic Interactions, University of Chinese Academy of Sciences, Beijing, China
- 3State Key Laboratory of Integrated Management of Pest Insects and Rodents, Institute of Zoology, Chinese Academy of Sciences, Beijing, China
The midgut microbial community composition, structure, and function of field-collected mosquitoes may provide a way to exploit microbial function for mosquito-borne disease control. However, it is unclear how adult mosquitoes acquire their microbiome, how the microbiome affects life history traits and how the microbiome influences community structure. We analyzed the composition of 501 midgut bacterial communities from field-collected adult female mosquitoes, including Aedes albopictus, Aedes galloisi, Culex pallidothorax, Culex pipiens, Culex gelidus, and Armigeres subalbatus, across eight habitats using the HiSeq 4000 system and the V3−V4 hyper-variable region of 16S rRNA gene. After quality filtering and rarefaction, a total of 1421 operational taxonomic units, belonging to 29 phyla, 44 families, and 43 genera were identified. Proteobacteria (75.67%) were the most common phylum, followed by Firmicutes (10.38%), Bacteroidetes (6.87%), Thermi (4.60%), and Actinobacteria (1.58%). The genera Rickettsiaceae (33.00%), Enterobacteriaceae (20.27%), Enterococcaceae (7.49%), Aeromonadaceae (7.00%), Thermaceae (4.52%), and Moraxellaceae (4.31%) were dominant in the samples analyzed and accounted for 76.59% of the total genera. We characterized the midgut bacterial communities of six mosquito species in Hainan province, China. The gut bacterial communities were different in composition and abundance, among locations, for all mosquito species. There were significant differences in the gut microbial composition between some species and substantial variation in the gut microbiota between individuals of the same mosquito species. There was a marked variation in different mosquito gut microbiota within the same location. These results might be useful in the identification of microbial communities that could be exploited for disease control.
Introduction
Mosquitoes (Culicidae) are vectors of many human diseases. To satisfy the reproductive nutritional needs, females required repeated blood meal from a host. This can result in the initial acquisition and later transmission of pathogens (Wang et al., 2017). Mosquitoes are the main vectors of several kinds of pathogenic factors of various human infectious diseases, such as O’nyong-nyong, Zika virus, dengue virus, chikungunya virus, and West Nile virus (Barrett and Higgs, 2007; Fauci et al., 2016; Tsetsarkin et al., 2016; Weaver et al., 2016; Rezza et al., 2017). The transmission of mosquito-borne pathogens involves interactions between vectors, pathogens, and vertebrate hosts. Once the mosquito has ingested a blood meal from an infected host, the pathogen first invades the midgut epithelium. It then moves through the hemolymph to secondary tissues, such as the trachea and fat body, and finally, infects the salivary glands. At this stage, the pathogen contaminates mosquito saliva and is injected into a vertebrate host when the mosquito takes a blood meal (Wang and Jacobs-Lorena, 2013; Sim et al., 2014; Wang et al., 2017). Through this process, the mosquito is infectious and can transmit the pathogen. However, the mosquito midgut has factors that might impede successful transmission of the pathogen and also affect the biology of the host (Wang et al., 2011; Baia-da-Silva et al., 2019). These factors include components of the mosquito innate immune system, such as lectins, bacteria-derived cytolysins (hemolysins), antimicrobial peptides, peroxidase, proteases, digestive enzymes, secondary metabolites, nitric oxide, and prophenoloxidase (Ramirez et al., 2012; Sim et al., 2014; Wang et al., 2019).
Culture-dependent and culture-independent approaches have been used to study the microbial communities in mosquito midguts. Midgut bacteria of mosquitoes have often coevolved with their hosts, and many of these endosymbionts cannot be cultured on standard microbiological media (Gandotra et al., 2018). Gut bacteria that can be cultured illustrate symbiotic associations. Some culturable gut symbionts have been functionally characterized in their host insects. For pathogen control, some studies have used genetic engineering techniques on symbiotic bacteria. Symbiotic bacteria are used to deliver anti-pathogen effector molecules to the midgut lumen and render host mosquitoes refractory to pathogen infection (Wang et al., 2012). The composition and diversity of gut microbiota vary within and between different mosquito species. Microbiota are influenced by host diet, developmental stage, population location, larval environment and pathogen infection (Muturi et al., 2016a). Studies on the microbial communities from different locations and species of mosquitoes can improve understanding of the gut microbiota. There is also a need to extend microbiome studies to other mosquito-borne disease systems that may be used for disease control.
Hainan province, in southern China, is separated by a strait from Guangdong Province and situated between latitudes 18°10’ N and 20°10’ N and longitudes 108°37’ E and 111°03’ E. The Island has a convergence of tropical and subtropical areas with a climate and environment favorable for mosquitoes. Most Hainan studies have focused on the surveillance and control of the vectors of Zika virus and malaria (Wang et al., 2014; Yasri and Wiwanitkit, 2017). The molecular characterization of species and the effects of insecticides or antifungal agents have also been investigated (Sun et al., 2017; Li et al., 2018; Ruiling et al., 2018). Little is known about bacteria in the mosquito midguts or microbial symbionts. To gain a better understanding of the diversity and function of mosquito gut microbiota in Hainan province, we conducted microbiome studies. We identified bacteria in the midguts of mosquitoes from eight geographical areas in Hainan to evaluate differences in diversity, composition, and structure of midgut microbiota between collection sites and mosquito species.
Materials and Methods
Mosquito Collection and Morphology Identification
Adult mosquitoes were collected in 2018 (July 1 to September 18) from eight sites in Hainan province, China (H1-H8: H1 = Haikou; H2 = Dingan; H3 = Wenchang; H4 = Tunchang; H5 = Wuzhishan; H6 = Lingshui; H7 = Sanya; H8 = Ledong) (Figure 1). Mosquitoes were collected using nets, aspirators, light traps coupled with a CO2 source, and human landings. The trap sites we selected include households, the woods, residential areas, near the pool, parks, and construction sites. Specifically, a light trap was placed at the sheltered site away from light and about 1.5 m above the ground. The light was on, and surveillance was performed at night from 1 h before sunset to 1 h after sunrise (Li et al., 2019). During the day, adult mosquitoes were collected in using human-landing catches. Larvae and pupae were sampled with pipettes and sieves. Adult mosquitoes from immature samples were collected after eclosion. Mosquitoes were identified to species using the keys of Wilke (Wilke et al., 2016), and returned to the laboratory. Species identifications were confirmed using a diagnostic PCR assay based on DNA barcode analysis (Ashfaq et al., 2014).
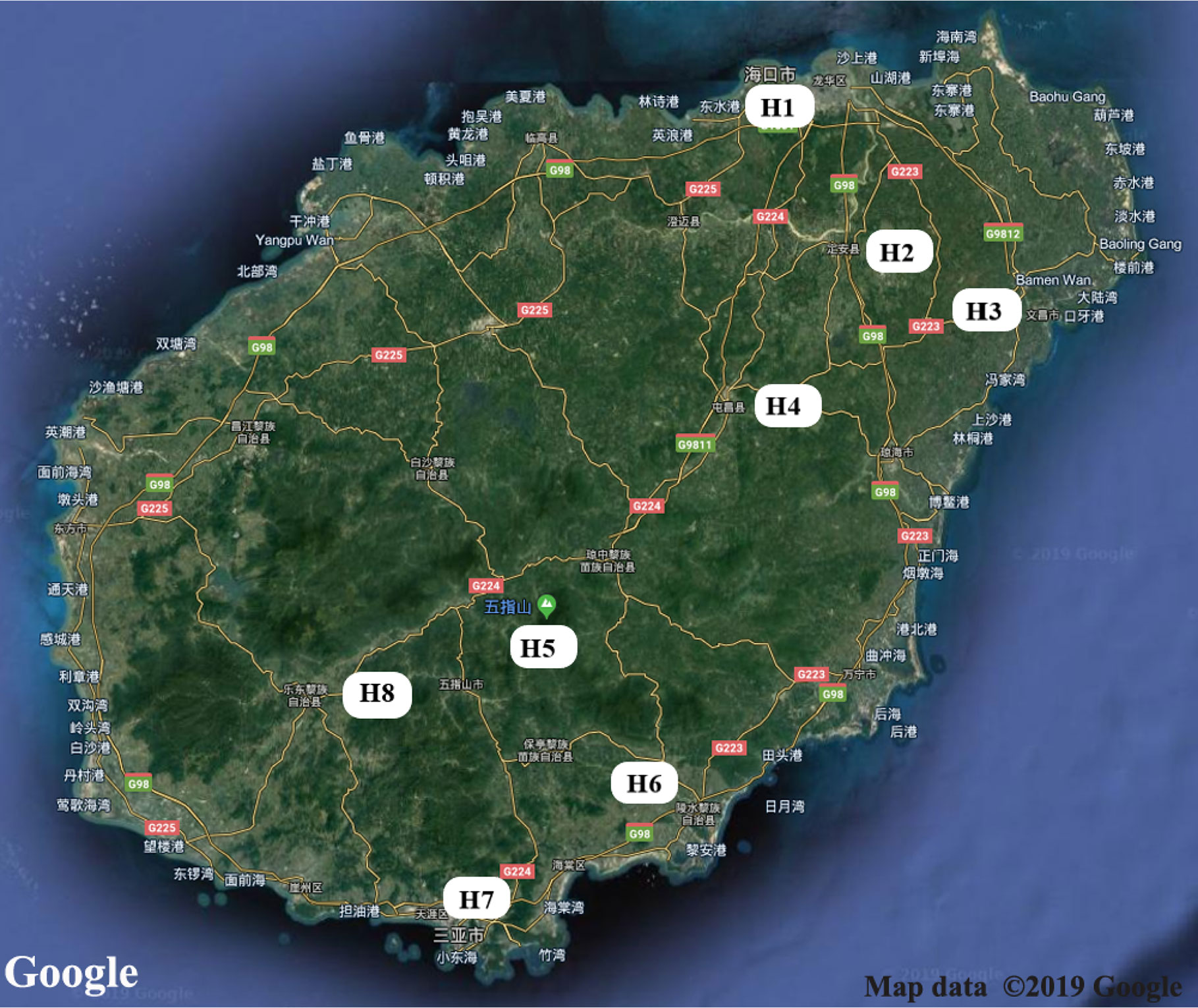
Figure 1 Map of Hainan province showing locations of the eight collection study sites. H1 = Haikou, Urbanna; H2 = Dingan, Country; H3 = Wenchang, Urbanna; H4 = Tunchang, farm; H5 = Wuzhishan, montane; H6 = Lingshui, montane; H7 = Sanya, Urbanna; H8 = Ledong, Urbanna.
Molecular Identification of Mosquitoes
For species identification, two legs were removed from each female adult and transferred to a well pre-loaded with 200 μl of 95% ethanol in a 96-well microplate. PCR was performed to amplify the 5’ cytochrome c oxidase subunit 1 (cox1) region of the mitochondrial DNA (Kumar et al., 2007) using the forward primer LCO1490 (5′-GGTCAACAAATCATAAAGATATTGG-3′) and reverse primer HCO2198 (5′-TAAACTTCAGGGTGACCAAAAAATCA-3′) (Steyn et al., 2016). Each amplification was performed in a 50 µl volume that included 1 µl of DNA template, 2.5 µl of the forward and reverse primers, 25 µl of 2×Taq PCR Mastermix (KT201), and 21.5 µl of Millipore water. The PCR cycle included initial denaturation 95°C for 3 min, 35 cycles each of denaturation 95°C for 30 s, primer annealing 55°C for 30 s, and primer extension 72°C for 1.5 min followed by 10 min extension at 72°C and storage at 4°C. PCR products were run in 1.0% agarose gel stained with ethidium bromide (EB) and visualized in a gel imaging system.
Midgut Dissection and DNA Extraction
All mosquitoes were kept in 1.5 ml Eppendorf tubes at –80˚C until DNA extraction, and all were processed at the same time to limit batch effects (Krajacich et al., 2018). The microbial communities were identified from a pooled sample. After molecular identification, a total of 11–30 midguts were dissected for each mosquito species, and the samples were evenly distributed, and three groups were repeated. Each female mosquito was surface sterilized in 75% ethanol for 10 min, then rinsed three times in sterile PBS solution. Midguts were dissected and stored individually at −20°C until processing. DNA was extracted using the DNeasy Blood & Tissue Kit following manufacturer instructions (Qiagen, Valencia, CA, USA). Isolated DNA was reconstituted in 50 μl of ddH2O and stored at −20°C for further processing. A 50 μl aliquot of the DNA isolate was used to build a microbiome library for Illumina HiSeq sequencing at BGI Shenzhen (Shenzhen, China).
Sample Processing and 16S rRNA Gene Library Preparation
A total of 501 midguts, from different mosquito species at eight sites, were processed (Table 1). The hypervariable regions V3−V4 of the bacterial 16S rRNA were amplified with primers 338F (5′-ACTCCTACGGGAGGCAGCAG-3′) and 806R (5′-GGACTACHVGGGTWTCTAAT-3′) (Zhang et al., 2018) containing multiplex identifier sequences (Wu et al., 2016). The PCR reaction of purified DNA from each midgut sample was set up as follows: each 25 μl reaction consisted of microbial DNA (5 ng/μl) 2.5 μl; amplicon PCR reverse primer (1 μmol/L) 5 μl; amplicon PCR forward primer (1 μmol/L) 5 μl; 2× KAPA HiFi Hot Start Ready Mix 12.5 μl. The plate was sealed, and PCR was performed in a thermal instrument (Applied Biosystems 9700, USA) including an initial denaturation step at 95°C for 3 min, followed by 25 cycles of denaturing at 95°C for 30 s, annealing at 55°C for 30 s, elongation at 72°C for 30 s, and a final extension at 72°C for 5 min (Wu et al., 2016). The PCR products were checked using electrophoresis in 1.0% (w/v) agarose gels in TBE buffer (Tris, boric acid, EDTA) stained with EB and visualized under UV light. For PCR products, the jagged ends of DNA fragments were converted into blunt ends using T4 DNA polymerase, Klenow Fragment, and T4 Polynucleotide Kinase. Then we added an ‘A’ base to each 3’ end to ease addition of adapters. Afterwards, short fragments were removed by Ampure beads. For genomic DNA, we used a fusion primer with dual index and adapters for PCR and short fragments were also removed by Ampure beads. In both cases, only the qualified Illumina library was used for sequencing on HiSeq 4000 system.
Data Processing, Filtering, and Fragment Assembly
To obtain more accurate results, raw data were pre-processed to get clean data using the following procedure: (1) Truncation of sequence reads lacking an average quality of 20 over a 30 bp sliding window based on the Phred algorithm, and trimming of reads with less than 75% of their original length, as well as paired reads; (2) Removal of reads contaminated by adapter (default parameter was 15 bases overlapped by reads and an adapter with more than three mismatched bases); (3) Removal of reads with an ambiguous base (N base), and its paired reads; (4) Removal of reads with low complexity (default: reads with 10 consecutive same bases). If the two paired-end reads overlapped, the consensus sequence was generated by the Software FLASH (Fast Length Adjustment of Short reads v1.2.11) (Magoc and Salzberg, 2011). The criteria were as follows: Minimal overlapping length was 15 bp and the allowable reads had an error outed using Quantitative Insights into Microbial Ecology v1.9.1 (QIIME) (Caporaso et al., 2010) quality filters.
OTU Clustering, Annotation, and Analysis
High-quality sequences were grouped in OTUs with an open-reference selection method at 97% similarity threshold (Navas-Molina et al., 2013) using USEARCH v 7.0.1090 (Edgar, 2013). UCHIME v4.2.40 (Edgar et al., 2011) was used to remove the chimeric sequences by PCR amplification from the OTU representative sequence. All tags are mapped to each OTU representative sequence using USEARCH GLOBAL (Wang et al., 2007). The tag number of each OTU in each sample was summarized by an OTU abundance table. OTU representative sequences were classified using the Ribosomal Database Project (RDP) Classifier v.2.2 (https://rdp.cme.msu.edu/index.jsp) (Wang et al., 2011) and trained on the Greengenes database. The parameters had confidence values of 0.8 if the length of tags was ≥ 250 bp; otherwise 0.5 confidence values were used as the cutoff. The taxonomic assignment was based on comparison with the closest matched sequences on the Greengene (v201305) (http://greengenes.lbl.gov/cgi-bin/nph-index.cgi) database. We removed unassigned OTUs and we removed OTUs that were not assigned to the target species. The filtered OTUs were used for downstream processing.
Bacterial Diversity Analysis
To assure that a randomly selected amplicon from a sample was previously sequenced, we used Good’s coverage index (the number of OTUs sampled more than once divided by the total number of OTUs) to estimate sequencing depths implemented in Mothur (http://www.mothur.org/wiki/Calculators). Rarefaction curves were drawn using the mean indices calculated with extracted tags, in R software, as a function of the number of randomly sampled tags with the vegan package (Huber et al., 2015).
Alpha diversity (α-diversity) describes within sample diversity, including species richness and evenness. Alpha diversity indices were calculated by Mothur v1.31.2. Corresponding rarefaction curves and box/bar plots were drawn using R software. The midgut bacterial communities’ indices included species richness (observed species, Chao1, ACE) and species diversity (Simpson index and Shannon). For calculation of each index we used formulas in http://www.mothur.org/wiki/Calculators. Differential analysis among groups was done using the alpha diversity indices. The Wilcoxon Rank-Sum Test was used for comparisons between two groups, while the Kruskal-Wallis Test was used for multi-group comparisons (Schloss et al., 2009). The analyses were performed using R v3.1.1 software.
Alpha diversity describes within sample diversity while beta diversity (β-diversity) describes the similarity of two samples. QIIME (Caporaso et al., 2010). The β-diversity of gut bacterial communities among locations was estimated using the Bray-Curtis dissimilarity index (Hernandez-Garcia et al., 2018). The unweighted Bray-Curtis index considers only phylogenetic richness and the weighted index considers both relative abundance and phylogenetic richness (Lozupone et al., 2010). Unweighted and weighted UniFrac Principal Coordinate Analysis (PCoA) plots were used to visualize microbial community structure relationships. PCoA (Mohammadi Shahrestani et al., 2015) was applied to taxon abundance profiles, including phylum, class, order, family, genus and species profiles. The ‘ade4’ package in R v3.1.1 was used for PCoA analysis.
The difference in the abundance of microbial communities between the two groups of samples was tested statistically, and the FDR (false discovery rate) was used to assess the significance of the difference. Metastats software (http://metastats.cbcb.umd.edu/) (default) or R software (Rank sum test, Fisher’s exact test, Chi-square test, T-test and Variance test) for significant differences were used for analysis. The correction of the p-value is performed via p-adjust in the R package (v3.1.1), and the correction method is BH (Benjamini - CHochberg) (White et al., 2009)
Results
Mosquito Species From Different Sampling Areas
We identified six mosquito species: Aedes albopictus, Aedes galloisi, Culex pallidothorax, Culex pipiens, Culex gelidus, and Armigeres subalbatus. These mosquito species had distinct geographical distributions. Ae. albopictus was found in all eight collection sites; Ar. subalbatus was found in Haikou, Wenchang, Sanya, and Wuzhishan; Cx pallidothorax was found in Tunchang, Dingan, and Wenchang; Cx. pipiens was found in Haikou, Wuzhishan, Lingshui, and Haikou; Ae. galloisi and Cx. gelidus were found in Lingshui and Haikou, respectively (Table 1). These results suggest that many mosquito species in Hainan are widely distributed and have regional characteristics.
Midgut Bacterial Species Composition Across Mosquito Species
We analyzed the V3-V4 hyper-variable region of the 16S rRNA gene to estimate the composition of bacterial communities in 501 midguts of field-collected adult female mosquitoes. A total of 5,154,464 raw reads (Table S1) (Mean ± SE = 62859.32 ± 7871.44 per mosquito midgut sample) were obtained from the 501 mosquito samples that were sequenced. After quality control, 4,585,398 clean reads (Table S1) remained for subsequent analyses.
Filtered tags were clustered into OTU (Operational Taxonomic Units) with 97% similarity. These sequences were clustered into 1,421 bacterial OTUs belonging to 29 phyla, 44 families, and 43 genera (including one unclassed and others) (Table S2). To avoid obvious deviations in the microbial richness, OTUs with relative abundance of more than 0.5% were selected for comparative analysis. Most of the sequences came from Proteobacteria (75.67%), including Alphaproteobacteria (38.84%), Gammaproteobacteria (35.43%), Betaproteobacteria (1.38%) (Figure 2A; Table S3). Other observed phyla included Firmicutes (10.38%), Bacteroidetes (6.87%), Thermi (4.60%), Actinobacteria (1.58%), Spirochaetes (0.35%), Cyanobacteria (0.28%), Tenericutes (0.20%), Chloroflexi (0.02%), TM7 (0.01%), Acidobacteria (0.009%), and Unclassified (0.002%) (Figure 2A; Table S3).
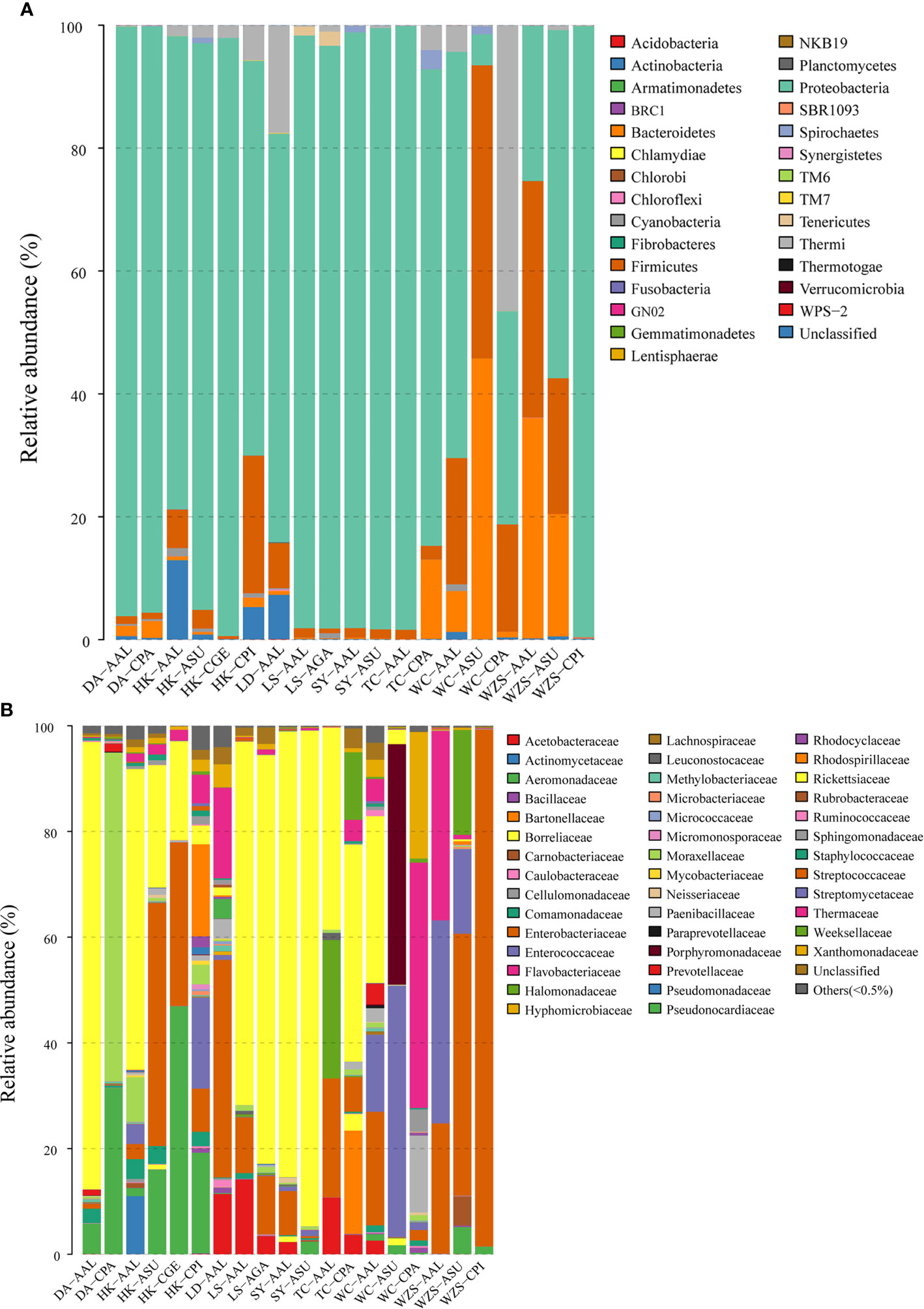
Figure 2 Mean relative abundances of bacterial phyla (A) and families (B) associated with six mosquito species at different sites and collection dates. Families with abundance less than 0.5% were pooled together as “Other.” (DA, Dingan; HK, Haikou; LD, Ledong; LS, Lingshui; SY, Sanya; TC, Tunchang; WC, Wenchang; WZS, Wuzhishan; AAL, Aedes albopictus; ASU, Armigeres subalbatus; CGE, Culex gelidus; AGA, Aedes galloisi; CPA, Culex pallidothorax; CPI, Culex pipiens).
Families with the highest OTU abundance included Rickettsiaceae (33.00%), Enterobacteriaceae (20.27%), Enterococcaceae (7.49%), Aeromonadaceae (7.00%), Thermaceae (4.52%), and Moraxellaceae (4.31%) (Figure 2B; Table S3). At the genus level, the most abundant microorganisms in the guts of mosquitoes were Wolbachia (31.89%), Unclassified (25.90%), Enterococcus (7.48%), Thermus (4.52%), Acinetobacter (4.28%), Escherichia (2.74%), Enterobacter (2.61%), Dysgonomonas (2.40%), Swaminathania (2.33%), Thorsellia (2.16%), Serratia (1.76%), Providencia (1.33%), and Rickettsia (1.10%). Other genera with relative abundance less than 0.5% were identified (Table S3; Figure S1).
Rickettsiaceae occurred in high abundance among all mosquito species except Ae. albopictus and Cx. pipiens from Wuzhishan. However, their amount was fewer in Cx. pipiens, Cx. pallidothorax, and Cx. gelidus compared to the remaining mosquito species. Among the Rickettsaceae, Wolbachia was more prevalent and abundant in the guts of Ae. albopictus and Ae. galloisi from most areas but had low abundance in Ar. subalbatus, Cx. pipiens and Ae. albopictus from Wuzhishan. Enterobacteriaceae was more common among Ae. albopictus from Ledong, Tunchangand, and Wenchang; it occurred in high abundance in the guts of a few individuals of Ar. subalbatus and Cx. pipiens from Wuzhishan (Figure 2B; Table S3). At the genus level, there were many unclassified, especially in Ae. albopictus and Cx. pipiens mosquitoes in Wuzhishan, which accounted for 60.30% and 99.14% of the total (Table S3).
The gut bacterial communities were different in composition and abundance among the locations of all mosquito species. The shifts of microbial compositions in dominant genera are shown on the heat map (Figure 3).
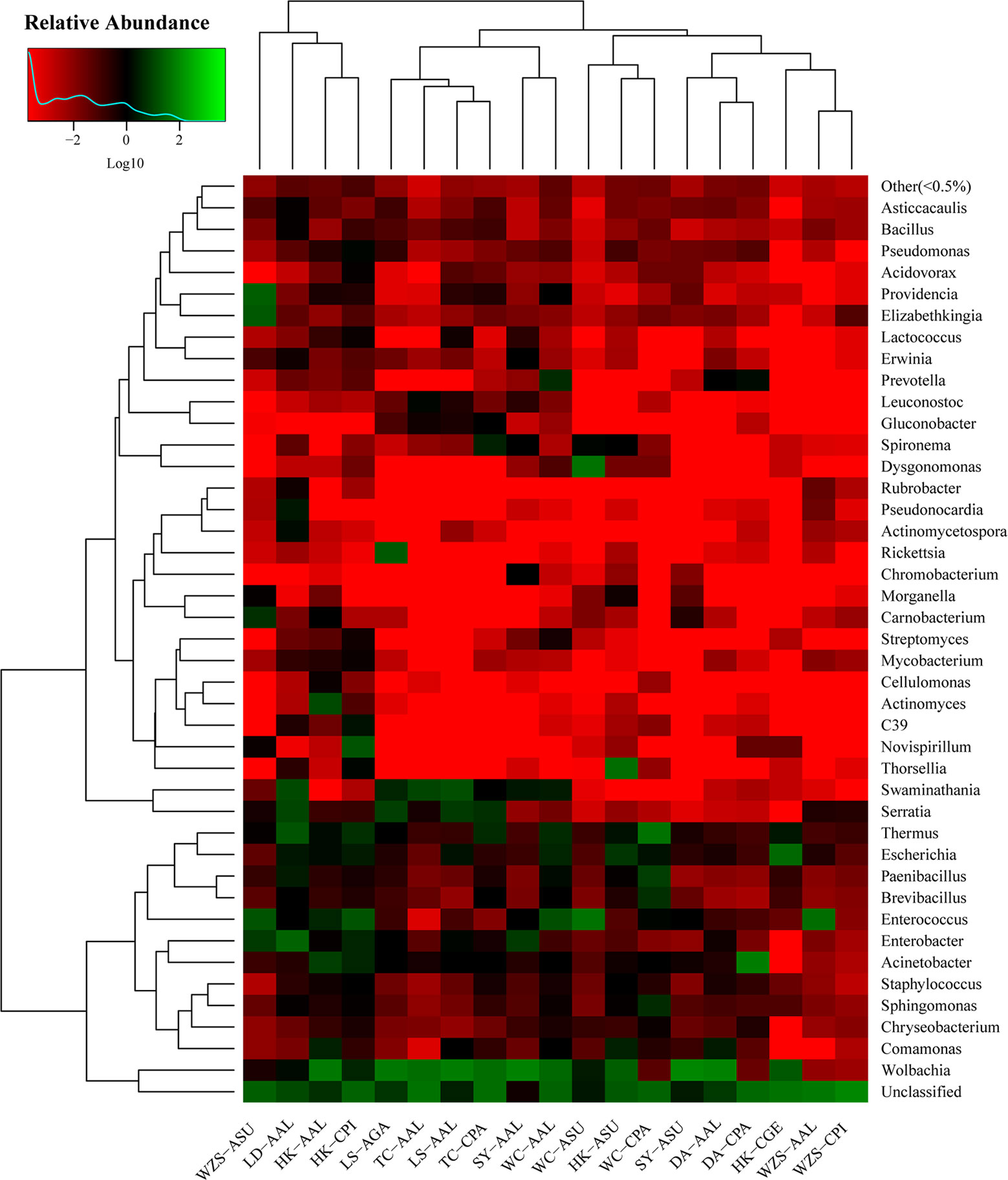
Figure 3 Heatmap in log scale depicting the gut bacterial community of mosquito midguts obtained with open reference OTU picking methods. Green colors represent high abundance and red colors represent low abundance; black indicates absence.
Diversity of Mosquito Microbiota
Rarefaction analysis showed that the microbial richness and diversity varied among individual mosquitoes, since the libraries were sampled and prepared at different depth (Figures 4A, B). Rarefaction curves for some individual mosquitoes did not plateau, indicating the potential for unrecovered rare bacterial taxa. To determine whether bacterial diversity and richness varied significantly among mosquito species and study sites, we computed the alpha diversity indices along with 95% confidence intervals (Table 2).
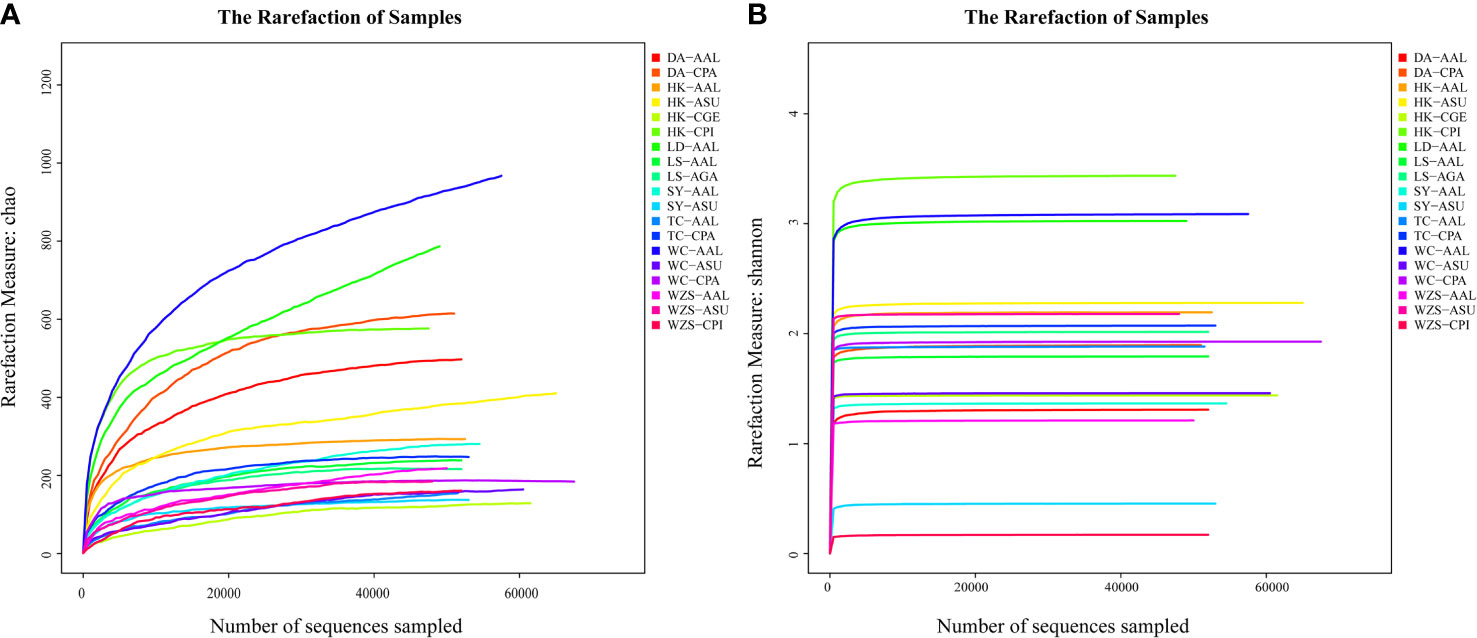
Figure 4 Rarefaction analysis of observed richness Chao (A) and Shannon index (B) within individual mosquitoes.
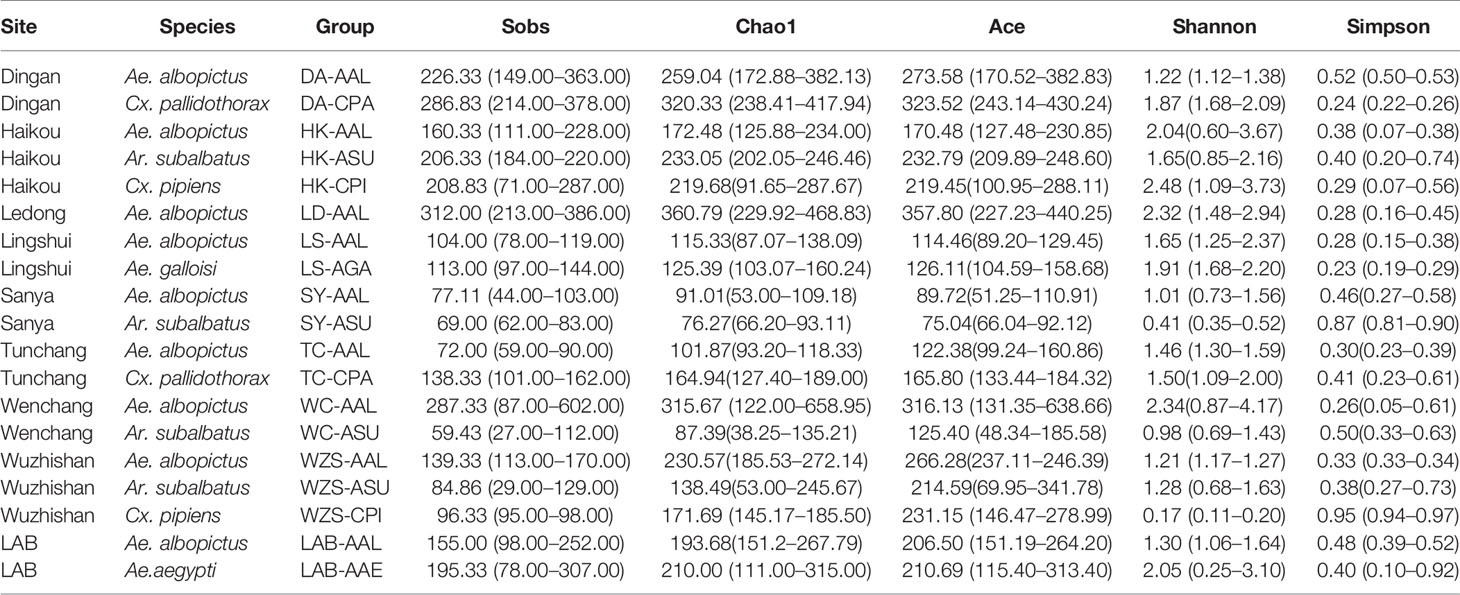
Table 2 Diversity and richness (mean and 95% confidence limits) of the midgut bacterial communities of six mosquito species from eight sites in Hainan province.
The midgut bacterial communities’ indices included species richness (observed species, Chao1, ace) and species diversity (Simpson index and Shannon) (Table 2). Chao1 and ace of species richness means the number of species in the community, regardless of the abundance of each species in the community. Shannon diversity and Simpson diversity of species diversity influenced by species richness and species evenness in the sampled community. The species richness was estimated by Chao1, and the difference was statistically significant in alpha diversity. Bacterial richness was significantly higher among Ae. albopictus from Ledong compared to Ae. albopictus from Sanya (Kruskal-Wallis test: p = 0.001, Fig 5A), Ar. subalbatus from Sanya (p = 0.036, Fig 5A) and Ae. albopictus from Wenchang. (p = 0.001, Fig 5A). In addition, Cx. pallidothorax from Dingan had significantly higher bacterial richness compared to Ae. albopictus from Sanya (p=0.003, Fig 5A) and Ar. subalbatus from Wenchang (p = 0.002, Figure 5A). The species diversity was estimated by Shannon, significantly different in Ae. albopictus from Dingan compared to Cx. pipiens from Wuzhishan (p = 0.027, Figure 5B). Species diversity was observed in other groups, but the difference was not statistically significant (Figure 5B). Values from all statistical tests are available in additional Table 2.
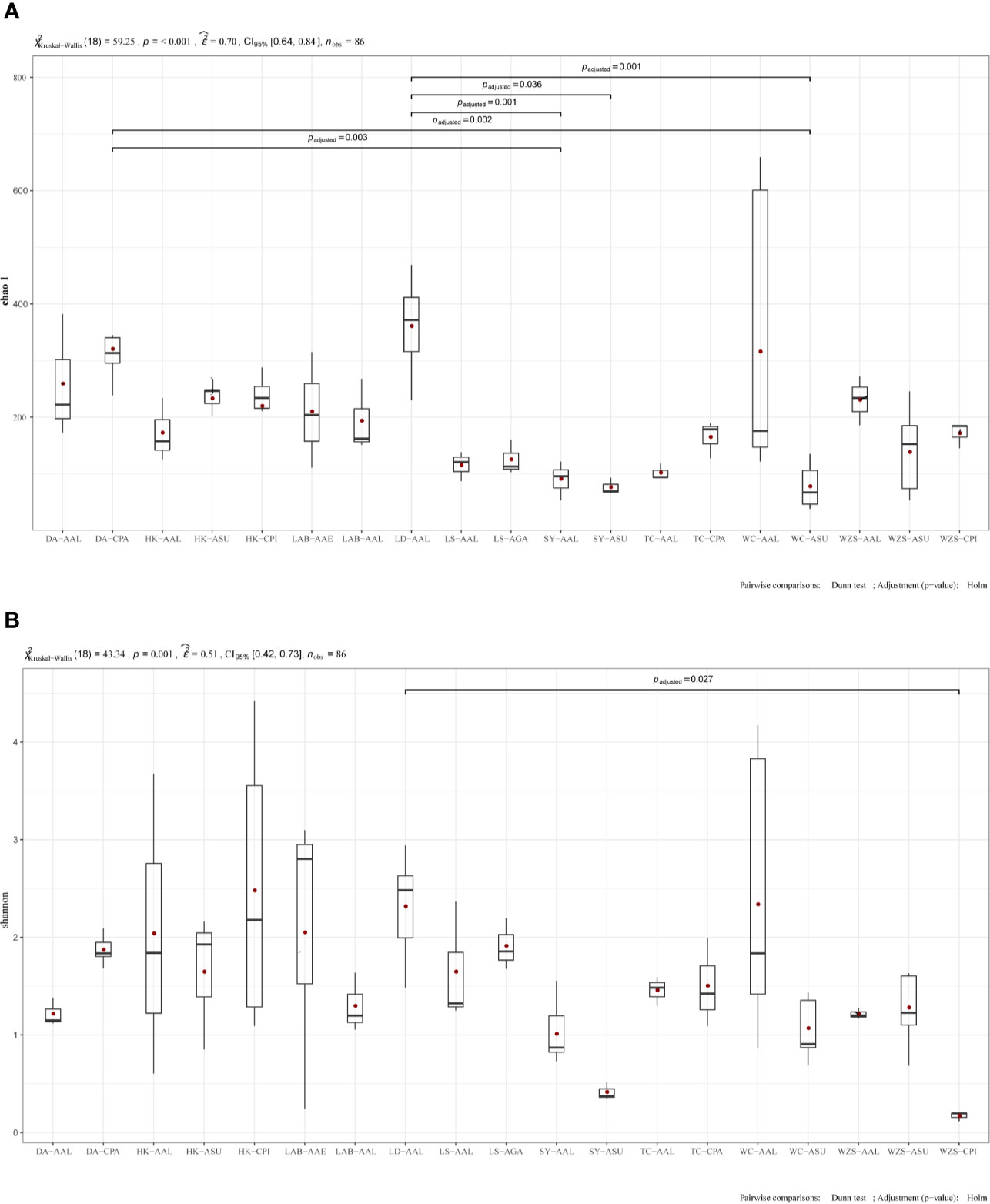
Figure 5 Boxplot representation of observed species. Boxplots show distribution of bacteria between mosquito samples categorized under different locations and mosquito species. Boxplot representation of chao1 (A) and Shannon diversity (B). Significant differences between the groups were investigated by Pairwise comparisons of means: Dunn test; Adjustment (p−value): Holm. Species richness is represented by the number of bands. Box plots show median value and minimum and maximum values. Black lines indicate medians and the value is shown in the figure. Values from all statistical tests are available in additional Table 2.
Variation of Midgut Bacterial Communities Across Mosquito Species
We used several different distance metrics to assess differences in bacterial community profiles between species and geographical locations. The QIIME analysis based on Bray-Curtis distances revealed a significant difference in microbial communities among the six mosquito species and eight locations. To visualize the results, a PCoA plot, based on an unweighted Unifrac distance matrix, was used to depict the differences in the composition of the gut microbiota from different locations.
Three PCoA coordinates using unweighted Unifrac (uwU) distance percent variation explained PCo1 - 26.75%, PCo2 - 12.20%, PCo3 - 10.41% of the total variation, respectively (Figure S2). Significant differences were found in midgut bacterial β-diversity among communities from different localities. For instance, Cx. pipiens from Wuzhishan and Haikou (Figure S2 A); Ae. albopictus from Wenchang and Wuzhishan (Figure S2 B); Ae. albopictus from Ledong and Ar. subalbatus from Sanya were not tightly clustered (Figure S2C). No significant difference was found among the bacterial communities of the same localities for the midgut of different mosquitoes (Figure S2A). These differences and similarities were mainly due to the presence or absence of unclassified taxa and geographical location, respectively.
A diversity matrix heatmap was generated based on Bray-Curtis distances (Figure 6A) and Weighted unifrac β-diversity (Figure 6B). Ordination based on this matrix heatmap showed not only clear separation but also clustering of the samples, which reflects both the diversity and the similarity between the gut microbiota. The R statistics ranged from 0.00 to 1.00. The closer the value is to 1, the greater the difference between samples. Values closer to 0, indicate more similar samples. Bonferroni correction for multiple comparisons revealed 19 significant pairwise comparisons (Figure 6). The community structure of microbiota of Cx. pipiens collected from Wuzhishan was substantially different from that of Cx. pipiens from Haikou (0.979), Ae. galloisi from Lingshui (0.994), Ae. albopictus from Ledong (0.986), Lingshui (0.994), Sanya (0.997) and Wenchang (0.984) (Figure 6A; Table S4). The community structure of microbiota of Ae. albopictus from Tunchang was substantially different from that of Ae. albopictus from Haikou (0.989), Cx. pallidothorax from Dingan (0.997) and Wenchang (0.996), Ar. Subalbatus from Sanya (0.988) and Wenchang (0.996) (Figure 6A; Table S4). A similar pattern of microbiota was observed between Ae. albopictus from Sanya and Ae. albopictus from Lingshui (0.100) and Ar. subalbatus from Sanya (0.093). In addition, Ar. subalbatus from Sanya, Ae. albopictus from Tunchang and Cx. gelidus from Haikou had a similar pattern of microbiota compared to Ae. albopictus from Haikou (0.202), Ae. albopictus from Lingshui (0.174) and Cx. pipiens from Wuzhishan (0.145). There was little separation between the microbiota of Ae. albopictus from Sanya and Dingan (0.0659) (Figure 6B; Table S4).
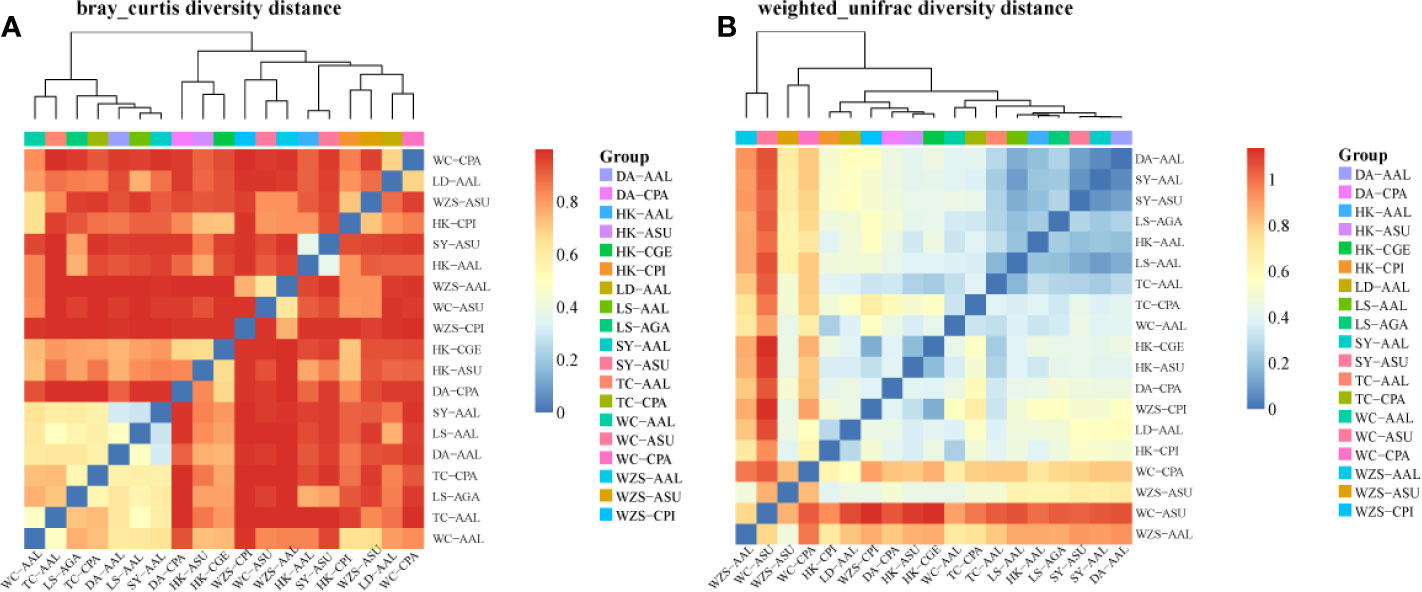
Figure 6 Matrix heatmap of Bray-Curtis distances (A) and Weighted unifrac Beta diversity (B) between microbial communities of six mosquito species from eight areas.
Discussion
Substantial interactions can occur between resident or introduced arthropods and invasive pathogens. The midgut bacteria of mosquitoes play an important role in vector parasite interactions (Dong et al., 2009). Many studies have reported microbial diversity in insect guts but few studies have reported the microbial populations associated with mosquito midguts in Hainan. We conducted microbial inventories of different species of mosquitoes by sequencing the 16S rRNA gene, and we systematically analyzed bacteria from different locations by evaluating 16S rRNA contents. These findings add to the limited knowledge of the microbiota of different mosquito species and show how geographical location or host habitat can influence the composition and diversity of mosquito microbiota.
We reported the diversity of midgut bacteria of six species of mosquito. These were Ae. albopictus, Cx. pipiens, Cx. gelidus, Ar. subalbatus, Cx. pallidothorax, Ae. galloisi (Table 1) collected from eight sites (Figure 1) in Hainan, including urban areas (Haikou, Sanya and Wenchang), rural areas (Ledong and Dingan), farms (Tunchang), and virgin forests (Lingshui and Wuzhishan). We characterized and compared the midgut bacterial communities, many of which are vectors of medical, veterinary, and wildlife significance. Ae. albopictus and Ar. subalbatus were the dominant species in the eight sites on Hainan Island. Ae. albopictus is a vector of the dengue virus and chikungunya virus (Zouache et al., 2012). Ar. subalbatus is a vector of the filarial worm Brugia pahangi (Aliota et al., 2010). There were few consistent differences in the composition of gut microbiota among the different mosquito species. There was great intraspecific variation in the bacterial taxa present indicated by the dominance of one to three bacterial OTUs.
The bacterial community in mosquito midguts was dominated by five main phyla, and the microbial communities in the mosquito midguts were similar at family and genus levels across eight locations. These five bacterial phyla are commonly reported in the guts of mosquitoes and other insects (Zouache et al., 2012). The Proteobacteria are highly diverse and contain a variety of species that are adapted to a wide range of environments. Its dominance in mosquito midguts is well established (Muturi et al., 2016b; Strand, 2018). However, Proteobacteria occurred in low abundance in Ae. albopictus from Wenchang and Ar. Subalbatus from Wuzhishan. The percentage of Actinobacteria was significantly lower (1.58%) than in a previous study (Muturi et al., 2017; Oliveira et al., 2018).
The functions of the major bacterial genera identified in this study are unclear. Wolbachia (Rickettsiaceae) are intracellular and maternally inherited micro-organisms widespread in arthropods (Muturi et al., 2016c). They induce several reproductive disorders such as cytoplasmic incompatibility in their hosts, parthenogenesis and feminization to facilitate their spread into host populations (Blagrove et al., 2012). Studies on mosquito endosymbionts showed that Wolbachia (Rickettsiaceae) occur in most populations of the Cx. pipiens species complex. This complex consists of Cx. pipiens and Cx. quinquefasciatus (Diptera: Culicidae) (Muturi et al., 2016c). In Cx. pipiens, Wolbachia causes partial or complete cytoplasmic incompatibility (CI) between males and females infected by incompatible strains (Sinkins et al., 2005). It confers a relative fitness advantage to infected females allowing Wolbachia to rapidly invade host populations (Turelli and Hoffmann, 1991). Wolbachia (Rickettsiaceae) was detected in the mosquito samples in our study and was one of the dominant bacterial taxa identified in Ar. Subalbatus (93.81%) and Ae. albopictus (84.34%) from Sanya and Ae. albopictus (84.69%) from Dingan. It is unclear why Wolbachia was very low in Cx. pallidothorax (0.031% and 0.053%) from Dingan and Wenchang and Cx. pipiens (0.0057%) from Wuzhishan. At the genus level, especially in Ae. albopictus and Cx. pipiens mosquitoes from Wuzhishan, the unclassified taxa accounted for 60.30% and 99.14% (Figure 2 and S1; Table S3). This might be due to variability in physiological conditions of individual mosquitoes or on habitat environmental conditions. Otherwise, most bacterial OTUs were sparsely distributed among individuals of the different mosquito species. This might be due to inter-individual variations in diet or genetic factors (Wang et al., 2011; Osei-Poku et al., 2012). These variations may indicate population and species level variation in vector competence. Certain bacterial species can increase (Apte-Deshpande et al., 2012) or reduce (Ramirez et al., 2012) vector susceptibility to pathogens.
There were differences in bacterial diversity and evenness between the mosquito species studied. The richness data (Figure 5A; Table 2) show that the Ae. albopictus from Ledong were significantly diverse and distributed compared to Ae. albopictus from Sanya. Significant differences in bacterial diversity and evenness between populations of the same mosquito species across different locations suggest that the sampling site environment is a key determinant of the bacterial profiles in mosquito guts. However, similar bacterial diversity and evenness between mosquito species across the four genera suggests that the mosquito midgut plays an important role in regulating the colonization and assembly of bacterial communities.
The β-diversity results showed that bacterial communities of mosquitoes vary geographically. The Bray-Curtis distances and the Weighted unifrac Beta diversity (Figures 6A, B) show that this variation in some mosquitoes resulted from the presence or absence of rare members, as well as some dominant genera. Cx. pipiens from Wuzhishan and Ae. albopictus from Tunchang were substantially different from other sample locations. In contrast, there was little separation between the microbiota of Ae. albopictus from Sanya and Dingan (Figure 6A; Table S4). Some members of the intraspecific core bacteriome of these mosquitoes were not the most abundant within the community, and their relative abundance varied among locations. A similar pattern of β-diversity variation, provided by the less-frequent members, was observed in another insect, include the pine weevil, bark beetles, brown planthopper and Haemaphysalis longicornis (Durand et al., 2015; Berasategui et al., 2016; Zhang et al., 2018; Zhang et al., 2019).
Midgut microbial composition and diversity are acquired through vertical inheritance and from the surrounding environment (Minard et al., 2013; Buck et al., 2016). These sources include food sources (e.g., nectar and blood meal), weather, and population growth. The environment of the sampling site is a key determinant of the bacteria that colonize the mosquitoes (Hansen et al., 2012). When the physiological conditions in the mosquito gut change, the pathogenic or commensal capacities of some bacteria may be influenced (Seaman et al., 2015; Short et al., 2017). Differences in food sources (Berasategui et al., 2016) may affect bacterial differences.
In summary, we characterized the midgut bacterial communities of six mosquito species in Hainan province, China. Proteobacteria and Wolbachia (Rickettsiaceae) were the major members of bacterial communities associated with most of the mosquito species. There were significant differences in the gut microbial composition between some species and substantial variation in the gut microbiota between individuals of the same mosquito species. There was a marked variation in different mosquito gut microbiota within the same location. These results might be useful in the identification of microbial communities that could be exploited for disease control.
Data Availability Statement
The datasets presented in this study can be found in online repositories. The names of the repository/repositories and accession number(s) can be found below: https://datadryad.org/stash, https://datadryad.org/stash/share/VZY6nQMlADnSMgkzQ8iLuA2PtkPFooCFwzHBO8umhNA.
Author Contributions
Conceptualization: LK. Data curation: YW, ZZ, QX. Formal analysis: ZZ, QX, AZ. Investigation: XK, YW, SL, YL, XS, XL. Methodology: XK, YW. Supervision: ZZ, QX. Writing original draft: XK, YW, MR, ZZ, QX. All authors contributed to the article and approved the submitted version.
Funding
This work was supported by the National Key Plan for Scientific Research and Development of China (2019YFC1200504), the National Science and Technology Major Project (2018ZX10101004), and the National Natural Science Foundation of China (81560002, 81960002), Key Laboratory of Vector Biology and Pathogen Control of Zhejiang Province, Huzhou University (HUZUL201901).
Conflict of Interest
The authors declare that the research was conducted in the absence of any commercial or financial relationships that could be construed as a potential conflict of interest.
Acknowledgments
We thank Manage station staff and Chengli Liu who made possible and contributed to sampling in various cities and counties in Hainan. We are grateful to research staff from Institute of Zoology, Chinese Academy of Sciences for their help during sampling in Hainan. We thank MR who provided insightful comments and English revision of the manuscript. We thank the anonymous referees who contributed to the manuscript revision.
Supplementary Material
The Supplementary Material for this article can be found online at: https://www.frontiersin.org/articles/10.3389/fcimb.2020.596750/full#supplementary-material
Supplementary Figure 1 | Relative abundance of the top 43 bacterial genera in all samples from different study sites. DA = Dingan, HK = Haikou, LD = Ledong, LS = Ledong, SY = Sanya, TC = Tunchang, WC = Wenchang, WZS = Wuzhishan; AAL = Aedes albopictus, ASU = Armigeres subalbatus, CGE = Culex gelidus, AGA = Aedes galloisi, CPA = Culex pallidothorax, CPI = Culex pipiens.
Supplementary Figure 2 | PCoA plot (A–C) based on an unweighted Unifrac distance matrix depicting differences in the composition of the gut microbiota from different locations. Colors represent community profiles of individual samples and location.
References
Aliota M. T., Fuchs J. F., Rocheleau T. A., Clark A. K., Hillyer J. F., Chen C. C., et al. (2010). Mosquito transcriptome profiles and filarial worm susceptibility in Armigeres subalbatus. PloS Negl. Trop. Dis. 4, e666. doi: 10.1371/journal.pntd.0000666
Fauci M. D., Morens M. D. (2016). Zika Virus in the Americas — Yet Another Arbovirus Threat. J Med 347, 601–4. doi: 10.1056/nejmp1600297
Apte-Deshpande A., Paingankar M., Gokhale M. D., Deobagkar D. N. (2012). Serratia odorifera a midgut inhabitant of Aedes aegypti mosquito enhances its susceptibility to dengue-2 virus. PloS One 7, e40401. doi: 10.1371/journal.pone.0040401
Ashfaq M., Hebert P. D., Mirza J. H., Khan A. M., Zafar Y., Mirza M. S. (2014). Analyzing mosquito (Diptera: culicidae) diversity in Pakistan by DNA barcoding. PloS One 9, e97268. doi: 10.1371/journal.pone.0097268
Baia-da-Silva D. C., Orfano A. S., Nacif-Pimenta R., de Melo F. F., Simoes S., Cabral I., et al (2019). The Midgut Muscle Network of Anopheles aquasalis (Culicidae, Anophelinae): Microanatomy and Structural Modification After Blood Meal and Plasmodium vivax (Haemosporida, Plasmodiidae) Infection. J. Med. Entomol 56, 421–431. doi: 10.1093/jme/tjy199
Barrett A. D., Higgs S. (2007). Yellow fever: a disease that has yet to be conquered. Annu. Rev. Entomol 52, 209–229. doi: 10.1146/annurev.ento.52.110405.091454
Berasategui A., Axelsson K., Nordlander G., Schmidt A., Borg-Karlson A. K., Gershenzon J., et al. (2016). The gut microbiota of the pine weevil is similar across Europe and resembles that of other conifer-feeding beetles. Mol. Ecol. 25, 4014–4031. doi: 10.1111/mec.13702
Blagrove M. S., Arias-Goeta C., Failloux A. B., Sinkins S. P. (2012). Wolbachia strain wMel induces cytoplasmic incompatibility and blocks dengue transmission in Aedes albopictus. Proc. Natl. Acad. Sci. U.S.A. 109, 255–260. doi: 10.1073/pnas.1112021108
Buck M., Nilsson L. K., Brunius C., Dabire R. K., Hopkins R., Terenius O. (2016). Bacterial associations reveal spatial population dynamics in Anopheles gambiae mosquitoes. Sci. Rep. 6, 22806. doi: 10.1038/srep22806
Caporaso J.G., Kuczynski J., Stombaugh J., Bittinger K., Ushman F. D., Costello E. K., et al. (2010). QIIME allows analysis of highthroughput community sequencing data. Nat. Methods 7, 335–336. doi: 10.1038/nmeth.f.303
Dong Y., Manfredini F., Dimopoulos G. (2009). Implication of the mosquito midgut microbiota in the defense against malaria parasites. PloS Pathog 5, e1000423. doi: 10.1371/journal.ppat.1000423
Durand A. A., Bergeron A., Constant P., Buffet J. P., Deziel E., Guertin C. (2015). Surveying the endomicrobiome and ectomicrobiome of bark beetles: The case of Dendroctonus simplex. Sci. Rep. 5, 17190. doi: 10.1038/srep17190
Edgar R. C., Haas B. J., Clemente J. C., Quince C., Knight R. (2011). UCHIME improves sensitivity and speed of chimera detection. Bioinformatics 27, 2194–2200. doi: 10.1093/bioinformatics/btr381
Edgar R. C. (2013). UPARSE: highly accurate OTU sequences from microbial amplicon reads. Nat. Methods 10, 996–998. doi: 10.1038/nmeth.2604
Fauci A. S., Morens D. M. (2016). Zika Virus in the Americas — Yet Another Arbovirus Threat. N. Engl. J Med. 347, 601–604. doi: 10.1056/nejmp1600297
Gandotra S., Kumar A., Naga K., Bhuyan P. M., Gogoi D. K., Sharma K., et al. (2018). Bacterial community structure and diversity in the gut of the muga silkworm, Antheraea assamensis (Lepidoptera: Saturniidae), from India. Insect Mol. Biol. 27, 603–619. doi: 10.1111/imb.12495
Hansen I. A., Marcombe S., Mathieu R. B., Pocquet N., Riaz M.-A., Poupardin R., et al. (2012). Insecticide Resistance in the Dengue Vector Aedes aegypti from Martinique: Distribution, Mechanisms and Relations with Environmental Factors. PloS One 7 (2), e30989. doi: 10.1371/journal.pone.0030989
Hernandez-Garcia J. A., Gonzalez-Escobedo R., Briones-Roblero C. I., Cano-Ramirez C., Rivera-Orduna F. N., Zuniga G. (2018). Gut Bacterial Communities of Dendroctonus valens and D. mexicanus (Curculionidae: Scolytinae): A Metagenomic Analysis across Different Geographical Locations in Mexico. Int. J. Mol. Sci. 19 (9), 2578. doi: 10.3390/ijms19092578
Huber W., Carey V. J., Gentleman R., Anders S., Carlson M., Carvalho B. S., et al. (2015). Orchestrating high-throughput genomic analysis with Bioconductor. Nat. Methods 12, 115–121. doi: 10.1038/nmeth.3252
Krajacich B. J., Huestis D. L., Dao A., Yaro A. S., Diallo M., Krishna A., et al. (2018). Investigation of the seasonal microbiome of Anopheles coluzzii mosquitoes in Mali. PloS One 13, e0194899. doi: 10.1371/journal.pone.0194899
Kumar N. P., Rajavel A. R., Natarajan R., Jambulingam P. (2007). DNA Barcodes Can Distinguish Species of Indian Mosquitoes (Diptera: Culicidae). J. Med. Entomol 44, 01–07. doi: 10.1093/jmedent/41.5.01
Li Y., Xu J., Zhong D., Zhang H., Yang W., Zhou G., et al. (2018). Evidence for multiple-insecticide resistance in urban Aedes albopictus populations in southern China. Parasit Vectors 11, 4. doi: 10.1186/s13071-017-2581-y
Li R., Xu L., Bjornstad O. N., Liu K., Song T., Chen A., et al. (2019). Climate-driven variation in mosquito density predicts the spatiotemporal dynamics of dengue. Proc. Natl. Acad. Sci. U.S.A. 116, 3624–3629. doi: 10.1073/pnas.1806094116
Lozupone C., Lladser M. E., Knights D., Stombaugh J., Knight R. (2010). UniFrac: an effective distance metric for microbial community comparison. ISME J. 5, 169–172. doi: 10.1038/ismej.2010.133
Magoc T., Salzberg S. L. (2011). FLASH: fast length adjustment of short reads to improve genome assemblies. Bioinformatics 27, 2957–2963. doi: 10.1093/bioinformatics/btr507
Minard G., Mavingui P., Moro C. V. (2013). Diversity and function of bacterial microbiota in the mosquito holobiont. Parasites Vectors 6, 146. doi: 10.1186/1756-3305-6-146
Mohammadi Shahrestani M., Marcussen T., Saeidi Mehrvarz S., Yousefi N. (2015). Morphological and phylogenetic relationships within Viola sect. Sclerosium (Violaceae) in Iran. Flora Morphol Distribution Funct. Ecol. Plants 215, 67–74. doi: 10.1016/j.flora.2015.07.008
Muturi E. J., Bara J. J., Rooney A. P., Hansen A. K. (2016a). Midgut fungal and bacterial microbiota of Aedes triseriatus and Aedes japonicus shift in response to La Crosse virus infection. Mol. Ecol. 25, 4075–4090. doi: 10.1111/mec.13741
Muturi E. J., Bara J. J., Rooney A. P., Hansen A. K. (2016b). Mosquitoes rely on their gut microbiota for development. Mol. Ecol. 25, 4075–4090. doi: 10.1111/mec.13741
Muturi E. J., Kim C. H., Bara J., Bach E. M., Siddappaji M. H. (2016c). Culex pipiens and Culex restuans mosquitoes harbor distinct microbiota dominated by few bacterial taxa. Parasit Vectors 9, 18. doi: 10.1186/s13071-016-1299-6
Muturi E. J., Ramirez J. L., Rooney A. P., Kim C. H. (2017). Comparative analysis of gut microbiota of mosquito communities in central Illinois. PloS Negl. Trop. Dis. 11, e0005377. doi: 10.1371/journal.pntd.0005377
Navas-Molina J. A., Peralta-Sanchez J. M., Gonzalez A., McMurdie P. J., Vazquez-Baeza Y., Xu Z., et al. (2013). Advancing our understanding of the human microbiome using QIIME. Methods Enzymol. 531, 371–444. doi: 10.1016/B978-0-12-407863-5.00019-8
Oliveira J. L., Cury J. C., Gurgel-Goncalves R., Bahia A. C., Monteiro F. A. (2018). Field-collected Triatoma sordida from central Brazil display high microbiota diversity that varies with regard to developmental stage and intestinal segmentation. PloS Negl. Trop. Dis. 12, e0006709. doi: 10.1371/journal.pntd.0006709
Osei-Poku J., Mbogo C. M., Palmer W. J., Jiggins F. M. (2012). Deep sequencing reveals extensive variation in the gut microbiota of wild mosquitoes from Kenya. Mol. Ecol. 21, 5138–5150. doi: 10.1111/j.1365-294X.2012.05759.x
Ramirez J. L., Souza-Neto J., Torres Cosme R., Rovira J., Ortiz A., Pascale J. M., et al. (2012). Reciprocal tripartite interactions between the Aedes aegypti midgut microbiota, innate immune system and dengue virus influences vector competence. PloS Negl. Trop. Dis. 6, e1561. doi: 10.1371/journal.pntd.0001561
Rezza G., Chen R., Weaver S. C. (2017). O’nyong-nyong fever: a neglected mosquito-borne viral disease. Pathog Glob Health 111, 271–275. doi: 10.1080/20477724.2017.1355431
Ruiling Z., Peien L., Xuejun W., Zhong Z. (2018). Molecular analysis and genetic diversity of Aedes albopictus (Diptera, Culicidae) from China. Mitochondrial DNA A DNA Mapp Seq Anal. 29, 594–599. doi: 10.1080/24701394.2017.1325481
Schloss P. D., Westcott S. L., Ryabin T., Hall J. R., Hartmann M., Hollister E. B., et al. (2009). Introducing mothur: open-source, platform-independent, community-supported software for describing and comparing microbial communities. Appl. Environ. Microbiol 75, 7537–7541. doi: 10.1128/AEM.01541-09
Seaman J. A., Alout H., Meyers J. I., Stenglein M. D., Dabire R. K., Lozano-Fuentes S., et al. (2015). Age and prior blood feeding of Anopheles gambiae influences their susceptibility and gene expression patterns to ivermectin-containing blood meals. BMC Genomics 16, 797. doi: 10.1186/s12864-015-2029-8
Short S. M., Mongodin E. F., MacLeod H. J., Talyuli O. A. C., Dimopoulos G. (2017). Amino acid metabolic signaling influences Aedes aegypti midgut microbiome variability. PloS Negl. Trop. Dis. 11, e0005677. doi: 10.1371/journal.pntd.0005677
Sim S., Jupatanakul N., Dimopoulos G. (2014). Mosquito immunity against arboviruses. Viruses 6, 4479–4504. doi: 10.3390/v6114479
Sinkins S. P., Walker T., Lynd A. R., Steven A. R., Makepeace B. L., Godfray H. C., et al. (2005). Wolbachia variability and host effects on crossing type in Culex mosquitoes. Nature 436, 257–260. doi: 10.1038/nature03629
Steyn A., Roets F., Botha A. (2016). Yeasts Associated with Culex pipiens and Culex theileri Mosquito Larvae and the Effect of Selected Yeast Strains on the Ontogeny of Culex pipiens. Microb Ecol. 71, 747–760. doi: 10.1007/s00248-015-0709-1
Strand M. R. (2018). Composition and functional roles of the gut microbiota in mosquitoes. Curr. Opin. Insect Sci. 28, 59–65. doi: 10.1016/j.cois.2018.05.008
Sun D. W., Wang G. Z., Zeng L. H., Li S. G., He C. H., Hu X. M., et al. (2017). Extensive Resistance of Anopheles sinensis to Insecticides in Malaria-Endemic Areas of Hainan Province, China. Am. J. Trop. Med. Hyg 97, 295–298. doi: 10.4269/ajtmh.16-0723
Tsetsarkin K. A., Chen R., Weaver S. C. (2016). Interspecies transmission and chikungunya virus emergence. Curr. Opin. Virol 16, 143–150. doi: 10.1016/j.coviro.2016.02.007
Turelli M., Hoffmann A. A. (1991). Rapid spread of an inherited incompatibility factor in California Drosophila. Nature 353, 440–442. doi: 10.1038/353440a0
Wang Q., Garrity G. M., Tiedje J. M., Cole J. R. (2007). Naive Bayesian classifier for rapid assignment of rRNA sequences into the new bacterial taxonomy. Appl. Environ. Microbiol 73, 5261–5267. doi: 10.1128/AEM.00062-07
Wang Y., Gilbreath T. M. 3., Kukutla P., Yan G., Xu J. (2011). Dynamic gut microbiome across life history of the malaria mosquito Anopheles gambiae in Kenya. PloS One 6, e24767. doi: 10.1371/journal.pone.0024767
Wang S., Ghosh A. K., Bongio N., Stebbings K. A., Lampe D. J., Jacobs-Lorena M. (2012). Fighting malaria with engineered symbiotic bacteria from vector mosquitoes. Proc. Natl. Acad. Sci. U.S.A. 109, 12734–12739. doi: 10.1073/pnas.1204158109
Wang S. Q., Li Y. C., Zhang Z. M., Wang G. Z., Hu X. M., Qualls W. A., et al. (2014). Prevention measures and socio-economic development result in a decrease in malaria in Hainan, China. Malar J. 13, 362. doi: 10.1186/1475-2875-13-362
Wang X., Hou Y., Saha T. T., Pei G., Raikhel A. S., Zou Z. (2017). Hormone and receptor interplay in the regulation of mosquito lipid metabolism. Proc. Natl. Acad. Sci. U.S.A. 114, E2709–E2718. doi: 10.1073/pnas.1619326114
Wang J. M., Cheng Y., Shi Z. K., Li X. F., Xing L. S., Jiang H., et al. (2019). Aedes aegypti HPX8C modulates immune responses against viral infection. PloS Negl. Trop. Dis. 13, e0007287. doi: 10.1371/journal.pntd.0007287
Wang S., Jacobs-Lorena M. (2013). Genetic approaches to interfere with malaria transmission by vector mosquitoes. Trends Biotechnol. 31, 185–193. doi: 10.1016/j.tibtech.2013.01.001
Weaver S. C., Costa F., Garcia-Blanco M. A., Ko A. I., Ribeiro G. S., Saade G., et al. (2016). Zika virus: History, emergence, biology, and prospects for control. Antiviral Res. 130, 69–80. doi: 10.1016/j.antiviral.2016.03.010
White J. R., Nagarajan N., Pop M. (2009). Statistical methods for detecting differentially abundant features in clinical metagenomic samples. PloS Comput. Biol. 5, e1000352. doi: 10.1371/journal.pcbi.1000352
Wilke A. B., Christe Rde O., Multini L. C., Vidal P. O., Wilk-da-Silva R., de Carvalho G. C., et al. (2016). Morphometric Wing Characters as a Tool for Mosquito Identification. PloS One 11, e0161643. doi: 10.1371/journal.pone.0161643
Wu X., Zhang H., Chen J., Shang S., Wei Q., Yan J., et al. (2016). Comparison of the fecal microbiota of dholes high-throughput Illumina sequencing of the V3-V4 region of the 16S rRNA gene. Appl. Microbiol Biotechnol. 100, 3577–3586. doi: 10.1007/s00253-015-7257-y
Yasri S., Wiwanitkit V. (2017). Non-human Hosts and Zika Virus Maintenance. Ecohealth 14, 200–202. doi: 10.1007/s10393-017-1246-9
Zhang J. H., Yu N., Xu X. X., Liu Z. W. (2018). Community structure, dispersal ability and functional profiling of microbiome existing in fat body and ovary of the brown planthopper, Nilaparvata lugens. Insect Sci 26, 683–694. doi: 10.1111/1744-7917.12575
Zhang R., Huang Z., Yu G., Zhang Z. (2019). Characterization of microbiota diversity of field-collected Haemaphysalis longicornis (Acari: Ixodidae) with regard to sex and blood meals. J. Basic Microbiol 59, 215–223. doi: 10.1002/jobm.201800372
Keywords: Hainan, mosquitoes, midgut microbiome, bacterial community, 16S rRNA
Citation: Kang X, Wang Y, Li S, Sun X, Lu X, Rajaofera MJN, Lu Y, Kang L, Zheng A, Zou Z and Xia Q (2020) Comparative Analysis of the Gut Microbiota of Adult Mosquitoes From Eight Locations in Hainan, China. Front. Cell. Infect. Microbiol. 10:596750. doi: 10.3389/fcimb.2020.596750
Received: 20 August 2020; Accepted: 17 November 2020;
Published: 15 December 2020.
Edited by:
Michail Kotsyfakis, Academy of Sciences of the Czech Republic (ASCR), CzechiaReviewed by:
Gaoqian Feng, Burnet Institute, AustraliaChiranjib Pal, West Bengal State University, India
Mathilde Gendrin, Institut Pasteur de la Guyane, French Guiana
Copyright © 2020 Kang, Wang, Li, Sun, Lu, Rajaofera, Lu, Kang, Zheng, Zou and Xia. This is an open-access article distributed under the terms of the Creative Commons Attribution License (CC BY). The use, distribution or reproduction in other forums is permitted, provided the original author(s) and the copyright owner(s) are credited and that the original publication in this journal is cited, in accordance with accepted academic practice. No use, distribution or reproduction is permitted which does not comply with these terms.
*Correspondence: Zhen Zou, em91emhlbkBpb3ouYWMuY24=; Qianfeng Xia, eGlhcWlhbmZlbmdAaGFpbm1jLmVkdS5jbg==
†These authors have contributed equally to this work