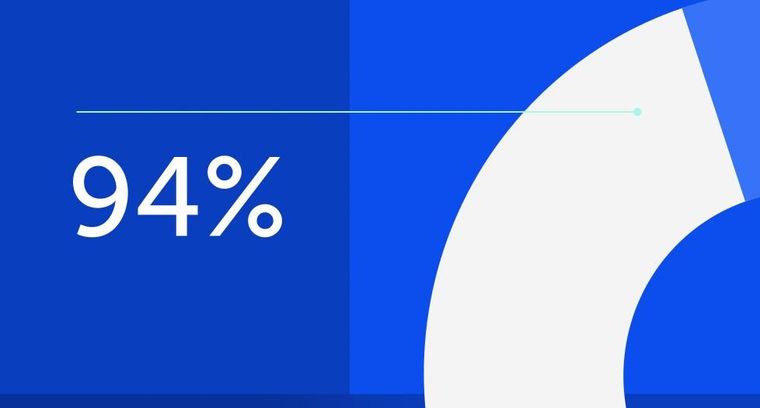
94% of researchers rate our articles as excellent or good
Learn more about the work of our research integrity team to safeguard the quality of each article we publish.
Find out more
REVIEW article
Front. Cell. Infect. Microbiol., 28 October 2020
Sec. Clinical Microbiology
Volume 10 - 2020 | https://doi.org/10.3389/fcimb.2020.560240
This article is part of the Research TopicCoronavirus Disease (COVID-19): Pathophysiology, Epidemiology, Clinical Management and Public Health ResponseView all 400 articles
Understanding the systems biology approaches for promoting the development of new therapeutic drugs is attaining importance nowadays. The threat of COVID-19 outbreak needs to be vanished for global welfare, and every section of research is focusing on it. There is an opportunity for finding new, quick, and accurate tools for developing treatment options, including the vaccine against COVID-19. The review at this moment covers various aspects of pathogenesis and host factors for exploring the virus target and developing suitable therapeutic solutions through systems biology tools. Furthermore, this review also covers the extensive details of multiomics tools i.e., transcriptomics, proteomics, genomics, lipidomics, immunomics, and in silico computational modeling aiming towards the study of host–virus interactions in search of therapeutic targets against the COVID-19.
COVID-19 (coronavirus disease 2019) outbreak is caused by an animal virus belonging to the family Coronaviridae (Ahmed et al., 2020; Cascella et al., 2020). This animal virus is transmitted to humans and causes severe respiratory syndromes (Mohd et al., 2016; Fung et al., 2020). The associated syndromes are Middle East respiratory syndrome (MERS) (Wernery et al., 2017), severe acute respiratory syndrome (SARS) (Lai et al., 2020), acute respiratory distress syndrome (ARDS) (Wu et al., 2020b), and most recently coronavirus disease (COVID-19). With the outbreaks related to the above mentioned coronavirus related syndromes, it is evident that human pathogenic coronavirus related mutants and strains occur and emerge from infected animal livestock during the past decade (Deng and Peng, 2020). In the present situation, COVID-19 has been spread globally (Khachfe et al., 2020). This crisis started in China in December 2019. The Wuhan market (China) was associated with animals and their meat products for domestic cooking purposes. The consumption of coronavirus infected meat products by nearby local people is the starting point of the pandemic (Schwartz and Graham, 2020). Within one month approximately, 9,066 positive coronavirus infected cases were found, and 213 patients died till January 2020 (Riou and Althaus, 2020). Moreover, the cases increased continuously at multiple rates around the world, leading to a global health emergency. The coronavirus outbreak has proven a threat to humanity. The coronavirus associated with COVID-19 shows 75–80% similarity with the severe acute respiratory syndrome coronavirus (SARS-CoV) and is more directly connected to numerous bat coronavirus. Unlike other coronaviruses, COVID-19 grows better in epithelial cells of human airway rather than in the cultured cells in the laboratory. It uses human angiotensin-converting enzyme 2 as its cellular receptor, so the infection is transmitted only after the infection of the lower respiratory tract (Perlman, 2020). The novel coronavirus causes severe respiratory disease, COVID-19. The patients suffer from pneumonia. They develop a cold, dry cough and a sudden rise in body temperature (Zhu et al., 2020). Human to human transmission of COVID-19 occurs through respiratory droplets of the sneezed particles or from the close contact of an infected person (Chen, 2020). The WHO (World Health Organization) is currently engaged in managing the pandemic situation with nations around the world by releasing guidelines for health workers. For the novel coronavirus 2019, the unavailability of vaccine tenders the importance of antiviral drugs and therapeutics for pandemic control programs and preventive measures in pandemic reoccurrence (Sohrabi et al., 2020). The current outbreak can be controlled by maintaining social distance and reducing the person to person transmission. The immediate step required to control disease (Archana et al., 2015) outbreak includes isolation, early diagnosis, and other supportive treatment (Czernin et al., 2020). It can also be preventive by maintaining personal hygiene, avoiding crowded places, wearing of fitted masks, and ventilation. Special measures should be taken for the children, old age, and immuno-compromised people as they are more prone to COVID-19 (Marchand-Senécal et al., 2020). The therapeutic drugs available to clinical workers for the treatment of coronavirus infections are only as a temporary option. This new demand gives the opportunity for researchers to save humankind from this menace. The SARS viruses are difficult and quite dangerous to handle in vivo, but the information of their genes, proteins, or the RNA acquired by sequencing is simple and easy to handle through artificial intelligence. The expectation from systems biology for therapeutic agent development is mentioned. Furthermore, the role of different components of multiomics is discussed for virulence assessment of coronavirus. Along with the importance of artificial intelligence in generating data for drug development and the requisite of data mining from the database, the in silico appeal for host–virus interaction study (viz. protein–protein interaction, computational modeling) and vaccine development are also described. Molecular docking studies have been used for the detection of medications to inhibit SARS-CoV-2 spike protein and protease enzyme in the past. Thus, molecular docking can pave the way for computational drug designing, which can further be utilized for the treatment of COVID-19 (Hall and Ji, 2020).
Coronavirus is an enveloped and single-stranded RNA virus. It is classified into four categories: α-coronavirus, β-coronavirus, δ-coronavirus, and γ-coronavirus (Yang and Wang, 2020). Earlier, there were six coronaviruses that infect humans and cause diseases. Despite the SARS-CoV and MERS-CoV, COVID-19 is caused by the seventh member of the coronavirus family to infect human, the novel SARS coronavirus and SARS-CoV share almost 79% genome similarity (Dawood, 2020). Like SARS-CoV and MERS-CoV, COVID-19 is considered in the family of β-coronavirus (Guo et al., 2020). These two (SARS-CoV and SARS-CoV-2) have identical domains for receptor binding and use angiotensin-converting enzyme 2 (ACE2) as the receptor. Significantly, S protein present on the surface is responsible for the identification of the receptors in the target host, facilitating the entry into the host cell (Hofmann and Pöhlmann, 2004). The binding efficiency of SARS-CoV-2 is ten times higher than the SARS-CoV. This shows that ACE2 could be a possible candidate for treatment (Wang X. et al., 2020). The fact that there is less information about the pathogenesis of SARS-CoV-2 and also that systems biology and omics technology cannot cover every specific cellular or physiological process for hindering the virulence strategy of virus are the main limitations. Patients suffering from COVID-19 have symptoms similar to SARS-CoV and MERS-CoV like fever, fatigue, non-productive coughs, myalgia, pneumonia, and decrease leukocyte count (Daga et al., 2019). Metabolic acidosis showing dysfunction of microcirculation was also observed. Additionally, kidney and liver functions were also affected in some patients. The blood and lower respiratory tract specimen cultures turned out to be negative for bacteria and fungus in 76% sepsis patients in a COVID-19 cohort. Therefore, viral sepsis would be more accurate to describe the clinical manifestations of severe or critically ill COVID-19 patients. Understanding the mechanism of viral sepsis in COVID-19 is warranted for exploring better clinical care for these patients (Zhou et al., 2020).
Therefore the pathogenesis mechanism of SAR-CoV and MERS-CoV will help in understanding the pathogenesis of SARS-CoV-2. Significantly spike proteins are determined to facilitate the entry of the virus into the host body (Xia et al., 2020). They bind to the receptor of the cells like ACE2 and CD209L. Initially, it was reported that the virus enters the cell by the fusion of the virus with the plasma membrane. The proteolytic cleavage of spike protein at position S2′ is essential for membrane fusion following viral infection (Belouzard et al., 2009; Walls et al., 2020). While in MERS-CoV, the membrane fusion was initiated by furin activation. Besides, SARS-CoV-2 uses clathrin-dependent and independent endocytosis methods to enter into the cell (Wang et al., 2008). The virus releases RNA genome into the cell to begin the process of replication. The glycoproteins form to facilitate the formation of the nucleocapsid. The germination of the virus particle takes place in an endoplasmic reticulum golgi intermediate compartment (ERGIC) (Risco et al., 2002). Afterward, the virus particle fused to the plasma membrane to release.
After entering into the host cell, the antigen peptides presented by major histocompatibility complex (MHC) and virus-specific cytotoxic T lymphocytes (CTLs) help in the identification. Therefore, the knowledge of antigen presentation of SARS-CoV-2 will significantly assist in interpreting the pathogenesis of COVID-19 (Prompetchara et al., 2020). As there is less information about antigen presentation for COVID-19, so the information behind SARS-CoV and MERS-CoV will significantly help the researchers in planning the methodologies (Yuen et al., 2020). Mainly SARS-CoV involves MHC I molecules for antigen presentation and also susceptible to different HLA (Human Leukocyte Antigen) polymorphisms. Most of the alleles like HLA-A∗0201, HLA-DR0301, and HLA-Cw1502 help in the protection from SARS disease. The alleles, like HLA-DRB1∗11:01 and HLA-DQB1∗02:01 are more sensitive to MERS-CoV disease (Risco et al., 2002). Nguyen et al., 2020 studied the binding efficiency of HLA and mentioned that HLA-B*15:03 is responsible for maximum binding with conserved peptide of SARS CoV2. Additionally Wang W. et al. (2020) applied next generation sequencing method and found that HLA‐C*07:29 and B*15:27 are highly significant in COVID-19 infected patients.
The mannose-binding lectin (MBL) is an important molecule in innate immunity and starts its function before the response of a specific antibody (Ip et al., 2005). People infected with COVID-19 have low level of MBL in their serum as compared to healthy ones. It is observed that MBL is associated with antigen presentation and is also linked with the infection of SARS (Mason and Tarr, 2015). Furthermore, this evidence will be a helping hand in understanding the mechanism of SARS-CoV-2 infection. In comparison with the humoral response, cellular immunity is more significant in the case of coronavirus (Wang F. et al., 2020).
Immune dysfunction such as severe respiratory failure is observed in COVID-19 patients in a case study by Giamarellos-Bourboulis et al. (2020). The macrophage activation syndrome, less human HLA-DR expression along with the reduced number of CD4 lymphocytes, natural killer cells, and CD19 lymphocytes were shown in severe respiratory failure along with sustained production of TNF-α and IL-6 (Magro et al., 2020). The inhibition of HLA-DR expression was performed by plasma of COVID-19 patients, and it could be partially restored via IL-6 blocker. Herein, IL-6 based HLA-DR expression is a characteristic feature and deals with hyper inflammation and cytokine production. Generally SARS-CoV-2 causes hyper inflammation by impairing the host immune response and subsequently dearranging the renin–angiotensin–aldosterone system (Henry et al., 2020a). Acute lung injury and coagulopathy were caused by an imbalance in RAAS (renin–angiotensin–aldosterone system) and hyper inflammation. RAAS is an essential hormone system that performs the function of blood pressure regulation and is also helpful in balancing the fluid within the body. Moreover, it would result into fibrinolysis, immunothrombosis, and multiple organ damage (Henry et al., 2020a). The patients in later stage have deteriorated conditions and die within a short period of time because of organ failure and acute respiratory distress syndrome. All these happen due to cytokine storm and it is significant in increasing the symptoms. Cytokine storm was also validated by clinical studies studying critical patients. Hence, the suppression of cytokine storm is another way to treat COVID-19 infected patients (Ye et al., 2020).
During the 2002–2003 SARS epidemics, the human population got infected from the cross-transmission of civet, raccoon, and Chinese ferret-badger. Initially, the animal handlers got infected from the wet market (Perlman and Netland, 2009). Although they do not have any symptoms of SARS-CoV during detection, their serum gave a high positive result. The infection rate increases when a physician gets infected by treating them, and consequently, the epidemic started. The genetic analysis of the isolated virus reveals its fast rate of adaption in the host cell. It was found in the live animal market and isolated from Rhinolophus spp. Hence the virus is transmitted from bats to mammals and then to humans (He et al., 2014). In civets and humans, the virus gets entry through the ACE2 receptor, which was not observed in the case of the bat. Apart from its wild ruminants, canine and feline were also susceptible to contamination with the same virus (Malik et al., 2020).
The study by sequence data analysis and molecular biology reveals that approximately 60 novel bat coronaviruses were found in Africa, North America, Europe, and China (Hu et al., 2015). This strain probably originated from the same source and got diverted based on adaption in a different host. The coronavirus isolated from Delphinapterus leucas was also categorized in sub-group, infecting mammals. The spike protein of SARS-CoV and new SARS-CoV-2 shares around 76.5% amino acid identity (Zhang et al., 2020). The coronavirus can quickly enter into the host cells via spike protein. The spike protein undergoes cleavage before entering into the target cell. Mostly, SARS-S involves angiotensin-converting enzyme 2 (ACE2) for receptor and also incorporate TMPRSS2, a cellular serine protease that is clinically proved to block the entry of the virus into the cell (Hoffmann et al., 2020). The study about SARS-S/ACE2 elucidated that it contributes an essential role in virus transmission, pathogenesis, and target as a therapeutic agent. SARS-CoV mainly infects pneumocytes and macrophages present in the lungs (Liu J. et al., 2020). Apart from lungs, ACE2 was also expressed in the extra-pulmonary surface. SARS-CoV-2 enters the cell by membrane diffusion and slows down the regulation of ACE2 receptor (Qian et al., 2013; Silhol et al., 2020). TMPRSS2 based entry of SARS-CoV-2 has been observed when cleavage of the S1/S2 site is mediated by furin in the virus-infected cell (Coutard et al., 2020). Thus, it can be deciphered that TMPRSS2 is a vital host factor responsible for COVID-19 spread like coronavirus and influenza A viruses. Iwata-Yoshikawa et al. (2019) reported that TMPRSS2 is a drug target as it contributes to the development of homeostasis. The Camostat mesylate is a serine protease inhibitor that can be sufficient to block the function of TMPRSS2, and Japan approved it for humans (D’Amico et al., 2020). Therefore, the above-discussed compounds possess antiviral activity and were suggested for the treatment of COVID-19. In SARS-CoV, lysosomotropic agents make the infection more severe and sensitive by disturbing endosomal pH (Docea et al., 2020). In SARS-CoV infection, mainly protease treatment nullifies the effect of blocking mediated by lysosomotropic-agent. Cathepsin L, which is an endosomal protease, can also block the SARS-CoV-2 infection (Liu T. et al., 2020) and is also significant in triggering the membrane fusion and is one of the extraordinary phenomena in the pathogenesis of SARS-CoV (Smieszek et al., 2020). The age is also an important factor in COVID-19 infection. In a clinical research, Lighter et al. (2020) reported that people more than sixty years are at higher risk of the infection. However, Jin et al., in a study found that men are more prone to the infection and their surviving potency is lower than females (Jin et al., 2020). Conditions of the patient become more severe if they are suffering from other diseases like hypertension, respiratory disorder, and cardiovascular diseases. Involvement of these diseases may enhance the mortality in this case (Yang Y. et al., 2020).
Presently, no specific drug and vaccine are available to combat COVID-19 infection. Although, various drug compounds are in the experimental and trial pipelines till now. EIDD-2801 is one of the potential clinical candidates for seasonal and pandemic influenza (Hampton, 2020). Hence, it can be suggested as a potential drug to treat COVID-19 only after clinical trials. Besides the implementation of neuraminidase inhibitors, RNA synthesis inhibitors, Lopinavir/Ritonavir, and peptide (EK1) can be used for its treatment (Rothan and Byrareddy, 2020). However, they are not sufficient to combat SARS-CoV-2 infection. According to recent reports, the antiviral remdesivir and chloroquine have safe records and can be efficiently implemented to treat COVID-19 infection (Zhang and Liu, 2020). Initially, it was suggested by the Washington Department of Health to use remdesivir intravenously to protect against COVID-19. Remdesivir is sufficient to block RNA synthesis by targeting RNA-dependent RNA polymerase and is being potentially used as an antiviral drug for the various RNA viruses (Patankar, 2020). Subsequently, remdesivir and chloroquine were implanted to treat COVID-19 infection. Favipiravir, ribavirin, and galidesivir are the nucleoside analog to be potentially used. Non-structural proteins i.e., chymotrypsin and papain-like protease, are required for virus replication and host immune response inhibition (Chen Y. W. et al., 2020). Inhibitors against them like cinanserin, flavonoids, and PLP inhibitors can be alternatively used for the treatment of the disease. More novel therapeutic agents are urgently required globally to fight against it. Furthermore, a list of non-specific drugs available to cure COVID-19 infection is mentioned in Table 1. Alternatively, some antiviral, i.e., nucleoside analogs and HIV-protease inhibitors, can be used to attenuate coronavirus viral infection. The treatment course included various drugs such as oseltamivir, lopinavir, and ritonavir. Along with the intravenous administration of ganciclovir, the patients are advised to take them twice a day for 3 to 14 days (Tobaiqy et al., 2020). However, in first-line treatment, paracetamol is used to treat fever, and expectorants (guaifenesin) should be given for non-productive cough. Oxygen therapy is required in critical conditions like severe acute respiratory infection and hypoxemia. The oxygen supply rate is 5 L/min in most of the children and non-pregnant women. However, in pregnant women, the supply rate is more than 92–95% (Huang et al., 2020). Patients suffering from AKI (acute kidney injury) should be subjected to renal replacement therapy. Antibiotic therapy starts within one hour after the confirmation of the symptoms. The bacterial and fungal infections can occur in the patients in the late and middle stages of diseases. So, it should be advisable to follow conventional and rational antibiotics followed as precision medicine applicable to a patient’s condition under critical care units (Figure 1). The implementation of IFN-α and lopinavir/ritonavir is recommended by the National Health Commission of the People’s Republic of China (Wang and Zhu, 2020). The implementation of the above medicines reduces the mortality rate in SARS infected patients (Chu et al., 2004). Methylprednisolone may also consider the children for a maximum of five days (Mouton et al., 2020). The patients suffering from the severe immune response are advised to take glucocorticoids. Different vaccine types such as subunit vaccines, attenuated viruses, and viral vector-based vaccines, inactivated viruses, DNA vaccines, and recombinant proteins can probably be used to cure COVID-19 infection (Saif, 2020). Trials on animal models are conducted to study the biological behavior of COVID-19. Presently, researchers worldwide are working for the development of a non-human primate model to know the mechanism of its interaction with the host (Chen et al., 2019).
Figure 1 The diagrammatic flow chart showing the implementation of drug to prevent the human health from COVID-19 infection.
The COVID-19 patients are generally diagnosed based on the clinical data. This takes time as the symptoms are generated after the infection sets deeper in the lungs. In such a situation, it becomes difficult for healthcare workers to treat the patients speedily. Biomarker identification provides an advantage over clinical diagnosis. Ulhaq and Soraya identified interleukin-6 (IL-6) as a potential biomarker for COVID-19 infection. As COVID-19 is linked with swift replication and a propensity to infect the lower respiratory tract, so it results in an increased response of IL-6-promoted severe respiratory distress. Thus, the levels of IL-6 can be linked to the disease progression in patients which can prove helpful in further treatment (Ulhaq and Soraya, 2020). C-reactive protein (CRP) is produced by the liver and induced by a range of inflammatory intermediaries such as IL-6. Regardless of its non-specificity, this acute phase reactant is used clinically as a biomarker for different inflammatory situations; an augmentation in CRP intensity is related with an increase in severity of disease. Lactate dehydrogenase is related to severity of pneumonia. Significant rise in LDH levels was observed among refractory COVID-19 patients. The COVID-19 infection leads to thrombocytopenia so platelet count is also a reliable marker for diagnosis of disease severity (Kermali et al., 2020). The Hematologic biomarkers include increase in leukocyte count as a distinguishing factor among infected and non-infected people. In a meta-analysis (Yang J . et al., 2020) carried out by Henry and colleagues in 2020, they found that among 2,984 COVID-19 patients there was significant difference in leukocyte count among severe and non-severe patients (Henry et al., 2020b). According to Bernheim et al., 2020, chest tomography (CT)/X-ray imaging is also a vital component to diagnose COVID-19 suspects when the number is large for diagnosis. In a study they found that CT scan of 56% infected individuals of COVID 19 came normal. Thus it has limited sensitivity and negative predictive value in the early stage of infection (Bernheim et al., 2020).
With the advent of next-generation technologies, the systems biology (Hu et al., 2020; Tang et al., 2020) is applied for the assessment of microbial virulence and associated pathogens (Pezeshki et al., 2019; Çakır et al., 2020; Eckhardt et al., 2020). Information and risk assessment of novel pathogens that emerged with time due to mutations, recombinants, horizontal/vertical gene transfer and reoccurrence of outbreaks need to be presented into the databases (McCormick, 2003; Pybus et al., 2007; Escobedo-Bonilla, 2013; Hartzell and Blaylock, 2014; Kaiser et al., 2015; Deneke et al., 2017; Liu et al., 2019; Olanya et al., 2019).
This update to the existing databases of newly emerged or novel pathogens create a challenge and an opportunity for multiomics experts to collect information/data, reunite and organize new standard datasets/databases or update the already existing databases (Kong et al., 2006; Winnenburg et al., 2007; Schumacher et al., 2014; Xie et al., 2017; Bloch and Bailin, 2019; Dong et al., 2019; Duncan et al., 2019; Yan et al., 2019; Chan et al., 2020). The new information will help the clinical and medical researchers to plan research methodologies for therapeutic and drug development. For instance, the novel coronavirus is a newly emerged pathogen which is creating nuisance to humankind. It is estimated that, globally, approximately 0.2 million patients out of thirteen million infected cases died with coronavirus infection, and the rates are still increasing (Baud et al., 2020; Chaurasiya et al., 2020; Kobayashi et al., 2020). The risk, hazards, and exposure characterization and assessment (Njage et al., 2019; Perera et al., 2019; Pavelić et al., 2020) are essential for building the background knowledge to evaluate the possibilities of novel therapeutics and drugs discovery against coronavirus. The systems biology majorly consists of multiomics, databases, and in silico studies (Arora and Singh, 2018). In silico approach is mainly dependent on computational designing (Koutsoukas et al., 2011; Lavecchia and Cerchia, 2016) and analyzing the interaction of proteins (Bultinck et al., 2012; Oany et al., 2014). The database mining also contributes to the in silico approach (Loging et al., 2007; Rao et al., 2014). As prerequisite to the database (Boissel et al., 2004; Nagata and Pastan, 2009), researching the information is required for the practical planning of methodologies (Wu et al., 2011). The study of heritable phenotypic changes, called as epigenomics, is supposed to play an important role in understanding the mortality rate among black and white individuals (Holmes et al., 2020). These changes may be inherited by the cell system as the memory (Holliday, 1987). The covalent modifications on lysine acetylation, lysine methylation, arginine methylation, serine, and threonine phosphorylation, lysine ubiquitination are the major epigenetic mechanisms which can determine the many genetic and phenotypic modulations (Holliday, 1987; Zhang et al., 2019). The heterogeneity in treatment success is also believed to be influenced by these epigenomic changes (Holmes et al., 2020). As the corona virus targets on the lung cells (Conti et al., 2020), the epigenetic control of ACE2 in the lungs cannot be denied. As Woo and Alenghat highlighted the regulation of transcription during host–microbe interaction under the epigenetic modifications, it may be possible that the rate of transcription of virus may be negatively influenced under genetic environmental pressure e.g. strong immune cells. Hence, the more epigenetic exploration is needed to prevent the corona virus infection (Woo and Alenghat, 2017). DNA methylation may be one of the major factors in providing fewer sites for attack of the viral genetic material. The components related to biological entities like DNA, RNA, proteins, metabolites (Jacob et al., 2019; MacMullan et al., 2019) correspond to genomics, proteomics, and metabolomics, respectively (Yan et al., 2019; Jenkins and Orsburn, 2020). Various other systems biology approaches which can be utilized for the development of a potent drug against COVID-19 include immunomics, host lipid omics, public health omics and quantitative dynamic omics. This multiomics approach (Figure 2) is also taking attention to therapeutic development (Donovan et al., 2019; Lee and Ruppin, 2019).
Figure 2 The multiomics approach for the systemic use of technologies for the development of new drug targets for coronavirus.
The interaction of any protein with its receptors always depends on the receptor-binding domains on the proteins (Lan et al., 2020a). These receptor binding domain recognizes their interactive sequences on the receptors and binds them with mostly non-covalent bonds in the human system. However, the SARS-Cov-2 viruses mainly attack the mucus membranes of the human system as their first site of attachment, but they are then bound to their receptors, i.e., angiotensin-converting enzyme 2 (ACE2), and finally helps the viruses to come inside the host cells (Lan et al., 2020b). Moreover, after entering the cell, they start their replication with the help of replication proteins and followed by multiplication steps, as shown in schematic Figure 3.
During the multiplication and their amplification steps, SARS-CoV-2 are responsible for the release of some proteases, which leads to the generation of reactive oxygen species (ROS) in the host cell (Chen Y. et al., 2020; Nasi et al., 2020). These ROS are toxic to the cell system and its environment. On the other hand, host defense mechanism activates in the form of their immune system, and the free-flowing neutrophils of the blood reached the target site, the lungs (mainly in the alveoli, where the severe acute respiratory virus reached after attaching the nasal, oral or mucus surfaces). In addition to neutrophils, monocytes differentiate into specific tissue macrophages, in response to the infection. However, neutrophils, basophils, monocyte etc. are myeloid progenitor cells and are parts of the innate immunity. These cells, upregulated in any foreign particle, enter into the human body, specifically the virus (van de Laar et al., 2016). The release of cytokines is also supposed to be initiated through adaptive and innate immunity cell signaling. In response to toxicity and foreign particles, the metabolism of the macrophages fluctuates, and they tend to release the inflammatory cytokines which finally affects the molecular signaling of responsive target cells and their neighbor cells. When the normal cellular signaling fluctuates either by ROS stress or by inflammatory cytokines resulting from the SARS-CoV-2 entering the host system, they lead to mucus accumulation in the lungs, abnormal and painful breathing, abnormal BP, etc. Here, a small study through computational biology and in silico tools for a better understanding of host–virus interaction is designed.
The Homo sapiens angiotensin I-converting enzyme 2 (ACE2), transcript variant 1, mRNA of Human origin, with a length of 3,339 bp, was extracted from the NCBI with the accession number NM_001371415.1. The mRNA was converted to the protein sequence by ExPASy; its 5–3 and 3–5 predicted sequences were found. Further, through protein blast, angiotensin-converting enzyme 2 precursor was finalized for the study based on its 100% identity with our query sequence. The accession number of the angiotensin-converting enzyme 2 precursor was NP_001358344.1, and this was the only protein showing 100% identity with our ExPASy query sequence.
The 3D modeling of the protein was done using SWISS-MODEL. The obtained protein model was a monomer containing ligands, as N-acetyl-D-glucosamine and Zinc. The template used by the SWISS-MODEL (Table 2) for model prediction was 6m17 PDB, and it showed the 100% sequence identity coverage with the predicted model, and that is angiotensin-converting enzyme 2. Now, interestingly, this ACE2 showed to be responsible for acting as a receptor for the coronavirus entry to the cells. The QMEAN value for the predicted protein model was −0.99 and is supposed to be of good quality to be used for the study. Figure 4 depicts the overall protein 3D model with side and top views, along with its QMEAN value to examine its quality. The Quality of the predicted model was also validated through the ProSA web server, based on NMR and X-ray data. For further validation of the protein model, the ProQ webserver was used, and it predicts the model of extreme quality was good based on the LG score. The Ramachandran plot assessment also suggests its 98% amino acids in the favored region of the plot.
Table 2 In silico tools used in present study (Protein-protein/Protein-ligand study for drug target and protein target inhibition).
Figure 4 The 3D structure of angiotensin-converting enzyme 2 isoform X1, (A) side view and (B) top view, and (C) the QMEAN value for the protein model showing the statistical representation of the protein model quality.
Similarly, the spike S1 protein of the coronavirus (source organism; Wuhan seafood market, Pneumonia virus) was downloaded from the RCSB protein data bank with the PDB ID, 6M17 (Yan et al., 2020). It was present as a 2019-nCoV RBD/ACE-B0AT1 complex in its PDB format, but to check the protein–protein interaction, the PDB file was modified except for one of the SARS-CoV-2 receptor binding domain. The 3D structure of the protein is shown in Figure 5.
Figure 5 (A) The protein-protein docked complex (cluster 5) of ACE2 isoform X1of Human cell (red color), and spike S1 protein (blue color) of corona virus, showing the close interaction. (B) The plot between HADDOCK score and RMSD values of the protein–protein docked clusters, showing the best suitability of cluster 5 in the plot and hence to be used to depict the interaction results of ACE2 and spike protein.
The tentative protein–protein interactions are crucial to understand further mechanisms, and a preview of protein–protein docked complex is presented in Table 3 (van de Laar et al., 2016). Among all the docked complexes, the best structure of cluster 5 complex was depicted in Figure 5 for its molecular interactions. The cluster 5 has the best HADDOCK score (883.4 +/– 9.7), the lowest Van der Waals energy (–171.7 +/– 9.8), and the Z-score (−2.2). This gives a good understanding towards future tools for understanding such interactions.
Table 3 The docking approaches between ACE2 isoform X1 and spike S1 protein of the corona virus by HADDOCK.
Vaccine development is in demand due to the increasing rate of mortality and morbidity of COVID-19 infection. Vaccines play an important role in the reduction of toxicity and the elimination of diseases (Dubey et al., 2018; Amanat and Krammer, 2020). The conventional technologies of vaccine designed have many limitations, including time consumption, laborious, costly, and many more. Perhaps somehow, the involvement of in silico tools is sufficient to overcome the mentioned limitations (Rauch et al., 2018). Immunoinformatics approach like reverse vaccinology, epitope prediction, rational vaccinology, and structural vaccinology is advantageous to be used for the designing of vaccines (Kazi et al., 2018). Moreover, side chain and backbone modeling are significant in designing the antibody structure to act as vaccine target. SCWRL and SCAP are the in silico tools used to identify the mutation in the proteins (He et al., 2015). RAMBLE and RAPER are the additional software applied for side chain prediction analysis. Multivant scaffolding is another tool applied for the designing of potential epitope (He et al., 2015). However, an epitope can be grafted by using multigraft interface technology. ORF-FINDER, GS FINDER, and GLIMMER are some of the in silico tools that performed the screening of ORFs and selected the most immunogenic peptide that alternatively help in vaccine designing (Davies and Darren, 2007).
Structural biology is an important area of immunoinformatics and the basis for the structure of proteins. On the basis of structure, rational vaccinology analyzes the structure of novel protein antigens that will be targeted as potential vaccine candidates (Hegde et al., 2018). Rational technology of vaccine design was significantly used against viral pathogens like influenza, HIV (Van Regenmortel, 2019) and Hepatitis C (He and Zhu, 2015). Furthermore, approaching systems biology enhances the understanding of host–pathogen interaction and also develops adjuvant that provides long-lasting immunity. Vaxijen is another important computation tool that contributes to vaccine development. It is an online software that uses the alignment-free approach to predict the antigenic nature of the proteins (Sinha and Shukla, 2019; Bappy et al., 2020). Majorly the critical process in vaccine development is the identification of epitopes that can be targeted as a vaccine candidate. A number of epitope prediction tools are available that can be significantly used for epitope prediction (Naz et al., 2020). The B-cell and T-cell epitopes of novel SARS-CoV-2 were analyzed by Ahmed et al. by approaching IEDB and other computational tools (Grifoni et al., 2020). Ong et al. (2020) reported the reverse vaccinology and machine learning approach were used to identify the potential vaccine candidate against COVID-19 infection (Ong et al., 2020). As reported by Chen and Wu (2020), ABCpred and BepiPred and IEDB are the epitope prediction tools used for the identification of epitopes in the novel SARS-CoV-2. Besides, the multigraft, multivalent scaffolding, codon optimization, and antibodyomics tools are also helpful in the recognition and construction of potential vaccine candidates (Sunita et al., 2020). However, at present computational tools are the first to be used for vaccine designing of emerging diseases. Later on, these will be validated by experimental studies. Collectively, the actual implementation of these disciplines accelerates the process of vaccine development (Chin et al., 2019).
The Chemdraw software is used to draw the molecular structure of a molecule or compound in the computer and is easy to handle in an offline mode. This gives an immediate and clear sharp image of the structure and can be saved in many file types, eg; mol file. The file needs not to be redrawn if any correction has to be added. Hence this provides an immediate output of the structures to be used in any docking study. The software, SWISS-MODEL is designed for the homology modeling of the proteins. The software works easily in windows system and gives the output in a simple manner so as the beginners in the field of systems biology can easily benefit. The protein model quality is also validated on the basis of Q mean score, which is the collective information of many parameters e.g. X-ray and NMR. After protein homology modeling, there is a need of the formed structure validation, and this task can be done by many online software applicatioms e.g.; ERRAT, ProSA, ProQ, RAMPAGE etc. Further, the ProQ also gives its own score called as LG score, for the highest ranked models in protein validation. This model validation can be analyzed based on their scores (software gives different scores more or less similar based on their inbuilt programming).
The Ramachandran plot provides the quantitative data for favored and unfavored regions, which basically used the protein backbone dihedral angles and is necessary for protein validation. The Schrödinger and AutoDock software is used for the molecular docking of protein–ligand or protein–protein. However, both the software programs can be used for the docking, but an expert should be needed to deal with these software programs, and it needs some prior knowledge to work with them. The software programs, UCSF Chimera and PyMOL are used for the molecular structure analysis, and it need some knowledge to handle the software programs correctly. But with basic knowledge of molecules and bioinformatics, the online tools can be handled easily and can give the best results in the drug discovery and exploration of proteins. The HADDOCK online server provides the quantitative description for the protein–protein molecular interaction. It provides many clusters of protein–protein interactions based on the possibility of the bond formations and the analysis involved many parameters eg; Haddock score, Z-score. These parameters are necessary for choosing the best cluster for further analysis of the molecular interactions using systems biology approach. Van der Waals interaction is the distance dependent interaction between atoms, and Haddock provides us the series of interactions (strongest to weaker). However, so software can easily give results based on their input algorithm, we have to analyze the parameters for the perfect study.
The MRA (Microbial Risk Assessment) (Brul et al., 2012; Haddad et al., 2018) is a clinical evaluation of virulence associated with pathogen, mainly foodborne pathogens i.e., animal meat and their products (Wassenaar et al., 2007; Franz et al., 2016). Nowadays, the utilization of multiomics datasets for improving and redesigning the role model of microbial risk assessment is being practiced by researchers (Buchanan et al., 2000; denBesten et al., 2018). The dose–response models are designed by clinical researchers for studies, especially for diseases associated with RNA viruses (Gale et al., 2014). This probabilistic approach firstly consists of the evaluation of viral infections via oral path, i.e., the host and virus interaction via cellular receptors with and without conquering the host defense system and replication in the host cell (Voysey and Brown, 2000; Huang and Haas, 2009), followed by designing the models to study disease progress immediately after infection. The virulence markers are the viral gene sequences representing the viral disease-related traits (Haddad et al., 2018; Liang J. et al., 2020). Therefore, it is observed that the above-discussed appeal must be undertaken for coronavirus probabilistic virulence assessment (Benvenuto et al., 2020). Since dose–response models are particular for RNA viruses thus, the possibility and chances of multiomics application (Zhang et al., 2018) to search the antiviral targets rise for the development of therapeutics and drugs against coronavirus (Fritsch et al., 2018; Jeon et al., 2020).
Genomics offers the task to reveal the characteristics of drugable genomes (Hopkins and Groom, 2002), which consist of sequences and alignments signifying the virulence trait (Losada et al., 2016; Lee et al., 2017). Data mining from databases can make it possible for the researcher to seek out queries related to coronavirus (Hatcher et al., 2017). For the purposive research regarding the newly emerged virus, the National Institute of Health (NIH) of United States created a resource (http://www.niaid.nih.gov), an integrated surveillance data for supporting researchers in collaboration with various research institutes working on systems biology (Squires et al., 2012; Uyeki et al., 2016). The main aim is to make the comparative genomic research for correlative analysis of coronaviridae family-related genus and strains for the predicted data and annotated genome sequences (Zhu et al., 2016; Liang J. et al., 2020; Randhawa et al., 2020). In this process, the MSA (Multiple Sequence Alignment) signifies the closely related RefSeq constructing the virus ortholog groups with associated protein playing role in virulence (Fumagalli et al., 2010; Chapman et al., 2011; Claytor et al., 2017). Till now, there is very little scientific data and literature available related to coronavirus. The genomics approach is leading and generating the manually curated research data (Brister et al., 2015; Manzoni et al., 2018) about the clinical coronavirus strains aiding the scientific literature country-wise in the above-discussed manner (Huang et al., 2007).
Transcriptomics is mainly concerned with gene expression profile (Irigoyen et al., 2016) i.e., by ribosome profiling (Irigoyen et al., 2016), RNA sequencing (Depledge et al., 2019), and high throughput DNA microarrays (Wang et al., 2009). The dose–response models are developed to study the factors affecting gene expression (Hashem et al., 2019) at different concentrations of virulence proteins (Haas, 2020). They also check the mRNA abundance at various levels of infection progression (Albariño et al., 2018). The metabolic enzymes run cellular metabolism (Ahmed Idris et al., 2020). The cellular metabolism is revealed through the intense study of genomics, transcriptomics, and proteomics (Fanos et al., 2020), which are directly and indirectly linked to pathways involved in metabolomics (Haas et al., 2016). The primary significance of metabolomics comes for diagnostic assessment (To et al., 2016). The concentrations of metabolites (Sinha et al., 2019) are detected by NMR (Nuclear Magnetic Resonance), HPLC/MS (High-performance Liquid Chromatography/Mass Spectrometry) (Peng and Liu, 2017). Metabolic profile analysis would reveal the binding and inactivation of metabolites by drugs (Eisfeld et al., 2017) that would arrest further disease progression (Zhao and Lin, 2014). In this manner, transcriptomics and metabolomics increase the possibility for developing therapeutics and drugs against the coronavirus.
Proteomics study reveals the functional role of proteins associated with host and pathogen (Zheng et al., 2018). With available resources of database and drug targets obtained by previous studies on influenza virus (Sadewasser et al., 2017), hepatitis C virus (Lupberger et al., 2019), poxvirus (Grossegesse et al., 2017), Nipah virus (Vera-Velasco et al., 2018), etc., it is feasible to develop novel drugs. The studies on GPCR (G-protein coupled receptors) (Sriram and Insel, 2018), ion channels (Lin, 2019; Duncan et al., 2020), and enzymes (Ding et al., 2017) provide the platform for researchers to study drug–target interactions. Zheng and Perlman, in 2018 discussed the importance of proteomics in the host immune system and respiratory virus interaction response. They insighted the landscape proteomics analysis formed by prediction of clinical data and the role of immune response gathered via host lipid omics, immunomics, phosphoproteomics, and public health omics (Zheng and Perlman, 2018). The recent study done by Kang et al., 2020, the western blotting, protein categorization, gel digestion, SDS-PAGE analysis, SILAC labeling for protein analysis, protein identification, separation and quantification methodologies for identification of structural proteins of coronavirus, mainly bronchitis virus particles were done. The above-discussed method is likely to be the strategy for finding the novel antiviral against coronavirus.
Immunomics is based on the efficiency of the host to eliminate pathogens that enter the human body. The immune system of organisms contains many cellular, molecular, and physical components that provide defense against invasive microorganisms. Dysregulation of immune cells such as inflammatory monocyte–macrophage and type I interferon (IFN) led to the occurrence of lethal pneumonia in mice infected with SARS-CoV (Channappanavar et al., 2016). This indicates that immune cells play a vital role in combating pathogens. The immune memory cells are able to protect the host from the early invasion of respiratory pathogens. Bioinformatics tools and sequence homology can be used to find potential immune targets and designing of a vaccine against COVID-19. Grifoni et al. used the Immune Epitope Database (IEDB) and Analysis Resource for prediction of COVID-19. They used SARS-CoV to predict epitope responses as it shows higher similarity to SARS-CoV-2. They found conserved regions in SARS-CoV and SARS-CoV-2 caused COVID-19. Vaccination approach proposed to target the immune response toward these conserved epitope regions could generate immunity. This will not only protect from Betacoronaviruses but also against moderately challenging virus that will emerge in future (Grifoni et al., 2020). Carbohydrates present on the host and viral proteins are potential targets for modulating the immune response. The use of computational tools and integrated omics approaches can lead to the development of vaccines and drugs for such targets of viral infection and receptors (Zheng and Perlman, 2018).
Lipids play a vital role in the interaction of the virus with the host cells. Lipids can act straightly as the receptors or co-factors of entry for viruses at the surface of cell or endosomes. Viral replication complex highly depends on them, and lipids also provide energy for replication of the virus. Lipids can help to order the suitable cellular allocation of viral protein and also the trafficking, assemblage, and liberation of viral particles. Thus, host lipid studies can play indispensable role in understanding virus propagation (Diamond et al., 2010; Das, 2020). Coronaviruses seize intracellular membranes of the host cells to produce fresh partitions called double-membrane vesicles (DMVs). These partitions help in the viral genome amplification. A current study showed that a primary lipid processing enzyme, cytosolic phospholipase A2α enzyme (cPLA2α), was directly linked with DMVs’ development and replication of coronaviruses (Müller et al., 2018). Coronaviruses require a specific composition of lipids for their replication. If this lipid homeostasis is broken, then the viral replication is affected. Yan and his co-workers found in a study that glycerophospholipids and fatty acids (FAs) were considerably increased in the HCoV-229E-infected cells, and the linoleic acid (LA) to arachidonic acid (AA) metabolism was strikingly disturbed upon infection of HCoV-229E. Exogenous supplementation of LA and AA decreased the replication of coronavirus. They came to the conclusion that there was an upregulation of lipids that were responsible for replication and membrane synthesis. The virus maintains homeostasis for its better replication, but when this homeostasis is broken by supplying lipids from outside the cell, the replication of the virus is disturbed. Thus, lipidomics can provide better treatment strategies if integrated with immunological data (Yan et al., 2019).
Public health omics takes into consideration the entire kinetic response of the host. In public health omics, the expressions of genes and transcriptome are studied. The interaction and regulation of different transcriptome datasets are studied. It takes into consideration the upregulation and down-regulation of different genes during infection. It takes account of molecular as well as clinical conditions of the host and pathogen. The pathway interaction and response of host are analyzed after the infection by utilizing this methodology. This renders the whole set of the idea in host–pathogen interaction with respect to the time of infection. Such systems biology methods draw attention to the significance of time-related factors in the study of multifactorial diseases such as influenza and coronavirus. A study by Dimitrakopoulou and his group revealed the temporal effect of the influenza virus by studying the interactome and signaling pathways. Their findings cooperatively update the budding area of public health omics and potential clinical trials intended to interpret dynamic host reactions to pathogens (Dimitrakopoulou et al., 2014). This is perhaps the unnoticed field of omics technology, but its application can give better results for understanding the spread of COVID-19. This technique will help in the development of time dependent drug development in case of infection (Pawelek et al., 2012).
Artificial intelligence plays a vital role in this global scenario to fight against COVID-19. Artificial intelligence and machine learning techniques have helped to group data of genomic taxonomic classification, detection assay based on CRISPR (Dangi et al., 2018; Vashistha et al., 2018), endurance calculation of patients, and identifying probable drug candidates for COVID-19. Metsky et al. screened SARS-CoV-2 by machine learning designs employing a CRISPR-based virus detection system with high speed and sensitivity (Metsky et al., 2020). Similarly, artificial intelligence can be used for the management of critical patients of COVID-19. Rahmatizadeh et al. applied a three-stage model based on input, process, and output. They took into consideration paraclinical, clinical, epidemiologic data, personalized medicine, diagnosis, risk stratification, treatment, prognosis, and management.
The AI (Artificial Intelligence) approach is helpful in stratifying patients and their timely cure (Rahmatizadeh et al., 2020). Thus, computational tools not only help in virus detection but also in drug development. Wu and his co-workers analyzed the proteins coded by the SARS-CoV-2 virus and modeled them for target prediction. They predicted potential targets and probable drugs against them. They screened 3-chymotrypsin-like protease (3CLpro), spike, RNA-dependent RNA polymerase (RdRp), and papain like protease (PLpro) thoroughly. 78 generally used antiviral drugs and compounds from ZINC database were used for positioning and structural analysis. Thus, in silico studies provide drug repositioning to treat COVID-19 (Wu et al., 2020a). A deep-learning based analysis structure of thoracic CT images was built for computerized recognition and observation of COVID-19 patients over time. Swift development of computerized diagnostic systems based on artificial intelligence and machine learning cannot only give improved diagnostic accurately and rapidly, but will also defend healthcare workers by diminishing their contacts with COVID-19 patients (Alimadadi et al., 2020).
The present review gives an insight into the applicability of systems biology tools for developing drugs against COVID-19 infection. The ultimate aim is to find the possible viral targets by exploring the pathogenicity and virulence strategy of coronavirus. The promising in silico application of molecular interaction and simulation study is done purposely for the understanding of host (human) and virus interaction to plan the future strategies for managing the situations of virus pandemics. It is worthy to mention that omics data and systems biology algorithms can combine data from cytokines, blood cell populations, proteomics, transcriptomics, clinical parameters, and epidemiological data to develop personalized medicine strategies and patient stratification based on omics. Although it is difficult to make the strategies or policies by the non-medical expertise of the administration, with the help of systems biology, the possibilities increase. The molecular docking and simulations study are presented to make it simple and approachable to non-target audience also, to ensure the seriousness of the COVID-19 as a global pandemic. In conclusion, it can be stated that the systems biology can lead based on the obtained sequencing data, to ensure the understanding of molecular and physiological mechanisms/phenomena and definitely help in breaking the coronavirus like epidemic outbreaks in future by potential antiviral drugs acting on target for preventing the associated disease progression and increasing the infected patient’s treatment effectiveness.
SJ wrote the first draft of the manuscript with contributions from MK, Mandeep, and Sunita. The final draft was read and edited by YS and PS. All authors contributed to the article and approved the submitted version.
PS acknowledges the infrastructural support from the Department of Science and Technology, New Delhi, Govt. of India, through FIST grant (Grant No. 1196/SR/FST/LS-I/2017/4). SJ and Sunita acknowledges Maharshi Dayanand University, Rohtak, India, for providing University Research Scholarship (URS). Mandeep acknowledges the Junior Research Fellowship from CSIR, India (Award No. 09/382(0211)/2019-EMR-I). MK acknowledges the support from Prof. D.K. Singh, DU for his permission to be a part of this manuscript and the Hindu College, DU for Innovation project (IP-2019-20/SC/05 and 06).
The authors declare that the research was conducted in the absence of any commercial or financial relationships that could be construed as a potential conflict of interest.
Ahmed S. F., Quadeer A. A., McKay M. R. (2020). Preliminary identification of potential vaccine targets for the COVID-19 coronavirus (SARS-CoV-2) based on SARS-CoV immunological studies. Viruses 12 (3), 254. doi: 10.3390/v12030254
Ahmed Idris S., Hyder M., Altayb N. (2020). The Important Role of Fever during Infection and the Use of Fever for Treatment of Coronavirus Patients. Mohamed and Altayb, Nawal, The Important Role of Fever during Infection and the Use of Fever for Treatment of Coronavirus Patients (March 14, 2020). doi: 10.2139/ssrn.3556215
Albariño C. G., Guerrero L. W., Chakrabarti A. K., Nichol S. T. (2018). Transcriptional analysis of viral mRNAs reveals common transcription patterns in cells infected by five different filoviruses. PloS One 13 (8), e0201827. doi: 10.1371/journal.pone.0201827
Alimadadi A., Aryal S., Manandhar I., Munroe P. B., Joe B., Cheng X. (2020). Artificial intelligence and machine learning to fight COVID-19. Physiol Genomics 52, 200–202. doi: 10.1152/physiolgenomics.00029.2020
Amanat F., Krammer F. (2020). SARS-CoV-2 vaccines: status report. Immunity. 52 (4), 583–589. doi: 10.1016/j.immuni.2020.03.007
Archana A., Kaur P., Kanodia S., Priyanka S. G., Khuntia P., Anant K. A., et al. (2015). Evaluating Microbial &Chemical Quality of Delhi-NCR Drinking Water, Enhancing its Standard & Spreading Mass Awareness. http://journals.du.ac.in/ugresearch. ISSN: ISSN 2395 - 2334, Volume 1, Issue 1, Feb 2015.
Arora D., Singh A. (2018). Systems biology approach deciphering the biochemical signaling pathway and pharmacokinetic study of PI3K/mTOR/p53-Mdm2 module involved in neoplastic transformation. Network Model. Anal. Health Inf. Bioinf. 7 (1), 2. doi: 10.1007/s13721-017-0162-9
Bappy S. S., Sultana S., Adhikari J., Mahmud S., Khan M. A., Kibria K. K., et al. (2020). Extensive Immunoinformatics study for the prediction of novel peptide-based epitope vaccine with docking confirmation against Envelope protein of Chikungunya virus: A Computational Biology Approach. J. Biomolecular Structure Dynamics 1–16. doi: 10.1080/07391102.2020.1726815
Baud D., Qi X., Nielsen-Saines K., Musso D., Pomar L., Favre G. (2020). Real estimates of mortality following COVID-19 infection. Lancet Infect. Dis. doi: 10.1016/S1473-3099(20)30195-X
Belouzard S., Chu V. C., Whittaker G. R. (2009). Activation of the SARS coronavirus spike protein via sequential proteolytic cleavage at two distinct sites. Proc. Natl. Acad. Sci. 106 (14), 5871–5876. doi: 10.1073/pnas.0809524106
Benvenuto D., Giovanetti M., Ciccozzi A., Spoto S., Angeletti S., Ciccozzi M. (2020). The 2019-new coronavirus epidemic: evidence for virus evolution. J. Med. Virol. 92 (4), 455–459. doi: 10.1002/jmv.25688
Bernheim A., Mei X., Huang M., Yang Y., Fayad Z. A., Zhang N., et al. (2020). Chest CT findings in coronavirus disease-19 (COVID-19): relationship to duration of infection. Radiology 295, 685–691, 200463. doi: 10.1148/radiol.2020200463
Bleasel M. D., Peterson G. M. (2020). Emetine, Ipecac, Ipecac Alkaloids and Analogues as Potential Antiviral Agents for Coronaviruses. Pharmaceuticals 13 (3), 51. doi: 10.3390/ph13030051
Bloch K. C., Bailin S. S. (2019). Update on fungal infections of the central nervous system: emerging pathogens and emerging diagnostics. Curr. Opin. Infect. Dis. 32 (3), 277–284. doi: 10.1097/QCO.0000000000000541
Boissel J. P., Amsallem E., Cucherat M., Nony P., Haugh M. C. (2004). Bridging the gap between therapeutic research results and physician prescribing decisions: knowledge transfer, a prerequisite to knowledge translation. Eur. J. Clin. Pharmacol. 60 (9), 609–616. doi: 10.1007/s00228-004-0816-2
Brister J. R., Ako-Adjei D., Bao Y., Blinkova O. (2015). NCBI viral genomes resource. Nucleic Acids Res. 43 (D1), D571–D577. doi: 10.1093/nar/gku1207
Brul S., Bassett J., Cook P., Kathariou S., McClure P., Jasti P. R., et al. (2012). ‘Omics’ technologies in quantitative microbial risk assessment. Trends Food Sci. Technol. 27 (1), 12–24. doi: 10.1016/j.tifs.2012.04.004
Buchanan R. L., Smith J. L., Long W. (2000). Microbial risk assessment: dose-response relations and risk characterization. Int. J. Food Microbiol. 58 (3), 159–172. doi: 10.1016/S0168-1605(00)00270-1
Bultinck J., Lievens S., Tavernier J. (2012). Protein-protein interactions: network analysis and applications in drug discovery. Curr. Pharm. Design 18 (30), 4619–4629. doi: 10.2174/138161212802651562
Çakır T., Panagiotou G., Uddin R., Durmuş S. (2020). Novel Approaches for Systems Biology of Metabolism-Oriented Pathogen-Human Interactions: A Mini-Review. Front. Cell. Infect. Microbiol. 10, 52. doi: 10.3389/fcimb.2020.00052
Cascella M., Rajnik M., Cuomo A., Dulebohn S. C., Di Napoli R. (2020). Features, evaluation and treatment coronavirus (COVID-19) (StatPearls: StatPearls Publishing).
Chan K. W., Wong V. T., Tang S. C. W. (2020). COVID-19: An Update on the Epidemiological, Clinical, Preventive and Therapeutic Evidence and Guidelines of Integrative Chinese-Western Medicine for the Management of 2019 Novel Coronavirus Disease. Am. J. Chin. Med. 68, 737–762. doi: 10.1142/S0192415X20500378
Channappanavar R., Fehr A. R., Vijay R., Mack M., Zhao J., Meyerholz D. K., et al. (2016). Dysregulated type I interferon and inflammatory monocyte-macrophage responses cause lethal pneumonia in SARS-CoV-infected mice. Cell Host Microbe 19, 181–193. doi: 10.1016/j.chom.2016.01.007
Chapman D. A., Darby A. C., Da Silva M., Upton C., Radford A. D., Dixon L. K. (2011). Genomic analysis of highly virulent Georgia 2007/1 isolate of African swine fever virus. Emerg. Infect. Dis. 17 (4), 599. doi: 10.3201/eid1704.101283
Chaurasiya P., Pandey P., Rajak U., Dhakar K., Verma M., Verma T. (2020). Epidemic and Challenges of Coronavirus Disease-2019 (COVID-19): India Response. SSRN 3569665. doi: 10.2139/ssrn.3569665
Chen Z., Long T., Wong P. Y., Ho W., Burk R. D., Chan P. K. (2019). Non-human Primate Papillomaviruses Share Similar Evolutionary Histories and Niche Adaptation as the Human Counterparts. Front. Microbiol. 10:2093. doi: 10.3389/fmicb.2019.02093
Chen Y., Liu Q., Guo D. (2020). Emerging coronaviruses: genome structure, replication, and pathogenesis. J. Med. Virol. 92 (4), 418–423. doi: 10.1002/jmv.25681
Chen Y. W., Yiu C. P. B., Wong K. Y. (2020). Prediction of the SARS-CoV-2 (2019-nCoV) 3C-like protease (3CL pro) structure: virtual screening reveals velpatasvir, ledipasvir, and other drug repurposing candidates. F1000Research 9. doi: 10.12688/f1000research.22457.2
Chen J. (2020). Pathogenicity and transmissibility of 2019-nCoV-a quick overview and comparison with other emerging viruses. Microbes Infect. doi: 10.1016/j.micinf.2020.01.004
Cheng C. Y., Lee Y. L., Chen C. P., Lin Y. C., Liu C. E., Liao C. H., et al. (2020). Lopinavir/ritonavir did not shorten the duration of SARS CoV-2 shedding in patients with mild pneumonia in Taiwan. J. Microbiol. Immunol. Infect. 53 (3), 448–492. doi: 10.1016/j.jmii.2020.03.032
Chin W. L., Zemek R. M., Lesterhuis W. J., Lassmann T. (2019). Functional genomics in cancer immunotherapy: computational approaches for biomarker and drug discovery. Mol. Syst. Design Eng. 4 (4), 689–700. doi: 10.1039/C9ME00029A
Choy K. T., Wong A. Y. L., Kaewpreedee P., Sia S. F., Chen D., Hui K. P. Y., et al. (2020). Remdesivir, lopinavir, emetine, and homoharringtonine inhibit SARS-CoV-2 replication in vitro. Antiviral Res. 178, 104786, 104786. doi: 10.1016/j.antiviral.2020.104786
Chu C. M., Cheng V. C. C., Hung I. F. N., Wong M. M. L., Chan K. H., Chan K. S., et al. (2004). Role of lopinavir/ritonavir in the treatment of SARS: initial virological and clinical findings. Thorax 59 (3), 252–256. doi: 10.1136/thorax.2003.012658
Claytor S. C., Subramaniam K., Landrau-Giovannetti N., Chinchar V. G., Gray M. J., Miller D. L., et al. (2017). Ranavirusphylogenomics: signatures of recombination and inversions among bullfrog ranaculture isolates. Virology 511, 330–343. doi: 10.1016/j.virol.2017.07.028
Colovos C., Yeates T. O. (1993). Verification of protein structures: patterns of nonbonded atomic interactions. Protein Sci. 2 (9), 1511–1519. doi: 10.1002/pro.5560020916
Conti P., Ronconi G., Caraffa A. L., Gallenga C. E., Ross R., Frydas I., et al. (2020). Induction of pro-inflammatory cytokines (IL-1 and IL-6) and lung inflammation by Coronavirus-19 (COVI-19 or SARS-CoV-2): anti-inflammatory strategies. J. Biol. Regul. Homeost. Agents 34 (2), 1.
Coutard B., Valle C., de Lamballerie X., Canard B., Seidah N. G., Decroly E. (2020). The spike glycoprotein of the new coronavirus 2019-nCoV contains a furin-like cleavage site absent in CoV of the same clade. Antiviral Res. 176, 104742. doi: 10.1016/j.antiviral.2020.104742
Czernin J., Fanti S., Meyer P. T., Allen-Auerbach M., Hacker M., Sathekge M., et al. (2020). Nuclear medicine operations in the times of COVID-19: strategies, precautions, and experiences. J. Nucl. Med. 61 (5), 626–629. doi: 10.2967/jnumed.120.245738
Daga M. K., Kumar N., Aarthi J., Mawari G., Garg S., Rohatgi I. (2019). From SARS-CoV to Coronavirus Disease 2019 (COVID-19)-A Brief Review. J. Advanced Res. Med. 6 (4), 1–9. doi: 10.24321/2349.7181.201917
Dangi A. K., Sinha R., Dwivedi S., Gupta S. K., Shukla P. (2018). Cell line techniques and gene editing tools for antibody production: a review. Front. Pharmacol. 9:630:630. doi: 10.3389/fphar.2018.00630
Das U. N. (2020). Can Bioactive Lipids Inactivate Coronavirus (COVID-19)? Arch. Med. Res. 51 (3), 282–286. doi: 10.1016/j.arcmed.2020.03.004
Davies M. N., Darren R. (2007). Flower. “Harnessing bioinformatics to discover new vaccines.”. Drug Discovery Today 12.9-10, 389–395. doi: 10.1016/j.drudis.2007.03.010
Dawood A. A. (2020). Mutated COVID-19, May Foretells Mankind in a Great Risk in the Future. New Microbes New Infect. 35, 100673. doi: 10.1016/j.nmni.2020.100673
DeLano W. L. (2002). Pymol: An open-source molecular graphics tool. CCP4 Newslett. Protein Crystallogr. 40 (1), 82–92.
denBesten H. M., Amézquita A., Bover-Cid S., Dagnas S., Ellouze M., Guillou S., et al. (2018). Next generation of microbiological risk assessment: potential of omics data for exposure assessment. Int. J. Food Microbiol. 287, 18–27. doi: 10.1016/j.ijfoodmicro.2017.10.006
Deneke C., Rentzsch R., Renard B. Y. (2017). PaPrBaG: A machine learning approach for the detection of novel pathogens from NGS data. Sci. Rep. 7 (1), 1–13. doi: 10.1038/srep39194
Deng S. Q., Peng H. J. (2020). Characteristics of and public health responses to the coronavirus disease 2019 outbreak in China. J. Clin. Med. 9 (2):575. doi: 10.3390/jcm9020575
Depledge D. P., Srinivas K. P., Sadaoka T., Bready D., Mori Y., Placantonakis D. G., et al. (2019). Direct RNA sequencing on nanopore arrays redefines the transcriptional complexity of a viral pathogen. Nat. Commun. 10 (1), 1–13. doi: 10.1038/s41467-019-08734-9
Diamond D. L., Syder A. J., Jacobs J. M., Sorensen C. M., Walters K. A., Proll S. C., et al. (2010). Temporal proteome and lipidome profiles reveal hepatitis C virus-associated reprogramming of hepatocellular metabolism and bioenergetics. PloS Pathog. 6 (1), e1000719. doi: 10.1371/journal.ppat.1000719
Dimitrakopoulou K., Dimitrakopoulos G. N., Wilk E., Tsimpouris C., Sgarbas K. N., Schughart K., et al. (2014). Influenza Aimmunomics and public health omics: the dynamic pathway interplay in host response to H1N1 infection. Omics: J. Integr. Biol. 18 (3), 167–183. doi: 10.1089/omi.2013.0062
Ding Y., Tang J., Guo F. (2017). Identification of drug-target interactions via multiple information integration. Inf. Sci. 418, 546–560. doi: 10.1016/j.ins.2017.08.045
Docea A. O., Tsatsakis A., Albulescu D., Cristea O., Zlatian O., Vinceti M., et al. (2020). A new threat from an old enemy: Re emergence of coronavirus. Int. J. Mol. Med. 45 (6), 1631–1643. doi: 10.3892/ijmm.2020.4555
Dong Q., Li F., Xu Y., Xiao J., Xu Y., Shang D., et al. (2019). RNAactDrug: a comprehensive database of RNAs associated with drug sensitivity from multi-omics data. Briefings Bioinf. doi: 10.1093/bib/bbz142
Donovan B. M., Bastarache L., Turi K. N., Zutter M. M., Hartert T. V. (2019). The current state of omics technologies in the clinical management of asthma and allergic diseases. Ann. Allergy Asthma Immunol. 123 (6), 550–557. doi: 10.1016/j.anai.2019.08.460
D’Amico F., Baumgart D. C., Danese S., Peyrin-Biroulet L. (2020). Diarrhea during COVID-19 infection: pathogenesis, epidemiology, prevention and management. Clin. Gastroenterol. Hepatol. 18, 1663–1672. doi: 10.1016/j.cgh.2020.04.001
Dromey R. G. (1996). Cornering the chimera [software quality]. IEEE Software 13 (1), 33–43. doi: 10.1109/52.476284
Dubey K. K., Luke G. A., Knox C., Kumar P., Pletschke B. II, Singh P. K., et al. (2018). Vaccine and antibody production in plants: developments and computational tools. Briefings Funct. Genomics 17 (5), 295–307. doi: 10.1093/bfgp/ely020
Duncan R., Grigorenko E., Fisher C., Hockman D., Lanning B. (2019). Advances in multiplex nucleic acid diagnostics for blood-borne pathogens: promises and pitfalls-an update. Expert Rev. Mol. Diagnostics 19 (1), 15–25. doi: 10.1080/14737159.2019.1559055
Duncan A. L., Song W., Sansom M. S. (2020). Lipid-dependent regulation of ion channels and G protein-coupled receptors: insights from structures and simulations. Annu. Rev. Pharmacol. Toxicol. 60, 31–50. doi: 10.1146/annurev-pharmtox-010919-023411
Eckhardt M., Hultquist J. F., Kaake R. M., Hüttenhain R., Krogan N. J. (2020). A systems approach to infectious disease. Nat. Rev. Genet. 21, 339–354. doi: 10.1038/s41576-020-0212-5
Eisfeld A. J., Halfmann P. J., Wendler J. P., Kyle J. E., Burnum-Johnson K. E., Peralta Z., et al. (2017). Multi-platform’omics analysis of human Ebola virus disease pathogenesis. Cell host Microbe 22 (6), 817–829. doi: 10.1016/j.chom.2017.10.011
Escobedo-Bonilla C. M. (2013). Application of RNA interference (RNAi) against viral infections in shrimp: a review.
Fanos V., Pintus M. C., Pintus R., Marcialis M. A. (2020). Lung microbiota in the acute respiratory disease: from coronavirus to metabolomics. J. Pediatr. Neonatal Individual. Med. (JPNIM) 9 (1), e090139.
Farag N. S., Breitinger U., Breitinger H. G., El Azizi M. A. (2020). Viroporins and inflammasomes: a key to understand virus-induced inflammation. Int. J. Biochem. Cell Biol. 105738. doi: 10.1016/j.biocel.2020.105738
Franz E., Gras L. M., Dallman T. (2016). Significance of whole genome sequencing for surveillance, source attribution and microbial risk assessment of foodborne pathogens. Curr. Opin. Food Sci. 8, 74–79. doi: 10.1016/j.cofs.2016.04.004
Fritsch L., Guillier L., Augustin J. C. (2018). Next generation quantitative microbiological risk assessment: refinement of the cold smoked salmon-related listeriosis risk model by integrating genomic data. Microbial Risk Anal. 10, 20–27. doi: 10.1016/j.mran.2018.06.003
Fumagalli M., Pozzoli U., Cagliani R., Comi G. P., Bresolin N., Clerici M., et al. (2010). Genome-wide identification of susceptibility alleles for viral infections through a population genetics approach. PloS Genet. 6 (2), e1000849. doi: 10.1371/journal.pgen.1000849
Fung S. Y., Yuen K. S., Ye Z. W., Chan C. P., Jin D. Y. (2020). A tug-of-war between severe acute respiratory syndrome coronavirus 2 and host antiviral defence: lessons from other pathogenic viruses. Emerg. Microbes Infect. 9 (1), 558–570. doi: 10.1080/22221751.2020.1736644
Gale P., Hill A., Kelly L., Bassett J., McClure P., Le Marc Y., et al. (2014). Applications of omics approaches to the development of microbiological risk assessment using RNA virus dose-response models as a case study. J. Appl. Microbiol. 117 (6), 1537–1548. doi: 10.1111/jam.12656
Giamarellos-Bourboulis E. J., Netea M. G., Rovina N., Akinosoglou K., Antoniadou A., Antonakos N., et al. (2020). Complex immune dysregulation in COVID-19 patients with severe respiratory failure. Cell Host Microbe. 16, 992–1000. doi: 10.1016/j.chom.2020.04.009
Grifoni A., Sidney J., Zhang Y., Scheuermann R. H., Peters B., Sette A. (2020). A Sequence Homology and Bioinformatic Approach Can Predict Candidate Targets for Immune Responses to SARS-CoV-2. Cell host Microbe. 27 (4), 671–680.e2. doi: 10.1016/j.chom.2020.03.002
Grossegesse M., Doellinger J., Tyshaieva A., Schaade L., Nitsche A. (2017). Combined Proteomics/Genomics Approach Reveals Proteomic Changes of Mature Virions as a Novel Poxvirus Adaptation Mechanism. Viruses 9 (11):337. doi: 10.3390/v9110337
Guo Y. R., Cao Q. D., Hong Z. S., Tan Y. Y., Chen S. D., Jin H. J., et al. (2020). The origin, transmission and clinical therapies on coronavirus disease 2019 (COVID-19) outbreak-an update on the status. Military Med. Res. 7 (1), 1–10. doi: 10.1186/s40779-020-00240-0
Haas C. T., Roe J. K., Pollara G., Mehta M., Noursadeghi M. (2016). Diagnostic ‘omics’ for active tuberculosis. BMC Med. 14 (1), 37. doi: 10.1186/s12916-016-0583-9
Haas C. N. (2020). Coronavirus and Environmental Engineering Science. Environ. Eng. Sci. 37 (4), 233–234. doi: 10.1089/ees.2020.0096
Haddad N., Johnson N., Kathariou S., Métris A., Phister T., Pielaat A., et al. (2018). Next generation microbiological risk assessment-Potential of omics data for hazard characterisation. Int. J. Food Microbiol. 287, 28–39. doi: 10.1016/j.ijfoodmicro.2018.04.015
Hall D. C., Ji H.-F. (2020). A search for medications to treat COVID-19 via in silicomolecular docking models of the SARS-CoV-2 spike glycoprotein and 3CL protease. Travel Med. Infect. Dis., 101646. doi: 10.1016/j.tmaid.2020.101646
Hampton T. (2020). New Flu Antiviral Candidate May Thwart Drug Resistance. Jama 323 (1), 17–17. doi: 10.1001/jama.2019.20225
Hartzell J. D., Blaylock J. M. (2014). Whooping cough in 2014 and beyond: an update and review. Chest 146 (1), 205–214. doi: 10.1378/chest.13-2942
Hashem A. M., Algaissi A., Agrawal A. S., Al-Amri S. S., Alhabbab R. Y., Sohrab S. S., et al. (2019). A Highly Immunogenic, Protective, and Safe Adenovirus-Based Vaccine Expressing Middle East Respiratory Syndrome Coronavirus S1-CD40L Fusion Protein in a Transgenic Human Dipeptidyl Peptidase 4 Mouse Model. J. Infect. Dis. 220 (10), 1558–1567. doi: 10.1093/infdis/jiz137
Hatcher E. L., Zhdanov S. A., Bao Y., Blinkova O., Nawrocki E. P., Ostapchuck Y., et al. (2017). Virus Variation Resource-improved response to emergent viral outbreaks. Nucleic Acids Res. 45 (D1), D482–D490. doi: 10.1093/nar/gkw1065
He L., Zhu J. (2015). Computational tools for epitope vaccine design and evaluation. Curr. Opin. Virol. 11, 103–112. doi: 10.1016/j.coviro.2015.03.013
He B., Zhang Y., Xu L., Yang W., Yang F., Feng Y., et al. (2014). Identification of diverse alphacoronaviruses and genomic characterization of a novel severe acute respiratory syndrome-like coronavirus from bats in China. J. Virol. 88 (12), 7070–7082. doi: 10.1128/JVI.00631-14
He L., Cheng Y., Kong L., Azadnia P., Giang E., Kim J., et al. (2015). Approaching rational epitope vaccine design for hepatitis C virus with meta-server and multivalent scaffolding. Sci. Rep. 5:12501. doi: 10.1038/srep12501
Hegde N. R., Gauthami S., Sampath Kumar H. M., Bayry J. (2018). The use of databases, data mining and immunoinformatics in vaccinology: where are we? Expert Opin. Drug Discovery 13 (2), 117–130. doi: 10.1080/17460441.2018.1413088
Henry B. M., Vikse J., Benoit S., Favaloro E. J., Lippi G. (2020a). Hyperinflammation and derangement of renin-angiotensin-aldosterone system in COVID-19: A novel hypothesis for clinically suspected hypercoagulopathy and microvascular immunothrombosis. Clinica Chimica Acta 507, 167–173. doi: 10.1016/j.cca.2020.04.027
Henry B. M., De Oliveira M. H. S., Benoit S., Plebani M., Lippi G. (2020b). Hematologic, biochemical and immune biomarker abnormalities associated with severe illness and mortality in coronavirus disease 2019 (COVID-19): a meta-analysis. Clin. Chem. Lab. Med. (CCLM) 58 (7), 1021–1028. doi: 10.1515/cclm-2020-0369
Hoffmann M., Kleine-Weber H., Schroeder S., Krüger N., Herrler T., Erichsen S., et al. (2020). SARS-CoV-2 cell entry depends on ACE2 and TMPRSS2 and is blocked by a clinically proven protease inhibitor. Cell. doi: 10.1016/j.cell.2020.02.052
Hofmann H., Pöhlmann S. (2004). Cellular entry of the SARS coronavirus. Trends Microbiol. 12 (10), 466–472. doi: 10.1016/j.tim.2004.08.008
Holliday R. (1987). The inheritance of epigenetic defects. Science 238 (4824), 163–170. 12(10), 466–472. doi: 10.1126/science.3310230
Holmes L., Enwere M., Williams J., Ogundele B., Chavan P., Piccoli T., et al. (2020). Black-White Risk Differentials in COVID-19 (SARS-COV2) Transmission, Mortality and Case Fatality in the United States: Translational Epidemiologic Perspective and Challenges. Int. J. Environ. Res. Public Health 17 (12):4322. doi: 10.3390/ijerph17124322
Hopkins A. L., Groom C. R. (2002). The druggable genome. Nat. Rev. Drug Discovery 1 (9), 727–730. doi: 10.1038/nrd892
Hu B., Ge X., Wang L. F., Shi Z. (2015). Bat origin of human coronaviruses. Virol. J. 12 (1), 221. doi: 10.1186/s12985-015-0422-1
Hu X., Go Y. M., Jones D. P. (2020). Omics integration for mitochondria systems biology. Antioxid. Redox Signaling 32 (12), 853–872. doi: 10.1089/ars.2019.8006
Huang Y., Haas C. N. (2009). Time-dose-response models for microbial risk assessment. Risk Anal.: Int. J. 29 (5), 648–661. doi: 10.1111/j.1539-6924.2008.01195.x
Huang Y., Lau S. K., Woo P. C., Yuen K. Y. (2007). CoVDB: a comprehensive database for comparative analysis of coronavirus genes and genomes. Nucleic Acids Res. 36 (suppl_1), D504–D511. doi: 10.1093/nar/gkm754
Huang C., Wang Y., Li X., Ren L., Zhao J., Hu Y., et al. (2020). Clinical features of patients infected with 2019 novel coronavirus in Wuhan, China. Lancet 395 (10223), 497–506. doi: 10.1016/S0140-6736(20)30183-5
Ip W. E., Chan K. H., Law H. K., Tso G. H., Kong E. K., Wong W. H., et al. (2005). Mannose-binding lectin in severe acute respiratory syndrome coronavirus infection. J. Infect. Dis. 191 (10), 1697–1704. doi: 10.1086/429631
Irigoyen N., Firth A. E., Jones J. D., Chung B. Y. W., Siddell S. G., Brierley I. (2016). High-resolution analysis of coronavirus gene expression by RNA sequencing and ribosome profiling. PloS Pathog. 12 (2), e1005473. doi: 10.1371/journal.ppat.1005473
Iwata-Yoshikawa N., Okamura T., Shimizu Y., Hasegawa H., Takeda M., Nagata N. (2019). TMPRSS2 contributes to virus spread and immunopathology in the airways of murine models after coronavirus infection. J. Virol. 93 (6), e01815–e01818. doi: 10.1128/JVI.01815-18
Jacob M., Lopata A. L., Dasouki M., Abdel Rahman A. M. (2019). Metabolomics toward personalized medicine. Mass Spectro. Rev. 38 (3), 221–238. doi: 10.1002/mas.21548
Jenkins C., Orsburn B. (2020). In silico approach to accelerate the development of mass spectrometry-based proteomics methods for detection of viral proteins: Application to COVID-19. BioRxiv. doi: 10.1101/2020.03.08.980383
Jeon S., Ko M., Lee J., Choi I., Byun S. Y., Park S., et al. (2020). Identification of antiviral drug candidates against SARS-CoV-2 from FDA-approved drugs. Antimicrob. Agents Chemother. 64 (7), e00819–e00820. doi: 10.1128/AAC.00819-20
Jin J. M., Bai P., He W., Wu F., Liu X. F., Han D. M., et al. (2020). Gender differences in patients with COVID-19: Focus on severity and mortality. Front. Public Health 8:152:152. doi: 10.3389/fpubh.2020.00152
Kaiser A. M., Hogen R., Bordeianou L., Alavi K., Wise P. E., Sudan R. (2015). Clostridium difficile infection from a surgical perspective. J. Gastrointest. Surg. 19 (7), 1363–1377. doi: 10.1007/s11605-015-2785-4
Kang S., Yang M., Hong Z., Zhang L., Huang Z., Chen X., et al. (2020). Crystal structure of SARS-CoV-2 nucleocapsid protein RNA binding domain reveals potential unique drug targeting sites. Acta Pharmaceut. Sin. B. 10 (7), 1228–1238. doi: 10.1016/j.apsb.2020.04.009
Kazi A., Chuah C., Majeed A. B. A., Leow C. H., Lim B. H., Leow C. Y. (2018). Current progress of immunoinformatics approach harnessed for cellular-and antibody-dependent vaccine design. Pathog. Global Health 112 (3), 123–131. doi: 10.1080/20477724.2018.1446773
Kermali M., Khalsa R. K., Pillai K., Ismail Z., Harky A. (2020). The role of biomarkers in diagnosis of COVID-19-A systematic review. Life Sci., 117788. doi: 10.1016/j.lfs.2020.117788
Khachfe H. H., Chahrour M., Sammouri J., Salhab H., Makki B., Fares M. Y. (2020). An epidemiological study on COVID-19: a rapidly spreading disease. Cureus 12 (3), e7313. doi: 10.7759/cureus.7313
Khalili J. S., Zhu H., Mak A., Yan Y., Zhu Y. (2020). Novel coronavirus treatment with ribavirin: Groundwork for evaluation concerning COVID-19. J. Med. Virol. 92, 740–746. doi: 10.1002/jmv.25798
Kobayashi T., Jung S. M., Linton N. M., Kinoshita R., Hayashi K., Miyama T., et al. (2020). Communicating the risk of death from novel coronavirus disease (COVID-19). J. Clin. Med. 9 (2), 580. doi: 10.3390/jcm9020580
Kong Q. P., Bandelt H. J., Sun C., Yao Y. G., Salas A., Achilli A., et al. (2006). Updating the East Asian mtDNA phylogeny: a prerequisite for the identification of pathogenic mutations. Hum. Mol. Genet. 15 (13), 2076–2086. doi: 10.1093/hmg/ddl130
Koutsoukas A., Simms B., Kirchmair J., Bond P. J., Whitmore A. V., Zimmer S., et al. (2011). From in silico target prediction to multi-target drug design: current databases, methods and applications. J. Proteomics 74 (12), 2554–2574. doi: 10.1016/j.jprot.2011.05.011
Lai C. C., Shih T. P., Ko W. C., Tang H. J., Hsueh P. R. (2020). Severe acute respiratory syndrome coronavirus 2 (SARS-CoV-2) and corona virus disease-2019 (COVID-19): the epidemic and the challenges. Int. J. Antimicrob. Agents, 105924. doi: 10.1016/j.ijantimicag.2020.105924
Lan J., Ge J., Yu J., Shan S., Zhou H., Fan S., et al. (2020a). Structure of the SARS-CoV-2 spike receptor-binding domain bound to the ACE2 receptor. Nature, 1–9. doi: 10.1101/2020.02.19.956235
Lan J., Ge J., Yu J., Shan S., Zhou H., Fan S., et al. (2020b). Crystal structure of the 2019-nCoV spike receptor-binding domain bound with the ACE2 receptor. bioRxiv. doi: 10.1101/2020.02.19.956235
Lavecchia A., Cerchia C. (2016). In silico methods to address polypharmacology: current status, applications and future perspectives. Drug Discovery Today 21 (2), 288–298. doi: 10.1016/j.drudis.2015.12.007
Lee J. S., Ruppin E. (2019). Multiomics prediction of response rates to therapies to inhibit programmed cell death 1 and programmed cell death 1 ligand 1. JAMA Oncol. 5 (11), 1614–1618. doi: 10.1001/jamaoncol.2019.2311
Lee S., Son K. Y., Noh Y. H., Lee S. C., Choi H. W., Yoon I. J., et al. (2017). Genetic characteristics, pathogenicity, and immunogenicity associated with cell adaptation of a virulent genotype 2b porcine epidemic diarrhea virus. Vet. Microbiol. 207, 248–258. doi: 10.1016/j.vetmic.2017.06.019
Lee V. S., Chong W. L., Sukumaran S. D., Nimmanpipug P., Letchumanan V., Goh B. H., et al. (2020). Computational screening and identifying binding interaction of anti-viral and anti-malarial drugs: Toward the potential cure for SARS-CoV-2. Prog. Drug Discovery Biomed. Sci. 3 (1), a0000065. doi: 10.36877/pddbs.a0000065
Liang J., Mao G., Yin X., Ma L., Liu L., Bai Y., et al. (2020). Identification and quantification of bacterial genomes carrying antibiotic resistance genes and virulence factor genes for aquatic microbiological risk assessment. Water Res. 168:115160. doi: 10.1016/j.watres.2019.115160
Lighter J., Phillips M., Hochman S., Sterling S., Johnson D., Francois F., et al. (2020). Obesity in patients younger than 60 years is a risk factor for Covid-19 hospital admission. Clin. Infect. Dis. 71(15) 896–897. doi: 10.1093/cid/ciaa415
Liu B., Zheng D., Jin Q., Chen L., Yang J. (2019). VFDB 2019: a comparative pathogenomic platform with an interactive web interface. Nucleic Acids Res. 47 (D1), D687–D692. doi: 10.1093/nar/gky1080
Liu J., Zheng X., Tong Q., Li W., Wang B., Sutter K., et al. (2020). Overlapping and discrete aspects of the pathology and pathogenesis of the emerging human pathogenic coronaviruses SARS-CoV, MERS-CoV, and 2019-nCoV. J. Med. Virol. 92 (5), 491–494. doi: 10.1002/jmv.25709
Liu T., Luo S., Libby P., Shi G. P. (2020). Cathepsin L-selective inhibitors: A potentially promising treatment for COVID-19 patients. Pharmacol. Ther. 213:107587. doi: 10.1016/j.pharmthera.2020.107587
Loging W., Harland L., Williams-Jones B. (2007). High-throughput electronic biology: mining information for drug discovery. Nat. Rev. Drug Discovery 6 (3), 220–230. doi: 10.1038/nrd2265
Losada L., DebRoy C., Radune D., Kim M., Sanka R., Brinkac L., et al. (2016). Whole genome sequencing of diverse Shiga toxin-producing and non-producing Escherichia coli strains reveals a variety of virulence and novel antibiotic resistance plasmids. Plasmid 83, 8–11. doi: 10.1016/j.plasmid.2015.12.001
Lovell S. C., Davis I. W., Arendall W. B., de Bakker P. II, Word J. M., Prisant M. G., et al. (2003). Structure validation by Calpha geometry: phi, psi and Cbeta deviation. Proteins 50, 437e450. doi: 10.1002/prot.10286
Lupberger J., Croonenborghs T., Suarez A. A. R., Van Renne N., Jühling F., Oudot M. A., et al. (2019). Combined analysis of metabolomes, proteomes, and transcriptomes of hepatitis C virus-infected cells and liver to identify pathways associated with disease development. Gastroenterology 157 (2), 537–551. doi: 10.1053/j.gastro.2019.04.003
MacMullan M. A., Dunn Z. S., Graham N., Yang L., Wang P. (2019). Quantitative Proteomics and Metabolomics Reveal Biomarkers of Disease as Potential Immunotherapy Targets and Indicators of Therapeutic Efficacy. Theranostics 9 (25), 7872. doi: 10.7150/thno.37373
Magro C., Mulvey J. J., Berlin D., Nuovo G., Salvatore S., Harp J., et al. (2020). Complement associated microvascular injury and thrombosis in the pathogenesis of severe COVID-19 infection: a report of five cases. Trans. Res. 220, 1–13. doi: 10.1016/j.trsl.2020.04.007
Malik Y. S., Sircar S., Bhat S., Vinodhkumar O. R., Tiwari R., Sah R., et al. (2020). Emerging Coronavirus Disease (COVID-19), a pandemic public health emergency with animal linkages: Current status update. Preprints 2020030343. doi: 10.20944/preprints202003.0343.v1
Manzoni C., Kia D. A., Vandrovcova J., Hardy J., Wood N. W., Lewis P. A., et al. (2018). Genome, transcriptome and proteome: the rise of omics data and their integration in biomedical sciences. Briefings Bioinf. 19 (2), 286–302. doi: 10.1093/bib/bbw114
Marchand-Senécal X., Kozak R., Mubareka S., Salt N., Gubbay J. B., Eshaghi A., et al. (2020). Diagnosis and Management of First Case of COVID-19 in Canada: Lessons applied from SARS. Clin. Infect. Dis. XX(XX):1–4. doi: 10.1093/cid/ciaa227
Mason C. P., Tarr A. W. (2015). Human lectins and their roles in viral infections. Molecules 20 (2), 2229–2271. doi: 10.3390/molecules20022229
McCormick M. (2003). Rats, communications, and plague: toward an ecological history. J. Interdiscip. History 34 (1), 1–25. doi: 10.1162/002219503322645439
Metsky H. C., Freije C. A., Kosoko-Thoroddsen T. S. F., Sabeti P. C., Myhrvold C. (2020). CRISPR-based COVID-19 surveillance using a genomically-comprehensive machine learning approach. bioRxiv. doi: 10.1101/2020.02.26.967026
Mohd H. A., Al-Tawfiq J. A., Memish Z. A. (2016). Middle East respiratory syndrome coronavirus (MERS-CoV) origin and animal reservoir. Virol. J. 13 (1), 87. doi: 10.1186/s12985-016-0544-0
Mouton J. P., Fortuin-de Smidt M. C., Jobanputra N., Mehta U., Stewart A., de Waal R., et al. (2020). Serious adverse drug reactions at two children’s hospitals in South Africa. BMC Pediatr. 20 (1), 3. doi: 10.1186/s12887-019-1892-x
Müller C., Hardt M., Schwudke D., Neuman B. W., Pleschka S., Ziebuhr J. (2018). Inhibition of cytosolic phospholipase A2α impairs an early step of coronavirus replication in cell culture. J. Virol. 92 (4), e01463–e01417. doi: 10.1128/JVI.01463-17
Nagata S., Pastan I. (2009). Removal of B cell epitopes as a practical approach for reducing the immunogenicity of foreign protein-based therapeutics. Advanced Drug Delivery Rev. 61 (11), 977–985. doi: 10.1016/j.addr.2009.07.014
Nasi A., McArdle S., Gaudernack G., Westman G., Melief C., Arens R., et al. (2020). Proteasome and reactive oxygen species dysfunction as risk factors for SARS-CoV infection; consider N-acetylcystein as therapeutic intervention. Toxicol. Rep. 7, 768–771.
Naz A., Obaid A., Shahid F., Dar H. A., Naz K., Ullah N., et al. (2020). Reverse vaccinology and drug target identification through pan-genomics. In Pan-genomics: Applications Challenges Future Prospects, 317–333. doi: 10.1016/B978-0-12-817076-2.00016-0
Nguyen A., David J. K., Maden S. K., Wood M. A., Weeder B. R., Nellore A., et al. (2020). Human leukocyte antigen susceptibility map for SARS-CoV-2. J. Virol. doi: 10.1128/JVI.00510-20
Njage P. M. K., Leekitcharoenphon P., Hald T. (2019). Improving hazard characterization in microbial risk assessment using next generation sequencing data and machine learning: predicting clinical outcomes in shigatoxigenic Escherichia coli. Int. J. Food Microbiol. 292, 72–82. doi: 10.1016/j.ijfoodmicro.2018.11.016
Norgan A. P., Coffman P. K., Kocher J. P. A., Katzmann D. J., Sosa C. P. (2011). Multilevel parallelization of AutoDock 4.2. J. Cheminform. 3 (1):12. doi: 10.1186/1758-2946-3-12
Oany A. R., Emran A. A., Jyoti T. P. (2014). Design of an epitope-based peptide vaccine against spike protein of human coronavirus: an in silico approach. Drug Design Dev. Ther. 8, 1139. doi: 10.2147/DDDT.S67861
Olanya O. M., Hoshide A. K., Ijabadeniyi O. A., Ukuku D. O., Mukhopadhyay S., Niemira B. A., et al. (2019). Cost estimation of listeriosis (Listeria monocytogenes) occurrence in South Africa in 2017 and its food safety implications. Food control 102, 231–239. doi: 10.1016/j.foodcont.2019.02.007
Ong E., Wong M. U., Huffman A., He Y. (2020). COVID-19 coronavirus vaccine design using reverse vaccinology and machine learning. BioRxiv. doi: 10.3389/fimmu.2020.01581
Patankar S. (2020). Deep learning-based computational drug discovery to inhibit the RNA Dependent RNA Polymerase: application to SARS-CoV and COVID-19. OSF Preprints 1–19. doi: 10.31219/osf.io/6kpbg
Pavelić S. K., Markova-Car E., Klobučar M., Sappe L., Spaventi R. (2020). Technological Advances in Preclinical Drug Evaluation: The Role of-Omics Methods. Curr. Med. Chem. 27 (8), 1337–1349.
Pawelek K. A., Huynh G. T., Quinlivan M., Cullinane A., Rong L., Perelson A. S. (2012). Modeling within-host dynamics of influenza virus infection including immune responses. PLoSComputBiol 8 (6), e1002588. doi: 10.1371/journal.pcbi.1002588
Peng D., Liu X. (2017). Research advances in biomarker for sepsis. In Advanced Trauma Surg., 235–251. doi: 10.1007/978-981-10-2425-2_15
Perera K. D., Rathnayake A. D., Liu H., Pedersen N. C., Groutas W. C., Chang K. O., et al. (2019). Characterization of amino acid substitutions in feline coronavirus 3C-like protease from a cat with feline infectious peritonitis treated with a protease inhibitor. Vet. Microbiol. 237:108398. doi: 10.1016/j.vetmic.2019.108398
Perlman S., Netland J. (2009). Coronaviruses post-SARS: update on replication and pathogenesis. Nat. Rev. Microbiol. 7 (6), 439–450. doi: 10.1038/nrmicro2147
Perlman S. (2020). Another decade, another coronavirus. N. Engl. J. Med. 382, 760–762. doi: 10.1056/NEJMe2001126
Pezeshki A., Ovsyannikova I. G., McKinney B. A., Poland G. A., Kennedy R. B. (2019). The role of systems biology approaches in determining molecular signatures for the development of more effective vaccines. Expert Rev. Vaccines 18 (3), 253–267. doi: 10.1080/14760584.2019.1575208
Prompetchara E., Ketloy C., Palaga T. (2020). Immune responses in COVID-19 and potential vaccines: Lessons learned from SARS and MERS epidemic. Asian Pac. J. Allergy Immunol. 38 (1), 1–9.
Pybus O. G., Rambaut A., Belshaw R., Freckleton R. P., Drummond A. J., Holmes E. C. (2007). Phylogenetic evidence for deleterious mutation load in RNA viruses and its contribution to viral evolution. Mol. Biol. Evol. 24 (3), 845–852. doi: 10.1093/molbev/msm001
Qian Z., Dominguez S. R., Holmes K. V. (2013). Role of the spike glycoprotein of human Middle East respiratory syndrome coronavirus (MERS-CoV) in virus entry and syncytia formation. PloS One 8 (10), e76469. doi: 10.1371/journal.pone.0076469
Rahmatizadeh S., Valizadeh-Haghi S., Dabbagh A. (2020). The role of Artificial Intelligence in Management of Critical COVID-19 patients. J. Cell. Mol. Anesthesia 5 (1), 16–22.
Randhawa G. S., Soltysiak M. P., El Roz H., de Souza C. P., Hill K. A., Kari L. (2020). Machine learning using intrinsic genomic signatures for rapid classification of novel pathogens: COVID-19 case study. Plos One 15 (4), e0232391.
Rao V. S., Srinivas K., Sujini G. N., Kumar G. N. (2014). Protein-protein interaction detection: methods and analysis. Int. J. Proteomics. 2014, 12. doi: 10.1155/2014/147648
Rauch S., Jasny E., Schmidt K. E., Petsch B. (2018). New vaccine technologies to combat outbreak situations. Front. Immunol. 9, 1963. doi: 10.3389/fimmu.2018.01963
Riou J., Althaus C. L. (2020). Pattern of early human-to-human transmission of Wuhan 2019 novel coronaviru-nCoV), December 2019 to January 2020. Eurosurveillance 25 (4), 2000058. doi: 10.2807/1560-7917.ES.2020.25.4.2000058
Risco C., Rodrguez J. R., Lpez-Iglesias C., Carrascosa J. L., Esteban M., Rodrguez D. (2002). Endoplasmic reticulum-Golgi intermediate compartment membranes and vimentin filaments participate in vaccinia virus assembly. J. Virol. 76 (4), 1839–1855. doi: 10.1128/jvi.76.4.1839-1855.2002
Rothan H. A., Byrareddy S. N. (2020). The epidemiology and pathogenesis of coronavirus disease (COVID-19) outbreak. J. Autoimmun., 102433. doi: 10.1016/j.jaut.2020.102433
Russell B., Moss C., Rigg A., Van Hemelrijck M. (2020). COVID-19 and treatment with NSAIDs and corticosteroids: should we be limiting their use in the clinical setting? Ecancermedicalscience 14. doi: 10.3332/ecancer.2020.1023
Sadewasser A., Paki K., Eichelbaum K., Bogdanow B., Saenger S., Budt M., et al. (2017). Quantitative proteomic approach identifies Vpr binding protein as novel host factor supporting influenza A virus infections in human cells. Mol. Cell. Proteomics 16 (5), 728–742. doi: 10.1074/mcp.M116.065904
Saif L. J. (2020). Vaccines for COVID-19: perspectives, prospects, and challenges based on candidate SARS, MERS, and animal coronavirus vaccines. Euro. Med. J. doi: 10.33590/emj/200324
Schumacher A., Rujan T., Hoefkens J. (2014). A collaborative approach to develop a multi-omics data analytics platform for translational research. Appl. Trans. Genomics 3 (4), 105–108. doi: 10.1016/j.atg.2014.09.010
Schwartz D. A., Graham A. L. (2020). Potential maternal and infant outcomes from (Wuhan) coronavirus 2019-nCoV infecting pregnant women: Lessons from SARS, MERS, and other human coronavirus infections. Viruses 12 (2):194. doi: 10.3390/v12020194
Seminari E., Colaneri M., Sambo M., Gallazzi I., Di Matteo A., Silvia R., et al. (2020). SARS Cov2 infection in a renal transplanted patients. A case report. Am. J. Transplant.
Silhol F., Sarlon G., Deharo J. C., Vaïsse B. (2020). Downregulation of ACE2 induces overstimulation of the renin-angiotensin system in COVID-19: should we block the renin-angiotensin system? Hypertension Res. 1–3. doi: 10.1038/s41440-020-0476-3
Sinha R., Shukla P. (2019). Protein Engineering for Improved Health: Technological Perspectives. Curr. Protein Pept. Sci. 20 (9), 856–860. doi: 10.2174/138920372009190917095307
Sinha R., Sharma B., Dangi A. K., Shukla P. (2019). Recent metabolomics and gene editing approaches for synthesis of microbial secondary metabolites for drug discovery and development. World J. Microbiol. Biotechnol. 35 (11), 166. doi: 10.1007/s11274-019-2746-2
Smieszek S., Przychodzen B., Polymeropoulos M. H. (2020). Amantadine disrupts lysosomal gene expression; potential therapy for COVID19. bioRxiv. doi: 10.1101/2020.04.05.026187
Sohrabi C., Alsafi Z., O’Neill N., Khan M., Kerwan A., Al-Jabir A., et al. (2020). World Health Organization declares global emergency: A review of the 2019 novel coronavirus (COVID-19). Int. J. Surg. doi: 10.1016/j.ijsu.2020.02.034
Squires R. B., Noronha J., Hunt V., García-Sastre A., Macken C., Baumgarth N., et al. (2012). Influenza research database: an integrated bioinformatics resource for influenza research and surveillance. Influenza other Respir. Viruses 6 (6), 404–416. doi: 10.1111/j.1750-2659.2011.00331.x
Sriram K., Insel P. A. (2018). G protein-coupled receptors as targets for approved drugs: how many targets and how many drugs? Mol. Pharmacol. 93 (4), 251–258. doi: 10.1124/mol.117.111062
Sunita S. A., Singh Y., Shukla P. (2020). Computational tools for modern vaccine development. Hum. Vaccines Immunother. 16 (3), 723–735. doi: 10.1080/21645515.2019.1670035
Tang H. H., Sly P. D., Holt P. G., Holt K. E., Inouye M. (2020). Systems biology and big data in asthma and allergy: recent discoveries and emerging challenges. Eur. Respir. J. 55 (1), 1900844. doi: 10.1183/13993003.00844-2019
To K. K., Lee K. C., Wong S. S., Sze K. H., Ke Y. H., Lui Y. M., et al. (2016). Lipid metabolites as potential diagnostic and prognostic biomarkers for acute community acquired pneumonia. Diagn. Microbiol. Infect. Dis. 85 (2), 249–254. doi: 10.1016/j.diagmicrobio.2016.03.012
Tobaiqy M., Qashqary M., Al-Dahery S., Mujallad A., Hershan A. A., Kamal M. A., et al. (2020). Therapeutic Management of COVID-19 Patients: A systematic review. medRxiv. doi: 10.1101/2020.04.02.20051029
Ulhaq Z. S., Soraya G. V. (2020). Interleukin-6 Is a Potential Biomarker of COVID-19 Progression: Evidence from a Meta-Analysis. Médecine et Maladies Infectieuses 3562887. doi: 10.2139/ssrn.3562887
Uyeki T. M., Erlandson K. J., Korch G., O’Hara M., Wathen M., Hu-Primmer J., et al. (2016). Development of medical countermeasures to Middle East respiratory syndrome coronavirus. Emerg. Infect. Dis. 22 (7), e160022. doi: 10.3201/eid2207.160022
van de Laar L., Saelens W., De Prijck S., Martens L., Scott C. L., Van Isterdael G., et al. (2016). Yolk sac macrophages, fetal liver, and adult monocytes can colonize an empty niche and develop into functional tissue-resident macrophages. Immunity 44 (4), 755–768. doi: 10.1016/j.immuni.2016.02.017
Van Regenmortel M. H. (2019). Development of a preventive HIV vaccine requires solving inverse problems which is unattainable by rational vaccine design. In HIV/AIDS: Immunochem. Reduct. Vaccine Design (Cham: Springer), 283–298.
Vashistha R., Chhabra D., Shukla P. (2018). Integrated artificial intelligence approaches for disease diagnostics. Indian J. Microbiol. 58 (2), 252–255. doi: 10.1007/s12088-018-0708-2
Vera-Velasco N. M., García-Murria M. J., delPino M. M. S., Mingarro I., Martinez-Gil L. (2018). Proteomic composition of Nipah virus-like particles. J. Proteomics 172, 190–200. doi: 10.1016/j.jprot.2017.10.012
Voysey P. A., Brown M. (2000). Microbiological risk assessment: a new approach to food safety control. Int. J. Food Microbiol. 58 (3), 173–179. doi: 10.1016/S0168-1605(00)00271-3
Wallner B. (2005). Protein Structure Prediction: Model Building and Quality Assessment (Doctoral dissertation, Institutionenförbiokemiochbiofysik).
Walls A. C., Park Y. J., Tortorici M. A., Wall A., McGuire A. T., Veesler D. (2020). Structure, function, and antigenicity of the SARS-CoV-2 spike glycoprotein. Cell. 181 (2), 281–292.e6. doi: 10.1016/j.cell.2020.02.058
Wang Y., Zhu L. Q. (2020). Pharmaceutical care recommendations for antiviral treatments in children with coronavirus disease 2019. World J. Pediatr. 1–4. doi: 10.1007/s12519-020-00353-5
Wang W., Zhang W., Zhang J., He J., Zhu F. (2020). Distribution of HLA allele frequencies in 82 Chinese individuals with coronavirus disease-2019. HLA 96 (2), 194–196.
Wang H., Yang P., Liu K., Guo F., Zhang Y., Zhang G., et al. (2008). SARS coronavirus entry into host cells through a novel clathrin-and caveolae-independent endocytic pathway. Cell Res. 18 (2), 290–301. doi: 10.1038/cr.2008.15
Wang Z., Gerstein M., Snyder M. (2009). RNA-Seq: a revolutionary tool for transcriptomics. Nat. Rev. Genet. 10 (1), 57–63. doi: 10.1038/nrg2484
Wang F., Nie J., Wang H., Zhao Q., Xiong Y., Deng L., et al. (2020). Characteristics of peripheral lymphocyte subset alteration in COVID-19 pneumonia. J. Infect. Dis. 221, 1762–1769. doi: 10.1093/infdis/jiaa150
Wang M., Cao R., Zhang L., Yang X., Liu J., Xu M., et al (2020). Remdesivir and chloroquine effectively inhibit the recently emerged novel coronavirus, (2019-nCoV) in vitro. Cell Res. 30 (3), 269–271. doi: 10.1038/s41422-020-0282-0
Wang X., Dhindsa R., Povysil G., Zoghbi A., Motelow J., Hostyk J., et al. (2020). Transcriptional Inhibition of Host Viral Entry Proteins as a Therapeutic Strategy for SARS-CoV-2. Preprints 2020030360. doi: 10.20944/preprints202003.0360.v1
Wassenaar T. M., Gamieldien J., Shatkin J., Luber P., Moyer N., Carpenter T., et al. (2007). The importance of virulence prediction and gene networks in microbial risk assessment. Hum. Ecol. Risk Assess. 13 (2), 254–268. doi: 10.1080/10807030701226855
Waterhouse A., Bertoni M., Bienert S., Studer G., Tauriello G., Gumienny R., et al. (2018). SWISS-MODEL: homology modelling of protein structures and complexes. Nucleic Acids Res. 46 (W1), W296–W303. doi: 10.1093/nar/gky427
Weißbarth G., Wiesen M. H., Fietz C., Streichert T., Ehren R., Weber L. T., et al. (2020). Pharmacodynamic Monitoring of Mycophenolic Acid Therapy: Improved Liquid Chromatography-Tandem Mass Spectrometry Method for Measuring Inosin-5’-Monophosphate Dehydrogenase Activity. Ther. Drug Monit. 42 (2), 282–288. doi: 10.1097/FTD.0000000000000688
Wernery U., Lau S. K., Woo P. C. (2017). Middle East respiratory syndrome (MERS) coronavirus and dromedaries. Vet. J. 220, 75–79. doi: 10.1016/j.tvjl.2016.12.020
Wiederstein M., Sippl M. J. (2007). ProSA-web: interactive web service for the recognition of errors in three-dimensional structures of proteins. Nucleic Acids Res. 35 (suppl_2), W407–W410. doi: 10.1093/nar/gkm290
Winnenburg R., Urban M., Beacham A., Baldwin T. K., Holland S., Lindeberg M., et al. (2007). PHI-base update: additions to the pathogen-host interaction database. Nucleic Acids Res. 36 (suppl_1), D572–D576. doi: 10.1093/nar/gkm858
Woo V., Alenghat T. (2017). Host-microbiota interactions: epigenomic regulation. Curr. Opin. Immunol. 44, 52–60. doi: 10.1016/j.coi.2016.12.001
Wu B., Ricchetti F., Sanguineti G., Kazhdan M., Simari P., Jacques R. (2011). Data-driven approach to generating achievable dose-volume histogram objectives in intensity-modulated radiotherapy planning. Int. J. Radiat. Oncol. Biol. Phys. 79 (4), 1241–1247. doi: 10.1016/j.ijrobp.2010.05.026
Wu C., Chen X., Cai Y., Zhou X., Xu S., Huang H., et al. (2020a). Risk factors associated with acute respiratory distress syndrome and death in patients with coronavirus disease 2019 pneumonia in Wuhan, China. JAMA Internal Med. doi: 10.1001/jamainternmed.2020.0994
Wu C., Liu Y., Yang Y., Zhang P., Zhong W., Wang Y., et al. (2020b). Analysis of therapeutic targets for SARS-CoV-2 and discovery of potential drugs by computational methods. Acta Pharmaceutica Sinica B. doi: 10.1016/j.apsb.2020.02.008
Xia S., Liu M., Wang C., Xu W., Lan Q., Feng S., et al. (2020). Inhibition of SARS-CoV-2 (previously 2019-nCoV) infection by a highly potent pan-coronavirus fusion inhibitor targeting its spike protein that harbors a high capacity to mediate membrane fusion. Cell Res., 1–13. doi: 10.1038/s41422-020-0305-x
Xie Q., Fan F., Wei W., Liu Y., Xu Z., Zhai L., et al. (2017). Multi-omics analyses reveal metabolic alterations regulated by hepatitis B virus core protein in hepatocellular carcinoma cells. Sci. Rep. 7 (1), 1–12. doi: 10.1038/srep41089
Yan B., Chu H., Yang D., Sze K. H., Lai P. M., Yuan S., et al. (2019). Characterization of the lipidomic profile of human coronavirus-infected cells: Implications for lipid metabolism remodeling upon coronavirus replication. Viruses 11 (1):73. doi: 10.3390/v11010073
Yan R., Zhang Y., Li Y., Xia L., Guo Y., Zhou Q. (2020). Structural basis for the recognition of SARS-CoV-2 by full-length human ACE2. Science 367 (6485), 1444–1448. doi: 10.1126/science.abb2762
Yang P., Wang X. (2020). COVID-19: a new challenge for human beings. Cell. Mol. Immunol. 17 (5), 555–557. doi: 10.1038/s41423-020-0407-x
Yang J., Zheng Y., Gou X., Pu K., Chen Z., Guo Q., et al. (2020). Prevalence of comorbidities in the novel Wuhan coronavirus (COVID-19) infection: a systematic review and meta-analysis. Int. J. Infect. Dis. 16, 1708–1717. doi: 10.1016/j.ijid.2020.03.017
Yang Y., Islam M. S., Wang J., Li Y., Chen X. (2020). Traditional Chinese Medicine in the Treatment of Patients Infected with 2019-New Coronavirus (SARS-CoV-2): A Review and Perspective. Int. J. Biol. Sci. 16 (10), 1708. doi: 10.7150/ijbs.45538
Ye Q., Wang B., Mao J. (2020). The pathogenesis and treatment of theCytokineStorm’in COVID-19. J. Infect. 80 (6), 607–613. doi: 10.1016/j.jinf.2020.03.037
Yuen K. S., Ye Z. W., Fung S. Y., Chan C. P., Jin D. Y. (2020). SARS-CoV-2 and COVID-19: The most important research questions. Cell Biosci. 10 (1), 1–5. doi: 10.1186/s13578-020-00404-4
Zhang L., Liu Y. (2020). Potential interventions for novel coronavirus in China: a systemic review. J. Med. Virol. 92 (5), 479–490. doi: 10.1002/jmv.25707
Zhang T., Zhang L., Payne P. R., Li F. (2018). Synergistic drug combination prediction by integrating multi-omics data in deep learning models. arXiv. preprint arXiv:1811.07054.
Zhang D., Tang Z., Huang H., Zhou G., Cui C., Weng Y., et al. (2019). Metabolic regulation of gene expression by histone lactylation. Nature 574 (7779), 575–580. doi: 10.1038/s41586-019-1678-1
Zhang H., Penninger J. M., Li Y., Zhong N., Slutsky A. S. (2020). Angiotensin-converting enzyme 2 (ACE2) as a SARS-CoV-2 receptor: molecular mechanisms and potential therapeutic target. Intensive Care Med. 1–5. doi: 10.1007/s00134-020-05985-9
Zhao Y. Y., Lin R. C. (2014). UPLC-MSE application in disease biomarker discovery: the discoveries in proteomics to metabolomics. Chemico-Biol. Interact. 215, 7–16. doi: 10.1016/j.cbi.2014.02.014
Zheng J., Perlman S. (2018). Immune responses in influenza A virus and human coronavirus infections: an ongoing battle between the virus and host. Curr. Opin. Virol. 28, 43–52. doi: 10.1016/j.coviro.2017.11.002
Zheng J., Yamada Y., Fung T. S., Huang M., Chia R., Liu D. X. (2018). Identification of N-linked glycosylation sites in the spike protein and their functional impact on the replication and infectivity of coronavirus infectious bronchitis virus in cell culture. Virology 513, 65–74. doi: 10.1016/j.virol.2017.10.003
Zhou F., Yu T., Du R., Fan G., Liu Y., Liu Z., et al. (2020). Clinical course and risk factors for mortality of adult inpatients with COVID-19 in Wuhan, China: a retrospective cohort study. Lancet 395, 1054–1062. doi: 10.1016/S0140-6736(20)30566-3
Zhu Z., Chan J. F. W., Tee K. M., Choi G. K. Y., Lau S. K. P., Woo P. C. Y., et al. (2016). Comparative genomic analysis of pre-epidemic and epidemic Zika virus strains for virological factors potentially associated with the rapidly expanding epidemic. Emerg. Microbes Infect. 5 (1), 1–12. doi: 10.1038/emi.2016.48
Zhu N., Zhang D., Wang W., Li X., Yang B., Song J., et al. (2020). A novel coronavirus from patients with pneumonia in China 2019. New Engl. J. Med. 382, 727–733. doi: 10.1056/NEJMoa2001017
Ziegler C. G., Allon S. J., Nyquist S. K., Mbano I. M., Miao V. N., Tzouanas C. N., et al. (2020). SARS-CoV-2 receptor ACE2 is an interferon-stimulated gene in human airway epithelial cells and is detected in specific cell subsets across tissues. Cell. 181, 1016–1035. doi: 10.1016/j.cell.2020.04.035
Keywords: systems biology, multiomics, in silico, database (DB), COVID-19, coronavirus, pathogenicity
Citation: Jaiswal S, Kumar M, Mandeep, Sunita, Singh Y and Shukla P (2020) Systems Biology Approaches for Therapeutics Development Against COVID-19. Front. Cell. Infect. Microbiol. 10:560240. doi: 10.3389/fcimb.2020.560240
Received: 11 May 2020; Accepted: 29 September 2020;
Published: 28 October 2020.
Edited by:
Rodolfo García-Contreras, National Autonomous University of Mexico, MexicoReviewed by:
Hector Quezada, Hospital Infantil de México Federico Gómez, MexicoCopyright © 2020 Jaiswal, Kumar, Mandeep, Sunita, Singh and Shukla. This is an open-access article distributed under the terms of the Creative Commons Attribution License (CC BY). The use, distribution or reproduction in other forums is permitted, provided the original author(s) and the copyright owner(s) are credited and that the original publication in this journal is cited, in accordance with accepted academic practice. No use, distribution or reproduction is permitted which does not comply with these terms.
*Correspondence: Pratyoosh Shukla, cHJhdHlvb3NoLnNodWtsYUBnbWFpbC5jb20=; orcid.org/0000-0002-9307-4126
Disclaimer: All claims expressed in this article are solely those of the authors and do not necessarily represent those of their affiliated organizations, or those of the publisher, the editors and the reviewers. Any product that may be evaluated in this article or claim that may be made by its manufacturer is not guaranteed or endorsed by the publisher.
Research integrity at Frontiers
Learn more about the work of our research integrity team to safeguard the quality of each article we publish.