- Beijing Key Laboratory for Drug Resistant Tuberculosis Research, Beijing Tuberculosis and Thoracic Tumor Research Institute, Beijing Chest Hospital, Capital Medical University, Beijing, China
Tuberculosis (TB) is still a serious threat to human health which is caused by mycobacterium tuberculosis (Mtb). The main reason for failure to eliminate TB is lack of clearly understanding the molecular mechanism of Mtb pathogenesis. Determining human Mtb-interacting proteins enables us to characterize the mechanism and identify potential molecular targets for TB diagnosis and treatment. However, experimentally systematic Mtb interactors are not readily available. In this study, we performed an unbiased, comprehensive two-way proteome microarray based approach to systematically screen global human Mtb interactors and determine the binding partners of Mtb effectors. Our results, for the first time, screened 84 potential human Mtb interactors. Bioinformatic analysis further highlighted these protein candidates might engage in a wide range of cellular functions such as activation of DNA endogenous promoters, transcription of DNA/RNA and necrosis, as well as immune-related signaling pathways. Then, using Mtb proteome microarray followed His tagged pull-down assay and Co-IP, we identified one interacting partner (Rv0577) for the protein candidate NRF1 and three binding partners (Rv0577, Rv2117, Rv2423) for SMAD2, respectively. This study gives new insights into the profile of global Mtb interactors potentially involved in Mtb pathogenesis and demonstrates a powerful strategy in the discovery of Mtb effectors.
Introduction
Tuberculosis (TB) is a chronic infectious disease caused by the intracellular bacterial pathogen Mycobacterium tuberculosis (Mtb). According to a WHO report, there were an estimated 10.4 million new cases of TB and 1.67 million TB-related deaths in 2016 (World Health Organization (WHO), 2017). Moreover, emergence of multi-drug resistant (MDR) and extensively-drug resistant (XDR) strains of Mycobacterium, as well as co-infection with retroviruses like HIV has further complicated TB treatment (Goldberg et al., 2012; Chang and Yew, 2013). To overcome this menace, comprehensive understanding of the molecular crosstalk between the invading pathogen and its human host will be extremely important to identify targets for the effective treatment of TB.
Mtb is one of the most successful pathogens that can survive and replicate within the macrophages of infected people (Cambier et al., 2014). After invasion of Mtb, macrophages can generate an immune response that utilizes effectors such as anti-bacterial peptides, hydrolases, and toxic reactive oxygen and nitrogen intermediates (Alipoor et al., 2016). In addition to macrophages, T-cells have been also shown to be involved in host cell immune response against Mtb (Gonzalez-Juarrero and Orme, 2001). However, Mycobacterium has developed multiple strategies to evade elimination by the host immune response and causes disease, such as arrest of phagosome-lysosome fusion, inhibition of apoptotic/autophagic pathways of invading macrophages, and promotion of neutralization of reactive nitrogen and oxygen intermediates (Russell, 2001; Gutierrez et al., 2004; Flannagan et al., 2009).
The protein-protein interactions (PPI) between Mtb and the host cell are often involved in the bacteria's pathogenesis and persistence in the host (Konig et al., 2008; Dyer et al., 2010). However, such systematic inter-species PPI are not readily available. Experimental studies have uncovered and characterized a few PPI between Mtb and humans (Table S1) (Mueller-Ortiz et al., 2001; Vergne et al., 2005; Deghmane et al., 2007; Bach et al., 2008; Danelishvili et al., 2010; Sun et al., 2010; Wong et al., 2011; Byun et al., 2012; Kim et al., 2012; Mehra et al., 2013; Sreejit et al., 2014; Wang et al., 2015, 2016; Dziadek et al., 2016). There are two main approaches applied to discover these interactions, including yeast two-hybrid (Y2H) system and affinity pull-down (AP) coupled with mass spectrometry (MS) (Jäger et al., 2011; Mehra et al., 2013; Rajagopala et al., 2014; Dziadek et al., 2016). However, the sensitivity of Y2H is estimated at only ~20%, and AP/MS can detect proteins in complexes but is unable to distinguish direct from indirect interactions when several proteins are purified together. Furthermore, these methods are time-consuming and expensive, especially when adopted in high-throughput mode (Liu et al., 2012). Recently, several computational approaches have been developed to predict host-pathogen PPI based on the sequence method of homology, interologs, interacting domain/motif, and structure (Rapanoel et al., 2013; Zhou et al., 2013). However, most of these works lack stringent verification. Thus, the accuracy of computational approaches in predicting host-pathogen PPI is largely unknown.
In this study, we attempt to fill this gap by identifying the global human interactors with Mtb using an unbiased, comprehensive protein-chip based approach and demonstrating a powerful strategy in the discovery of Mb effectors. Our results could provide potential targets for a further study of pathogenesis of Mtb and drug development for TB.
Experimental Procedures
Mtb Secreted Protein (SP) and Cellular Protein (CP) Sample Preparation
Mtb SP and CP were extracted as described by Andersen and his colleagues with minor modifications (Andersen et al., 1991). In short, Mtb H37Rv were cultured in Middlebrook 7H9 broth (BD-Defico, USA) added with 10% OADC and 0.05% Tween 80 until the logarithmic phase (usually 3 weeks). The bacteria were collected and heat inactivated at 90°C for 30 min. The cellular proteins were extracted by using multigelation for 3 times and ultrasonication under conditions of 800 W, 2 s/2 s for 40 min followed by centrifugation and collection of supernatant. The cultures were centrifuged and the supernatants were sterile filtered. After concentration using Amicon ultra-15 (3 kDa) (Millipore, USA), the supernatants were extensively washed in PBS while still in ultrafiltration cell. The protein content was determined by coomassie brilliant blue staining. Then SP and CP were diluted to 0.3 mg/ml and labeled with CyDye Protein Labeling Cy3/Cy5 (GE-Healthcare, USA) respectively. Two microliters of the proteins were loaded on Nitrocellulose(NC) membrane followed by BSA blocking and PBS washing, then the signal was detected by a fluorescent scanner to evaluate the laveling efficiency.
Human Proteome Microarray Fabrication
HuProt™ version 3.0 microarray were purchased from CDI Laboratories Inc (USA). It contains more than 19,000 unique proteins which encompasses 15,581 unique human genes and 124 unique mouse gene symbols. Recombinant proteins are expressed in the yeast S. cerevisiae, purified, and printed on glass slides in duplicate, Control proteins, including histone H1, histone H2, histone H3, histone H4, BSA, and biotinylated BSA, GST, IgM, IgG were also spotted in duplicate. The printed microarrays were stored at −80°C prior to use.
Identification of Mtb SP Interactors (SPI) and CP Interactors (CPI) Using the Human Proteome Microarrays
Proteome microarrays were blocked with blocking buffer (5% BSA in 0.1% Tween 20 TBST) for 1.5 h at room temperature with gentle agitation. SP and CP samples were diluted to 1 μg/ml and incubated on the proteome microarray at room temperature for 2 h. The microarrays were each washed with TBST three times for 5 min, and followed by three quick washes in ddH2O. The microarrays were spun dry at 250 g for 3 min and were scanned with a GenePix 4200A microarray scanner (Molecular Devices, CA, USA) to visualize and record the results. The proteome microarray data were extracted with GenePix Pro 6.0 (Molecular Devices, CA, USA) and processed as previously described (Wang et al., 2012).
Bioinformatic Analysis of Human Mtb Interactors
The protein candidates were classified using the PANTHER classification system with default settings (Mi et al., 2007). To identify the enrichment of the candidates, the potential candidates were analyzed using DAVID 6.8 (Huang et al., 2009a,b). Significantly enriched categories in the subontology of the KEGG pathway with a P value < 0.05 were chosen. We also performed analysis on functions, pathways and networks using the Ingenuity Pathway Analysis (IPA) software (https://www.qiagenbioinformatics.com), which were evaluated by p-value (p-value < 0.05). The protein symbol of each candidate was used as input data.
Identification the Mtb Effectors Using Mtb Proteome Microarray
To identify the Mtb targets of these human interactors, representative proteins SMAD2 and NRF1 were cloned into the pCMV-C-myc vector, respectively, and purified as previously reported (Li et al., 2013). The protein concentration was determined by standard silver staining. Then the myc-tagged SMAD2 and NRF1 were labeled with Cy3 and diluted to 4 μg/ml. Mtb proteome microarrays (BC Biotech, China) were blocked for 3 h at 4°C with shaking in blocking buffer (3% BSA in PBS). The protein samples were incubated with microarrays overnight at 4°C with shaking, then washed three times in PBS (pH 7.4) with 0.1% Tween 20 (PBST) followed by twice ddH2O quick washes. Microarrays were spun dry at 250 g for 3 min and then scanned with a GenePix 4200A microarray scanner. Data were analyzed with GenePix Pro 6.0 and processed as previously described (Jeong et al., 2012).
His Tagged Pull-Down Assay and Co-Immunoprecipitation
To validate the interactions between screened Mtb effectors and human SMAD2 or NRF1, His tagged Mtb proteins were purified as previously described (Biertümpfel et al., 2010). PMA-differentiated THP-1 cells lysed in HEPEs lysis buffer (50 mM HEPEs pH 7.5, 150 mM NaCl, 1 mM EDTA, 1 mM EGTA, 10% Glycerol, 1% TritonX-100, 10 μM ZnCl2) supplemented with 0.5 mM PMSF (Sigma-Aldrich, Saint Louis, USA). The His pull-down were performed as previously described (Zhang et al., 2009). Protein separation and detection were performed using an automated capillary electrophoresis system (Simple Western system and Compass software; proteinsimple; USA). Antibodies against the following proteins were used; anti-NRF1 (#46743, CST, 1:50), anti-SMAD2 (#5339, CST, 1:500) and anti-His-tag (#12698, CST, 1:100). Signals were detected by HRP-conjugated secondary anti-rabbit antibody and were visualized using Compass software. For Co-IP, Flag-Rv2117, -Rv2423, and -Rv0577 were overexpressed in HEK293T cells and the whole-cell lysates were immunoprecipitated with anti-Flag M2 agarose (Sigma, St Louis, MO, USA) as described previously(Guo et al., 2003). Samples were separated by SDS-PAGE and detected by immunoblotting with antibodies anti-Flag M2 (#14793, CST, 1:1000), anti-SMAD2 (1:2000) and anti-NRF1 (1:2000).
Experimental Design and Statistical Rationale
The experiment was designed to conduct two separated proteome microarray assays. The proteome microarray data were extracted with GenePix Pro 6.0 and processed as previously reported (Jeong et al., 2012; Wang et al., 2012). For human proteome microarray data analysis, the signal-to-noise ratio (SNR) was defined as the ratio of median of foreground signal (F-median) deducted of median of Background signal (B-median) to B-median [(F-median-B-median)/B-median] and was calculated for each protein. The SNR of each protein was averaged for the two duplicated spots. To call the final potential candidates, the cut-off value was set as SNR ≥ 2. For Mtb proteome microarray data analysis, the signal to noise ratio (SNR) was defined as F-median/B-median. Z-score of the proteins was calculated to normalize signal intensity for any pair of spots. To call the positive binding partners, the cutoff was set as SNR ≥ 1.4 fold above the background and Z-score ≥ 3.0.
Results
Screening of Human Proteins Interacted With Mtb Proteins Using Human Proteome Microarray
In order to globally identify human proteins that directly interacted with Mtb proteins, we designed the experiment as shown in Figure 1. Briefly, The Mtb SP and CP were prepared and labeled with distinct fluorescein, respectively. After being incubated with human proteome microarray, the potential Mtb interactors were screened. To call for Mtb effectors, human proteins were expressed and purified in vitro, reversely incubated with Mtb proteome microarray to find out its binding partners in Mtb. Further validation of His pull-down assay and Co-IP were performed to verify these interactions between Mtb effectors and its human interactors so as to identify the Mtb-host PPI.
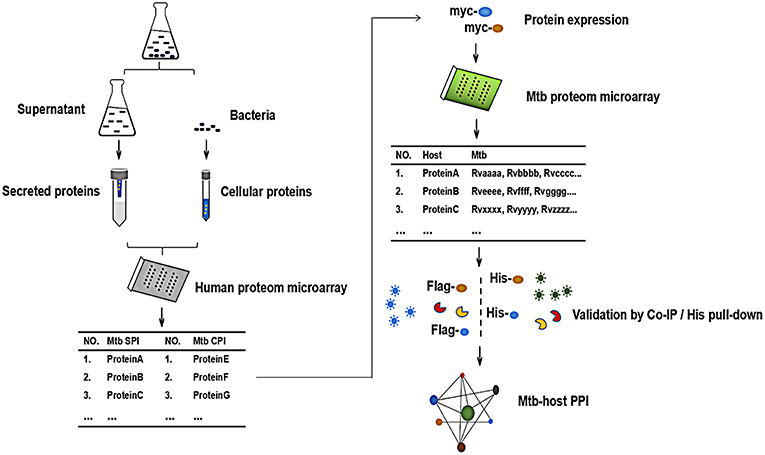
Figure 1. The schematic diagram of the proteome microarray strategy for identification of Mtb-human PPI. Briefly, the Mtb secreted protein (SP) and cellular protein (CP) were prepared and labeled with distinct fluorescein, respectively. After incubated with human proteome microarray, the potential Mtb interactors were screened. To call for Mtb effectors, human proteins were expressed and purified in vitro, reversely incubated with Mtb proteome microarray. Following experimental validation by His pull-down assay and Co-IP determined the interactions and the Mtb-host PPI.
Mtb membrane proteins and secreted proteins are important effectors that may play important roles in host-pathogen interactions (Poirier and Av-Gay, 2012). To harvest all the Mtb proteins, we isolated Mtb SP from the supernatant of bacterial cultures and CP from the cell pellet, respectively (Figure 2A). After labeling with Cy3 or Cy5, the SP and CP were incubated with a human proteome microarray with 15,581 individually affinity-purified N-terminal GST-tagged human proteins. The signals at 532 nm and 647 nm were collected, and the reproducibility of the duplicate spots was assessed. To screen the potential human Mtb interactors, the cut-off was set as the signal-to-noise ratio (SNR) > 2. In this way, we identified 54 Mtb secreted protein interactors (SPI) and 74 human Mtb cellular protein interactors (CPI) with 44 proteins in common between the two parts and 84 proteins in total as potential Mtb interactors (Figure 2B). Four representatives of the potential interactors are shown in Figure 2C. Notably, there were 10 SP-unique interactors and 30 CP-unique interactors (Supplementary Figure 1), which suggested different regulatory roles for Mtb SP and CP during Mtb invasion of host cells.
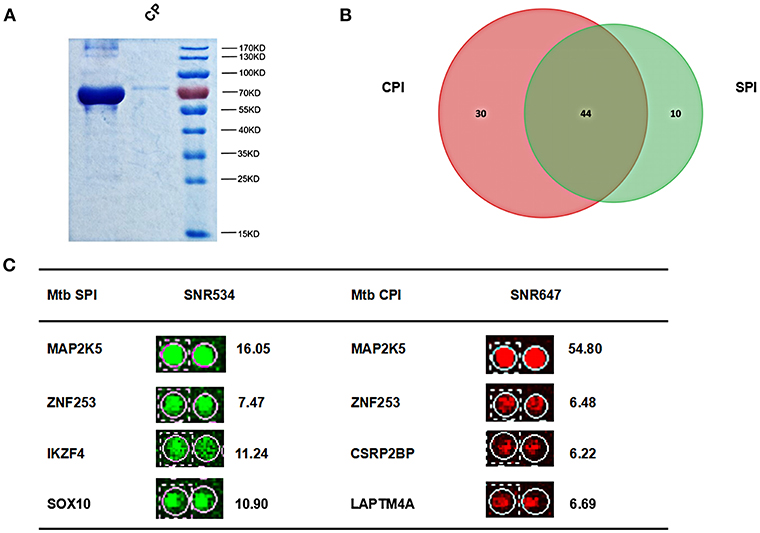
Figure 2. Identification of Mtb SPI and CPI on a human proteome microarray. (A) Extraction and purification of Mtb SP and CP. The proteins were detected by SDS-PAGE followed by coomassie brilliant blue staining. (B) The Venn diagram of Mtb SPI (green) and CPI (red). (C) Representatives of the newly identified Mtb SPI and CPI. The cutoff for calling positive candidates was set as signal-to-noise (SNR) ratio > 2.0. Experiments were performed in two replicates.
Categorization and Go Analysis of the Mtb SPI and CPI
Applying the online PANTHER classification system to the newly defined Mtb potential interactors, we determined the classification for protein class, specific biological process (BP) and molecular function (MF). The CPI could be classified into 11 protein classes, covering 6 items categorized for SPI (Figure 3A). The top two protein classes were transcription factor (PC00218) and nucleic acid binding (PC00171) for both SPI and CPI. Besides, the SPI could also be classified into 8 groups of BP, while additional 2 groups including cellular component organization or biogenesis (GO:0071840) and biological adhesion (GO:0022610) were defined in BP categories of CPI. The top three classes are cellular process (GO:0009987), biological regulation (GO:0065007), and metabolic process (GO:0008152), counting for 26.2, 23.8, and 21.4% in SPI and 27.9, 19.8, and 22.5% in CPI, respectively. As expected, response to stimulus (GO:0050896) and immune system process (GO:0002376) were defined in BP classes of both SPI and CPI (Figure 3C). Finally, the candidates of SPI could be defined as 4 classes of MF, which were also found in CPI, however, CPI had one more category marked as transporter activity (GO:0005215). Except for the top one MF class that was binding (GO:0005488), more candidates of CPI (23.1%) play roles in catalytic activity (GO:0003824) compared with those of SPI (6.3%) (Figure 3B). Next, we carried out gene ontology analysis by using DAVID as mentioned in EXPERIMENTAL PROCEDURES. Interestingly, we found that Mtb SP- and CP-unique interactors specifically preferred to bind with host transcription regulators, but they enriched into reverse GO BP terms (Table 1). Among the SP-unique interactors, PPARδ, BARX2, IRF2, MBD2, FOXD3 were enriched for term of negative regulation of transcription from RNA polymerase II (GO:0000122). While Mtb CP-unique interactors were significantly enriched for term of positive regulation of transcription (GO:GO:0045893). These results suggested that Mtb SP could arise most biological responses in host as part of the whole bacterial components. The different regulation of SP- and CP-unique interactors in host transcription might give some clues on the sequential and spatial regulatory role of Mtb after infection, battle with host immune system and finally persistence or death.
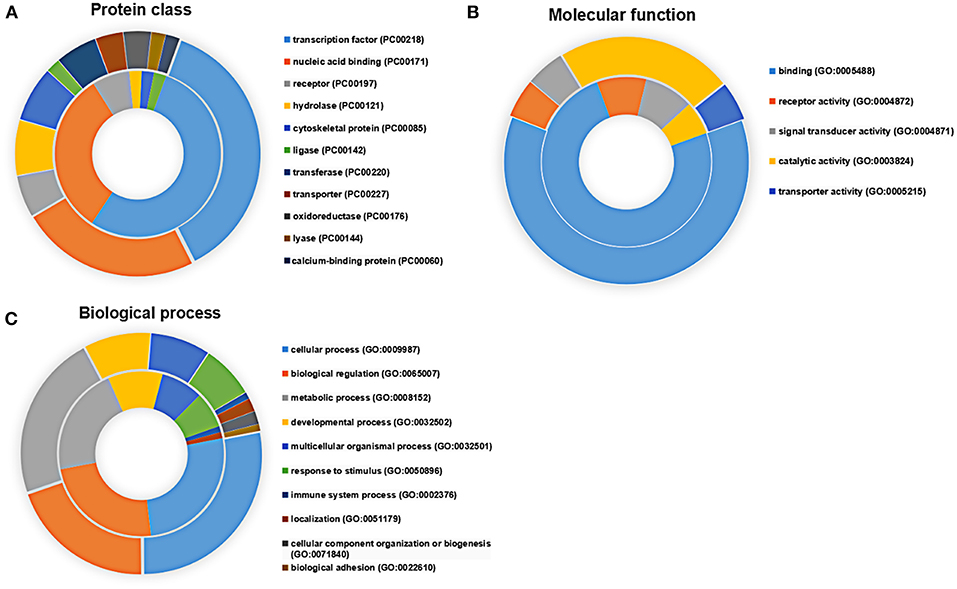
Figure 3. Functional distribution of the SPI (inner circle) and CPI (outer circle) Protein candidates were classified according to their (A) protein class, (B) molecular function, and (C) biological process using the PANTHER classification system (Mi et al., 2007) with default settings. Gene symbols were used as input.
Function, Pathway and Network Analysis of the Global Mtb Potential Interactors
Using Ingenuity Pathway Analysis (IPA) software, we further gained an insight into the role for the global Mtb potential interactors in canonical pathways, cellular functions and networks. In canonical pathway analysis, these proteins were involved in the most significant item of “TGF-β signaling” which was responsible for early inhibition to facilitate Mtb clearance (Feruglio et al., 2017). Besides, other key immune-related signaling pathways were also defined such as “regulation of IL-2 expression in activated and anergic T Lymphocytes,” “IL-22 signaling,” “role of JAK family kinases in IL-6-type cytosine signaling,” and “antiproliferative role of TOB in T cell signaling.” The top 20 canonical pathway items could be found in Figure 4A and Table S2. Among all the IPA “Diseases and Bio Functions” items, the top 10 significantly changed items were showed and listed in Figure 4B and Table S3, which covered the two IPA categories including the “Molecular and Cellular Functions” and “Physiological System Development and Function.”
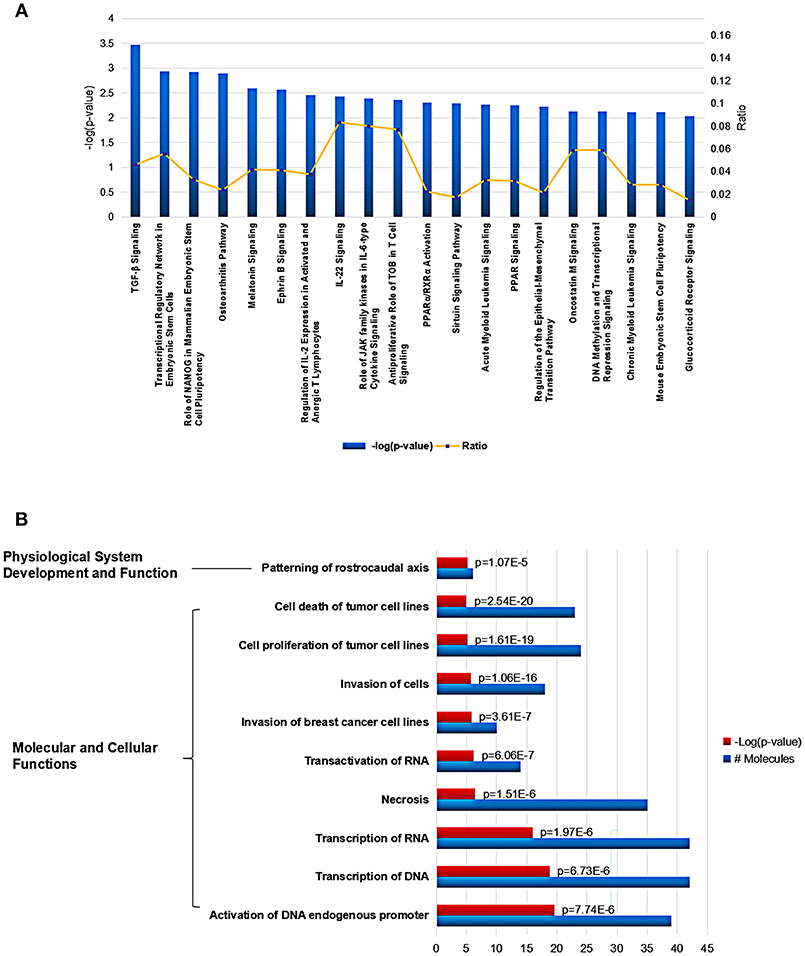
Figure 4. Cellular function and pathway analysis of the global Mtb interactors using IPA software. (A) The IPA canonical pathway analysis showed 20 significantly changed pathways (p-value < 0.05). (B) The top-10 significantly changed items covered the two IPA categories including the “Molecular and Cellular Functions,” and “Physiological System Development and Function”.
In addition, various networks were found to engage for the human Mtb interactors, the top three networks involved “Gene Expression, Embryonic Development, Organismal Development,” “Cellular Assembly and Organization, Endocrine System Disorders, Gastrointestinal Disease,” and “Gene Expression, Carbohydrate Metabolism, Small Molecule Biochemistry” (Table S4). The top five tox lists engaged “TGF-signaling,” “Liver Proliferation,” “Renal Necrosis/Cell Death,” “PARP/RXR Activation,” and “Mechanism of Gene Regulation by Peroxisome Proliferators via PPAR” (Table S5).
Identification of Mtb Effectors Binding With Representative Human Protein Candidates
To identify the Mtb effectors interacting with host protein candidates, we selected two of the candidates NRF1 and SMAD2 as bait proteins (Supplementary Figure 2), which were both detected to be positive interactors with Mtb CP as well as SP with high SNR signals (SNR > 4). Meanwhile, SMAD2 was listed as one of the genes that were significantly enriched in the top 1 of IPA pathway item of “TGF-β Signaling” (Table S2), and NRF1 played an important role in the top 1 of IPA cellular function of “Activation of DNA endogenous promoter” (Table S3). As transcriptional factors, they play important roles in regulating many functional genes' expressions involved in various biological pathways, including stress adaptation and immune homeostasis (Sykiotis and Bohmann, 2010; Sekiya et al., 2016). First, myc-tagged NRF1 and SMAD2 were constructed, expressed, purified and detected by standard silver staining (Figure 5A). After Cy3 labeling, these two proteins were incubated with Mtb functional proteome microarray that was consisting of 3,829 and 433 proteins from Mtb strains H37Rv and CDC1551, respectively (Deng J. Y. et al., 2014). To identify potential candidates, the cut-off was set as the signal-to-noise ratio (SNR) > 1.4 and Z-score>3.0 (Figure 5B). We identified three potential NRF1 binding partners Rv2239c (Uncharacterized protein), Rv0577 (Putative glyoxylase Cfp32), Rv2499c (Possible oxidase regulatory-related protein) and six SMAD2 binding partners Rv0577, Rv2499c, Rv2423 (Methyltransferase type 12), Rv3241c (Ribosome hibernation promotion factor, Hpf), Rv3153 (NADH-quinone oxidoreductase subunit I, NuoI) and Rv2117 (Uncharacterized protein), of which Rv0577 and Rv2499c interacted with both NRF1 and SMAD2 (Table 2). Notably, Rv0577 had been identified as one of the Mtb secreted proteins and interacted with host TLR2 in a previous study (de Souza et al., 2011; Byun et al., 2012), while Rv2239c and Rv2423 were found to be Mtb effectors by He and his colleagues recently (He et al., 2017). In order to validate these interactions between Mtb effectors and NRF1 or SMAD2, we successfully purified His tagged Rv0577, Rv3153, Rv2117, and Rv2423 (Supplementary Figure 3), and incubated each of them with PMA-treated THP-1 lysate before performing His tagged pull-down. In this way, we found that endogenously expressed NRF1 could be enriched by His tagged Rv0577, meanwhile, SMAD2 could be enriched by His tagged Rv2117 and Rv2423, partially pulled down by Rv0577 but not binding to Rv3153 (Figures 5C,D, Supplementary Figures 1, 2). Further validation by Co-IP of Flag-Rv0577 with endogenouse NRF1 while Flag-Rv0577, -Rv2117 or -Rv2423 with endogenouse SMAD2 were also constant with the His pull-down results (Figure 6). Thus, Rv0577 was a key node for establishing a preliminary Mtb-human PPI network under the limited verification (Supplementary Figure 4). These results suggested that our strategy was effective to discover the Mtb-host protein-protein interactions which will facilitate to depict the complete interactome between the pathogen and host.
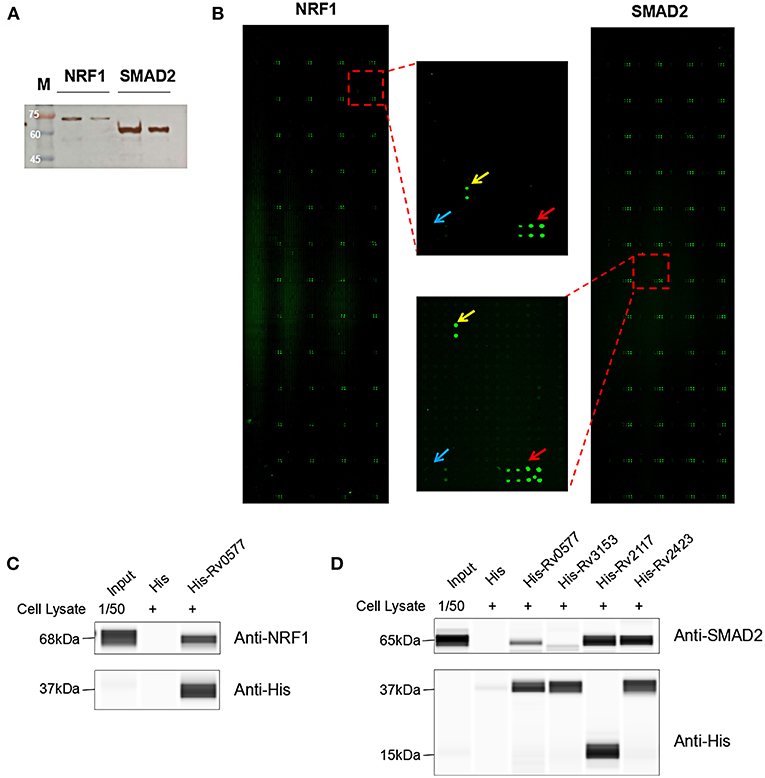
Figure 5. The NRF1 and SMAD2 interactors identified on a Mtb proteome microarray and validation by His pull-down assay. (A) Silver staining to detect the purified NRF1-myc and SMAD2-myc. (B) NRF1 and SMAD2 binding study with Mtb proteome microarray. Each array contained Cy3 labeled BSA as a positive control (marked with red arrows) and BSA as a negative control (marked with blue arrows). Positive proteins were marked with yellow arrows. The cutoff for calling positive candidates was set as signal-to-noise (SNR) ratio ≥ 1.4 and Z-score ≥ 3.0. (C) His tagged -Rv0577 pull-down assay with endogenously expressed NRF1. (D) His tagged -Rv0577, -Rv3153, -Rv2117, and -Rv2423 with endogenously expressed SMAD2, respectively. Protein separation and detection were performed using an automated capillary electrophoresis system. The results are representative of two independent experiments.
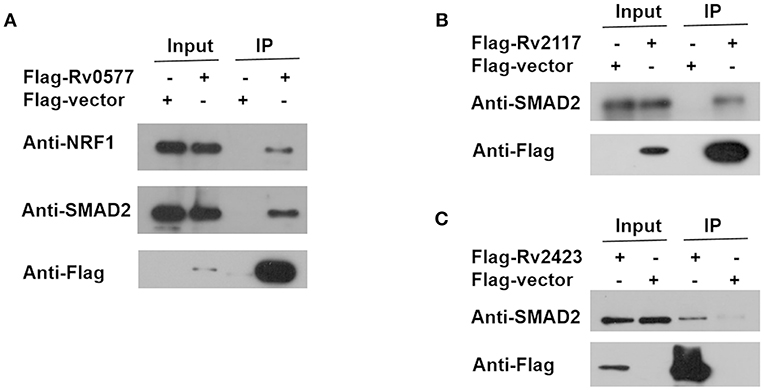
Figure 6. Co-IP of endogenous NRF1 with Rv0577 and endogenous SMAD2 with Rv0577, Rv2117, and Rv2423. (A) Co-IP of Flag-Rv0577 with endogenously expressed NRF1 and SMAD2. (B,C) Co-IP of Flag-Rv2117 and Flag-Rv2423 with endogenously expressed SMAD2, respectively. Western blot shown are representative of two independent experiments.
Discussion
The outcome of infection by Mtb largely depends on how the host responds to the invading bacteria and how the bacteria manipulates the host, which is facilitated by PPI. Elucidating protein interactions between human and Mtb would enable us to get insight into molecular mechanisms of pathogenesis. In the present study, we designed experiments to systematically determine all the human Mtb binding proteins and developed a strategy to identify Mtb effectors using an unbiased, comprehensive protein-chip based approach. Since extensive remodeling on the host is attributed to Mtb secreted virulence factors, cellular membrane proteins with access to the exterior, as well as part of cytosolic Mtb proteins released from lysate of bacterial cells inside phagocytes (He et al., 2017), we extracted Mtb SP and CP to cover most of Mtb effectors. According to human proteome microarray screening, a total of 84 interacting partners in a human host were successfully identified to bind with Mtb proteins. A bioinformatics analysis showed that these Mtb interactors were mainly related to gene expression, specifically transcription regulation. Further, they played roles in a wide range of cellular functions, such as activation of DNA endogenous promoters, transcription of DNA/RNA and necrosis, as well as signaling pathways, including TGF-β signaling and immune-related signaling pathways. What's more, to identify their Mtb effectors, we chose two protein candidates SMAD2 and NRF1 for further expression and incubation with Mtb proteome microarray, by which we screened for three binding partners for NRF1 and six interacting factors for SMAD2. Finally, His tagged pull-down assay and Co-IP verified several Mtb effectors, including Rv0577, Rv3153, Rv2117, and Rv2423.
To our knowledge, this is a pioneer experimental study on global human Mtb interactors, but has some similarities with a recent research (He et al., 2017) in which they globally profiled Mtb proteins interacting with proteins from macrophage by using Mtb proteome microarray. However, only membrane or secreted proteins were selected and considered as potential Mtb effectors. Besides, their human binders were identified by using GST-pull down followed by mass spectrometry analysis. Our strategy is differently based on a human proteome microarray containing 15,581 affinity-purified human proteins to profile potential Mtb interactors in human, and then reversely screening of their Mtb effectors by using Mtb proteome microarray. Specifically, there are several advantages of our two-way microarray strategy. First, it can globally and unbiasedly profile the bindings of more than 15,000 human proteins with Mtb SP and CP in a single experiment. Second, Mtb SP and CP, which comprise most Mtb effectors in a physiological condition, were both harvested and incubated with human proteome microarray to discover as much as Mtb interactors, not only membrane or secreted proteins. Third, all the proteins on the chips were overexpressed and purified under the same procedure, which means the local concentration of proteins on the microarray was relatively high, facilitating the PPI discovery. However, our strategy also has its limitation that we might have missed some positive interacting proteins not printed on microarray, and there are also lots of work related to validation that needs to be performed so as to get a comprehensive PPI network between Mtb and its human host.
To systematically explore the mechanism of Mtb pathogenesis in host cells, the functions and pathways that human Mtb interactors engaged in were analyzed. As expected, the presence of cancer related functions and pathways were detected in our analysis, as cancer shares many similarities with pathogen infection, such as evading immune response, tumor cell proliferation, death, metastasis, and invading cells. Among these overlapping pathways, couples of them have been experimentally tested and verified as having a role in tuberculosis. For example, TGF-β signaling pathway modulated T cell responses in early Mtb infection and facilitated inhibition of Mtb viability (Feruglio et al., 2017). Melatonin signaling was found to be effective in combating various bacterial and viral infections, including infections induced by Mtb (Srinivasan et al., 2012). IL-22 dependent pathways in both epithelial cells and macrophages mediate protective mechanisms for Mtb control (Treerat et al., 2017). Consistent with protein classification which showed these candidate proteins were classified into transcription factor, nucleic acid binding and receptor, our analysis results give a clue that Mtb manipulated immune and other related signaling pathways according to binding with receptors, transcription factors or DNA elements on the genome to regulate functional genes expression.
As expected, we found few overlaps between our newly identified Mtb interactors and currently known Mtb interactors (Table S1). While many reported interactors were discovered using different methods, such as Y2H or Mass-spec which have their own pros and cons, the results provided complementary types of information (Deng R. P. et al., 2014). Our discovery illustrated some novel potential molecules that were worthy of further exploring. For example, previous study has reported that activation and increased expression of PPARγ triggered by mycobacterial infection might aid the mycobacteria in circumventing the host response as an escape mechanism (Almeida et al., 2012). Recent published work indicated that PPARα was essential for antimycobacterial responses (Kim et al., 2017). PPARδ, as a sub-type of PPARs which mainly take part in the metabolism, cell proliferation and immune response, might also have important functions in response to Mtb infection. Indeed, it has been reported that PPARδ played a role in lipid absorption and metabolism (Luquet et al., 2005). MBD2, as a reader of DNA methylation, might contribute to interpret DNA methylation patterns into different functional output, including immune system function (Wood and Zhou, 2016). Although the role of MBD2 in tuberculosis is undefined, previous study reported that mycobacterial proteins could modify gene methylation in mammalian host tissues (Danjuma et al., 2017). For example, BCG-induced epigenetic reprogramming of immune cell function could enhance anti-mycobacterial immunity in macrophages (Verma et al., 2017). MAPK3 has been predicted to be an interactor in human in response to Mtb infection (Rapanoel et al., 2013). It is an enzyme which is a member of a MAPK family. Induction of the MAPK pathway is required for the expression of TNF-α, IL-10, and MCP-1 by human monocytes during Mtb infection (Song et al., 2003). SMAD2 is an intracellular signal transducer and transcriptional modulator. Formation of the SMAD2/SMAD4 complex, activates transcription. It has been reported that SMAD2 were up-regulated and absent in LTBI individuals (Stern et al., 2009). A recent authoritative study reported that NRF1 directly bound to and specifically sensed cholesterol in the endoplasmic reticulum (ER), acting as a central role in cholesterol homeostasis (Widenmaier et al., 2017). While previous study has indicated that Mtb could disturb cholesterol homeostasis in the host cell to support sustained infection (Vermeulen et al., 2017). Our results implied that Mtb might modulate this process by binding with NRF1. Combined with bioinformatics analysis results that most Mtb interactors identified in our study regulated gene expression, NRF1 and SMAD2 were selected to find their binding partners in Mtb. Finally, we found that Rv0577, which has been identified to be an interactor of TLR2 to induce maturation of dendritic cells and drives Th1 immune response (Byun et al., 2012), could bind with both NRF1 and SMAD2. Rv2423, having been identified as a Mtb effector (He et al., 2017), was also detected to be an interacting partner for SMAD2 in our study. Although His tagged Rv2239c were unsuccessfully purified at last, it has been detected as the top one potential binding protein with NRF1 in our microarray assay, which was also found to be Mtb effector by He et al. (2017).
Taken together, we have performed, to our knowledge, the first global protein chip analysis of human interactors with Mtb using the human proteome microarray and screened 84 proteins out of more than 15,000 proteins in human which might be responsible for pathogenesis in human after Mtb infection. In addition, we also verified the Mtb effectors of two representative proteins by reversely incubating with Mtb proteome microarray followed validation of His tagged pull-down assay and Co-IP, which will fill the gap in Mtb-human PPI data. However, there is far more verification work that needs to be done before we can show a comprehensive PPI network between Mtb and its human host. We believe that our results could serve as a valuable resource for both basic study and clinical research in the future.
Data Availability
The raw data supporting the conclusions of this manuscript will be made available by the authors, without undue reservation, to any qualified researcher.
Author Contributions
TC and ZZ conceived and designed the experiments. HJ, TC, and LL performed the experiments. LL analyzed the data and wrote the paper. JW, FD, LP, ZL, AX, YM, and JX provided suggestions on analysis and writing. ZZ wrote and revised the manuscript. All authors read and approved the final manuscript.
Conflict of Interest Statement
The authors declare that the research was conducted in the absence of any commercial or financial relationships that could be construed as a potential conflict of interest.
Acknowledgments
This work was supported by National Science and Technology Major Project (2017ZX10201301-004 and 2015ZX10004801-003), National Natural Science Foundation of China (31700668), Beijing Natural Science Foundation (5174035), Beijing Municipal Administration of Hospitals' Youth Programme (QML20181601). Collaborative Innovation Center of Infectious Diseases (PXM2016_014226_000052), Beijing Municipal Administration of Hospitals' Ascent Plan (DFL20181601), and Tongzhou District Science and Technology Committee (KJ2017CX076). We also thank the Beijing Protein Innovation for chip assays and data processing.
Supplementary Material
The Supplementary Material for this article can be found online at: https://www.frontiersin.org/articles/10.3389/fcimb.2019.00065/full#supplementary-material
References
Alipoor, S. D., Mortaz, E., Garssen, J., Movassaghi, M., Mirsaeidi, M., and Adcock, I. M. (2016). Exosomes and exosomal miRNA in respiratory diseases. Mediators Inflamm. 2016:5628404. doi: 10.1155/2016/5628404
Almeida, P. E., Carneiro, A. B., Silva, A. R., and Bozza, P. T. (2012). PPARγ expression and function in mycobacterial infection: roles in lipid metabolism, immunity, and bacterial killing. PPAR Res. 2012:383829. doi: 10.1155/2012/383829
Andersen, P., Askgaard, D., Ljungqvist, L., Bennedsen, J., and Heron, I. (1991). Proteins released from Mycobacterium tuberculosis during growth. Infect. immunity 59, 1905–1910.
Bach, H., Papavinasasundaram, K. G., Wong, D., Hmama, Z., and Av-Gay, Y. (2008). Mycobacterium tuberculosis virulence is mediated by PtpA dephosphorylation of human vacuolar protein sorting 33B. Cell Host Microbe 3, 316–322. doi: 10.1016/j.chom.2008.03.008
Biertümpfel, C., Zhao, Y., Kondo, Y., Ramón-Maiques, S., Gregory, M., Lee, J. Y., et al. (2010). Structure and mechanism of human DNA polymerase η. Nature 465, 1044–1048. doi: 10.1038/nature09196
Byun, E. H., Kim, W. S., Kim, J. S., Jung, I. D., Park, Y. M., Kim, H. J., et al. (2012). Mycobacterium tuberculosis Rv0577, a novel TLR2 agonist, induces maturation of dendritic cells and drives Th1 immune response. FASEB J. 26, 2695–2711. doi: 10.1096/fj.11-199588
Cambier, C. J., Falkow, S., and Ramakrishnan, L. (2014). Host evasion and exploitation schemes of Mycobacterium tuberculosis. Cell 159, 1497–1509. doi: 10.1016/j.cell.2014.11.024
Chang, K. C., and Yew, W. W. (2013). Management of difficult multidrug-resistant tuberculosis and extensively drug-resistant tuberculosis: update 2012. Respirology 18, 8–21. doi: 10.1111/j.1440-1843.2012.02257.x
Danelishvili, L., Yamazaki, Y., Selker, J., and Bermudez, L. E. (2010). Secreted Mycobacterium tuberculosis Rv3654c and Rv3655c proteins participate in the suppression of macrophage apoptosis. PLoS ONE 5:e10474. doi: 10.1371/journal.pone.0010474
Danjuma, L., Ling, M. P., Hamat, R. A., Higuchi, A., Alarfaj, A. A., Marlina, B. G., et al. (2017). Genomic plasticity between human and mycobacterial DNA: a review. Tuberculosis 107, 38–47. doi: 10.1016/j.tube.2017.03.006
de Souza, G. A., Leversen, N. A., Malen, H., and Wiker, H. G. (2011). Bacterial proteins with cleaved or uncleaved signal peptides of the general secretory pathway. J. Proteomics 75, 502–510. doi: 10.1016/j.jprot.2011.08.016
Deghmane, A.-E., Soualhine, H., Bach, H., Sendide, K., Itoh, S., Tam, A., et al. (2007). Lipoamide dehydrogenase mediates retention of coronin-1 on BCG vacuoles, leading to arrest in phagosome maturation. J. Cell Sci. 120, 3489–3489. doi: 10.1242/jcs.022335
Deng, J. Y., Bi, L. J., Zhou, L., Guo, S. J., Fleming, J., Jiang, H. W., et al. (2014). Mycobacterium tuberculosis proteome microarray for global studies of protein function and immunogenicity. Cell Rep. 9, 2317–2329. doi: 10.1016/j.celrep.2014.11.023
Deng, R. P., He, X., Guo, S. J., Liu, W. F., Tao, Y., and Tao, S. C. (2014). Global identification of OGlcNAc transferase (OGT) interactors by a human proteome microarray and the construction of an OGT interactome. Proteomics 14, 1020–1030. doi: 10.1002/pmic.201300144
Dyer, M. D., Neff, C., Dufford, M., Rivera, C. G., Shattuck, D., Bassaganya-Riera, J., et al. (2010). The human-bacterial pathogen protein interaction networks of Bacillus anthracis, Francisella tularensis, and Yersinia pestis. PLoS ONE 5:e12089. doi: 10.1371/journal.pone.0012089
Dziadek, B., Brzostek, A., Grzybowski, M., Fol, M., Krupa, A., Kryczka, J., et al. (2016). Mycobacterium tuberculosis AtsG (Rv0296c), GlmU (Rv1018c) and SahH (Rv3248c) proteins function as the human IL-8-binding effectors and contribute to pathogen entry into human neutrophils. PLoS ONE 11:e0148030. doi: 10.1371/journal.pone.0148030
Feruglio, S. L., Kvale, D., and Dyrhol-Riise, A. M. (2017). T cell responses and regulation and the impact of in vitro IL-10 and TGF-β modulation during treatment of active tuberculosis. Scand. J. Immunol. 85, 138–146. doi: 10.1111/sji.12511
Flannagan, R. S., Cosío, G., and Grinstein, S. (2009). Antimicrobial mechanisms of phagocytes and bacterial evasion strategies. Nat. Rev. Microbiol. 7, 355–366. doi: 10.1038/nrmicro2128
Goldberg, D. E., Siliciano, R. F., and Jacobs, W. R. Jr. (2012). Outwitting evolution: fighting drug resistant TB, malaria, and HIV. Cell 148, 1271–1283. doi: 10.1016/j.cell.2012.02.021
Gonzalez-Juarrero, M., and Orme, I. M. (2001). Characterization of murine lung dendritic cells infected with Mycobacterium tuberculosis. Infect. Immun. 69, 1127–1133. doi: 10.1128/IAI.69.2.1127-1133.2001
Guo, C., Fischhaber, P. L., Luk-Paszyc, M. J., Masuda, Y., Zhou, J., Kamiya, K., et al. (2003). Mouse Rev1 protein interacts with multiple DNA polymerases involved in translesion DNA synthesis. EMBO J. 22, 6621–6630. doi: 10.1093/emboj/cdg626
Gutierrez, M. G., Master, S. S., Singh, S. B., Taylor, G. A., Colombo, M. I., and Deretic, V. (2004). Autophagy is a defense mechanism inhibiting BCG and Mycobacterium tuberculosis survival in infected macrophages. Cell 119, 753–766. doi: 10.1016/j.cell.2004.11.038
He, X., Jiang, H. W., Chen, H., Zhang, H. N., Liu, Y., Xu, Z. W., et al. (2017). Systematic identification of mycobacterium tuberculosis effectors reveals that bfrb suppresses innate immunity. Mol. Cell Proteomics 16, 2243–2253.
Huang, D. W., Sherman, B. T., and Lempicki, R. A. (2009a). Bioinformatics enrichment tools: Paths toward the comprehensive functional analysis of large gene lists. Nucleic Acids Res. 37, 1–13. doi: 10.1093/nar/gkn923
Huang, D. W., Sherman, B. T., and Lempicki, R. A. (2009b). Systematic and integrative analysis of large gene lists using david bioinformatics resources. Nat. Protoc. 4, 44–57. doi: 10.1038/nprot.2008.211
Jäger, S., Cimermancic, P., Gulbahce, N., Johnson, J. R., McGovern, K. E., Clarke, S. C., et al. (2011). Global landscape of HIV human protein complexes. Nature 481, 365–370. doi: 10.1038/nature10719
Jeong, J. S., Jiang, L., Albino, E., Marrero, J., Rho, H. S., Hu, J., et al. (2012). Rapid identification of monospecific monoclonal antibodies using a human proteome microarray. Mol. Cell Proteomics 11:O111.016253. doi: 10.1074/mcp.O111.016253
Kim, K. H., An, D. R., Song, J., Yoon, J. Y., Kim, H. S., Yoon, H. J., et al. (2012). Mycobacterium tuberculosis Eis protein initiates suppression of host immune responses by acetylation of DUSP16/MKP-7. Proc. Natl. Acad. Sci. U.S.A. 109, 7729–7734. doi: 10.1073/pnas.1120251109
Kim, Y. S., Lee, H. M., Kim, J. K., Yang, C. S., Kim, T. S., Jung, M., et al. (2017). PPAR-α activation mediates innate host defense through induction of tfeb and lipid catabolism. J. Immunol. 198, 3283–3295. doi: 10.4049/jimmunol.1601920
Konig, R., Zhou, Y., Elleder, D., Diamond, T. L., Bonamy, G. M., Irelan, J. T., et al. (2008). Global analysis of host-pathogen interactions that regulate early-stage HIV-1 replication. Cell 135, 49–60. doi: 10.1016/j.cell.2008.07.032
Li, C. Y., Peng, J., Ren, L. P., Gan, L. X., Lu, X. J., Liu, Q., et al. (2013). Roles of histone hypoacetylation in LAT expression on T cells and Th2 polarization in allergic asthma. J. Transl. Med. 11:26. doi: 10.1186/1479-5876-11-26
Liu, X., Liu, B., Huang, Z., Shi, T., Chen, Y., and Zhang, J. (2012). SPPS: a sequence-based method for predicting probability of protein-protein interaction partners. PLoS ONE 7:e30938. doi: 10.1371/journal.pone.0030938
Luquet, S., Gaudel, C., Holst, D., Lopez-Soriano, J., Jehl-Pietri, C., Fredenrich, A., et al. (2005). Roles of PPAR delta in lipid absorption and metabolism: a new target for the treatment of type 2 diabetes. Biochim. Biophys. Acta 1740, 313–317. doi: 10.1016/j.bbadis.2004.11.011
Mehra, A., Zahra, A., Thompson, V., Sirisaengtaksin, N., Wells, A., Porto, M., et al. (2013). Mycobacterium tuberculosis type VII secreted effector EsxH targets host ESCRT to impair trafficking. PLoS Pathog. 9:e1003734. doi: 10.1371/journal.ppat.1003734
Mi, H. Y., Guo, N., Kejariwal, A., and Thomas, P. D. (2007). PANTHER version 6: protein sequence and function evolution data with expanded representation of biological pathways. Nucleic Acids Res. 35, 247–252. doi: 10.1093/nar/gkl869
Mueller-Ortiz, S. L., Wanger, A. R., and Norris, S. J. (2001). Mycobacterial protein HbhA binds human complement component C3. Infect. Immun. 69, 7501–7511. doi: 10.1128/IAI.69.12.7501-7511.2001
Poirier, V., and Av-Gay, Y. (2012). Mycobacterium tuberculosis modulators of the macrophage's cellular events. Microbes Infect. 14, 1211–1219. doi: 10.1016/j.micinf.2012.07.001
Rajagopala, S. V., Sikorski, P., Kumar, A., Mosca, R., Vlasblom, J., Arnold, R., et al. (2014). The binary protein-protein interaction landscape of Escherichia coli. Nat. Biotech. 32, 285–290. doi: 10.1038/nbt.2831
Rapanoel, H. A., Mazandu, G. K., and Mulder, N. J. (2013). Predicting and analyzing interactions between Mycobacterium tuberculosis and its human host. PLoS ONE 8:e67472. doi: 10.1371/journal.pone.0067472
Russell, D. G. (2001). Mycobacterium tuberculosis: here today, and here tomorrow. Nat. Rev. Mol. Cell. Biol. 2, 569–586. doi: 10.1038/35085034
Sekiya, T., Nakatsukasa, H., Lu, Q., and Yoshimura, A. (2016). Roles of transcription factors and epigenetic modifications in differentiation and maintenance of regulatory T cells. Microbes Infect. 18, 378–386. doi: 10.1016/j.micinf.2016.02.004
Song, C. H., Lee, J. S., Lee, S. H., Lim, K., Kim, H. J., Park, J. K., et al. (2003). Role of mitogen-activated protein kinase pathways in the production of tumor necrosis factor-alpha, interleukin-10, and monocyte chemotactic protein-1 by Mycobacterium tuberculosis H37Rv-infected human monocytes. J. Clin. Immunol. 23, 194–201.
Sreejit, G., Ahmed, A., Parveen, N., Jha, V., Valluri, V. L., Ghosh, S., et al. (2014). The ESAT-6 protein of Mycobacterium tuberculosis interacts with beta-2-microglobulin (beta2M) affecting antigen presentation function of macrophage. PLoS Pathog. 10:e1004446. doi: 10.1371/journal.ppat.1004446
Srinivasan, V., Mohamed, M., and Kato, H. (2012). Melatonin in bacterial and viral infections with focus on sepsis: a review. Recent Pat. Endocr. Metab. Immune Drug Discov. 6, 30–39.
Stern, J. N., Keskin, D. B., Romero, V., Zuniga, J., Encinales, L., Li, C., et al. (2009). Molecular signatures distinguishing active from latent tuberculosis in peripheral blood mononuclear cells, after in vitro antigenic stimulation with purified protein derivative of tuberculin (PPD) or Candida: a preliminary report. Immunol. Res. 45, 1–12. doi: 10.1007/s12026-008-8024-2
Sun, J., Wang, X., Lau, A., Liao, T. Y., Bucci, C., and Hmama, Z. (2010). Mycobacterial nucleoside diphosphate kinase blocks phagosome maturation in murine RAW 264.7 macrophages. PLoS ONE 5:e8769. doi: 10.1371/journal.pone.0008769
Sykiotis, G. P., and Bohmann, D. (2010). Stress-activated cap ‘n’ collar transcription factors in aging and human disease. Sci. Signal. 3:re3. doi: 10.1126/scisignal.3112re3
Treerat, P., Prince, O., Cruz-Lagunas, A., Muñoz-Torrico, M., Salazar-Lezama, M. A., Selman, M., et al. (2017). Novel role for IL-22 in protection during chronic Mycobacterium tuberculosis HN878 infection. Mucosal Immunol. 10, 1069–1081. doi: 10.1038/mi.2017.15
Vergne, I., Chua, J., Lee, H. H., Lucas, M., Belisle, J., and Deretic, V. (2005). Mechanism of phagolysosome biogenesis block by viable Mycobacterium tuberculosis. Proc. Natl. Acad. Sci. U.S.A. 102, 4033–4038. doi: 10.1073/pnas.0409716102
Verma, D., Parasa, V. R., Raffetseder, J., Martis, M., Mehta, R. B., Netea, M., et al. (2017). Anti-mycobacterial activity correlates with altered DNA methylation pattern in immune cells from BCG-vaccinated subjects. Sci. Rep. 7:12305. doi: 10.1038/s41598-017-12110-2
Vermeulen, I., Baird, M., Al-Dulayymi, J., Smet, M., Verschoor, J., and Grooten, J. (2017). Mycolates of Mycobacterium tuberculosis modulate the flow of cholesterol for bacillary proliferation in murine macrophages. J. Lipid Res. 58, 709–718. doi: 10.1194/jlr.M073171
Wang, J., Li, B. X., Ge, P. P., Li, J., Wang, Q., Gao, G. F., et al. (2015). Mycobacterium tuberculosis suppresses innate immunity by coopting the host ubiquitin system. Nat Immunol 16, 237–245. doi: 10.1038/ni.3096
Wang, J., Teng, J. L., Zhao, D., Ge, P., Li, B., Woo, P. C., et al. (2016). The ubiquitin ligase TRIM27 functions as a host restriction factor antagonized by Mycobacterium tuberculosis PtpA during mycobacterial infection. Sci. Rep. 6:34827. doi: 10.1038/srep34827
Wang, Z. X., Deng, R. P., Jiang, H. W., Guo, S. J., Le, H. Y., Zhao, X. D., et al. (2012). Global identification of prokaryotic glycoproteins based on an Escherichia coli proteome microarray. PLoS ONE 7:e49080. doi: 10.1371/journal.pone.0049080
Widenmaier, S. B., Snyder, N. A., Nguyen, T. B., Arduini, A., Lee, G. Y., Arruda, A. P., et al. (2017). NRF1 is an ER membrane sensor that is central to cholesterol homeostasis. Cell 171, 1094–1109. doi: 10.1016/j.cell.2017.10.003
Wong, D., Bach, H., Sun, J., Hmama, Z., and Av-Gay, Y. (2011). Mycobacterium tuberculosis protein tyrosine phosphatase (PtpA) excludes host vacuolar-H+-ATPase to inhibit phagosome acidification. Proc. Natl. Acad. Sci. U.S.A. 108, 19371–19376. doi: 10.1073/pnas.1109201108
Wood, K. H., and Zhou, Z. (2016). Emerging molecular and biological functions of MBD2, a reader of DNA methylation. Front. Genet. 7:93. doi: 10.3389/fgene.2016.00093
Zhang, Y., Sivasankar, S., Nelson, W. J., and Chu, S. (2009). Resolving cadherin interactions and binding cooperativity at the single-molecule level. Proc. Natl. Acad. Sci. U.S.A. 106, 109–114. doi: 10.1073/pnas.0811350106
Keywords: human proteome microarray, Mtb proteome microarray, host-pathogen interaction, NRF1, SMAD2
Citation: Cao T, Lyu L, Jia H, Wang J, Du F, Pan L, Li Z, Xing A, Xiao J, Ma Y and Zhang Z (2019) A Two-Way Proteome Microarray Strategy to Identify Novel Mycobacterium tuberculosis-Human Interactors. Front. Cell. Infect. Microbiol. 9:65. doi: 10.3389/fcimb.2019.00065
Received: 01 November 2018; Accepted: 01 March 2019;
Published: 28 March 2019.
Edited by:
Kathleen McDonough, Wadsworth Center, United StatesReviewed by:
Sheng-ce Tao, Shanghai Jiao Tong University, ChinaBeatriz Arellano Reynoso, National Autonomous University of Mexico, Mexico
Copyright © 2019 Cao, Lyu, Jia, Wang, Du, Pan, Li, Xing, Xiao, Ma and Zhang. This is an open-access article distributed under the terms of the Creative Commons Attribution License (CC BY). The use, distribution or reproduction in other forums is permitted, provided the original author(s) and the copyright owner(s) are credited and that the original publication in this journal is cited, in accordance with accepted academic practice. No use, distribution or reproduction is permitted which does not comply with these terms.
*Correspondence: Zongde Zhang, zzd417@163.com
†These authors have contributed equally to this work