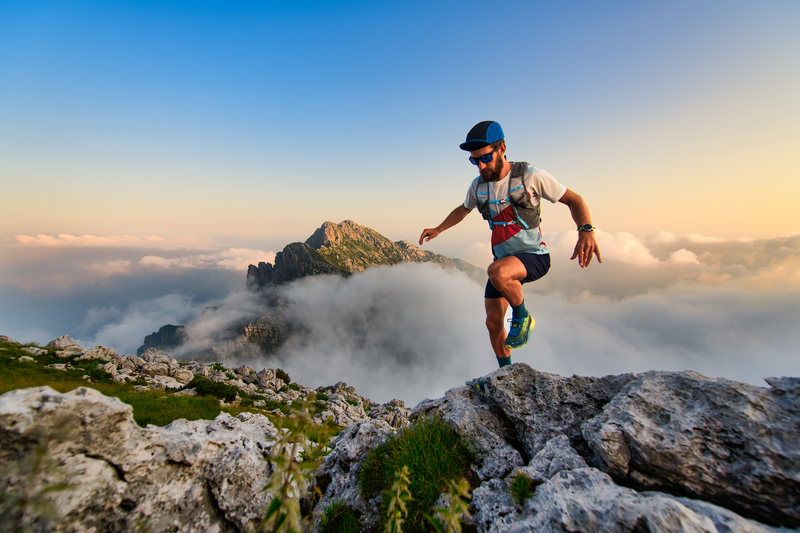
94% of researchers rate our articles as excellent or good
Learn more about the work of our research integrity team to safeguard the quality of each article we publish.
Find out more
ORIGINAL RESEARCH article
Front. Cell. Infect. Microbiol. , 14 June 2016
Sec. Clinical Microbiology
Volume 6 - 2016 | https://doi.org/10.3389/fcimb.2016.00060
This article is part of the Research Topic Molecular pathogenesis of pneumococus View all 11 articles
Influenza pandemics and seasonal outbreaks have shown the potential of Influenza A virus (IAV) to enhance susceptibility to a secondary infection with the bacterial pathogen Streptococcus pneumoniae (Sp). The high morbidity and mortality rate revealed the poor efficacy of antiviral drugs and vaccines to fight IAV infections. Currently, the most effective treatment for IAV is by antiviral neuraminidase inhibitors. Among them, the most frequently stockpiled is Oseltamivir which reduces viral release and transmission. However, effectiveness of Oseltamivir is compromised by the emergence of resistant IAV strains and secondary bacterial infections. To date, little attention has been given to evaluate how Oseltamivir treatment strategies alter Influenza viral infection in presence of Sp coinfection and a resistant IAV strain emergence. In this paper we investigate the efficacy of current approved Oseltamivir treatment regimens using a computational approach. Our numerical results suggest that the curative regimen (75 mg) may yield 47% of antiviral efficacy and 9% of antibacterial efficacy. An increment in dose to 150 mg (pandemic regimen) may increase the antiviral efficacy to 49% and the antibacterial efficacy to 16%. The choice to decrease the intake frequency to once per day is not recommended due to a significant reduction in both antiviral and antibacterial efficacy. We also observe that the treatment duration of 10 days may not provide a clear improvement on the antiviral and antibacterial efficacy compared to 5 days. All together, our in silico study reveals the success and pitfalls of Oseltamivir treatment strategies within IAV-Sp coinfection and calls for testing the validity in clinical trials.
Influenza A virus (IAV) and Streptococcus pneumoniae (Sp) are common causative agents of morbidity and mortality, respectively (Kilbourne, 2006; Morens et al., 2008; World Health Organization, 2009a). Over the last century four major influenza pandemics in 1918, 1957, 1968, and 2009 have had a significant impact worldwide. The Great Pandemic also known as the Spanish flu of 1918/1919 is considered as the deadliest pandemic with an estimated mortality of about 100 million around the globe (Johnson and Mueller, 2002). Interestingly, during the 1918 pandemic over 71% of the blood and sputum samples from fatal victims tested positive for Sp (Louria et al., 1959; McCullers and Rehg, 2002; McCullers, 2006, 2014), indicating a clear predisposition to lethal secondary bacterial infection in IAV preinfected patients.
Even though the mortality rate due to coinfections has decreased during the succeeding pandemics mostly because of antibiotic implementation, it still remains to be the most likely cause of death in 10–55% of the 2009 H1N1 victims. Thus, bacterial coinfection is a critical clinical outcome of viral infection and great attempts have been made to understand the pathogenesis and treatment course. The underlying mechanism for copathogenesis has been widely studied in animal models, providing evidence for a multifaceted disease affecting both lung physiology and immune responses (Shahangian et al., 2009; Small et al., 2010; Kash et al., 2011; Li et al., 2012). IAV-mediated immune aberrations such as immune cell dysfunction and apoptosis, dysregulated cytokine milieu and immunopathology in the lungs (Murray et al., 2014) have been implicated to have both immediate and long-term effects on anti-pneumococcal defense. The impact of coinfection is not limited to the bacterial outgrowth but also impairs antiviral immunity. Therefore, it is important for clinical treatment of coinfections to have a combinatorial approach to focus on all aspects of disease pathogenesis: the virus, bacteria, and host immune responses.
For prevention and treatment of acute IAV infection, antiviral drugs are an important adjunct to influenza vaccines (Goldstein and Lipsitch, 2009). The most commonly used Food and Drug Administration approved (FDA) antiviral drugs are neuraminidase inhibitors, e.g., Zanamivir, Peramivir, and Oseltamivir. The viral neuraminidase is an enzyme found on IAV surface enabling IAV virions to be released from the infected host cell. The neuraminidase inhibitors block this activity, thus interfering with viral spread and infectivity in the lungs (Moscona, 2005). In vivo administration of Oseltamivir is effective in controlling viral loads and immunopathology during lethal infection (McNicholl and McNicholl, 2001). In humans, the drug reduces clinical symptoms by 0.7–1.5 days when treatment is started 2 days after laboratory confirmed influenza, representing great potential if used appropriately to prevent the development of resistance (McNicholl and McNicholl, 2001). In the case of coinfections, the murine study in McCullers (2004) showed that treatment with Oseltamivir improved the survival by 75% in the coinfected group which further improved after combinatorial therapy with ampicillin. The first line of therapy following pneumococcal pneumonia is penicillin or other beta lactams, however the higher inflammatory status of the lung following coinfection with highly pathogenic virus strains may call for the use of non-lytic bacteriostatic agents such as clindamycin and azithromycin (Karlström et al., 2009). Furthermore, the anti-inflammatory and immunomodulatory action of corticosteroids used to treat many immune diseases could have a potent additive effect. In fact, the in vivo murine study by Trappetti et al. (2009) suggested a positive role of neuraminidase in Sp biofilm formation, thus Oseltamivir would be beneficial in preventing colonization. Following this study, the inhibiting effect of the approved anti-IAV drugs (Oseltamivir and Zanamivir) on Sp neuraminidase was confirmed by an in vitro kinetic study (Gut et al., 2011). Despite the existing combinatorial therapies against coinfections, the cumulative effect of neuraminidase inhibitor (Oseltamivir), the correct antibiotic and corticosteroids (Dexamethasone) is yet to be studied. With the increase in Oseltamivir use, drug resistant IAV strains may emerge bearing mutations such as H275Y in neuraminidase (Sheu et al., 2008). So far, the potentially detrimental effect of such mutant virus strains on secondary bacterial infections remains elusive.
The effectiveness of the Oseltamivir treatment depends on the dose regimen, intake frequency, time delay between infection and treatment, and treatment duration. The antiviral efficacy of neuraminidase inhibitors such as Oseltamivir, Amantadine and Peramivir has been investigated experimentally (Tanaka et al., 2015) and theoretically (Handel et al., 2007; Canini et al., 2014; Kamal et al., 2015). Recently, pharmaceutical companies have taken a strategic initiative to promote the use of modeling approaches within drug projects. The value of a model-based approach for improved efficiency and decision making during the preclinical stage of drug development has been largely advocated (Visser et al., 2013). Drug administration considers mainly two phenomena, i.e., the pharmacokinetic (PK) and pharmacodynamic (PD). The PK regards the temporal distribution of drug concentration in different organs of host body, while the PD describes the effect of a drug on the organism (Lahoz-Beneytez et al., 2015).
To the best of our knowledge, Oseltamivir treatment strategies for IAV infection in presence of Sp coinfection and a resistant IAV strain has not been investigated. In this paper, we tested the approved Oseltamivir treatment efficacy, combining a mathematical model of IAV-Sp coinfection with the PK/PD model of Oseltamivir. A possible emergence of an IAV Oseltamivir-resistant strain is also considered. Our computational results showed that Oseltamivir treatment with a dose of 150 mg, twice per day for 5 days is the minimum requirement recommended to achieve an antiviral efficacy of 49% and an antibacterial efficacy of 16%. Moreover, we found that in case of 75 mg dose administration, the intake frequency should not be lower than twice per day. A prolongation of the treatment up to 10 days with an intake frequency of twice per day, did not produce a clear benefit in terms of efficacy. This theoretical framework revealed the success and pitfalls of Oseltamivir strategies within IAV-Sp coinfection, paving the way for further refinement of therapeutic applications and clinical trials.
The PK model of Oseltamivir consists of a two compartment model (Rayner et al., 2008; Wattanagoon et al., 2009; Canini et al., 2014), one for Oseltamivir phosphate (OP) and one for its active metabolic compound form Oseltamivir Carboxylate (OC). The system of ordinary differential equations describing the concentrations of OP and OC is as follows:
where G is the depot compartment representing the OP dose administered, before it is adsorbed inside the blood with the adsorption rate ka. The parameter kf is the conversion rate from OP to OC and ke is the OC elimination rate. The initial conditions of this system are G(0) = Dose, OP(0) = 0, OC(0) = 0. As the explicit effect of OC is to inhibit the viral release of IAV from infected cells, we modeled in similar vein to Canini et al. (2014) the OC action by modifying the viral replication rate to p = (1 − ϵS(t))p, where ϵS(t) is the time varying drug efficacy defined as a function of OC concentration:
is the OC concentration providing the 50% of drug efficacy. Cell culture assays found the values of in the range [0.0008 − 35] μM (Tamiflu (R), 2009). Simulation environments will be based on values of equal to 0.5, 10, and 35 μM.
The scheme of the mathematical model of IAV-Sp coinfection and Oseltamivir interaction is illustrated in Figure 1. The dynamic of the IAV Oseltamivir-sensitive strain V is described by the target cell model with the eclipse phase (Nowak and May, 2000; Baccam et al., 2006; Beauchemin and Handel, 2011; Boianelli et al., 2015). Then, this is incorporated with the mathematical model of IAV-Sp coinfection proposed by Smith et al. (2013). We denote with U the uninfected cells, I1 the non productively infected cells, I2 the productively infected cells. U is infected by V with infection rate β. 1∕k is the average time in which I1 cells become productively infected cells. δ is the clearance rate of productively infected cells I2 while p is the viral replication rate of V by I2. c is the viral clearance rate of V. We fix the initial number of uninfected cells U(0) at 107. The initial conditions for the sensitive strain V and Sp B are in Table 2, while for the others model variables are set to zero. The IAV and Sp initial conditions for performing simulations are in the concentration units of TCID50mL−1 and CFUmL−1. The volume (mL) in the initial condition refers to the volume used (50 μL) in Smith et al. (2013) for the IAV and Sp (D39 strain) inoculum. According to the effect of OC on productively infected cells, the viral replication rate p is modified in p(1 − ϵS(t)). We assume that an IAV Oseltamivir-resistant mutant strain (H275Y) VR could emerge from the sensitive type as a consequence of Oseltamivir treatment (Sheu et al., 2008; World Health Organization, 2010; Chen et al., 2011; Dobrovolny et al., 2011; Renaud et al., 2011). The kinetic parameters of VR are assumed equal to those of V. The emergence of VR is considered to be with the probability μ. VR and V can compete for the same target cells U (Govorkova et al., 2010). Then, we denote with J1 and J2 the non productively and productively infected cells respectively of VR, where ϵR(t) is the Oseltamivir efficacy against VR having the same form in (4). It has been shown that for VR, is 400 times higher than those for V (Gubareva et al., 2001). We also explore the case where it is 200 times higher.
Figure 1. Mathematical model for IAV-Sp coinfection with the antiviral Oseltamivir carboxylate OC. The IAV sensitive strain V and resistant mutant strain VR (H275Y) are cleared at rate c. The uninfected cells become non productively infected cells I1 and J1 at the rate β by V and VR respectively. I1 and J1 become productively infected cells at the rate k, that is I2 and J2 respectively. V and VR replicate at the rate p(1 − μ) and μp respectively. I2 and J2 are cleared at rate δ. The bacteria B proliferate at the rate m and increase the viral replication rate a. In turn V and VR increase the bacterial carrying capacity with the parameter ϕ. Macrophages MA phagocytose the bacteria with the rate γMf(B, MA). The phagocytosis rate is decreased by V and VR with the parameter ψ. Bacteria increase the toxicity rate of non productively and productively infected cells of V and VR with the rate parameter η. The Oseltamivir carboxylate OC decreases the V and VR viral replication rate p to p(1 − ϵS(t)) and p(1 − ϵR(t)) respectively.
To investigate the synergy between IAV and Sp, Smith et al. (2013) modeled the bacteria dynamics and interaction with alveolar macrophages MA. The macrophages phagocytosis rate γMf(B, MA) of free bacteria is expressed by the mathematical function , where n is the maximum number of bacteria phagocytosed per MA, γM is the maximum phagocytosis rate. MA cells number is considered in quasi steady state, denoted as . Thus, the phagocytosis rate f(B, MA) is a decreasing function of B. The pneumococcus growth is assumed to be logistic with rate m and carrying capacity KB. The IAV is assumed to increase the pneumococcal adherence to epithelial cells. This is translated by increasing the bacterial carrying capacity KB(1 + ϕV) where ϕ is a proportionality constant. Moreover, another contribution of the IAV is the decreased rate of phagocytosis by MA. This effect is included with the saturation function ψV∕(KPV + V), where ψ is the maximal reduction of the phagocytosis rate and KPV is the half saturation constant. On the other hand, the pneumococcus effects on the IAV that may cause viral rebound are unknown. One plausible hypothesis assumes that the bacterial neuraminidase supports the viral neuraminidase to enhance the viral particle release from infected cells (McCullers, 2014). This is taken into account by considering an additional term in the viral replication rate p(1 + aBz), where z is the nonlinearity order coefficient and a is the positive term of bacterial effect. The model also included the toxicity effect of B on I1, J1, and I2, J2 with the toxicity rate η. This model is extended including the dynamics of the resistant virus, assuming that VR influences B in the same way of V and vice versa. The modified model is as follows:
The parameters value used for our population approach are in Table 1. These values represent the median value estimated in Canini et al. (2014), Wattanagoon et al. (2009) and Smith et al. (2013). More specifically, IAV-Sp model parameters were estimated from adult mice in Smith et al. (2013). It should be noted that kinetics and time scales of viral titer as well as immune parameters estimated from murine data can offer a reasonable approximation of IAV-Sp dynamics in humans (Small et al., 2010; Beauchemin and Handel, 2011). The parameter μ was estimated from human studies in Hayden (2001), as well as the Oseltamivir PK/PD model was inferred for human adults (Wattanagoon et al., 2009).
Table 1. IAV-Sp and PK/PD Oseltamivir model parameters with ranges used for the population approach.
The approved regimens stated by the guidelines for Oseltamivir administration in human adults (World Health Organization, 2009b; Canini et al., 2014) are: 75 mg twice per day for 5 days (curative regimen), 150 mg twice per day for 5 days (recommended regimen for pandemic influenza). These regimens are shown in Table 2 as a benchmark for the treatment evaluation. Oseltamivir regimens were evaluated with the antiviral efficacy index defined in Canini et al. (2014) as:
where AUCVT and AUCRT are the area under the curve of V and VR in presence of treatment, while AUCV and AUCR are the area under the curve without treatment. We also computed the antibacterial efficacy of the Oseltamivir treatment:
where AUCBT and AUCB are the area under the curve of the bacterial time course with and without treatment.
In order to take into account the individual heterogeinity observed in vivo (Canini and Carrat, 2011), we performed 10,000 simulations by sampling from a uniform distribution centered in the estimated values of Table 1 with a variation of ±30%. Model parameter ranges are showed in Table 1. We computed the antiviral and antibacterial efficacy defined in Equations (13)–(14) of the curative regimen with 75, 150, 300, and 450 mg, twice per day for 5 days. Moreover, a different intake frequency of once per day for 5 days with dosage of 75 mg was explored. A different treatment duration of 10 days with 75 mg and intake frequency of twice per day was also investigated. In order to mimic a realistic scenario, we assumed a random sampling for the starting time of drug treatment, time of coinfection and initial values of viral and bacterial titers. In fact, the amount of viral and bacterial burden is unknown when an individual is infected by IAV and Sp. Moreover, it is also unknown after how many days post the infection time the antiviral treatment is started. This is because the time of infection is not known. In the same way, the time of coinfection is typically unknown in naturally acquired Sp coinfection. The ranges of experimental values are presented in Table 2. For the correct viral dynamics simulation, we imposed the viral titer V to be constant when it crosses lower values than the threshold of 2.8 × 10−7 TCID50mL−1. The minimum therapy initiation time was considered starting at 2 days post infection, because at this time symptoms are clearly visible (Aoki et al., 2003; Louie et al., 2012; Muthuri et al., 2012).
We performed the one way ANOVA statistical significance test and then Bonferroni test on the 10,000 stochastic simulations. The statistical significance difference for antiviral and antibacterial distributions between the 75 mg dose (curative regimen) and 150 (pandemic regimen), 300, and 450 mg were computed. We compared also the curative regimen intake frequency of twice per day with intake frequency of once per day. Moreover, we investigated the statistical significance between treatment duration of 5 and 10 days. The comparison is done for values of 0.5, 10, and 35 μM.
To analyse to which extent each parameter affected the model outputs, we simulated the viral and bacterial dynamics by changing the parameters in Table 1 once per time of 10, 30 and 50% and keeping the others fixed (see Supplementary Figures S4, S5).
In this section we evaluated the antiviral and antibacterial efficacy for Oseltamivir dose of 75, 150, 300, 450 mg, twice per day for a treatment duration of 5 days. Figure 2 displays histograms obtained for different doses and . The histograms represent the distribution of antiviral and antibacterial efficacy values computed from 10,000 samples. Tables 3 and 4 show the median values of antiviral and antibacterial efficacy for different doses and values. Interestingly, the antibacterial efficacy histograms in Figure 2 presented a bimodal distribution for both different doses and values. The antibacterial dichotomy may be a result of two factors. The first could be attributed to the bacterial growth rate (m) and macropaghes phagocytosis rate (γM), greatly affecting Sp dynamics. The second refers to IAV parameters responsible for macrophages phagocytosis decrease (Kpv) and bacterial carrying capacity increase (ϕ) that influence the pneumococcal time course as well (Supplementary Figure S5). Then, combinations of these viral and bacterial parameters can promote alternatively bacterial colonization or bacterial clearance. This implies that in favorable conditions, Oseltamivir treatment strategies may be able to inhibit viral dynamics (high antiviral efficacy) and in turn modulate bacterial growth (high antibacterial efficacy). Otherwise, in worst scenarios, Oseltamivir treatment strategies may fail to control bacterial dynamics.
Figure 2. Histograms of the antiviral (green) and antibacterial efficacy (grey) for different Oseltamivir dose of 75, 150, 300, and 450 mg, with intake frequency of twice per day and a duration of 5 days for (A) μM, (B) μM, and (C) μM. The x-axis represents the Oseltamivir antiviral/antibacterial efficacy against IAV ranging from 0 (0%) to 1 (100%).
Table 3. Antiviral efficacy median values for different and different dose regimens with intake frequency of twice per day and treatment duration of 5 days.
Table 4. Antibacterial efficacy median values for different and different dose regimens with intake frequency of twice per day for a duration of 5 days.
For the best scenario where Oseltamivir treatment is effective ( μM), the first set of 10,000 simulations revealed that the distribution of antiviral and antibacterial efficacy were significantly different by changing the dose from 75 to 150 mg. In fact, for the curative regimen of 75 mg, the antiviral efficacy median value was 47%, remaining quite stable for higher values of dose (49%). The same pattern was conserved for higher values of , where for the highest dosage tested (450 mg), the median antiviral efficacy values were 45 and 28.9%, while for the curative regimen were 22 and 8.7% respectively. Concurrently, in Table 4, the antibacterial efficacy for the curative regimen with μM presented a value of 9% that further decreased to 0.3% for μM. Similarly, for 450 mg, the antibacterial efficacy dropped from 41 to 1.5%.
We also investigated the sensitivity of the Oseltamivir antiviral and antibacterial efficacy with respect to the values. The previous results were with . Thus, we tested the same treatment regimens of 75, 150, 300, and 450 mg with intake frequency of twice per day, for 5 days where . We computed the antiviral and antibacterial efficacy for the previous existing three different values of (see Supplementary Figure S1). Histograms of antiviral and antibacterial efficacy presented the same properties observed when . More specifically, in agreement with the previous case with , a statistically significant difference was observed between 75 and 150 mg for the lowest value of . We noted similar median values of the antiviral efficacy for different doses and with respect to the case where . In the same way, the antibacterial efficacy for 200 and 400 times the value of also presented consistent values (see Supplementary Tables S1, S2).
In order to investigate the effect of the intake frequency treatment on the coinfection course dynamics, we simulated in another set of 10,000 simulations, the administration of 75 mg dose with intake frequency of once per day, for 5 days. These treatment regimens are explored for the same values of considered previously and with the value of . The antiviral and antibacterial efficacy values are reported in Table 5. Histograms obtained from 10,000 simulations are shown in Figure 3.
Table 5. Comparison of antiviral and antibacterial efficacy median values for different values with intake frequency of once and twice per day and treatment duration of 5 days.
Figure 3. Histograms of the antiviral (green) and antibacterial (grey) efficacy for the dose of 75 mg and intake frequency of once per day, with the duration of 5 days for (A) μM, (B) μM, (C) μM. The x-axis represents the Oseltamivir antiviral/antibacterial efficacy against IAV/Sp ranging from 0 (0%) to 1 (100%).
Both antiviral and antibacterial histogram values significantly decreased when the intake frequency was once per day or for higher . The bimodal distribution was conserved for the antibacterial histograms due to reasons stated previously. The distributions of antiviral and antibacterial efficacy presented statistically significant difference (P < 0.05) for both different values of intake frequency and . Notably, the values in Table 5 with intake frequency of once per day were lower compared to the median values of the antiviral efficacy of the curative regimens for different values of . Therefore, the antibacterial efficacy medians with intake frequency of once per day showed approximately half values with respect to those with intake frequency of twice per day. These results stressed the importance of intake frequency to determine the clearance of the IAV-Sp coinfection. Furthermore, the case where (Supplementary Figure S2) was also investigated, noting that in this regimen both the antiviral and antibacterial efficacy medians possessed similar ranges compared with those obtained when (see Supplementary Table S3).
In order to test the influence of the treatment duration on the Oseltamivir efficacy against coinfection dynamics, we assumed the possibility of treatment duration of 10 days with dose of 75 mg and intake frequency of twice per day. The median values of antiviral and antibacterial efficacy are in Table 6. The histograms showing the antiviral and antibacterial efficacy distributions obtained from 10,000 simulations for different values are presented in Figure 4.
Table 6. Comparison of antiviral and antibacterial efficacy medians for different values and intake frequency of twice per day with the treatment duration of 5 and 10 days.
Figure 4. Histograms of the antiviral (green) and antibacterial (grey) efficacy for the dose of 75 mg and intake frequency of twice per day with the duration of 10 days for (A) , (B) μM, (C) μM. The x-axis represents the Oseltamivir antiviral/antibacterial efficacy against IAV/Sp ranging from 0 (0%) to 1 (100%).
Antiviral efficacy distributions for treatment duration of 5 and 10 days show similar median values for all of (no statistical significance differences are noted, P > 0.05). Moreover, the antibacterial efficacy with treatment durations of 5 and 10 days confirmed the same pattern, in particular for μM. We also investigated the same treatment regimens using the value of (see Supplementary Figure S3). In this setting also the antiviral efficacy distributions with treatment durations for 5 and 10 days were not statistically significant. We noted similar median values for the antiviral and antibacterial efficacy when (see Supplementary Table S4), for all the values of considered. Overall analysis suggested that the resistant mutant strain behavior may not really altered the efficacy of the Oseltamivir against IAV-Sp coinfection.
In the last decades many mathematical models have been developed describing the IAV infection dynamics in different hosts (Baccam et al., 2006; Tridane and Kuang, 2010; Hernandez-Vargas and Meyer-Hermann, 2012; Smith et al., 2013; Canini and Perelson, 2014; Boianelli et al., 2015), and in presence of treatment (Beauchemin and Handel, 2011; Canini and Perelson, 2014; Canini et al., 2014; Boianelli et al., 2015). However, the history of IAV pandemics have highlighted the role of the secondary bacterial infection in the increased morbidity and mortality. To date, the only mathematical model describing the IAV-pneumococcus coinfection was developed by Smith et al. (2013).
In this paper, we extend the coinfection model from Smith et al. (2013), by adding the pharmacokinetic and pharmacodynamic effects of Oseltamivir and taking into account a possible emergence of resistant mutant strain (H275Y) induced by Oseltamivir treatment. In our model, we simulate the intra-subject variability of influenza infection and also a time dependent Oseltamivir drug efficacy. We test the capability of the current approved Oseltamivir treatment regimens to achieve antiviral and antibacterial efficacy in a stochastic environment. Here, we simulate a more realistic scenario for coinfection and Oseltamivir treatment strategies. For example, we assume a random time of treatment as we do not know the delay between viral infection and treatment initiation. Secondly, we consider the time of coinfection randomly, because the time of secondary Sp infection is unknown. Moreover, in real life infection the exact amount of the viral and bacterial burden is usually unknown as well. The possibility of different intake frequencies and treatment duration according to the approved treatment regimens is explored.
Our results show that the curative regimen (75 mg for 5 days, twice per day) may offer the 47% of antiviral efficacy and 9% of antibacterial efficacy only in the case where the Oseltamivir is effective ( μM) against IAV. Increasing the dose from 75 to 150 mg with the same value of results in a statistically significant gain in terms of antiviral (49%) and antibacterial efficacy (16%). Then, for the case of IAV-Sp coinfection, the pandemic regimen could be recommended. Moreover, increasing the dose may not represent a reasonable gain of antiviral and antibacterial efficacy. However, in the case of the lowest efficiency of Oseltamivir ( μM), a significant increase in antiviral and antibacterial efficacy is obtained with a dose of 450 mg. With this dose, twice per day, for 5 days, antiviral and antibacterial efficacy display 28.9 and 1.5% median values, respectively. In the same range of treatment strategies for the value of the antiviral and antibacterial efficacy presented no significant differences compared to the case where . Moreover, reducing the intake frequency from twice to once per day with a dose of 75 mg could determine a significant reduction in the antiviral and antibacterial efficacy for the ranges of explored. In particular, from the best scenario ( μM), the antiviral efficacy reduces from 47 to 43% and the antibacterial efficacy from 9 to 4%. This reduction is more pronounced in the worst case ( μM), where both antiviral and antibacterial efficacy reduce approximately to the half of those values presented for a dosage of twice per day.
Against intuition, when the treatment duration is prolonged to 10 days with dose of 75 mg, this does not significantly increase the antiviral and antibacterial efficacy for all the values of . Concerning the antiviral efficacy, this result can be mainly attributed to the positive feedback of the bacterial secondary infection on IAV dynamics and in turn on the viral area under the curve. On the other hand, the antibacterial efficacy is also influenced, since the viral dynamics can modulate the bacterial growth via macrophages deactivation and can increase bacterial carrying capacity. The latter statement is also one of the factors that could lead to the bimodal distribution of the antibacterial efficacy histograms observed in all the treatment strategies. Importantly, from our computational study, the pharmacokinetic parameter directly influences the outcome of the Oseltamivir drug on IAV-Sp coinfection for all the tested treatment regimens. On the contrary, it turns out that the sensitivity of the antiviral and antibacterial efficacy to the parameter is low. This implies to presume that the resistant mutant strain does not really affect the antiviral and antibacterial efficacy. This is in agreement with in silico results obtained in Canini et al. (2014) where the authors evaluated the impact of Oseltamivir treatment strategies in the presence of the emerging resistant strain. In fact, for the treatment strategies considered in our work, the authors observed similar values of the antiviral efficacy (Treanor et al., 2000) when treatment is initiated at day 2 post infection. Therefore, our results of antibacterial efficacy (9%) obtained with curative regimen are lower than the experimental work of McCullers (2004), reporting an antibacterial efficacy value of 25% for murine data.
However, there are limitations in our simulation studies. Concerning the applied model (1)–(12), we did not consider the role of the immune response to clear the influenza virus. In fact, our investigations cannot be applied with hosts shedding preexisting immunity. Future studies should consider different models for the viral infection including the dynamics of the immune response such as CD8+T cells (Hancioglu et al., 2007; Lee et al., 2009; Miao et al., 2010; Tridane and Kuang, 2010; Dobrovolny et al., 2013), Interferon type I (Canini and Carrat, 2011; Pawelek et al., 2012; Hernandez-Vargas et al., 2014) and Natural killer cells (Canini and Carrat, 2011). In addition, the PK/PD dynamics have been estimated only for adults (Wattanagoon et al., 2009). This implies that for other groups such as children and seniors, our computational study should be tested with appropriate PK/PD parameter estimates. In fact for elderly, PK/PD parameters, e.g., apparent volume of distribution (prolongation of elimination half-life) can have important changes due to age modification in organ physiology (Mangoni and Jackson, 2004).
In summary, we find that the actual recommended regimens for Oseltamivir, i.e., curative and pandemic regimens may not completely able to control the colonization of a secondary bacterial coinfection. Higher doses, such as 150 and 300 mg, are recommended. Nevertheless, even this treatment regimen may not control coinfection in case of low Oseltamivir effectiveness. Moreover, our computational study suggests clear disadvantages of reducing the intake frequency below twice per day for a treatment duration of 5 and 10 days. Future clinical studies are needed to verify our results towards improved therapeutic treatments to fight coinfections (Dunning et al., 2014).
AB and EH designed the computational study and revised the manuscript. AB performed the simulations. AB, EH, NS, and DB discussed and wrote the manuscript.
The authors declare that the research was conducted in the absence of any commercial or financial relationships that could be construed as a potential conflict of interest.
This work was supported by iMed — the Helmholtz Initiative on Personalized Medicine and DAAD Germany through the program PROALMEX funding the project “OPTREAT.” In addition, we thank for support provided by the Department of Systems immunology (HZI), the Measures for the Establishment of Systems Medicine (e:Med) projects in Systems Immunology and Image Mining in Translational Bio-marker Research (SYSIMIT), contract number 01ZX1308B and in identification of predictive response and resistance factors to targeted therapy in gastric cancer using a systems medicine approach (SYS-Stomach), contract number 01ZX1310C by the Federal Ministry of Education and Research (BMBF), Germany. We thank for support by the Human Frontier Science Program (HFSP), RGP0033/2015. We also thank the support of the German Research Foundation (DFG), contract number SFB854. We thank the support by the International Research Training Group 1273 (IRTG1273) funded by the German Research Foundation (DFG).
The Supplementary Material for this article can be found online at: http://journal.frontiersin.org/article/10.3389/fcimb.2016.00060
Aoki, F. Y., Macleod, M. D., Paggiaro, P., Carewicz, O., El Sawy, A., Wat, C., et al. (2003). Early administration of oral oseltamivir increases the benefits of influenza treatment. J. Antimicrob. Chemother. 51, 123–129. doi: 10.1093/jac/dkg007
Baccam, P., Beauchemin, C., Macken, C. A., Hayden, F. G., and Perelson, A. S. (2006). Kinetics of influenza A virus infection in humans. J. Virol. 80, 7590–7599. doi: 10.1128/JVI.01623-05
Beauchemin, C. A., and Handel, A. (2011). A review of mathematical models of influenza A infections within a host or cell culture: lessons learned and challenges ahead. BMC Public Health 11:S7. doi: 10.1186/1471-2458-11-S1-S7
Boianelli, A., Nguyen, V. K., Ebensen, T., Schulze, K., Wilk, E., Sharma, N., et al. (2015). Modeling influenza virus infection: a roadmap for influenza research. Viruses 7, 5274–5304. doi: 10.3390/v7102875
Canini, L., and Carrat, F. (2011). Population modeling of influenza A/H1N1 virus kinetics and symptom dynamics. J. Virol. 85, 2764–2770. doi: 10.1128/JVI.01318-10
Canini, L., Conway, J. M., Perelson, A. S., and Carrat, F. (2014). Impact of different oseltamivir regimens on treating influenza A virus infection and resistance emergence: insights from a modelling study. PLoS Comput. Biol. 10:e1003568. doi: 10.1371/journal.pcbi.1003568
Canini, L., and Perelson, A. S. (2014). Viral kinetic modeling: state of the art. J. Pharmacokinet. Pharmacodyn. 41, 431–443. doi: 10.1007/s10928-014-9363-3
Chen, L. F., Dailey, N. J., Rao, A. K., Fleischauer, A. T., Greenwald, I., Deyde, V. M., et al. (2011). Cluster of oseltamivir-resistant 2009 pandemic influenza A (H1N1) virus infections on a hospital ward among immunocompromised patientsnorth carolina, 2009. J. Infect. Diseases 203, 838–846. doi: 10.1093/infdis/jiq124
Dobrovolny, H. M., Gieschke, R., Davies, B. E., Jumbe, N. L., and Beauchemin, C. A. (2011). Neuraminidase inhibitors for treatment of human and avian strain influenza: a comparative modeling study. J. Theoret. Biol. 269, 234–244. doi: 10.1016/j.jtbi.2010.10.017
Dobrovolny, H. M., Reddy, M. B., Kamal, M. A., Rayner, C. R., and Beauchemin, C. A. (2013). Assessing mathematical models of influenza infections using features of the immune response. PLoS ONE 8:e57088. doi: 10.1371/journal.pone.0057088
Dunning, J., Baillie, J. K., Cao, B., Hayden, F. G., and International Severe Acute Respiratory Emerging Infection Consortium (ISARIC) (2014). Antiviral combinations for severe influenza. Lancet Infect. Diseases 14, 1259–1270. doi: 10.1016/S1473-3099(14)70821-7
Goldstein, E., and Lipsitch, M. (2009). Antiviral usage for H1N1 treatment: pros, cons and an argument for broader prescribing guidelines in the united states. PLoS Curr. 1:RRN1122. doi: 10.1371/currents.RRN1122
Govorkova, E. A., Ilyushina, N. A., Marathe, B. M., McClaren, J. L., and Webster, R. G. (2010). Competitive fitness of oseltamivir-sensitive and-resistant highly pathogenic H5N1 influenza viruses in a ferret model. J. Virol. 84, 8042–8050. doi: 10.1128/JVI.00689-10
Gubareva, L. V., Webster, R. G., and Hayden, F. G. (2001). Comparison of the activities of zanamivir, oseltamivir, and RWJ-270201 against clinical isolates of influenza virus and neuraminidase inhibitor-resistant variants. Antimicrob. Agents Chemother. 45, 3403–3408. doi: 10.1128/AAC.45.12.3403-3408.2001
Gut, H., Xu, G., Taylor, G. L., and Walsh, M. A. (2011). Structural basis for Streptococcus pneumoniae nana inhibition by influenza antivirals zanamivir and oseltamivir carboxylate. J. Molecul. Biol. 409, 496–503. doi: 10.1016/j.jmb.2011.04.016
Hancioglu, B., Swigon, D., and Clermont, G. (2007). A dynamical model of human immune response to influenza A virus infection. J. Theoret. Biol. 246, 70–86. doi: 10.1016/j.jtbi.2006.12.015
Handel, A., Longini, I. M. Jr., and Antia, R. (2007). Neuraminidase inhibitor resistance in influenza: assessing the danger of its generation and spread. PLoS Comput. Biol. 3:e240. doi: 10.1371/journal.pcbi.0030240
Hayden, F. G. (2001). Perspectives on antiviral use during pandemic influenza. Philos. Trans. R Soc. Lond. B Biol. Sci. 356, 1877–1884. doi: 10.1098/rstb.2001.1007
Hernandez-Vargas, E. A., and Meyer-Hermann, M. (2012). “Innate immune system dynamics to influenza virus,” in Proceedings of the 8th IFAC Symposium on Biological and Medical Systems (Budapest), 29–31.
Hernandez-Vargas, E. A., Wilk, E., Canini, L., Toapanta, F. R., Binder, S. C., Uvarovskii, A., et al. (2014). Effects of aging on influenza virus infection dynamics. J. Virol. 88, 4123–4131. doi: 10.1128/JVI.03644-13
Johnson, N. P., and Mueller, J. (2002). Updating the accounts: global mortality of the 1918-1920 “spanish” influenza pandemic. Bull. Hist. Med. 76, 105–115. doi: 10.1353/bhm.2002.0022
Kamal, M. A., Gieschke, R., Lemenuel-Diot, A., Beauchemin, C. A., Smith, P. F., and Rayner, C. R. (2015). A drug-disease model describing the effect of oseltamivir neuraminidase inhibition on influenza virus progression. Antimicrob. Agents Chemother. 59, 5388–5395. doi: 10.1128/AAC.00069-15
Karlström, Å., Boyd, K., English, B. K., and McCullers, J. A. (2009). Treatment with protein synthesis inhibitors improves outcomes of secondary bacterial pneumonia after influenza. J. Infect. Diseases 199, 311–319. doi: 10.1086/596051
Kash, J. C., Walters, K.-A., Davis, A. S., Sandouk, A., Schwartzman, L. M., Jagger, B. W., et al. (2011). Lethal synergism of 2009 pandemic H1N1 influenza virus and Streptococcus pneumoniae coinfection is associated with loss of murine lung repair responses. MBio 2, e00172–11. doi: 10.1128/mBio.00172-11
Kilbourne, E. D. (2006). Influenza pandemics of the 20th century. Emerging Infect. Diseases 12:9. doi: 10.3201/eid1201.051254
Lahoz-Beneytez, J., Schnizler, K., and Eissing, T. (2015). A pharma perspective on the systems medicine and pharmacology of inflammation. Mathemat. Biosci. 260, 2–5. doi: 10.1016/j.mbs.2014.07.006
Lee, H. Y., Topham, D. J., Park, S. Y., Hollenbaugh, J., Treanor, J., Mosmann, T. R., et al. (2009). Simulation and prediction of the adaptive immune response to influenza a virus infection. J. Virol. 83, 7151–7165. doi: 10.1128/JVI.00098-09
Li, W., Moltedo, B., and Moran, T. M. (2012). Type I interferon induction during influenza virus infection increases susceptibility to secondary Streptococcus pneumoniae infection by negative regulation of γδ T cells. J. Virol. 86, 12304–12312. doi: 10.1128/JVI.01269-12
Louie, J. K., Yang, S., Acosta, M., Yen, C., Samuel, M. C., Schechter, R., et al. (2012). Treatment with neuraminidase inhibitors for critically ill patients with influenza A (H1N1) pdm09. Clin. Infect. Diseases 55, 1198–1204. doi: 10.1093/cid/cis636
Louria, D. B., Blumenfeld, H. L., Ellis, J. T., Kilbourne, E. D., and Rogers, D. E. (1959). Studies on influenza in the pandemic of 1957-1958. II. pulmonary complications of influenza. J. Clin. Invest. 38(1 Pt 1-2), 213.
Mangoni, A. A., and Jackson, S. H. (2004). Age-related changes in pharmacokinetics and pharmacodynamics: basic principles and practical applications. Br. J. Clin. Pharmacol. 57, 6–14. doi: 10.1046/j.1365-2125.2003.02007.x
McCullers, J. A. (2004). Effect of antiviral treatment on the outcome of secondary bacterial pneumonia after influenza. J. Infect. Diseases 190, 519–526. doi: 10.1086/421525
McCullers, J. A. (2006). Insights into the interaction between influenza virus and pneumococcus. Clin. Microbiol. Rev. 19, 571–582. doi: 10.1128/CMR.00058-05
McCullers, J. A. (2014). The co-pathogenesis of influenza viruses with bacteria in the lung. Nat. Rev. Microbiol. 12, 252–262. doi: 10.1038/nrmicro3231
McCullers, J. A., and Rehg, J. E. (2002). Lethal synergism between influenza virus and Streptococcus pneumoniae: characterization of a mouse model and the role of platelet-activating factor receptor. J. Infect. Diseases 186, 341–350. doi: 10.1086/341462
McNicholl, I. R., and McNicholl, J. J. (2001). Neuraminidase inhibitors: zanamivir and oseltamivir. Ann. Pharmacother. 35, 57–70. doi: 10.1345/aph.10118
Miao, H., Hollenbaugh, J. A., Zand, M. S., Holden-Wiltse, J., Mosmann, T. R., Perelson, A. S., et al. (2010). Quantifying the early immune response and adaptive immune response kinetics in mice infected with influenza A virus. J. Virol. 84, 6687–6698. doi: 10.1128/JVI.00266-10
Morens, D. M., Taubenberger, J. K., and Fauci, A. S. (2008). Predominant role of bacterial pneumonia as a cause of death in pandemic influenza: implications for pandemic influenza preparedness. J. Infect. Diseases 198, 962–970. doi: 10.1086/591708
Moscona, A. (2005). Neuraminidase inhibitors for influenza. New England J. Med. 353, 1363–1373. doi: 10.1056/NEJMra050740
Murray, P. J., Allen, J. E., Biswas, S. K., Fisher, E. A., Gilroy, D. W., Goerdt, S., et al. (2014). Macrophage activation and polarization: nomenclature and experimental guidelines. Immunity 41, 14–20. doi: 10.1016/j.immuni.2014.06.008
Muthuri, S. G., Myles, P. R., Venkatesan, S., Leonardi-Bee, J., and Nguyen-Van-Tam, J. S. (2012). Impact of neuraminidase inhibitor treatment on outcomes of public health importance during the 2009-10 influenza A (H1N1) pandemic: a systematic review and meta-analysis in hospitalized patients. J. Infect. Diseases. 207:jis726. doi: 10.1093/infdis/jis726
Nowak, M., and May, R. M. (2000). Virus Dynamics: Mathematical Principles of Immunology and Virology: Mathematical Principles of Immunology and Virology. Oxford, UK: Oxford University Press.
Pawelek, K. A., Huynh, G. T., Quinlivan, M., Cullinane, A., Rong, L., and Perelson, A. S. (2012). Modeling within-host dynamics of influenza virus infection including immune responses. PLoS Comput. Biol. 8:e1002588. doi: 10.1371/journal.pcbi.1002588
Rayner, C. R., Chanu, P., Gieschke, R., Boak, L. M., and Jonsson, E. N. (2008). Population pharmacokinetics of oseltamivir when coadministered with probenecid. J. Clin. Pharmacol. 48, 935–947. doi: 10.1177/0091270008320317
Renaud, C., Boudreault, A. A., Kuypers, J., Lofy, K. H., Corey, L., Boeckh, M. J., et al. (2011). H275Y mutant pandemic (H1N1) 2009 virus in immunocompromised patients. Emerg. Infect. Dis. 17, 653–660. doi: 10.3201/eid1704.101429
Shahangian, A., Chow, E. K., Tian, X., Kang, J. R., Ghaffari, A., Liu, S. Y., et al. (2009). Type I IFNs mediate development of postinfluenza bacterial pneumonia in mice. J. Clin. Invest. 119, 1910. doi: 10.1172/JCI35412
Sheu, T. G., Deyde, V. M., Okomo-Adhiambo, M., Garten, R. J., Xu, X., Bright, R. A., et al. (2008). Surveillance for neuraminidase inhibitor resistance among human influenza A and B viruses circulating worldwide from 2004 to 2008. Antimicrob. Agents Chemother. 52, 3284–3292. doi: 10.1128/AAC.00555-08
Small, C.-L., Shaler, C. R., McCormick, S., Jeyanathan, M., Damjanovic, D., Brown, E. G., et al. (2010). Influenza infection leads to increased susceptibility to subsequent bacterial superinfection by impairing NK cell responses in the lung. J. Immunol. 184, 2048–2056. doi: 10.4049/jimmunol.0902772
Smith, A. M., Adler, F. R., Ribeiro, R. M., Gutenkunst, R. N., McAuley, J. L., McCullers, J. A., et al. (2013). Kinetics of coinfection with influenza a virus and Streptococcus pneumoniae. PLoS Pathog. 9:e1003238. doi: 10.1371/journal.ppat.1003238
Tamiflu (R). (2009). Tamiflu (R) (oseltamivir phosphate) Capsules and for Oral Suspension. Package insert. Foster city, California: Roche laboratories Inc., Gilead Sciences Inc. Available online at: http://www.fda.gov/downloads/Drugs/DrugSafety/DrugShortages/UCM183850.pdf
Tanaka, A., Nakamura, S., Seki, M., Iwanaga, N., Kajihara, T., Kitano, M., et al. (2015). The effect of intravenous peramivir, compared with oral oseltamivir, on the outcome of post-influenza pneumococcal pneumonia in mice. Antiv. Ther. 20, 11–19. doi: 10.3851/IMP2744
Trappetti, C., Kadioglu, A., Carter, M., Hayre, J., Iannelli, F., Pozzi, G., et al. (2009). Sialic acid: a preventable signal for pneumococcal biofilm formation, colonization, and invasion of the host. J. Infect. Diseases 199, 1497–1505. doi: 10.1086/598483
Treanor, J. J., Hayden, F. G., Vrooman, P. S., Barbarash, R., Bettis, R., Riff, D., et al. (2000). Efficacy and safety of the oral neuraminidase inhibitor oseltamivir in treating acute influenza: a randomized controlled trial. Jama 283, 1016–1024. doi: 10.1001/jama.283.8.1016
Tridane, A., and Kuang, Y. (2010). Modeling the interaction of cytotoxic T lymphocytes and influenza virus infected epithelial cells. Mathemat. Biosci. Eng. 7, 171–185. doi: 10.3934/mbe.2010.7.171
Visser, S. A., Aurell, M., Jones, R. D., Schuck, V. J., Egnell, A.-C., Peters, S. A., et al. (2013). Model-based drug discovery: implementation and impact. Drug Discov. Today 18, 764–775. doi: 10.1016/j.drudis.2013.05.012
Wattanagoon, Y., Stepniewska, K., Lindegårdh, N., Pukrittayakamee, S., Silachamroon, U., Piyaphanee, W., et al. (2009). Pharmacokinetics of high-dose oseltamivir in healthy volunteers. Antimicrob. Agents Chemother. 53, 945–952. doi: 10.1128/AAC.00588-08
World Health Organization. (2009a). Influenza (seasonal). Fact sheet no. 211. World Health Organization, Geneva, Switzerland. Available online at: http://www.who.int/mediacentre/factsheets/fs211/en/index.html
Keywords: viral infection, S. pneumoniae coinfection, Oseltamivir treatment, PK/PD model, microbial resistance, population modeling, viral dynamic model
Citation: Boianelli A, Sharma-Chawla N, Bruder D and Hernandez-Vargas EA (2016) Oseltamivir PK/PD Modeling and Simulation to Evaluate Treatment Strategies against Influenza-Pneumococcus Coinfection. Front. Cell. Infect. Microbiol. 6:60. doi: 10.3389/fcimb.2016.00060
Received: 25 January 2016; Accepted: 23 May 2016;
Published: 14 June 2016.
Edited by:
Jorge Eugenio Vidal, Emory University, USACopyright © 2016 Boianelli, Sharma-Chawla, Bruder and Hernandez-Vargas. This is an open-access article distributed under the terms of the Creative Commons Attribution License (CC BY). The use, distribution or reproduction in other forums is permitted, provided the original author(s) or licensor are credited and that the original publication in this journal is cited, in accordance with accepted academic practice. No use, distribution or reproduction is permitted which does not comply with these terms.
*Correspondence: Esteban A. Hernandez-Vargas, ZXN0ZWJhbi52YXJnYXNAaGVsbWhvbHR6LWh6aS5kZQ==
Disclaimer: All claims expressed in this article are solely those of the authors and do not necessarily represent those of their affiliated organizations, or those of the publisher, the editors and the reviewers. Any product that may be evaluated in this article or claim that may be made by its manufacturer is not guaranteed or endorsed by the publisher.
Research integrity at Frontiers
Learn more about the work of our research integrity team to safeguard the quality of each article we publish.