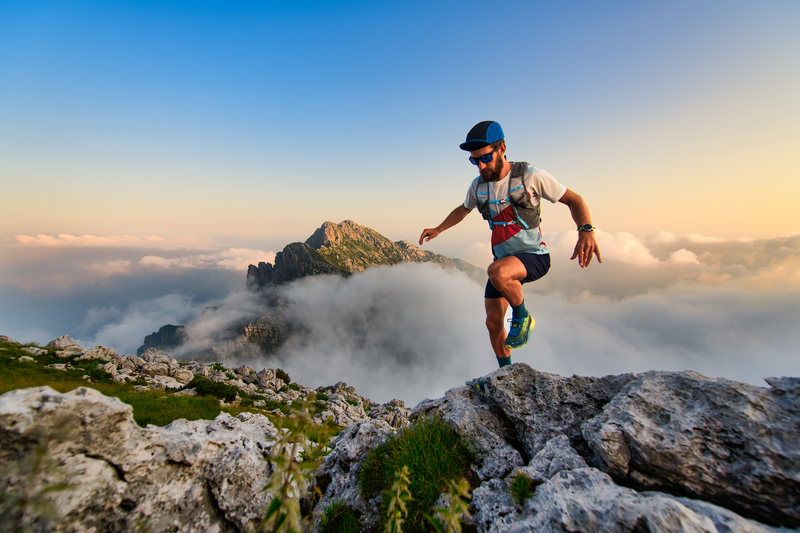
95% of researchers rate our articles as excellent or good
Learn more about the work of our research integrity team to safeguard the quality of each article we publish.
Find out more
REVIEW article
Front. Cell Dev. Biol.
Sec. Cancer Cell Biology
Volume 13 - 2025 | doi: 10.3389/fcell.2025.1554268
This article is part of the Research Topic 3D Models in Cancer Research: Bridging Tumor Biology and Personalized Medicine View all 9 articles
The final, formatted version of the article will be published soon.
You have multiple emails registered with Frontiers:
Please enter your email address:
If you already have an account, please login
You don't have a Frontiers account ? You can register here
Lung cancer is a major malignancy that poses a significant threat to human health, with its complex pathogenesis and molecular characteristics presenting substantial challenges for treatment. Traditional two-dimensional cell cultures and animal models are limited in their ability to accurately replicate the characteristics of different lung cancer patients, thereby hindering research on disease mechanisms and treatment strategies. The development of organoid technology has enabled the growth of patientderived tumor cells in three-dimensional cultures, which can stably preserve the tumor's tissue morphology, genomic features, and drug response. There have been significant advancements in the field of patient-derived lung cancer organoids (PDLCOs), challenges remain in the reproducibility and standardization of PDLCOs models due to variations in specimen sources, subsequent processing techniques, culture medium formulations, and Matrigel batches. This review summarizes the cultivation and validation processes of PDLCOs and explores their clinical applications in personalized treatment, drug screening after resistance, PDLCOs biobanks construction, and drug development. Additionally, the integration of PDLCOs with cutting-edge technologies in various fields, such as tumor assembloid techniques, artificial intelligence, organoid-on-a-chip, 3D bioprinting, gene editing, and single-cell RNA sequencing, has greatly expanded their clinical potential. This review, incorporating the latest research developments in PDLCOs, provides an overview of their cultivation, clinical applications, and interdisciplinary integration, while also addressing the prospects and challenges of PDLCOs in precision medicine for lung cancer.
Keywords: lung cancer, Organoids, cultivation, clinical applications, Transforming
Received: 01 Jan 2025; Accepted: 25 Mar 2025.
Copyright: © 2025 Chaoxing, Shi, Qi, Gu, Yu, Zhang and Qiu. This is an open-access article distributed under the terms of the Creative Commons Attribution License (CC BY). The use, distribution or reproduction in other forums is permitted, provided the original author(s) or licensor are credited and that the original publication in this journal is cited, in accordance with accepted academic practice. No use, distribution or reproduction is permitted which does not comply with these terms.
* Correspondence:
Liu Chaoxing, Yangzhou University, Yangzhou, China
Disclaimer: All claims expressed in this article are solely those of the authors and do not necessarily represent those of their affiliated organizations, or those of the publisher, the editors and the reviewers. Any product that may be evaluated in this article or claim that may be made by its manufacturer is not guaranteed or endorsed by the publisher.
Research integrity at Frontiers
Learn more about the work of our research integrity team to safeguard the quality of each article we publish.