- 1Faculty of Life Science and Technology, Kunming University of Science and Technology, Kunming, China
- 2Department of Ophthalmology, The Affiliated Hospital of Kunming University of Science and Technology, Kunming, China
- 3Department of Ophthalmology, The First People’s Hospital of Yunnan Province, Kunming, China
- 4Medical School, Kunming University of Science and Technology, Kunming, China
- 5Institute of Basic and Clinical Medicine, The First People’s Hospital of Yunnan Province, Kunming, China
Diabetic retinopathy (DR) is a leading global cause of vision impairment, with its prevalence increasing alongside the rising rates of diabetes mellitus (DM). Despite the retina’s complex structure, the underlying pathology of DR remains incompletely understood. Single-cell RNA sequencing (scRNA-seq) and recent advancements in multi-omics analyses have revolutionized molecular profiling, enabling high-throughput analysis and comprehensive characterization of complex biological systems. This review highlights the significant contributions of scRNA-seq, in conjunction with other multi-omics technologies, to DR research. Integrated scRNA-seq and transcriptomic analyses have revealed novel insights into DR pathogenesis, including alternative transcription start site events, fluctuations in cell populations, altered gene expression profiles, and critical signaling pathways within retinal cells. Furthermore, by integrating scRNA-seq with genetic association studies and multi-omics analyses, researchers have identified novel biomarkers, susceptibility genes, and potential therapeutic targets for DR, emphasizing the importance of specific retinal cell types in disease progression. The integration of scRNA-seq with metabolomics has also been instrumental in identifying specific metabolites and dysregulated pathways associated with DR. It is highly conceivable that the continued synergy between scRNA-seq and other multi-omics approaches will accelerate the discovery of underlying mechanisms and the development of novel therapeutic interventions for DR.
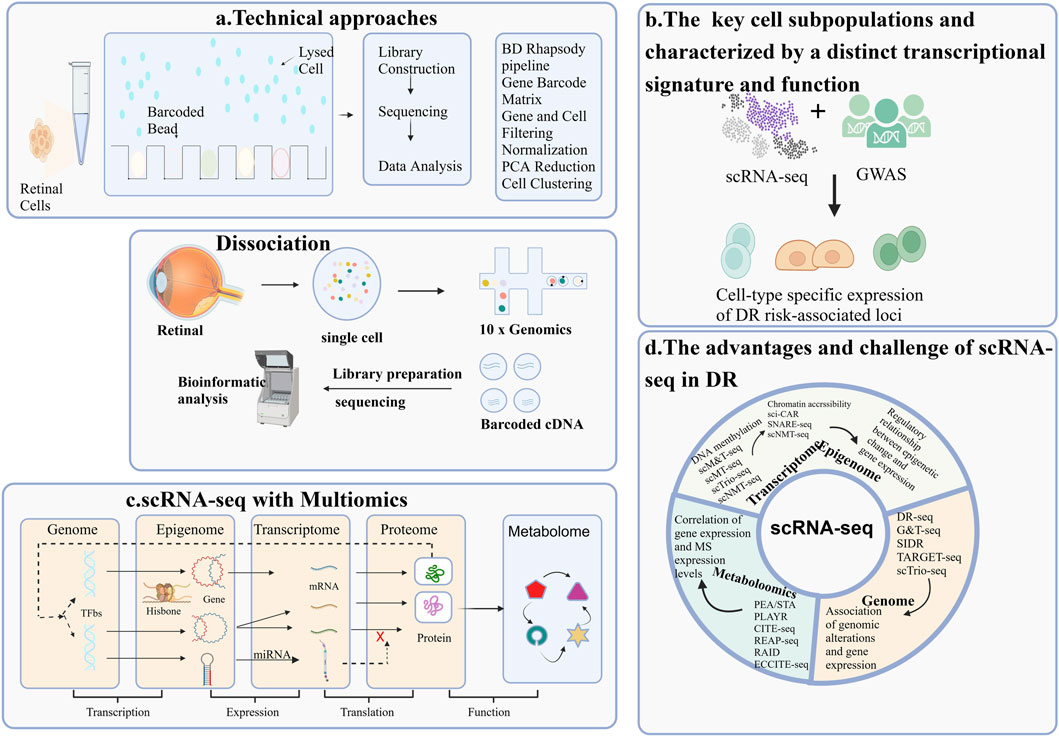
GRAPHICAL ABSTRACT | Overview of single-cell RNA sequencing (scRNA-seq) Applications in Diabetic Retinopathy Research. The application of single-cell RNA sequencing (scRNA-seq) methodologies in the study of diabetic retinopathy(DR) highlights four key areas: (A) The workflow of a typical scRNA-seq experiment; (B) The identification of key cell subpopulations, each characterized by a distinct transcriptional signature and function; (C) The integration of scRNA-seq with multi-omics approaches for DR research; (D) The advantages and challenges associated with scRNA-seq.
1 Introduction
Diabetic retinopathy (DR) is a leading cause of vision impairment and blindness among the working-age population globally (Bryl et al., 2022; Tan and Wong, 2022; Wurl et al., 2023). Projections indicate a substantial increase in the number of affected adults, with estimates reaching 129.84 million by 2030 and 160.50 million by 2045, posing significant societal and economic burdens (Ashraf et al., 2020; Yang et al., 2024; Yuan et al., 2024). The molecular mechanisms underlying DR encompass pathways such as inflammation, oxidative stress, renin-angiotensin system activation, and vascular endothelial growth factor (VEGF) signaling (Nouri et al., 2024). However, there remains a considerable gap in our understanding of the specific cellular alterations and their complex interactions in the disease’s progression. Emerging evidence suggests that abnormalities in cellular metabolic pathways, such as glucose, amino acid, and lipid metabolism, also play crucial roles in DR pathogenesis (Rohlenova et al., 2020; Yang Z et al., 2023). These metabolic disruptions lead to cellular stress, inflammation, and vascular damage, which are pivotal in disease development. Recognized increasingly as a neurodegenerative and neuroinflammatory disorder, DR involves intricate interactions among dysfunctional glial cells, neurons, and endothelial cells (ECs), highlighting its complexity (Llorian-Salvador et al., 2024).
Transcriptomic profiling of aqueous humor (AH), vitreous humor (VH), and retinal tissue has offered valuable insights into the global genomic alterations associated with DR pathogenesis, contributing to the identification of potential biomarkers and therapeutic targets (Wolf et al., 2023). However, the retina is a complex tissue composed of diverse cell types. Conventional transcriptomic profiles of DR, derived from heterogeneous cell populations, obscure crucial information about specific vulnerable cell types. Single-cell RNA sequencing (scRNA-seq) emerges as an unbiased and potent technique for characterizing distinct cell populations within complex tissues under both healthy and diseased conditions (Blanchard et al., 2022).
The exponential growth of scRNA-seq has dramatically enhanced our capacity to quantify ligand and receptor expression across diverse cell types, enabling the systematic elucidation of intercellular communication networks that underlie tissue function in both health and disease states (He et al., 2020; Ma et al., 2023).
Additionally, Xia et al. (2023) employed scRNA-seq in conjunction with genetic perturbation to precisely localize a specific pericyte subpopulation adjacent to pathological neovascularization tufts (Liu et al., 2022a). These findings underscore the potential of single-cell analyses to provide in-depth insights into distinct cell subpopulations and their roles in pathophysiological processes.
Multi-omics analyses (Fan and Pedersen, 2021; Guo et al., 2024) at the bulk retina level have been instrumental in providing a comprehensive understanding of cellular processes by integrating diverse molecular data, including mutations, mRNAs, proteins, and metabolites. Wang N et al. (2022) pioneered advanced methodologies for concurrent genomics and transcriptomics, leading to the identification of genes associated with DR. This finding was validated across independent cohorts and computational models, demonstrating the study’s robustness (Koh and Bäckhed, 2020).
The innovative approach employed in this research holds broad implications for understanding the complex genetic underpinnings of various diseases (Zhang and Zhao, 2016). This study also lays the foundation for developing novel therapeutic strategies for DR, with the potential to surpass current treatments such as laser surgery and intraocular injections.
In conclusion, integrating gene expression data from relevant cellular models with genetic association data has provided critical insights into the functional relevance of genetic risk factors for complex diseases such as DR. The identification of disease-associated differential gene expression and the utilization of expression quantitative trait loci based genome-wide association studies (GWAS) have been instrumental in elucidating potential causal genetic pathways underlying DR (Peng et al., 2023).
Identifying ligand-receptor interactions from scRNA-seq data involves integrating annotated relationships with statistical methods (Mao et al., 2024). This approach has spurred the development of single-cell multi-omics technologies, utilizing diverse experimental protocols such as mRNA-DNA methylation and mRNA-protein analysis to investigate cell type-specific gene regulation (Lv et al., 2022). Yao et al. (2022) employed scRNA-seq to elucidate EC heterogeneity and functional diversity. Their systematic analysis identified a unique EC cluster within the diabetic retina characterized by elevated inflammatory gene expression (Ma et al., 2023; Samanta et al., 2024). Therefore, single-cell multi-omics analysis offers a more comprehensive understanding of cell type-specific gene regulation than single-cell mono-omics approaches. This integrated approach significantly enhances our comprehension of the cellular and molecular landscape in DR, enabling the identification of affected cell types, elucidation of dysfunctional pathways, and discovery of potential therapeutic targets.
In this review, we explore the specific applications of scRNA-seq in DR from four distinct perspectives (Figure 1). Initially, we provide an overview of the foundational technologies underpinning single-cell sequencing, focusing on mRNA-genome sequencing, isolation techniques, specific protocols, and the analysis of scRNA-seq data. Next, we examine DR research through the lens of scRNA-seq, juxtaposing its findings with those from complementary multi-omics approaches, including transcriptomics, genomics, metabolomics, and lipidomics. We delineate key cell subpopulations and their dynamic changes throughout DR progression. The synergistic potential of integrating scRNA-seq with these multi-omics techniques for advancing DR research is also emphasized. Furthermore, we provide an overview of the current challenges and future opportunities in translating scRNA-seq findings into novel therapeutics for DR.
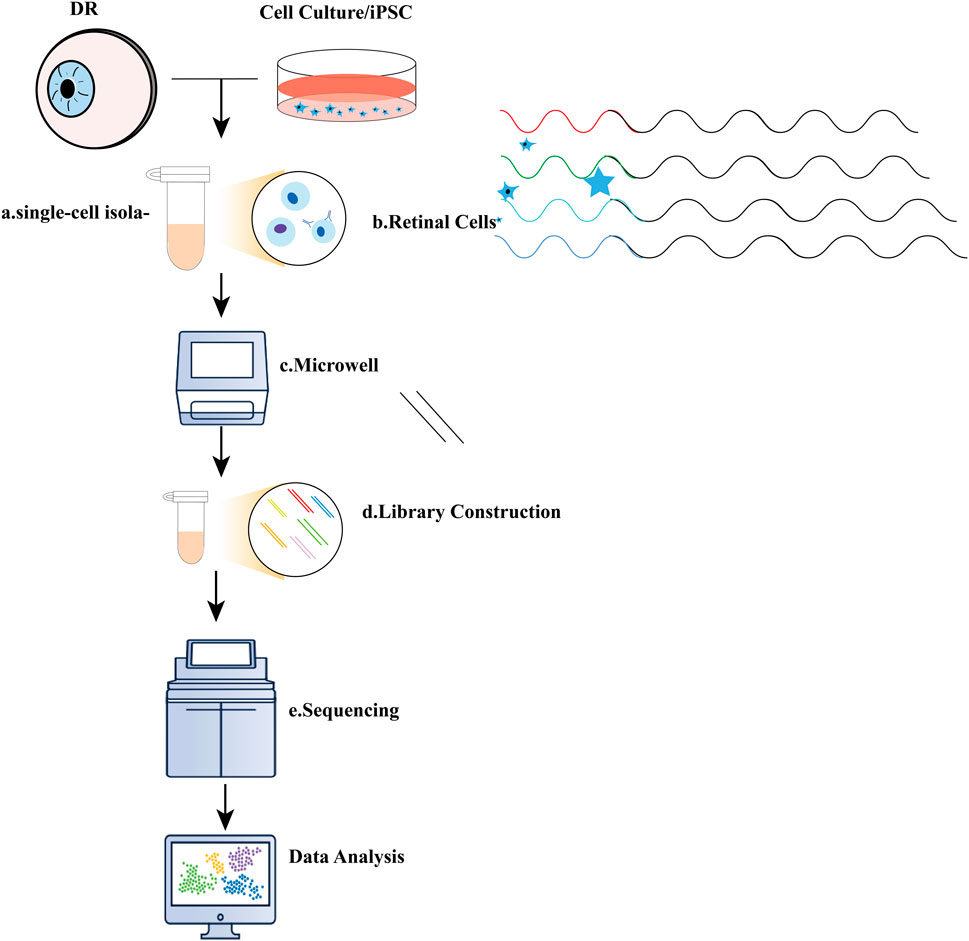
Figure 1. Illustration of single-cell RNA sequencing (scRNA-seq) experiments. (A) Typical single-cell RNA sequencing (scRNA-seq) workflow encompasses several key steps: a. single-cell isolation, which can be achieved through techniques such as micromanipulation, laser-capture microdissection, microfluidics, or fluorescence-activated cell sorting; (B). Cell lysis; (C) Reverse transcription of mRNA into complementary DNA (cDNA); (D) Amplification of cDNA and library preparation; (E) Sequencing.
2 Technical approaches
2.1 Technical approaches
In recent years, scRNA-seq (Ding et al., 2020) has revolutionized our understanding of cellular heterogeneity and regulation within diverse tissues during development and disease. This technology enables high-throughput, high-resolution transcriptomic profiling of individual cells by isolating and sequencing their RNA, thereby revealing distinct cellular states and functions (Lei et al., 2021).
2.1.1 ScRNA-seq workflow
The scRNA-seq workflow begins with the collection (Liu et al., 2024) and isolation of single cells from DR tissues (Chen et al., 2024b). Unlike conventional methods, scRNA-seq enables the differentiation of various retinal cell types, including ganglion cells, cone photoreceptors, and rod photoreceptors, at the single-cell level (Zhang et al., 2024). Figure 2 illustrates the essential steps involved in a typical scRNA-seq experiment.
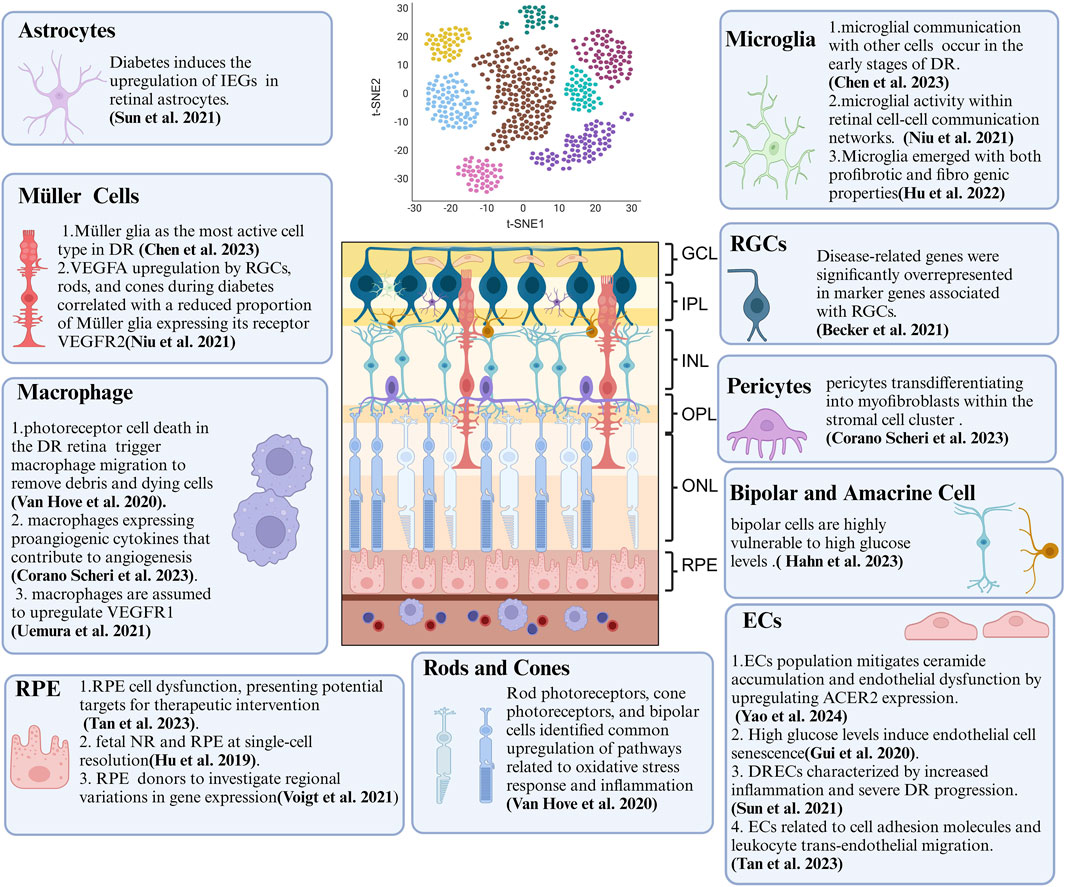
Figure 2. Summary of Crucial Cellular Components and Their Features in DR Revealed by scRNA-seq. ScRNA-seq technology provides biological information at the single-cell level and has been extensively applied to DR research. This review concludes that DR-associated microenvironments are characterized by diverse, highly heterogeneous, and dynamic cell populations. scRNA-seq has been instrumental in identifying various temporal states of different cell types, each with a distinct transcriptional signature and function. As scRNA-seq technology matures, it offers new approaches to study DR, deepening our understanding of its pathogenesis and paving the way for innovative treatments. Key cellular components include endothelial cells (ECs), retinal pigment epithelium (RPE), and retinal ganglion cells (RGCs).
2.2 Single-cell isolation
In DR, single-cell isolation techniques are essential due to the retina’s intricate tissue architecture (Niu et al., 2021), the need to identify rare cell populations, and the complexity of the generated data (Xu et al., 2021). These techniques (Kleino et al., 2022), coupled with high-throughput sequencing and bioinformatics, provide profound insights into DR pathogenesis and potential therapeutic targets (Van de Sande et al., 2023). Table 1 summarizes these experimental techniques, outlining their respective advantages and disadvantages.
2.3 Specific protocols for scRNA-seq
Specific protocols for scRNA-seq in DR research necessitate meticulous tissue preparation to ensure high cell viability (Heumos et al., 2023). This involves marker-based cell sorting to identify and isolate rare cell populations. Subsequent data integration with other omics approaches and advanced bioinformatics analysis are crucial for unraveling disease mechanisms and identifying potential therapeutic targets (Gérard et al., 2020). This methodology empowers comprehensive transcriptomic analysis at the single-cell level (Temple, 2023).
2.4 Analysis of single-cell sequencing data
ScRNA-seq is revolutionizing our comprehension of DR by facilitating the identification of differentially expressed genes (DEGs) and signature gene profiles (Wang et al., 2022). This technology affords granular insights into the cellular and molecular underpinnings of DR through comparative analysis of gene expression between healthy and diseased retinal cells (Zhang X et al., 2023). The retina’s diverse cellular composition, including ganglion, cone, and rod cells, necessitates specialized algorithms for precise classification and analysis (De Rop et al., 2022). DR-specific pathological alterations in retinal cells demand tailored analytical approaches to detect and interpret these changes at the single-cell level (Yu et al., 2022). To accurately capture the progressive nature of DR, single-cell data analysis must track evolving cellular states and interactions over time (Replogle et al., 2020). Understanding the intricate interplay between various retinal cell types within the microenvironment is pivotal for elucidating DR pathogenesis (Vu et al., 2016). Advanced computational methodologies, such as machine learning, enhance DEGs detection and provide deeper insights into disease mechanisms (McNulty et al., 2023). Integration with other omics data, enabled by tools like Cell Ranger.
3 ScRNA-seq application in diabetic retinopathy research
The advent of novel sequencing technologies has been instrumental in overcoming the limitations of traditional approaches and advancing our comprehension of DR pathogenesis. Advancements in scRNA-seq have provided invaluable insights into the heterogeneity of cell types and their corresponding states (Zhang P et al., 2023). In 2020, Van Hove et al. (2020) pioneered a single-cell transcriptomic retinal atlas, correlating genes associated with DR risk with cell type-specific expression patterns. Their findings emphasized distinct fibrotic, inflammatory, and gliotic profiles within macroglia subpopulations. Recent investigations have identified a unique retinal EC population in diabetes alongside a negative feedback regulatory pathway that attenuates endothelial dysfunction by upregulating alkaline ceramidase 2 (ACER2) expression to decrease ceramide content (Yao et al., 2024).
ScRNA-seq provides a highly sensitive method to detect gene expression changes within specific cell types, significantly enhancing our understanding of the molecular pathways involved in DR (Jovic et al., 2022). Identification of DEGs sheds light on the cellular and molecular mechanisms contributing to DR, thus facilitating the development of targeted therapies (Bawa et al., 2022). These gene expression alterations lead to dysfunction and subsequent injury across various retinal cell types, including retinal pigment epithelium (RPE), cone photoreceptors, macroglia, microglia, and vascular cells (Ghita et al., 2023). Furthermore, genetic risk variants, cellular statuses, and microenvironmental deterioration further propel DR progression. This section will explore the diverse applications of scRNA-seq in DR research (Figure 3; Table 2 for details).
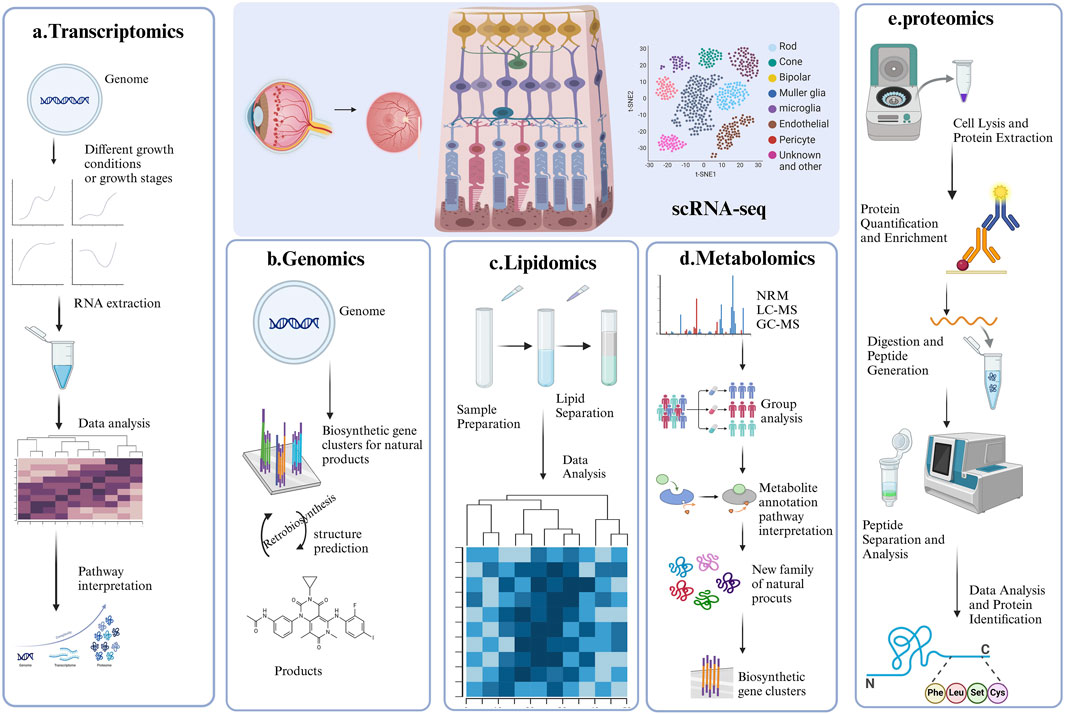
Figure 3. Representative strategy of single-cell RNA sequencing (scRNA-seq) and Multi-Omics. This figure summarizes the four key applications of single-cell RNA sequencing in DR research, each accompanied by a corresponding graph for better understanding. (A) ScRNA-seq combined with Transcriptomics; (B) ScRNA-seq combined with Genomics; (C) ScRNA-seq combined with lipidomic; (D) ScRNA-seq combined with Metabolomic; (E) ScRNA-seq combined with proteomics.
3.1 ECs
ECs are critical for the formation and maintenance of the blood-retinal barrier and retinal vascular integrity (Yang and Liu, 2022). In DR, EC dysfunction underlies vascular leakage and neovascularization. ScRNA-seq has become a valuable tool for identifying gene expression alterations in ECs that contribute to these vascular pathologies, providing novel insights into the mechanisms of vascular permeability and abnormal blood vessel growth (Hu et al., 2023).
Studies employing scRNA-seq have generated comprehensive transcriptional profiles of retinal cells in DR. Yao et al. (2024) characterized the heterogeneity and functional diversity of retinal ECs in the human choroid, identifying a novel diabetes-specific EC population. They further elucidated a negative feedback regulatory pathway that attenuates ceramide accumulation and endothelial dysfunction by upregulating ACER2 expression. These findings emphasize the crucial interplay between ECs and other cellular components in DR. Subsequent research by Gui et al. (2020) and Bertelli et al. (2022) reinforced the pivotal role of ECs in DR progression. High glucose levels were found to induce EC senescence, with p53 identified as a key regulator of this process. Inhibition of p53 in human retinal microvascular ECs diminished senescence markers, while overexpression exacerbated them. Sun et al. (2021) constructed a transcriptome atlas encompassing over 14,000 single cells from both healthy and diabetic murine retinas. This analysis identified a distinct subgroup of ECs, termed diabetic retina-specific ECs, characterized by heightened inflammation and accelerated DR progression. The study linked the HIF-1 signaling pathway to dysregulated genes in both general diabetic ECs and DRECs, emphasizing its pivotal role in DR pathogenesis. Subsequent analysis of 80 human post-mortem retinal samples from patients with varying DR stages demonstrated significant enrichment of DR-associated genes in ECs, specifically those linked to cell adhesion molecules and leukocyte trans-endothelial migration (Tan Y et al., 2023).
The application of scRNA-seq has markedly propelled DR research, enabling the identification of ECs subclusters exhibiting noncanonical transcriptional profiles and active angiogenesis. This high-resolution methodology provides unparalleled insights into the cellular and molecular underpinnings of DR, thereby facilitating the development of innovative therapeutic approaches.
3.2 RPE
RPE cells are crucial for maintaining photoreceptor cell function and overall retinal health (Liu et al., 2022a). Dysfunction of RPE cells in DR can lead to neural retinal (NR) degeneration and subsequent visual impairment. ScRNA-seq has emerged as a powerful tool for identifying distinct RPE cell populations based on their unique gene expression profiles, providing invaluable insights into the molecular mechanisms underlying RPE cell dysfunction in diabetic conditions.
Over the years, studies utilizing scRNA-seq have identified substantial alterations in gene expression associated with oxidative stress, inflammation, and cellular metabolism within RPE cells during DR (Tan Y et al., 2023). These investigations have pinpointed key regulatory pathways and genes implicated in RPE cell dysfunction, providing potential targets for therapeutic interventions. Notably, Voigt et al. (2021a) isolated RPE tissue from the macula and periphery of three human donors to examine regional variations in gene expression. Their research culminated in a comprehensive expression atlas of RPE and choroidal cell types, significantly surpassing the resolution of previous bulk transcriptomic studies of the RPE. Another study (Hu et al., 2019) characterized the transcriptome of human fetal NR and RPE at single-cell resolution, revealing distinct gene expression profiles: NR genes linked to nervous system development and RPE genes associated with retinol metabolism. Despite these differences, both tissues exhibited developmental processes ranging from active proliferation to visual perception maturation, suggesting functional interactions during later developmental stages. Disruptions in these interactions have been implicated in the formation of a multilayered retina-like structure by the RPE.
The application of scRNA-seq to individual RPE cells has significantly advanced our comprehension of normal visual physiology and the dysfunctions associated with DR. By providing granular insights into the gene expression profiles and regulatory pathways of RPE cells, scRNA-seq research holds the potential to inform novel therapeutic strategies targeting RPE dysfunction and its contribution to DR.
3.3 Retinal ganglion cells (RGCs)
RGCs are essential for relaying complex, integrated visual information from the retina to the brain’s visual centers (Kim et al., 2021). In DR, damage to RGCs is a primary cause of vision loss (Zhang et al., 2017). ScRNA-seq has provided compelling evidence of RGC depletion in the early stages of DR.
A comprehensive study analyzed 80 human post-mortem retinal samples from 43 patients with varying stages of DR using RNA sequencing (Becker et al., 2021). This investigation identified disease-related genes that were significantly overrepresented among marker genes associated with RGCs, indicating a progressive downregulation of RGC-specific genes during DR progression and suggesting a general loss of this cell type. Notably, the study revealed partial RGC loss even in diabetic patients without clinically diagnosed DR, aligning with optical coherence tomography findings of RGC damage in this pre-pathological stage of DR. These results underscore the critical importance of early detection and intervention to prevent further RGC loss and preserve vision in diabetic patients (Lee et al., 2021). One study (Yang Z et al., 2023) investigates Using scRNA-seq, EMPA mitigates microglia-mediated neuroinflammation and prevents RGC loss in retinal injury, with Mfn1 and Opa1 as essential contributors to its mitochondrial protective effects.
Notably, RGCs show substantial diversity, with each subtype expressing different members of the synuclein family. ScRNA-seq has revealed cross-species expression patterns of synuclein family members, offering new avenues to investigate the distinct roles of RGC subtypes in visual signal processing and transmission (Laboissonniere et al., 2019; Peng et al., 2024). These findings hold promise for clinical advancements, potentially leading to novel diagnostic and therapeutic approaches for retinal and neurodegenerative diseases.
3.4 Müller cells
Müller cells are essential for providing structural and functional support to retinal neurons (Tang et al., 2023). In the context of DR, these cells undergo reactive gliosis, compromising their ability to maintain retinal homeostasis (Perais et al., 2023). scRNA-seq has been instrumental in elucidating the molecular alterations within Müller cells during DR, particularly in genes associated with inflammation, gliosis, and their supportive roles in the retina.
Employing scRNA-seq, Chen et al. (2023b) identified Müller glia as the most active cell type under these conditions. While overall communication among Müller glia remained consistent, a decrease in interactions mediated by growth factors and a corresponding increase in cytokine-driven interactions were observed. In another study (Niu et al., 2021) utilizing scRNA-seq, Müller glia cluster six was highlighted as exhibiting a stronger correlation with retinal alterations in diabetic mice compared to other clusters.
Moreover, diabetic conditions were associated with upregulated VEGFA expression in RGCs, rods, and cones. Concurrently, there was a decreased proportion of Müller glia expressing its receptor, VEGF receptor 2(VEGFR2). These findings highlight the complex molecular adaptations occurring within Müller cells in response to the diabetic environment. ScRNA-seq has provided invaluable insights into the multifaceted roles of Müller cells in DR, elucidating alterations in their gene expression profiles and interactions with other retinal cells in response to diabetes. These findings have identified potential therapeutic targets for modulating Müller cell function and mitigating retinal damage associated with DR.
3.5 Photoreceptors cells (rods and cones)
Photoreceptor cells are essential for converting light into neural signals. Their degeneration, significantly contributing to vision loss in the advanced stages of DR, has been a focal point of research (Rauscher et al., 2024). ScRNA-seq has been instrumental in elucidating alterations in gene expression within photoreceptors under hyperglycemic and ischemic conditions, providing crucial insights into the molecular mechanisms underlying their degeneration (Xiong et al., 2019).
A recent study (Van Hove et al., 2020) reported substantial alterations in retinal cell population composition, notably impacting rod photoreceptors and specific inflammatory cell subsets. Differential gene expression analysis of rods, cones, and bipolar cells (BCs) identified a common upregulation of pathways associated with oxidative stress response and inflammation in Akimba models.
Through scRNA-seq, researchers have acquired a comprehensive understanding of the transcriptional heterogeneity within neuronal and inflammatory cell populations in DR. This in-depth knowledge offers significant potential for developing targeted therapies designed to mitigate photoreceptor degeneration and preserve vision in patients with DR.
3.6 Microglia
Microglia, the resident macrophages of the retina, play crucial roles in both inflammation and neuroprotection within the tissue (Kinuthia et al., 2020). In DR, activated microglia can contribute to inflammatory damage. ScRNA-seq has been instrumental in characterizing the diverse activation states of microglia and macrophages, distinguishing between pro-inflammatory and neuroprotective phenotypes, and identifying the underlying signaling pathways involved in their activation (Van Hove et al., 2020).
A study (Chen et al., 2023b) observed microglial activation in BKSDB mice, suggesting impaired communication with other cell types during the early stages of DR. Analysis revealed disparate changes in receptor-ligand interactions, with decreased microglial communication mediated by growth factors and enhanced interactions via cytokines and other signaling pathways in BKSDB compared to BKSWT mice. Another study (Niu et al., 2021) reported elevated microglial activity within retinal cell-cell communication networks in BKSDB mice at 8 months of age. Wang Y et al. (2024) reveals significant early-stage changes in retinal microglia under diabetic conditions, identifying three new microglial subtypes and constructing an inflammatory network, which provides insights into early intervention strategies for DR through scRNA-seq analysis.
ScRNA-seq of fibrovascular membranes in a study of PDR identified eight distinct cellular populations, with microglia being the predominant cell type (Hu et al., 2022). A subpopulation of microglia expressing Glycoprotein Non-Metastatic Protein B exhibited both profibrotic and fibrogenic characteristics, suggesting a potential role in PDR progression. Pseudotime analysis revealed unique differentiation trajectories of profibrotic microglia from resident microglia within the PDR context. The identification of ligand-receptor interactions between profibrotic microglia and upregulated cytokines in PDR vitreous implied their activation within the PDR microenvironment.
The above studies overlap in their assertion of distinct microglial phenotypes in DR, providing novel insights into the cellular and molecular underpinnings of DR pathogenesis.
3.7 Astrocytes
Astrocytes, glial cells that support neuronal function and maintain the integrity of the blood-retinal barrier, are essential for retinal homeostasis. These cells exhibit a dynamic response to injury and inflammation (Xia et al., 2022). ScRNA-seq enables precise characterization of astrocytes, differentiating them from other glial cell types based on specific marker genes (Shi et al., 2023). This technique has elucidated molecular alterations in astrocytes under diabetic conditions, including changes in gene expression related to neuroinflammation and gliosis (Pun et al., 2023). Through scRNA-seq, researchers have investigated the role of astrocytes in DR, focusing on their involvement in inflammation and neurovascular damage. Diabetes has been shown to induce the upregulation of immediate early genes in retinal astrocytes (Shi et al., 2023). By identifying a subset of ECs exhibiting heightened deregulation, specifically in diabetic retinas, termed DRECs, researchers have elucidated pathways associated with these genes. These findings underscore the substantial contribution of astrocytes to DR progression (Pun et al., 2023).
3.8 Macrophage
Macrophages, differentiated mononuclear phagocytes, are essential for inflammation, tissue repair, and immune responses. These cells phagocytose pathogens, cellular debris, and apoptotic cells, and secrete cytokines to regulate immune function (Wu et al., 2021). In DR, macrophages contribute to neuroinflammation and tissue damage by releasing pro-inflammatory cytokines and mediating immune responses. ScRNA-seq identifies macrophages based on their expression of marker genes such as CD68 and CD163, as well as various cytokines, revealing gene expression profiles associated with phagocytosis, antigen presentation, and inflammation (Cochain et al., 2018).
Van Hove et al. (2020) identified significant enrichment of pathways associated with the activation of inflammatory monocyte-derived macrophages, microglia, macrophages, leukocytes, and lymphocytes, as well as monocyte and leukocyte differentiation. These findings suggest a potential contribution to DR progression. Conversely, extensive photoreceptor cell death in the diabetic retina may induce macrophage migration to clear cellular debris. Further analysis of inflammatory cells revealed a macrophage subcluster expressing proangiogenic cytokines, implicating these cells in angiogenesis (Corano Scheri et al., 2023). Monocyte-derived macrophages are implicated in upregulating VEGF receptor 1 (VEGFR1) (Uemura et al., 2021) in various tissues, including the retina. Activation of VEGFR1 in these mononuclear phagocytes enhances their production of pro-inflammatory and pro-angiogenic cytokines such as CC chemokine ligand 2, interleukin-1β, interleukin-6, tumor necrosis factor-α, and VEGFA (Van Bergen et al., 2019). Based on these findings, targeting ischemia-related M1-like macrophages may represent a novel therapeutic approach for DR.
3.9 Pericytes
While pericyte degeneration is a feature of other diseases, its severity in the retina during DR suggests the involvement of unique intraocular microenvironmental factors in pericyte loss (Spencer et al., 2020). ScRNA-seq profiling of pericytes has the potential to illuminate pathways essential for their survival and function, thereby identifying therapeutic targets to preserve retinal vascular integrity (Zhang et al., 2022). Studies of DR patients have identified a subpopulation of pericytes undergoing transdifferentiation into myofibroblasts within the stromal cell compartment (Corano Scheri et al., 2023). Adipocyte Enhancer-binding Protein 1 (AEBP1) exhibits significant upregulation in these myofibroblast clusters, suggesting its involvement in this transformation process. Experiments exposing human retinal pericytes to high-glucose conditions have confirmed this molecular shift, with siRNA-mediated knockdown of AEBP1 attenuating the expression of profibrotic markers. These findings provide critical insights into the molecular mechanisms underlying pericyte dysfunction in DR.
3.10 Bipolar and amacrine cells
Bipolar and amacrine cells integrate signals from photoreceptors and relay them to RGCs (Soni et al., 2021). Studies (Shekhar et al., 2016; Matosin et al., 2023) indicate that BCs are particularly susceptible to hyperglycemia. While diabetic mouse retinas exhibit BC-intrinsic defense mechanisms against diabetes-induced degeneration, a recent study Chen et al. (2023b) employed massively parallel scRNA-seq and computational analysis to categorize approximately 25,000 mouse retinal BCs into 15 subtypes. These included previously characterized subtypes and two novel subtypes, one displaying atypical morphology and localization (Ising et al., 2019).
ScRNA-seq is a systematic approach enabling comprehensive molecular classification of neurons, identification of novel neuronal subtypes, and elucidation of intra-class transcriptional heterogeneity. This technique is instrumental in advancing our comprehension of the cellular and molecular mechanisms underlying DR, particularly in understudied cell types such as BCs.
4 Application of scRNA-seq with multi-omics for DR research
Omics analyses aim to characterize the diverse molecules essential to life, but individual omics datasets often fail to capture the complexity of molecular interactions. Multi-omics integrates data from genomics, transcriptomics, proteomics, metabolomics, lipidomics, epigenomics and spatial transcriptomics to provide a comprehensive perspective (Beneyto-Calabuig et al., 2023). This holistic approach facilitates the exploration of relationships across biological levels, deepening our understanding of molecular changes in development, cellular responses, and disease (Gan et al., 2024). Disruptions in cellular metabolic pathways, such as glucose, amino acid, and lipid metabolism, are also recognized as key pathogenic mechanisms in DR (Sun et al., 2021). Advances in technology, computational tools, and commercial platforms have significantly accelerated multi-omics analysis (Zhang Y et al., 2024). The emergence of single-cell omics extends this approach to the individual cell level, offering unprecedented insights into gene regulation (Sun et al., 2024). This integrated methodology has revealed intricate interactions between cell types and gene expression, providing novel perspectives on DR, as depicted in Figure 3. The identification of unique cell clusters and their transformations in DR facilitates a deeper understanding of the disease’s pathogenesis and potential therapeutic interventions (Figure 4).
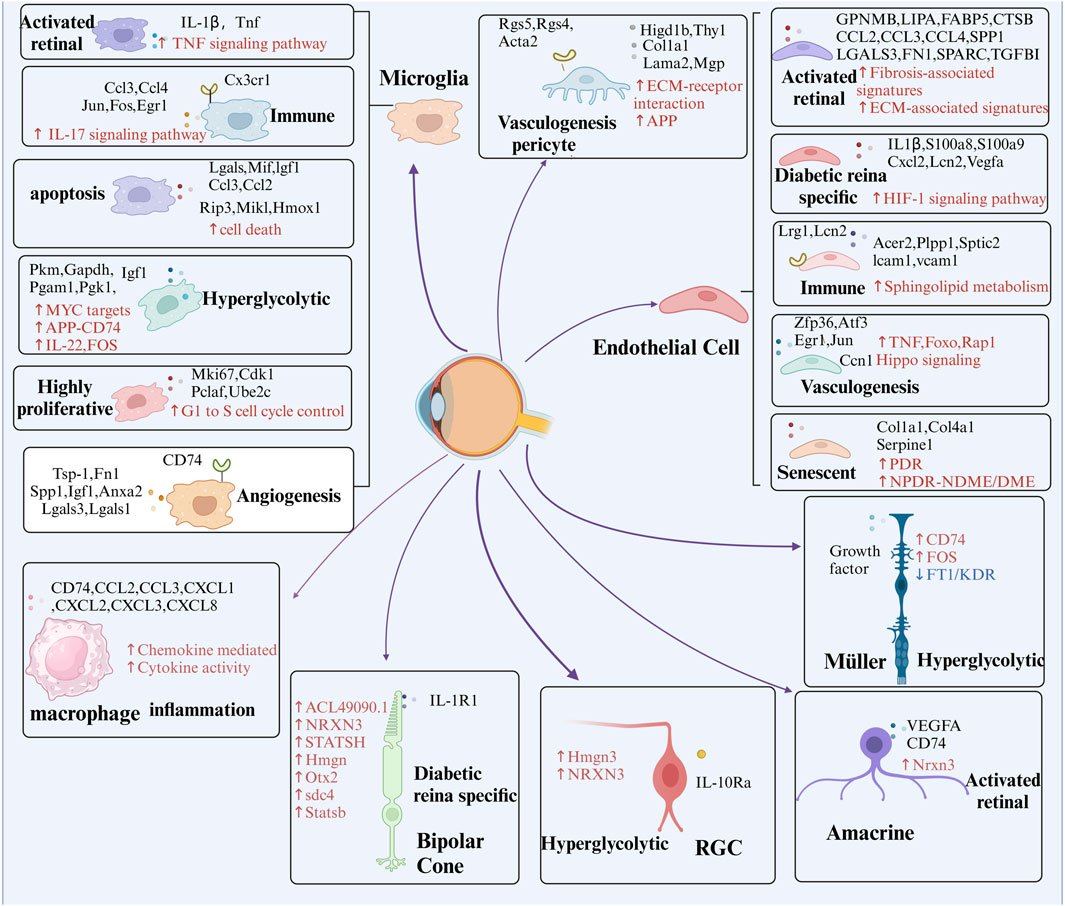
Figure 4. Presents the identification of key cell subpopulations in DR through multi-omics analysis. The figure highlights distinct cell subpopulations to emphasize the cellular heterogeneity observed during DR progression, offering insights into the specific roles of different cell clusters in the disease’s pathophysiology. Red arrows and text represent pathways that are significantly upregulated within these subpopulations, while blue arrows and text signify pathways that are downregulated. Additionally, black text denotes unique intracellular or secreted proteins, as well as membrane receptors, that are specifically expressed within each subpopulation.
4.1 ScRNA-seq combined with genomics
Advances in genetic research have elucidated the complex mechanisms underlying DR (Kang and Yang, 2020). Integrating scRNA-seq with genetic association studies offers a powerful approach to identifying susceptibility genes for DR (Corano Scheri et al., 2023). Traditionally, gene expression signatures have been derived from conventional molecular profiling methods, such as microarray and genomics, which assess average gene expression across entire cell populations. To correlate gene expression with genotype, researchers often integrate single-cell transcriptomes and single-cell genomes, as summarized in Table 3, which highlights their combined benefits. Primarily, this approach enables the delineation of cell lineage and evolution. By analyzing both cellular expression profiles and genetic variants, investigators can reconstruct lineage relationships between different cell types and trace the evolutionary paths of cell populations over time (Weber et al., 2021). Such knowledge is essential for comprehending the development of diseases characterized by cellular heterogeneity, including DR.
Furthermore, this integrated analysis facilitates the identification and characterization of cellular subpopulations. Researchers can identify and characterize specific subgroups defined by unique combinations of gene expression profiles and genetic mutations (Valecha and Posada, 2022). This capability is invaluable for studying the cellular diversity within complex tissues and identifying novel targets for therapeutic intervention. In contrast, scRNA-seq offers detailed profiling at the individual cell level, unveiling gene expression differences within distinct cell types. Consequently, scRNA-seq facilitates the identification of novel biomarkers associated with DR progression and response to targeted therapies.
One study (Zhang et al., 2019) presents the first unbiased and comprehensive cellular and transcriptional characterization of PDR retinal neovascular (RNV) membranes obtained during vitreoretinal surgery. They findings underscore the pivotal role of M2 macrophages in RNV formation and highlight the significant contribution of hyalocytes to the myofibroblast population. Further investigation is anticipated to identify additional factors that can improve prognostic prediction and uncover novel therapeutic targets. The integration of scRNA-seq with multi-omics methodologies holds the potential to revolutionize DR treatment by providing a deeper understanding of the cellular and molecular mechanisms underlying the disease, thereby facilitating the development of precise and efficacious therapeutic interventions.
Population-based GWAS have consistently identified multiple genetic variants associated with DR (McIntosh et al., 2024). Similar to other complex diseases, such as age-related macular degeneration, many of these DR-associated variants are located within non-coding regions and exert crucial regulatory functions on gene expression (Herrera-Luis et al., 2022). Notably, single nucleotide polymorphisms identified through meta-GWAS of DR are enriched in DNase hypersensitivity sites, suggesting their potential to influence gene expression by altering chromatin states or transcription factor binding (Khan et al., 2020).
Research by Chen et al. (2023b) identified rod BCs, cone BCs, and amacrine cells as retinal neuronal cell types most strongly associated with DR risk. The gene Neurexin 3, a DR risk candidate, is primarily expressed in interneurons, suggesting their critical role in DR pathogenesis. Subsequent research by Niu et al. (2021) employed scRNA-seq to classify 11 retinal cell types and analyze the cell-type-specific expression of DR-associated loci. These studies unveiled diverse expression patterns, including elevated acid-sensing ion channel five in specific cell clusters and altered expression of genes such as Fos, madd, and pttg1 across multiple cell types. In diabetic mice, Retinaldehyde Binding Protein 1 (RLBP1), encoding Cellular Retinaldehyde-Binding Protein, exhibited decreased expression in Müller glia but increased expression in other retinal cells. Furthermore, Calcium Voltage-Gated Channel Subunit Alpha1 H, encoding a calcium channel subunit, was downregulated in cone BCs during DR.
Importantly, research on proliferative DR in mice revealed associations between amyloid-beta precursor protein (APP) gene expression and Alzheimer’s disease (AD) risk genes identified through GWAS (Xu et al., 2023). APP expression exhibited negative correlations with SCIMP, ABI3, ABCA7, and APOE, and positive correlations with MINDY2 and ADAMTS1, suggesting a potential link to AD risk.
These findings offer a comprehensive characterization of the retinal environment under both diabetic and normal conditions, identifying novel pathogenic factors that may serve as therapeutic targets for DR and related disorders.
4.2 ScRNA-seq combined with transcriptomics
Alternative transcription starts sites (TSS) generate diverse 5′-UTR isoforms, influencing mRNA stability and translation (Golubnitschaja et al., 2024). Techniques such as Cap Analysis of Gene Expression and TSS-seq measure TSS in bulk tissues but mask cellular diversity, whereas scRNA-seq can profile cell-type-specific changes (Stuart et al., 2019). scRNA-seq enables detailed gene expression profiling at the individual cell level, identifying ligand-receptor interactions and decoding intercellular communication networks (Zhu et al., 2023). scRNA-seq provides detailed gene expression profiles at the individual cell level, revealing cellular heterogeneity and rare cell types, although with greater complexity and cost (Liu et al., 2024). In contrast, traditional bulk RNA sequencing provides simpler, cost-effective, and robust average expression profiles yet cannot resolve to detect differences between individual cells (Tan Y et al., 2023). Table 4 provides a comparison of scRNA-seq and bulk RNA analysis.
Bulk RNA-seq and scRNA-seq are often employed synergistically in multi-omics studies. While bulk RNA-seq provides a global gene expression overview, it lacks the resolution to discern gene expression at the single-cell level. Conversely, scRNA-seq excels at identifying distinct cell subpopulations but does not directly correlate these with specific phenotypes (Tan Z et al., 2023; Yu et al., 2022). By integrating both methods, researchers can comprehensively map the transcriptomic landscape, revealing cell-specific gene expression patterns and enabling the identification of cell subpopulations linked to particular phenotypes. This comprehensive approach is instrumental in elucidating disease progression and informing the development of targeted therapies (Wang, Kumar and Liu, 2021).
Integrating scRNA-seq with transcriptomics and other omics approaches provides a comprehensive framework for elucidating the cellular and molecular underpinnings of DR (Xia et al., 2023). Through multi-omic analyses, researchers have successfully identified specific cell types, signaling pathways, and potential therapeutic targets implicated in DR pathogenesis.
Mao et al. (2023) identified alternative TSS in retinal cells, underscoring the significance of 5′-UTRs in post-transcriptional regulation. Corano Scheri et al. (2023) characterized cellular composition within preretinal fibrovascular membranes, implicating endothelial, inflammatory, and stromal cells in angiogenesis and fibrosis. Becker et al. (2021) identified DEGs and pathways associated with late-stage DR, suggesting potential therapeutic targets. Investigations in diabetic models revealed dynamic changes in retinal cell populations and macroglia function, linking these cell types to retinal neurodegeneration (Li et al., 2021; Van Hove et al., 2020). Additionally, transcriptomic analysis of circulating immune cells identified Jun Proto-Oncogene D (JUND) as a key player in DR pathogenesis. Xiao et al. (2021) investigated ligand-receptor interactions in non-human primate retinas, establishing the retinal interactome and identifying TNF-α signaling in microglia activation. Deng et al. (2024) identified Müller cells as pivotal interaction hubs in the retinal microenvironment, with diverse transcriptional responses to DR.
These findings underscore the potential for developing therapeutic interventions by targeting specific cellular and molecular pathways implicated in DR. The identification of intricate cellular interactions and the discovery of novel therapeutic targets offer promising avenues for future research and clinical translation.
4.3 ScRNA-seq combined with metabolomic
In DR, chronic hyperglycemia disrupts the retinal metabolic microenvironment, an essential regulator of retinal cell function (Zhang J et al., 2022). Recent advancements in methodology have enabled numerous studies combining scRNA-seq with metabolomics to investigate the pathophysiology of DR using patient samples (Das et al., 2015).
Metabolomics, the comprehensive analysis of metabolites within biological samples, emerges as a promising omics tool for unraveling metabolic alterations and underlying pathophysiology in diseases such as DR. Recent studies (Eid et al., 2019; Pfeifer et al., 2023) have identified a panel of metabolites, including L-glutamine, L-lactic acid, pyruvic acid, acetic acid, L-glutamic acid, D-glucose, L-alanine, L-threonine, citrulline, L-lysine, and succinic acid, as potential biomarkers for DR, shedding light on novel disease pathways.
Serum metabolite and metabolic pathway analyses were conducted across different stages of DR in patients with type 2 diabetes mellitus. Compared to controls, DR patients from the Asian population exhibited dysregulated pathways, including arginine biosynthesis, linoleic acid metabolism, glutamate metabolism, and D-glutamine and D-glutamate metabolism. Notably, elevated levels of glutamate (Ren et al., 2024), aspartate (Zhu et al., 2018), glutamine (Yeh et al., 2020), N-acetyl-L-glutamate (Plumbly et al., 2019), and N-acetyl-L-aspartate (Ahmad et al., 2019), along with decreased levels of dihomo-gamma-linolenate, docosahexaenoic acid, and eicosatetraenoic acid, were identified as potential metabolic signatures capable of differentiating proliferative from non-proliferative DR within this population.
Advances in metabolomics technologies are illuminating critical pathways, biomarkers, and therapeutic targets for DR management (Xu et al., 2022). These insights deepen our comprehension of the disease and provide a foundation for innovative treatment strategies.
4.4 ScRNA-seq combined with lipidomics
Accumulating evidence underscores lipid metabolism disruption as an early hallmark in the pathogenesis of diabetic complications (Eid et al., 2019). Diverse lipid species, including glycerophospholipids (Jin et al., 2024), sphingolipids (Motloch et al., 2024), and glycerolipids (Singer et al., 2022), have been implicated as critical risk factors for DR and its associated sequelae. Conversely, reduced very long-chain ceramide levels have been linked to the onset of macroalbuminuria in diabetes. Accelerated sphingolipid catabolism, resulting in elevated glucosylceramide or glycosphingolipid concentrations, may contribute to the neuronal pathologies characteristic of DR (Ancel et al., 2023). Additionally, sphingomyelin, a lipid connected to insulin resistance and an independent predictor of cardiovascular disease, is formed by the transfer of a phosphocholine group from phosphatidylcholine to the ceramide backbone (Fernandes Silva, et al., 2023). Collectively, these findings highlight the substantial contribution of dysregulated lipid metabolism to the development of DM and its complications, although the precise lipid species driving DR remain to be fully elucidated (Pratama et al., 2022).
Lipidomic analysis, when integrated with scRNA-seq, offers a robust framework for the identification of novel lipid mediators implicated in lipid metabolism and associated biochemical processes, thereby unveiling promising avenues for disease prediction and detection (Bertelli et al., 2022). Using a quantitative metabolomics approach, Maria et al. (Bertelli et al., 2022) conducted a comparative analysis of metabolite concentrations in aqueous humor and serum from elderly individuals with and without diabetes who underwent cataract surgery.
To comprehensively characterize local metabolic alterations in activated microglia and illuminate the metabolic milieu contributing to immune responses, Lv et al. (2022) conducted integrated lipidomics and RNA profiling analyses on microglial cell line models representative of the DR microenvironment. Their findings unveiled a substantial accumulation of TAGs within activated microglia (Yousri et al., 2022). Notably, lipopolysaccharide-stimulated macrophages exhibited a similar increase in TAG synthesis (Ren et al., 2023). These observations suggest that TAG accumulation in activated microglia may serve as a potential therapeutic target for mitigating inflammation in DR.
Within the context of diabetic retinopathy, the integration of scRNA-seq with targeted metabolomic analysis has demonstrated efficacy in the precise identification of disease-associated biomarkers.
4.5 ScRNA-seq combined with proteomics
While scRNA-seq has yielded promising insights, its findings are limited to the transcriptomic level. A comprehensive protein expression profile is crucial for understanding cell subpopulations in DR (Fu et al., 2023). There is a strong need for single-cell proteomics, capable of detecting and quantifying over 1,000 proteins within a single mammalian cell.
High-throughput proteomics has become a valuable tool in ophthalmic research, particularly in studying DR (Starr et al., 2023). Researchers have analyzed various samples, including tears, serum, AH, VH, and retina, using techniques like mass spectrometry and liquid chromatography (Varughese and Jacob, 2023). These studies have identified key DR biomarkers, such as VEGF, IL-6, and ICAM-1. While serum and VH are directly connected to the retina, their collection can be invasive, unlike serum and tear samples (Gurel and Sheibani, 2018). Proteomic changes in these biofluids not only enhance understanding of DR pathogenesis but also hold potential for personalized medicine (Xu et al., 2014). For example, elevated plasma kallikrein in VH may suggest that standard anti-VEGF treatments are ineffective for certain patients. Wang et al. (2024) provide significant insights into the cellular and molecular mechanisms underlying PDR by identifying potential biomarkers and therapeutic targets through an integrated approach involving scRNA-seq, machine learning, AlphaFold protein structure predictions, and molecular docking techniques. Abbas et al. (2024) found scRNA-seq and proteomics are advancing our understanding of DR by enabling detailed analysis of microvesicle cargo and their roles in modulating inflammation, oxidative stress, and immune responses, thereby supporting the development of MV-based biomarkers and therapies for DR.
4.6 ScRNA-seq combined with other multi-omics
Ocular neovascularization can affect nearly all tissues of the eye, including the cornea, iris, retina, and choroid (Voight et al., 2010). Pathological neovascularization is a primary cause of vision loss in prevalent ocular diseases such as DR, retinopathy of prematurity, and age-related macular degeneration (Lin et al., 2023). Glycosylation, the most common covalent post-translational modification of proteins in mammalian cells, plays a significant role in angiogenesis (Zelniker et al., 2019). Emerging evidence indicates that glycosylation affects the activation, proliferation, and migration of ECs, as well as the interactions between angiogenic ECs and other cell types essential for blood vessel formation (Lin et al., 2023). Recent studies suggest that members of the galectin family, which are β-galactosidase-binding proteins, modulate angiogenesis through novel carbohydrate-based recognition systems (Ko and Moon, 2023). These systems involve interactions between the glycans on angiogenic cell surface receptors and galectins.
Recent research (Netea et al., 2020; Wimmers et al., 2021) underscores the epigenomes central role in regulating fundamental biological processes by maintaining specific chromatin states over extended periods, enabling the durable storage of gene-expression information (Khan et al., 2020). Integrating scRNA-seq with epigenomic SNP-to-gene maps, as proposed by Chen et al. (2023b), provides a comprehensive framework to identify cell types and processes affected by genetic variants, offering new insights into DR pathogenesis and potential therapeutic targets. GWAS have identified genetic variants associated with DR, helping to clarify disease susceptibility (Skol et al., 2020). Researchers have assessed DR risk genes across various cell types to identify those most vulnerable to DR, emphasizing the sensitivity of bipolar, amacrine, and Müller glial cells. Since many DR-related variants lie in noncoding regions, their interpretation using scRNA-seq alone is limited (Jagadeesh et al., 2022). By linking GWAS data with scRNA-seq and tissue-specific enhancer-gene mappings, researchers can detect tissue-specific SNP-to-gene associations that reveal differences in disease heritability across cell types. Furthermore, analyzing disease-specific cell states in both healthy and diseased tissues highlights cell-type changes linked to DR. Finally, non-negative matrix factorization enables the identification of cellular process programs—such as MAPK signaling—that extend across cell types, deepening our understanding of DR pathogenesis at cellular and molecular levels.
Spatial gene expression is an advancing technology that has recently seen the development of commercial systems capable of achieving true single-cell or subcellular resolution of gene expression within a spatial transcriptomics (Voigt et al., 2023). However, these systems often rely on probe-based hybridization, which requires the design of custom gene panels (Feng et al., 2024). Unbiased sequencing-based platforms are also progressing, with improvements in spot resolution approaching near single-cell levels (Marneros, 2023). This study demonstrates the utility of spatial technology in investigating gene expression within focal pathological areas and along regional gradients in the heterogeneous retina, RPE, and choroid. Ongoing research, coupled with technological advancements, will continue to enhance our understanding of the pathogenic mechanisms underlying macular neovascularization and other regional retinal diseases (Chen X et al., 2024). The insights gained from this powerful technology may inform the development of future targeted therapies, potentially reducing vision loss associated with DR.
High-throughput technologies and omics data, such as glycolipidosis, proteomics, and spatial transcriptomics analysis, have advanced our understanding of DR by identifying risk factors and developing novel biomarkers (Tolentino et al., 2023). By integrating these data through bioinformatics, researchers gain comprehensive insights into the susceptibility genes, mechanistic pathways, and disease stage markers of DR (Kakihara et al., 2023). However, scRNA analyses of glycolipidosis, epigenomes and spatial transcriptomics in DR remain underreported.
5 The advantages and challenges of scRNA-seq with multi-omics in DR research
5.1 The advantages of scRNA-seq
5.1.1 Computational resources: Databases of interacting proteins
CellPhone DB, a newly established public resource, comprehensively catalogs ligands, receptors, and their interactions to facilitate the analysis of cell-cell communication molecules (Chen et al., 2022). This framework leverages single-cell transcriptomic data to quantify ligand and receptor expression across diverse cell types, identifying cell-type-specific ligand-receptor pairs through empirical shuffling (Xu et al., 2023a). Unlike many databases, CellPhone DB accurately represents heteromeric complexes by considering the subunit architecture of both ligands and receptors (Qin et al., 2023).
The latest CellPhone DB update incorporates enhanced functionalities, facilitating the inclusion of novel interacting molecules and streamlining analyses of extensive datasets (Cabrera et al., 2022). These advancements significantly improve the annotation of intricate ligand-receptor interactions using scRNA-seq data. Future research integrating scRNA-seq with CyTOF and CellPhone DB holds the potential to uncover novel disease mechanisms (Yang et al., 2023). Additionally, optimization methods like SoptSC and tools from the FANTOM5 project offer alternative approaches to deciphering cell-cell relationships from single-cell data (Sørensen et al., 2023). This approach enables a more comprehensive and precise investigation of previously unknown cell-cell interactions, offering opportunities for further validation beyond traditional methods. In this context, we outline the cell-cell communication observed in DR using scRNA-seq from prior studies, highlighting the enhanced interactions between retinal cells under hyperglycemic conditions and the intercellular crosstalk identified among cells within fibrovascular membranes (FVMs) (Figure 4).
5.1.2 Modeling and assessing predicted interaction networks
Single-cell multi-omics methodologies provide a holistic perspective on cellular expression, function, and identity, though a complete comprehension of these intricate systems remains elusive (Pelka et al., 2021). While a substantial portion of research has focused on utilizing scRNA-seq to elucidate communication networks through ligand-receptor interactions within the complex DR microenvironment (Luo et al., 2023), recent endeavors by Chen and Mar have delved into evaluating the efficacy of single-cell network modeling techniques in uncovering established interaction networks within single-cell datasets (Zhang et al., 2021). These methods, including SCENIC, SCODE, and PIDC, employ disparate approaches, respectively, leveraging co-expression networks with bioinformatics knowledge, ordinary differential equations, and mutual information-based strategies (Jumeau et al., 2018).
A comparative analysis of existing methods revealed significant limitations in accurately predicting network structures from single-cell expression data (Saint-Antoine and Singh, 2020). While these approaches offer valuable insights, they consistently failed to achieve high predictive accuracy, and the resulting networks exhibited substantial variability across different methodologies (Deligiannidis et al., 2023; Karyotaki et al., 2021). This inconsistency poses a considerable challenge for accurately predicting cell-cell communication networks, as the choice of modeling approach can substantially influence the outcomes (Hussain et al., 2021).
Although this review does not delve deeply into the intricacies of these modeling methodologies, a comprehensive exploration of their predictive capabilities is indispensable for a thorough understanding of their potential applications and limitations.
5.1.3 Validating causal relationships
Integrating single-cell multi-omics data with perturbation experiments, such as RNA interference or CRISPR-Cas9, offers a robust approach for validating causal regulatory programs (Bowling et al., 2020). Recent advancements in high-throughput gene technologies, exemplified by Perturb-seq, have merged CRISPR/Cas9-mediated gene perturbation with single-cell sequencing (Li et al., 2023), demonstrating comparable efficacy in identifying causal relationships to traditional RNAi or CRISPR-Cas9-based gene activation/deletion methods while exhibiting reduced invasiveness (Raj et al., 2018).
Advances in single-cell technologies are poised to expand the scope of cellular parameters measurable at the individual cell level (Choi, 2020). Concurrent multi-modal profiling within single cells holds great promise for predicting drug sensitivities in retinal cells, potentially obviating the need for extensive in vivo or in vitro experimentation (Pei et al., 2020). The application of these techniques within the framework of single-cell multi-omics studies on DR has the potential to significantly enhance our comprehension of the disease and accelerate the development of targeted therapeutic interventions (Bucheli et al., 2021; Wang et al., 2020).
5.2 The challenges in scRNA-seq
5.2.1 Cell dissociation protocols
The mammalian retina is a complex tissue composed of interconnected neurons, glial cells, and photoreceptors (Ye et al., 2020). Achieving high cell viability and quality in scRNA-seq studies of DR is particularly challenging due to the retina’s delicate nature (Lin et al., 2024). The requisite process of cell dissociation to isolate individual cells for sequencing can induce cellular stress and damage, potentially distorting gene expression profiles and introducing spurious artifacts that obscure authentic biological signals (Yao et al., 2022).
Preserving cell viability and minimizing stress during dissociation are paramount for accurately capturing gene expression profiles that reflect in vivo conditions (Raj et al., 2018). Such precision is indispensable for elucidating the cellular and molecular underpinnings of DR. Photoreceptors, characterized by their delicate structure, are susceptible to both enzymatic and mechanical dissociation, resulting in RNA leakage from compromised cells (VanHorn and Morris, 2021). Notably, disease states such as DR may render cells even more fragile during isolation.
Obtaining high-quality, viable retinal cells is paramount for accurately identifying cell types most affected by DR. Data derived from damaged or stressed cells can lead to unreliable results, potentially misrepresenting cell vulnerability or resilience (Peng et al., 2020). Precise gene expression profiling is essential for discovering biomarkers and therapeutic targets. Artifacts introduced by cell stress or damage can generate false positives or negatives, impeding the identification of meaningful biomarkers and targets (Wang et al., 2023). Moreover, maintaining high cell viability and quality is crucial for the reproducibility of scRNA-seq studies. Consistent and reliable data across multiple studies and laboratories are fundamental for validating findings and advancing our understanding of DR (Beltrami et al., 2022).
5.2.2 Spatial and temporal information
Human NR are light-sensitive tissues distinguished by a complex spatial and cellular architecture (Lukowski et al., 2019). While single-cell omics technologies offer a comprehensive characterization of cellular types and states, the intricate functionality of tissues is profoundly shaped by the spatial disposition of these cells (Quadrato et al., 2017). Despite its capacity to analyze cellular interactions, scRNA-seq is unable to delineate cell localization or spatial context (Lancaster et al., 2013). Cells residing within local microenvironments exert specific physiological roles through juxtacrine and paracrine signaling, as well as intercellular interactions (Cohen et al., 2021).
The retina’s precise spatial organization is fundamental to its function, and disruptions caused by infections, inflammation, or injuries can significantly alter this architecture, impacting visual acuity (Deng et al., 2024). Understanding these organizational changes is crucial for diagnosing and treating retinal diseases. Traditional histopathological techniques, complemented by in situ hybridization and immunohistochemistry (Md Pauzi et al., 2021), examine tissue architecture and molecular markers. However, these methods are limited in their ability to comprehensively characterize the retina’s molecular landscape, as they can only assess a restricted number of transcripts or proteins per experiment.
Spatial context is paramount in comprehending complex tissue function, as cellular positioning and interactions within microenvironments significantly influence tissue behavior (Fang et al., 2022). Spatial transcriptomics offers unprecedented insights into disease progression by mapping cellular and tissue-level changes across time and space (Cao et al., 2023). This technology enables the identification of disease-specific microenvironments within the retina, revealing how local cellular contexts contribute to disease pathology (Liu et al., 2023). By elucidating the spatial distribution of gene expression alterations, researchers can uncover novel therapeutic targets and develop treatments precisely targeted to affected tissue regions (Geng et al., 2021).
5.2.3 Intercellular communication
The retina’s unique architecture, characterized by complex cellular interactions and signaling pathways, distinguishes it from other tissues, including the brain (Huang et al., 2023). This intricate organization has historically limited investigations into cell-cell communication within the retina, particularly in the context of DR development (Li et al., 2023; Lou et al., 2022; Yi et al., 2021). Nevertheless, comprehending these intercellular relationships is indispensable for elucidating the mechanisms underlying retinal degeneration (Figure 5).
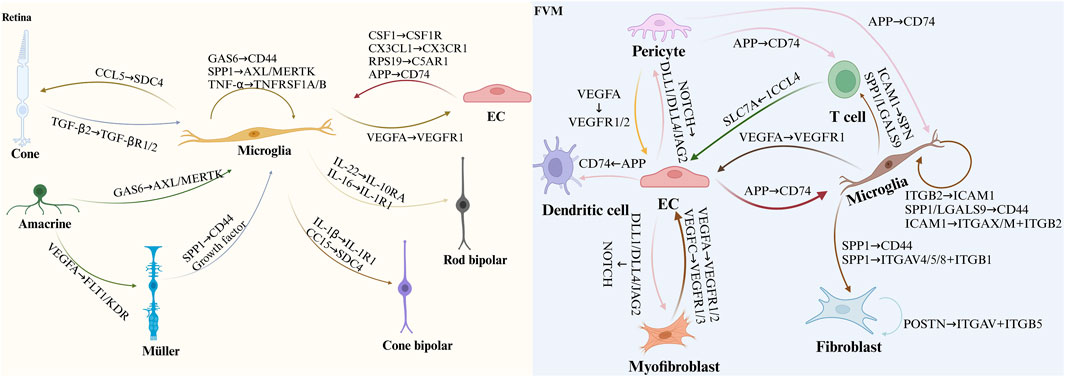
Figure 5. Illustrates cell-cell communication in DR as uncovered by scRNA-seq. The figure depicts ligand-receptor interactions associated with DR, based on findings from previous scRNA-seq studies. In the diagram, the arrowhead points to the receptor protein and the corresponding cell expressing it, while the arrowtail indicates the ligand protein and the cell producing it. This representation sheds light on the disrupted communication networks in diabetic retinas and the intercellular signaling within FVM, which may contribute to the pathogenesis of DR.
Normally, the retina is protected from systemic circulation by the blood-retinal barrier, composed of the RPE in the outer retina and ECs, pericytes, and astrocyte end-feet in the inner retina (Voigt et al., 2019). While various retinal cell types exhibit differential susceptibility to DR in mice, Müller glia and clusters of rods, rod BCs, cones, and vascular ECs are prominently involved in proliferative DR pathogenesis (Mullin et al., 2023; Voigt et al., 2021b). The precise role and activation mechanisms of immune cells in DR remain to be fully elucidated (Nishikawa and Koyama, 2021).
Exploring key retinal cell types and their interactions holds promise for developing novel therapeutic approaches not only for DR but also for other neurodegenerative diseases affecting the central nervous system (Niu et al., 2021). In both diabetic and healthy mice, RGCs, vascular ECs, and Müller glia exhibit significant activity (Tresenrider et al., 2023; Yao et al., 2023). Notably, microglial involvement in retinal cell-cell communication networks is markedly increased in DR mice compared to controls (Parikh et al., 2023). These findings emphasize the critical role of understanding cellular interactions and the functions of various cell types in DR pathogenesis (Beilke et al., 1991). Such knowledge could lead to the identification of promising therapeutic targets for disease management and visual function preservation (Tan et al., 2021).
6 Future outlook
Recent advancements in scRNA-seq have significantly deepened our understanding of DR by providing comprehensive molecular profiles of retinal cell types, including photoreceptors, ECs, and pericytes, integral to blood-retinal barrier integrity (Chen et al., 2024; Rangasamy et al., 2020; Sun et al., 2021; Zhan, 2023). Profiling these cells reveals critical pathways for their survival and function, identifying potential therapeutic targets. Data-sharing platforms like the Single Cell Portal and Spectacle database facilitate the integration of diverse datasets, aiding in elucidating DR pathogenesis (Corano Scheri et al., 2023). Addressing data heterogeneity through tools such as SIDA and Harmony is imperative for analyzing large-scale scRNA-seq data (Paik et al., 2020). While current studies predominantly offer gene expression snapshots, longitudinal studies and spatial transcriptomics are essential for comprehending DR dynamics and evaluating therapeutic efficacy (Fadakar et al., 2024). Liquid biopsy, particularly exosome analysis, shows promise for early detection. Integrating multi-omics approaches, encompassing transcriptomic, proteomic, and metabolomic data, will provide a comprehensive understanding of DR mechanisms and inform the development of effective treatments (Manochkumar et al., 2023; Xu et al., 2023).
7 Conclusion
ScRNA-seq is a revolutionary technology enabling transcriptomic analysis at the individual cell level, providing unprecedented insights into the complex cellular heterogeneity of DR. By revealing cellular landscapes, identifying biomarkers, and informing treatment strategies, scRNA-seq is accelerating the development of precision medicine for DR. As technology advances, integration with other omics approaches, particularly spatial gene expression, holds the potential to unlock deeper understanding of gene regulatory networks and disease mechanisms. While challenges remain, the synergy between laboratory research and clinical applications promises to optimize patient outcomes through the development of targeted interventions.
Author contributions
XL: Conceptualization, Data curation, Formal Analysis, Methodology, Writing–original draft, Writing–review & editing. XD: Investigation, Writing–review & editing. WZ: Conceptualization, Supervision, Writing–review & editing. ZS: Conceptualization, Investigation, Writing–review & editing. ZL: Conceptualization, Writing–original draft. YS: Conceptualization, Writing–original draft. LL: Supervision, Writing–review & editing. NN: Conceptualization, Validation, Writing–review & editing. YM: Project administration, Resources, Writing–original draft.
Funding
The author(s) declare that financial support was received for the research, authorship, and/or publication of this article. This study was funded by the Clinical Research Center of the First People's Hospital of Yunnan Province (No. 2023YJZX-LN01), “The Kunming University of Science and Technology School of Medicine Postgraduate Innovation Fund”, National Natural Science Foundation of China (82460210). This study also was supported by the Joint project of Yunnan Science and Technology Department - Kunming Medical University Applied Fundamental Research (No. 202201AY070001-264), and the Key Laboratory of Clinical Virology of First People's Hospital of Yunnan Province (No. 2023A4010403-02).
Acknowledgments
We would like to express our sincere gratitude to all figures were created with BioRender.com.
Conflict of interest
The authors declare that the research was conducted in the absence of any commercial or financial relationships that could be construed as a potential conflict of interest.
Publisher’s note
All claims expressed in this article are solely those of the authors and do not necessarily represent those of their affiliated organizations, or those of the publisher, the editors and the reviewers. Any product that may be evaluated in this article, or claim that may be made by its manufacturer, is not guaranteed or endorsed by the publisher.
References
Abbas, A., Almaghrbi, H., Giordo, R., Zayed, H., and Pintus, G. (2024). Pathogenic mechanisms, diagnostic, and therapeutic potential of microvesicles in diabetes and its complications. Archives Biochem. Biophysics 761, 110168. doi:10.1016/j.abb.2024.110168
Ahmad, A. F., Dwivedi, G., O'Gara, F., Caparros-Martin, J., and Ward, N. C. (2019). The gut microbiome and cardiovascular disease: current knowledge and clinical potential. Am. J. Physiology. Heart Circulatory Physiology 317 (5), H923–H938. doi:10.1152/ajpheart.00376.2019
Ancel, P., Martin, J. C., Doukbi, E., Houssays, M., Gascon, P., Righini, M., et al. (2023). Untargeted multiomics approach coupling lipidomics and metabolomics profiling reveals new insights in diabetic retinopathy. Int. J. Mol. Sci. 24 (15), 12053. doi:10.3390/ijms241512053
Ashraf, M., Sampani, K., Rageh, A., Silva, P. S., Aiello, L. P., and Sun, J. K. (2020). Interaction between the distribution of diabetic retinopathy lesions and the association of optical coherence tomography angiography scans with diabetic retinopathy severity. JAMA Ophthalmol. 138 (12), 1291–1297. doi:10.1001/jamaophthalmol.2020.4516
Bawa, G., Liu, Z., Yu, X., Qin, A., and Sun, X. (2022). Single-cell RNA sequencing for plant research: insights and possible benefits. Int. J. Mol. Sci. 23 (9), 4497. doi:10.3390/ijms23094497
Becker, K., Klein, H., Simon, E., Viollet, C., Haslinger, C., Leparc, G., et al. (2021). In-depth transcriptomic analysis of human retina reveals molecular mechanisms underlying diabetic retinopathy. Sci. Rep. 11 (1), 10494. doi:10.1038/s41598-021-88698-3
Beilke, M. A., In, D. R., Gravell, M., Hamilton, R. S., Mora, C. A., Leon-Monzon, M., et al. (1991). In situ hybridization detection of HTLV-I RNA in peripheral blood mononuclear cells of TSP/HAM patients and their spouses. J. Med. Virology 33 (1), 64–71. doi:10.1002/jmv.1890330113
Beltrami, E. J., Brown, A. C., Salmon, P. J. M., Leffell, D. J., Ko, J. M., and Grant-Kels, J. M. (2022). Artificial intelligence in the detection of skin cancer. J. Am. Acad. Dermatology 87 (6), 1336–1342. doi:10.1016/j.jaad.2022.08.028
Beneyto-Calabuig, S., Merbach, A. K., Kniffka, J.-A., Antes, M., Szu-Tu, C., Rohde, C., et al. (2023). Clonally resolved single-cell multi-omics identifies routes of cellular differentiation in acute myeloid leukemia. Cell Stem Cell 30 (5), 706–721.e8. doi:10.1016/j.stem.2023.04.001
Bertelli, P. M., Pedrini, E., Hughes, D., McDonnell, S., Pathak, V., Peixoto, E., et al. (2022). Long term high glucose exposure induces premature senescence in retinal endothelial cells. Front. Physiology 13, 929118. doi:10.3389/fphys.2022.929118
Blanchard, J. W., Akay, L. A., Davila-Velderrain, J., von Maydell, D., Mathys, H., Davidson, S. M., et al. (2022). APOE4 impairs myelination via cholesterol dysregulation in oligodendrocytes. Nature 611 (7937), 769–779. doi:10.1038/s41586-022-05439-w
Bowling, S., Sritharan, D., Osorio, F. G., Nguyen, M., Cheung, P., Rodriguez-Fraticelli, A., et al. (2020). An engineered CRISPR-cas9 mouse line for simultaneous readout of lineage histories and gene expression profiles in single cells. Cell 181 (6), 1410–1422. doi:10.1016/j.cell.2020.04.048
Bryl, A., Mrugacz, M., Falkowski, M., and Zorena, K. (2022). The effect of diet and lifestyle on the course of diabetic retinopathy-A review of the literature. Nutrients 14 (6), 1252. doi:10.3390/nu14061252
Bucheli, O. T. M., Sigvaldadóttir, I., and Eyer, K. (2021). Measuring single-cell protein secretion in immunology: technologies, advances, and applications. Eur. J. Immunol. 51 (6), 1334–1347. doi:10.1002/eji.202048976
Cabrera, J. P., Camino-Willhuber, G., Guiroy, A., Carazzo, C. A., Gagliardi, M., and Joaquim, A. F. (2022). Vertebral augmentation plus short-segment fixation versus vertebral augmentation alone in Kümmell's disease: a systematic review and meta-analysis. Neurosurg. Rev. 45 (2), 1009–1018. doi:10.1007/s10143-021-01661-8
Cao, N., Ouyang, H., Zhang, X., Xu, Y., Li, J., and Chen, Y. (2023). Integration of scRNA-Seq and bulk RNA-Seq uncover perturbed immune cell types and pathways of Kawasaki disease. Front. Immunol. 14, 1259353. doi:10.3389/fimmu.2023.1259353
Chen, B., Zou, J., Xie, L., Cai, Y., Li, B., Tan, W., et al. (2024a). WNT-inhibitory factor 1-mediated glycolysis protects photoreceptor cells in diabetic retinopathy. J. Transl. Med. 22 (1), 245. doi:10.1186/s12967-024-05046-5
Chen, K., Wang, Y., Huang, Y., Liu, X., Tian, X., Yang, Y., et al. (2023b). Cross-species scRNA-seq reveals the cellular landscape of retina and early alterations in type 2 diabetes mice. Genomics 115 (4), 110644. doi:10.1016/j.ygeno.2023.110644
Chen, S., Guo, D., Zhu, Y., Xiao, S., Xie, J., Zhang, Z., et al. (2024b). Amyloid β oligomer induces cerebral vasculopathy via pericyte-mediated endothelial dysfunction. Alzheimer's Res. & Ther. 16 (1), 56. doi:10.1186/s13195-024-01423-w
Chen, Y., Wang, H., Yang, Q., Zhao, W., Chen, Y., Ni, Q., et al. (2022). Single-cell RNA landscape of the osteoimmunology microenvironment in periodontitis. Theranostics 12 (3), 1074–1096. doi:10.7150/thno.65694
Cheng Y, Y., Zhang, M., Xu, R., Fu, L., Xue, M., Xu, C., et al. (2024). p53 accelerates endothelial cell senescence in diabetic retinopathy by enhancing FoxO3a ubiquitylation and degradation via UBE2L6. Exp. Gerontol. 188, 112391. doi:10.1016/j.exger.2024.112391
Chen X, X., Qin, X., Bai, W., Ren, J., Yu, Y., Nie, H., et al. (2024). Kavain alleviates choroidal neovascularization via decreasing the activity of the HIF-1α/VEGF-A/VEGFR2 signaling pathway and inhibiting inflammation. Adv. Pharm. Bull. 14 (2), 469–482. doi:10.34172/apb.2024.036
Choi, J. R. (2020). Advances in single cell technologies in immunology. BioTechniques 69 (3), 226–236. doi:10.2144/btn-2020-0047
Cochain, C., Vafadarnejad, E., Arampatzi, P., Pelisek, J., Winkels, H., Ley, K., et al. (2018). Single-cell RNA-seq reveals the transcriptional landscape and heterogeneity of aortic macrophages in murine atherosclerosis. Circulation Res. 122 (12), 1661–1674. doi:10.1161/CIRCRESAHA.117.312509
Cohen, Y. C., Zada, M., Wang, S.-Y., Bornstein, C., David, E., Moshe, A., et al. (2021). Identification of resistance pathways and therapeutic targets in relapsed multiple myeloma patients through single-cell sequencing. Nat. Med. 27 (3), 491–503. doi:10.1038/s41591-021-01232-w
Corano Scheri, K., Lavine, J. A., Tedeschi, T., Thomson, B. R., and Fawzi, A. A. (2023). Single-cell transcriptomics analysis of proliferative diabetic retinopathy fibrovascular membranes reveals AEBP1 as fibrogenesis modulator. JCI Insight 8 (23), e172062. doi:10.1172/jci.insight.172062
Das, A., McGuire, P. G., and Rangasamy, S. (2015). Diabetic macular edema: pathophysiology and novel therapeutic targets. Ophthalmology 122 (7), 1375–1394. doi:10.1016/j.ophtha.2015.03.024
Deligiannidis, K. M., Meltzer-Brody, S., Maximos, B., Peeper, E. Q., Freeman, M., Lasser, R., et al. (2023). Zuranolone for the treatment of postpartum depression. Am. J. Psychiatry 180 (9), 668–675. doi:10.1176/appi.ajp.20220785
Deng, X., Mo, Y., and Zhu, X. (2024). Deciphering Müller cell heterogeneity signatures in diabetic retinopathy across species: an integrative single-cell analysis. Eur. J. Med. Res. 29 (1), 265. doi:10.1186/s40001-024-01847-y
De Rop, F. V., Ismail, J. N., Bravo González-Blas, C., Hulselmans, G. J., Flerin, C. C., Janssens, J., et al. (2022). Hydrop enables droplet-based single-cell ATAC-seq and single-cell RNA-seq using dissolvable hydrogel beads. ELife 11, e73971. doi:10.7554/eLife.73971
Ding, S., Chen, X., and Shen, K. (2020). Single-cell RNA sequencing in breast cancer: understanding tumor heterogeneity and paving roads to individualized therapy. Cancer Commun (Lond) 40 (8), 329–344. doi:10.1002/cac2.12078
Eid, S., Sas, K. M., Abcouwer, S. F., Feldman, E. L., Gardner, T. W., Pennathur, S., et al. (2019). New insights into the mechanisms of diabetic complications: role of lipids and lipid metabolism. Diabetologia 62 (9), 1539–1549. doi:10.1007/s00125-019-4959-1
Fadakar, K., Rahmani, S., Tedeschi, T., Lavine, J. A., and Fawzi, A. A. (2024). Short term effect of pre-operative anti-VEGF on angiogenic and fibrotic profile of fibrovascular membranes of proliferative diabetic retinopathy. Investigative Ophthalmol. & Vis. Sci. 65 (4), 37. doi:10.1167/iovs.65.4.37
Fan, Y., and Pedersen, O. (2021). Gut microbiota in human metabolic health and disease. Nat. Rev. Microbiol. 19 (1), 55–71. doi:10.1038/s41579-020-0433-9
Fang, Z., Li, J., Cao, F., and Li, F. (2022). Integration of scRNA-seq and bulk RNA-seq reveals molecular characterization of the immune microenvironment in acute pancreatitis. Biomolecules 13 (1), 78. doi:10.3390/biom13010078
Feng, D.-C., Zhu, W.-Z., Wang, J., Li, D.-X., Shi, X., Xiong, Q., et al. (2024). The implications of single-cell RNA-seq analysis in prostate cancer: unraveling tumor heterogeneity, therapeutic implications and pathways towards personalized therapy. Mil. Med. Res. 11 (1), 21. doi:10.1186/s40779-024-00526-7
Fernandes Silva, L., Hokkanen, J., Vangipurapu, J., Oravilahti, A., and Laakso, M. (2023). Metabolites as risk factors for diabetic retinopathy in patients with type 2 diabetes: a 12-year follow-up study. J. Clin. Endocrinol. Metabolism 109 (1), 100–106. doi:10.1210/clinem/dgad452
Fu, Q., Jiang, H., Qian, Y., Lv, H., Dai, H., Zhou, Y., et al. (2023). Single-cell RNA sequencing combined with single-cell proteomics identifies the metabolic adaptation of islet cell subpopulations to high-fat diet in mice. Diabetologia 66 (4), 724–740. doi:10.1007/s00125-022-05849-5
Gan, G., Lin, S., Luo, Y., Zeng, Y., Lu, B., Zhang, R., et al. (2024). Unveiling the oral-gut connection: chronic apical periodontitis accelerates atherosclerosis via gut microbiota dysbiosis and altered metabolites in apoE-/- Mice on a high-fat diet. Int. J. Oral Sci. 16 (1), 39. doi:10.1038/s41368-024-00301-3
Gao, N., Hao, S., Huang, G., Hao, W., and Su, L. (2022). The integrated transcriptome bioinformatics analysis identifies key genes and cellular components for proliferative diabetic retinopathy. PLoS One 17 (11), e0277952. doi:10.1371/journal.pone.0277952
Geng, Z., Tao, Y., Zheng, F., Wu, L., Wang, Y., Wang, Y., et al. (2021). Altered monocyte subsets in kawasaki disease revealed by single-cell RNA-sequencing. J. Inflamm. Res. 14, 885–896. doi:10.2147/JIR.S293993
Gérard, A., Woolfe, A., Mottet, G., Reichen, M., Castrillon, C., Menrath, V., et al. (2020). Author Correction: high-throughput single-cell activity-based screening and sequencing of antibodies using droplet microfluidics. Nat. Biotechnol. 38 (6), 756. doi:10.1038/s41587-020-0563-7
Ghita, L., Yao, Z., Xie, Y., Duran, V., Cagirici, H. B., Samir, J., et al. (2023). Global and cell type-specific immunological hallmarks of severe dengue progression identified via a systems immunology approach. Nat. Immunol. 24 (12), 2150–2163. doi:10.1038/s41590-023-01654-3
Golubnitschaja, O., Polivka, J., Potuznik, P., Pesta, M., Stetkarova, I., Mazurakova, A., et al. (2024). The paradigm change from reactive medical services to 3PM in ischemic stroke: a holistic approach utilising tear fluid multi-omics, mitochondria as a vital biosensor and AI-based multi-professional data interpretation. EPMA J. 15 (1), 1–23. doi:10.1007/s13167-024-00356-6
Gui, F., You, Z., Fu, S., Wu, H., and Zhang, Y. (2020). Endothelial dysfunction in diabetic retinopathy. Front. Endocrinol. (Lausanne) 11, 591. doi:10.3389/fendo.2020.00591
Guo, X., Ning, J., Chen, Y., Liu, G., Zhao, L., Fan, Y., et al. (2024). Recent advances in differential expression analysis for single-cell RNA-seq and spatially resolved transcriptomic studies. Briefings Funct. Genomics 23 (2), 95–109. doi:10.1093/bfgp/elad011
Gurel, Z., and Sheibani, N. (2018). O-Linked β-N-acetylglucosamine (O-GlcNAc) modification: a new pathway to decode pathogenesis of diabetic retinopathy. Clin. Sci. Lond. Engl. 1979 132 (2), 185–198. doi:10.1042/CS20171454
He, H., Suryawanshi, H., Morozov, P., Gay-Mimbrera, J., Del Duca, E., Kim, H. J., et al. (2020). Single-cell transcriptome analysis of human skin identifies novel fibroblast subpopulation and enrichment of immune subsets in atopic dermatitis. J. Allergy Clin. Immunol. 145 (6), 1615–1628. doi:10.1016/j.jaci.2020.01.042
He, X., Wen, S., Tang, X., Wen, Z., Zhang, R., Li, S., et al. (2024). Glucagon-like peptide-1 receptor agonists rescued diabetic vascular endothelial damage through suppression of aberrant STING signaling. Acta Pharm. Sin. B 14 (6), 2613–2630. doi:10.1016/j.apsb.2024.03.011
Herrera-Luis, E., Ortega, V. E., Ampleford, E. J., Sio, Y. Y., Granell, R., de Roos, E., et al. (2022). Multi-ancestry genome-wide association study of asthma exacerbations. Pediatr. Allergy Immunol. Official Publ. Eur. Soc. Pediatr. Allergy Immunol. 33 (6), e13802. doi:10.1111/pai.13802
Heumos, L., Schaar, A. C., Lance, C., Litinetskaya, A., Drost, F., Zappia, L., et al. (2023). Best practices for single-cell analysis across modalities. Nat. Rev. Gene. 24 (8), 550–572. doi:10.1038/s41576-023-00586-w
Hu, Y., Wang, X., Hu, B., Mao, Y., Chen, Y., Yan, L., et al. (2019). Dissecting the transcriptome landscape of the human fetal neural retina and retinal pigment epithelium by single-cell RNA-seq analysis. PLoS Biol. 17 (7), e3000365. doi:10.1371/journal.pbio.3000365
Hu, Z., Mao, X., Chen, M., Wu, X., Zhu, T., Liu, Y., et al. (2022). Single-cell transcriptomics reveals novel role of microglia in fibrovascular membrane of proliferative diabetic retinopathy. Diabetes 71 (4), 762–773. doi:10.2337/db21-0551
Hu, Z., Wang, J., Pan, T., Li, X., Tao, C., Wu, Y., et al. (2023). The exosome-transmitted lncRNA LOC100132249 induces endothelial dysfunction in diabetic retinopathy. Diabetes 72 (9), 1307–1319. doi:10.2337/db22-0435
Huang, L., Li, R., Ye, L., Zhang, S., Tian, H., Du, M., et al. (2023). Deep Sc-RNA sequencing decoding the molecular dynamic architecture of the human retina. Sci. China. Life Sci. 66 (3), 496–515. doi:10.1007/s11427-021-2163-1
Hussain, Z., Rahim, M. A., Jan, N., Shah, H., Rawas-Qalaji, M., Khan, S., et al. (2021). Cell membrane cloaked nanomedicines for bio-imaging and immunotherapy of cancer: improved pharmacokinetics, cell internalization and anticancer efficacy. J. Control. Release Official J. Control. Release Soc. 335, 130–157. doi:10.1016/j.jconrel.2021.05.018
Ising, M., Maccarrone, G., Brückl, T., Scheuer, S., Hennings, J., Holsboer, F., et al. (2019). FKBP5 gene expression predicts antidepressant treatment outcome in depression. Int. J. Mol. Sci. 20 (3), 485. doi:10.3390/ijms20030485
Jagadeesh, K. A., Dey, K. K., Montoro, D. T., Mohan, R., Gazal, S., Engreitz, J. M., et al. (2022). Identifying disease-critical cell types and cellular processes by integrating single-cell RNA-sequencing and human genetics. Nat. Genet. 54 (10), 1479–1492. doi:10.1038/s41588-022-01187-9
Jin, Y., Yong, S., Ke, S., Zhang, C., Liu, Y., Wang, J., et al. (2024). Deep learning assisted fluid volume calculation for assessing anti-vascular endothelial growth factor effect in diabetic macular edema. Heliyon 10 (8), e29775. doi:10.1016/j.heliyon.2024.e29775
Jovic, D., Liang, X., Zeng, H., Lin, L., Xu, F., and Luo, Y. (2022). Single-cell RNA sequencing technologies and applications: a brief overview. Clin. Transl. Med. 12 (3), e694. doi:10.1002/ctm2.694
Jumeau, R., Nguyen-Tan, P. F., Bahig, H., Liem, X., Lambert, L., Schmittbuhl, M., et al. (2018). Pre-irradiation dental care: ready-to-use templates for oropharyngeal cancers. Rep. Pract. Oncol. Radiotherapy J. Gt. Cancer Cent. Poznan Pol. Soc. Radiat. Oncol. 23 (4), 270–275. doi:10.1016/j.rpor.2018.06.007
Kakihara, S., Matsuda, Y., Hirabayashi, K., Imai, A., Iesato, Y., Sakurai, T., et al. (2023). Role of adrenomedullin 2/intermedin in the pathogenesis of neovascular age-related macular degeneration. Laboratory Investigation; a J. Tech. Methods Pathology 103 (4), 100038. doi:10.1016/j.labinv.2022.100038
Kang, Q., and Yang, C. (2020). Oxidative stress and diabetic retinopathy: molecular mechanisms, pathogenetic role and therapeutic implications. Redox Biol. 37, 101799. doi:10.1016/j.redox.2020.101799
Karyotaki, E., Efthimiou, O., Miguel, C., Bermpohl, F. M. G., Furukawa, T. A., Cuijpers, P., et al. (2021). Internet-based cognitive behavioral therapy for depression: a systematic review and individual patient data network meta-analysis. JAMA Psychiatry 78 (4), 361–371. doi:10.1001/jamapsychiatry.2020.4364
Khan, N., Paterson, A. D., Roshandel, D., Raza, A., Ajmal, M., Waheed, N. K., et al. (2020). Association of IGF1 and VEGFA polymorphisms with diabetic retinopathy in Pakistani population. Acta Diabetol. 57 (2), 237–245. doi:10.1007/s00592-019-01407-5
Kim, U. S., Mahroo, O. A., Mollon, J. D., and Yu-Wai-Man, P. (2021). Retinal ganglion cells-diversity of cell types and clinical relevance. Front. Neurology 12, 661938. doi:10.3389/fneur.2021.661938
Kinuthia, U. M., Wolf, A., and Langmann, T. (2020). Microglia and inflammatory responses in diabetic retinopathy. Front. Immunol. 11, 564077. doi:10.3389/fimmu.2020.564077
Kleino, I., Frolovaitė, P., Suomi, T., and Elo, L. L. (2022). Computational solutions for spatial transcriptomics. Comput. Struct. Biotechnol. J. 20, 4870–4884. doi:10.1016/j.csbj.2022.08.043
Ko, J., and Moon, S. J. (2023). Risk of diabetic retinopathy between sodium-glucose cotransporter-2 inhibitors and glucagon-like peptide-1 receptor agonists. Diabetes Metab. J. 47, 394–404. doi:10.4093/dmj.2023.0164
Koh, A., and Bäckhed, F. (2020). From association to causality: the role of the gut microbiota and its functional products on host metabolism. Mol. Cell 78 (4), 584–596. doi:10.1016/j.molcel.2020.03.005
Laboissonniere, L. A., Goetz, J. J., Martin, G. M., Bi, R., Lund, T. J. S., Ellson, L., et al. (2019). Molecular signatures of retinal ganglion cells revealed through single cell profiling. Sci. Rep. 9 (1), 15778. doi:10.1038/s41598-019-52215-4
Lancaster, M. A., Renner, M., Martin, C.-A., Wenzel, D., Bicknell, L. S., Hurles, M. E., et al. (2013). Cerebral organoids model human brain development and microcephaly. Nature 501 (7467), 373–379. doi:10.1038/nature12517
Lee, M.-W., Lee, W.-H., Ryu, C.-K., Lee, Y.-M., Lee, Y.-H., and Kim, J.-Y. (2021). Peripapillary retinal nerve fiber layer and microvasculature in prolonged type 2 diabetes patients without clinical diabetic retinopathy. Investigative Ophthalmol. & Vis. Sci. 62 (2), 9. doi:10.1167/iovs.62.2.9
Lei, Y., Tang, R., Xu, J., Wang, W., Zhang, B., Liu, J., et al. (2021). Applications of single-cell sequencing in cancer research: progress and perspectives. J. Hematol. Oncol. 14 (1), 91. doi:10.1186/s13045-021-01105-2
Li, L., Bowling, S., McGeary, S. E., Yu, Q., Lemke, B., Alcedo, K., et al. (2023). A mouse model with high clonal barcode diversity for joint lineage, transcriptomic, and epigenomic profiling in single cells. Cell 186 (23), 5183–5199.e22. doi:10.1016/j.cell.2023.09.019
Li, X., Yu, Z.-W., Li, H.-Y., Yuan, Y., Gao, X.-Y., and Kuang, H.-Y. (2021). Retinal microglia polarization in diabetic retinopathy. Vis. Neurosci. 38, E006. doi:10.1017/S0952523821000031
Liao, D., Fan, W., Li, N., Li, R., Wang, X., Liu, J., et al. (2024). A single cell atlas of circulating immune cells involved in diabetic retinopathy. IScience 27 (2), 109003. doi:10.1016/j.isci.2024.109003
Lin, P., Gan, Y.-B., He, J., Lin, S.-E., Xu, J.-K., Chang, L., et al. (2024). Advancing skeletal health and disease research with single-cell RNA sequencing. Mil. Med. Res. 11 (1), 33. doi:10.1186/s40779-024-00538-3
Lin, T.-Y., Kang, E. Y.-C., Shao, S.-C., Lai, E. C.-C., Garg, S. J., Chen, K.-J., et al. (2023). Risk of diabetic retinopathy between sodium-glucose cotransporter-2 inhibitors and glucagon-like peptide-1 receptor agonists. Diabetes & Metabolism J. 47 (3), 394–404. doi:10.4093/dmj.2022.0221
Liu, H., Stepicheva, N. A., Ghosh, S., Shang, P., Chowdhury, O., Daley, R. A., et al. (2022a). Reducing Akt2 in retinal pigment epithelial cells causes a compensatory increase in Akt1 and attenuates diabetic retinopathy. Nat. Commun. 13 (1), 6045. doi:10.1038/s41467-022-33773-0
Liu, J., Liao, X., Li, N., Xu, Z., Yang, W., Zhou, H., et al. (2024). MedComm 5 (4), e534. [Not Available]. doi:10.1002/mco2.534
Liu, X., Luo, T., Fan, Z., Li, J., Zhang, Y., Lu, G., et al. (2023). Single cell RNA-seq resolution revealed CCR1+/SELL+/XAF+ CD14 monocytes mediated vascular endothelial cell injuries in Kawasaki disease and COVID-19. Biochimica Biophysica Acta. Mol. Basis Dis. 1869 (5), 166707. doi:10.1016/j.bbadis.2023.166707
Liu, Z., Shi, H., Xu, J., Yang, Q., Ma, Q., Mao, X., et al. (2022b). Single-cell transcriptome analyses reveal microglia types associated with proliferative retinopathy. JCI Insight 7 (23), e160940. doi:10.1172/jci.insight.160940
Li W, W., Lou, X., Zha, Y., Qin, Y., Zha, J., Hong, L., et al. (2023). Single-cell RNA-seq of heart reveals intercellular communication drivers of myocardial fibrosis in diabetic cardiomyopathy. ELife 12, e80479. doi:10.7554/eLife.80479
Llorian-Salvador, M., Cabeza-Fernandez, S., Gomez-Sanchez, J. A., and de la Fuente, A. G. (2024). Glial cell alterations in diabetes-induced neurodegeneration. Cell Mol. Life Sci. 81 (1), 47. doi:10.1007/s00018-023-05024-y
Lou, B., Zeng, L., Gao, X., Qian, X., Li, J. J., Gu, X., et al. (2022). A single-cell transcriptomic atlas of the human ciliary body. Cell. Mol. Life Sci. CMLS 79 (10), 528. doi:10.1007/s00018-022-04559-w
Lukowski, S. W., Lo, C. Y., Sharov, A. A., Nguyen, Q., Fang, L., Hung, S. S., et al. (2019). A single-cell transcriptome atlas of the adult human retina. EMBO J. 38 (18), e100811. doi:10.15252/embj.2018100811
Luo, J., Deng, M., Zhang, X., and Sun, X. (2023). ESICCC as a systematic computational framework for evaluation, selection, and integration of cell-cell communication inference methods. Genome Res. 33 (10), 1788–1805. doi:10.1101/gr.278001.123
Lv, K., Ying, H., Hu, G., Hu, J., Jian, Q., and Zhang, F. (2022). Integrated multi-omics reveals the activated retinal microglia with intracellular metabolic reprogramming contributes to inflammation in STZ-induced early diabetic retinopathy. Front. Immunol. 13, 942768. doi:10.3389/fimmu.2022.942768
Lv Z, Z., Han, J., Li, J., Guo, H., Fei, Y., Sun, Z., et al. (2022). Single cell RNA-seq analysis identifies ferroptotic chondrocyte cluster and reveals TRPV1 as an anti-ferroptotic target in osteoarthritis. EBioMedicine 84, 104258. doi:10.1016/j.ebiom.2022.104258
Ma, P., Amemiya, H. M., He, L. L., Gandhi, S. J., Nicol, R., Bhattacharyya, R. P., et al. (2023). Bacterial droplet-based single-cell RNA-seq reveals antibiotic-associated heterogeneous cellular states. Cell 186 (4), 877–891.e14. doi:10.1016/j.cell.2023.01.002
Manochkumar, J., Cherukuri, A. K., Kumar, R. S., Almansour, A. I., Ramamoorthy, S., and Efferth, T. (2023). A critical review of machine-learning for “multi-omics” marine metabolite datasets. Comput. Biol. Med. 165, 107425. doi:10.1016/j.compbiomed.2023.107425
Mao, P., Shen, Y., Mao, X., Liu, K., and Zhong, J. (2023). The single-cell landscape of alternative transcription start sites of diabetic retina. Exp. Eye Res. 233, 109520. doi:10.1016/j.exer.2023.109520
Mao, S., Wang, Y., Chao, N., Zeng, L., and Zhang, L. (2024). Integrated analysis of single-cell RNA-seq and bulk RNA-seq reveals immune suppression subtypes and establishes a novel signature for determining the prognosis in lung adenocarcinoma. Cell. Oncol. Dordr. 47, 1697–1713. doi:10.1007/s13402-024-00948-4
Marneros, A. G. (2023). Role of inflammasome activation in neovascular age-related macular degeneration. FEBS J. 290 (1), 28–36. doi:10.1111/febs.16278
Matosin, N., Arloth, J., Czamara, D., Edmond, K. Z., Maitra, M., Fröhlich, A. S., et al. (2023). Associations of psychiatric disease and ageing with FKBP5 expression converge on superficial layer neurons of the neocortex. Acta Neuropathol. 145 (4), 439–459. doi:10.1007/s00401-023-02541-9
McIntosh, T., Walsh, H., Baldwin, K., Iltis, A., Mohan, S., Sawinski, D., et al. (2024). Evaluating ApoL1 genetic testing policy options for transplant centers: a delphi consensus panel project with stakeholders. Clin. J. Am. Soc. Nephrol. CJASN 19 (4), 494–502. doi:10.2215/CJN.0000000000000397
McNulty, R., Sritharan, D., Pahng, S. H., Meisch, J. P., Liu, S., Brennan, M. A., et al. (2023). Probe-based bacterial single-cell RNA sequencing predicts toxin regulation. Nat. Microbiol. 8 (5), 934–945. doi:10.1038/s41564-023-01348-4
Md Pauzi, S. H., Masir, N., Yahaya, A., Mohammed, F., Tizen Laim, N. M. S., Mustangin, M., et al. (2021). HER2 testing by immunohistochemistry in breast cancer: a multicenter proficiency ring study. Indian J. Pathology & Microbiol. 64 (4), 677–682. doi:10.4103/IJPM.IJPM_983_20
Motloch, K., Soler, V., Delyfer, M.-N., Vasseur, V., Wolff, B., Issa, M., et al. (2024). Efficacy and safety of 0.19-mg fluocinolone acetonide implant in postoperative cystoid macular edema after pars plana vitrectomy: the ILUvien in Postoperative CYstoid macular eDema study. Ophthalmol. Retina. doi:10.1016/j.oret.2024.07.004
Mullin, N. K., Voigt, A. P., Flamme-Wiese, M. J., Liu, X., Riker, M. J., Varzavand, K., et al. (2023). Multimodal single-cell analysis of nonrandom heteroplasmy distribution in human retinal mitochondrial disease. JCI Insight 8 (14), e165937. doi:10.1172/jci.insight.165937
Netea, M. G., Domínguez-Andrés, J., Barreiro, L. B., Chavakis, T., Divangahi, M., Fuchs, E., et al. (2020). Defining trained immunity and its role in health and disease. Nat. Rev. Immunol. 20 (6), 375–388. doi:10.1038/s41577-020-0285-6
Nishikawa, H., and Koyama, S. (2021). Mechanisms of regulatory T cell infiltration in tumors: implications for innovative immune precision therapies. J. For Immunother. Cancer 9 (7), e002591. doi:10.1136/jitc-2021-002591
Niu, T., Fang, J., Shi, X., Zhao, M., Xing, X., Wang, Y., et al. (2021). Pathogenesis study based on high-throughput single-cell sequencing analysis reveals novel transcriptional landscape and heterogeneity of retinal cells in type 2 diabetic mice. Diabetes 70 (5), 1185–1197. doi:10.2337/db20-0839
Nouri, H., Abtahi, S.-H., Mazloumi, M., Samadikhadem, S., Arevalo, J. F., and Ahmadieh, H. (2024). Optical coherence tomography angiography in diabetic retinopathy: a major review. Surv. Ophthalmol. 69 (4), 558–574. doi:10.1016/j.survophthal.2024.03.004
Paik, D. T., Cho, S., Tian, L., Chang, H. Y., and Wu, J. C. (2020). Single-cell RNA sequencing in cardiovascular development, disease and medicine. Nat. Rev. Cardiol. 17 (8), 457–473. doi:10.1038/s41569-020-0359-y
Parikh, B. H., Blakeley, P., Regha, K., Liu, Z., Yang, B., Bhargava, M., et al. (2023). Single-cell transcriptomics reveals maturation of transplanted stem cell-derived retinal pigment epithelial cells toward native state. Proc. Natl. Acad. Sci. U. S. A. 120 (26), e2214842120. doi:10.1073/pnas.2214842120
Pei, W., Shang, F., Wang, X., Fanti, A.-K., Greco, A., Busch, K., et al. (2020). Resolving fates and single-cell transcriptomes of hematopoietic stem cell clones by PolyloxExpress barcoding. Cell Stem Cell 27 (3), 383–395. doi:10.1016/j.stem.2020.07.018
Pelka, K., Hofree, M., Chen, J. H., Sarkizova, S., Pirl, J. D., Jorgji, V., et al. (2021). Spatially organized multicellular immune hubs in human colorectal cancer. Cell 184 (18), 4734–4752.e20. doi:10.1016/j.cell.2021.08.003
Peng, G., Cui, G., Ke, J., and Jing, N. (2020). Using single-cell and spatial transcriptomes to understand stem cell lineage specification during early embryo development. Annu. Rev. Genomics Hum. Genet. 21, 163–181. doi:10.1146/annurev-genom-120219-083220
Peng, H., Li, H., Ma, B., Sun, X., and Chen, B. (2024). DJ-1 regulates mitochondrial function and promotes retinal ganglion cell survival under high glucose-induced oxidative stress. Front. Pharmacol. 15, 1455439. doi:10.3389/fphar.2024.1455439
Peng, W., Gutierrez Reyes, C. D., Gautam, S., Yu, A., Cho, B. G., Goli, M., et al. (2023). MS-based glycomics and glycoproteomics methods enabling isomeric characterization. Mass Spectrom. Rev. 42 (2), 577–616. doi:10.1002/mas.21713
Perais, J., Agarwal, R., Evans, J. R., Loveman, E., Colquitt, J. L., Owens, D., et al. (2023). Prognostic factors for the development and progression of proliferative diabetic retinopathy in people with diabetic retinopathy. Cochrane Database Syst. Rev. 2 (2), CD013775. doi:10.1002/14651858.CD013775.pub2
Pfeifer, C. W., Walsh, J. T., Santeford, A., Lin, J. B., Beatty, W. L., Terao, R., et al. (2023). Dysregulated CD200-CD200R signaling in early diabetes modulates microglia-mediated retinopathy. Proc. Natl. Acad. Sci. U. S. A. 120 (45), e2308214120. doi:10.1073/pnas.2308214120
Plumbly, W., Brandon, N., Deeb, T. Z., Hall, J., and Harwood, A. J. (2019). L-type voltage-gated calcium channel regulation of in vitro human cortical neuronal networks. Sci. Rep. 9 (1), 13810. doi:10.1038/s41598-019-50226-9
Pratama, S., Lauren, B. C., and Wisnu, W. (2022). The efficacy of vitamin B12 supplementation for treating vitamin B12 deficiency and peripheral neuropathy in metformin-treated type 2 diabetes mellitus patients: a systematic review. Diabetes & Metabolic Syndrome 16 (10), 102634. doi:10.1016/j.dsx.2022.102634
Pun, M., Pratt, D., Nano, P. R., Joshi, P. K., Jiang, L., Englinger, B., et al. (2023). Common molecular features of H3K27M DMGs and PFA ependymomas map to hindbrain developmental pathways. Acta Neuropathol. Commun. 11 (1), 25. doi:10.1186/s40478-023-01514-z
Qin, G. B., Wu, Y. H., Chen, H. S., Huang, Y. T., Yi, J. F., and Xiao, Y. (2023). Correlation analysis between morphologic characteristics of the thoracolumbar basivertebral foramen and Kummell's disease in patients with osteoporosis using imaging techniques. BMC Musculoskelet. Disord. 24 (1), 513. doi:10.1186/s12891-023-06609-1
Quadrato, G., Nguyen, T., Macosko, E. Z., Sherwood, J. L., Min Yang, S., Berger, D. R., et al. (2017). Cell diversity and network dynamics in photosensitive human brain organoids. Nature 545 (7652), 48–53. doi:10.1038/nature22047
Raj, B., Gagnon, J. A., and Schier, A. F. (2018a). Large-scale reconstruction of cell lineages using single-cell readout of transcriptomes and CRISPR-Cas9 barcodes by scGESTALT. Nat. Protoc. 13 (11), 2685–2713. doi:10.1038/s41596-018-0058-x
Raj, B., Wagner, D. E., McKenna, A., Pandey, S., Klein, A. M., Shendure, J., et al. (2018b). Simultaneous single-cell profiling of lineages and cell types in the vertebrate brain. Nat. Biotechnol. 36 (5), 442–450. doi:10.1038/nbt.4103
Rangasamy, S., Monickaraj, F., Legendre, C., Cabrera, A. P., Llaci, L., Bilagody, C., et al. (2020). Transcriptomics analysis of pericytes from retinas of diabetic animals reveals novel genes and molecular pathways relevant to blood-retinal barrier alterations in diabetic retinopathy. Exp. Eye Res. 195, 108043. doi:10.1016/j.exer.2020.108043
Rauscher, F. G., Elze, T., Francke, M., Martinez-Perez, M. E., Li, Y., Wirkner, K., et al. (2024). Glucose tolerance and insulin resistance/sensitivity associate with retinal layer characteristics: the LIFE-Adult-Study. Diabetologia 67 (5), 928–939. doi:10.1007/s00125-024-06093-9
Ren, H., Zhang, Y., Zhong, M., Hussian, J., Tang, Y., Liu, S., et al. (2023). Calcium signaling-mediated transcriptional reprogramming during abiotic stress response in plants. TAG. Theor. Appl. Genet. Theor. Und Angewandte Genet. 136 (10), 210. doi:10.1007/s00122-023-04455-2
Ren, L., Xia, J., Huang, C., Bai, Y., Yao, J., Li, D., et al. (2024). Single-cell transcriptomic analysis reveals the antiangiogenic role of Mgarp in diabetic retinopathy. BMJ Open Diabetes Res. & Care 12 (4), e004189. doi:10.1136/bmjdrc-2024-004189
Replogle, J. M., Norman, T. M., Xu, A., Hussmann, J. A., Chen, J., Cogan, J. Z., et al. (2020). Combinatorial single-cell CRISPR screens by direct guide RNA capture and targeted sequencing. Nat. Biotechnol. 38 (8), 954–961. doi:10.1038/s41587-020-0470-y
Rohlenova, K., Goveia, J., García-Caballero, M., Subramanian, A., Kalucka, J., Treps, L., et al. (2020). Single-cell RNA sequencing maps endothelial metabolic plasticity in pathological angiogenesis. Cell Metab. 31 (4), 862–877. doi:10.1016/j.cmet.2020.03.009
Saddala, M. S., Mundla, S., Patyal, N., and Dash, S. (2023). Single-cell RNA sequencing (scRNA-Seq) data analysis of retinal homeostasis and degeneration of microglia. Methods Mol. Biol. 2678, 91–106. doi:10.1007/978-1-0716-3255-0_6
Saint-Antoine, M. M., and Singh, A. (2020). Network inference in systems biology: recent developments, challenges, and applications. Curr. Opin. Biotechnol. 63, 89–98. doi:10.1016/j.copbio.2019.12.002
Samanta, P., Cooke, S. F., McNulty, R., Hormoz, S., and Rosenthal, A. (2024). ProBac-seq, a bacterial single-cell RNA sequencing methodology using droplet microfluidics and large oligonucleotide probe sets. Nat. Protoc. 19, 2939–2966. doi:10.1038/s41596-024-01002-1
Shekhar, K., Lapan, S. W., Whitney, I. E., Tran, N. M., Macosko, E. Z., Kowalczyk, M., et al. (2016). Comprehensive classification of retinal bipolar neurons by single-cell transcriptomics. Cell 166 (5), 1308–1323. doi:10.1016/j.cell.2016.07.054
Shi, S., Ding, C., Zhu, S., Xia, F., Buscho, S. E., Li, S., et al. (2023). PERK inhibition suppresses neovascularization and protects neurons during ischemia-induced retinopathy. Investigative Ophthalmol. & Vis. Sci. 64 (11), 17. doi:10.1167/iovs.64.11.17
Singer, M. A., Sheth, V., Mansour, S. E., Coughlin, B., and Gonzalez, V. H. (2022). Three-year safety and efficacy of the 0.19-mg fluocinolone acetonide intravitreal implant for diabetic macular edema: the PALADIN study. Ophthalmology 129 (6), 605–613. doi:10.1016/j.ophtha.2022.01.015
Skol, A. D., Jung, S. C., Sokovic, A. M., Chen, S., Fazal, S., Sosina, O., et al. (2020). Integration of genomics and transcriptomics predicts diabetic retinopathy susceptibility genes. ELife 9, e59980. doi:10.7554/eLife.59980
Soni, D., Sagar, P., and Takkar, B. (2021). Diabetic retinal neurodegeneration as a form of diabetic retinopathy. Int. Ophthalmol. 41 (9), 3223–3248. doi:10.1007/s10792-021-01864-4
Sørensen, C. B., Adams, T. B., Pedersen, E. R., Nielsen, J., and Schmidt, J. H. (2023). AMTASTM and user-operated smartphone research application audiometry-An evaluation study. PLoS One 18 (9), e0291412. doi:10.1371/journal.pone.0291412
Spencer, B. G., Estevez, J. J., Liu, E., Craig, J. E., and Finnie, J. W. (2020). Pericytes, inflammation, and diabetic retinopathy. Inflammopharmacology 28 (3), 697–709. doi:10.1007/s10787-019-00647-9
Starr, C. R., Zhylkibayev, A., Mobley, J. A., and Gorbatyuk, M. S. (2023). Proteomic analysis of diabetic retinas. Front. Endocrinol. 14, 1229089. doi:10.3389/fendo.2023.1229089
Stuart, T., Butler, A., Hoffman, P., Hafemeister, C., Papalexi, E., Mauck, W. M., et al. (2019). Comprehensive integration of single-cell data. Cell 177 (7), 1888–1902. doi:10.1016/j.cell.2019.05.031
Sun, F., Li, H., Sun, D., Fu, S., Gu, L., Shao, X., et al. (2024). Single-cell omics: experimental workflow, data analyses and applications. Sci. China. Life Sci. doi:10.1007/s11427-023-2561-0
Sun, L., Wang, R., Hu, G., Liu, H., Lv, K., Duan, Y., et al. (2021). Single cell RNA sequencing (scRNA-Seq) deciphering pathological alterations in streptozotocin-induced diabetic retinas. Exp. Eye Res. 210, 108718. doi:10.1016/j.exer.2021.108718
Tan, A., Li, T., Ruan, L., Yang, J., Luo, Y., Li, L., et al. (2021). Knockdown of Malat1 alleviates high-glucose-induced angiogenesis through regulating miR-205-5p/VEGF-A axis. Exp. Eye Res. 207, 108585. doi:10.1016/j.exer.2021.108585
Tan, T.-E., and Wong, T. Y. (2022). Diabetic retinopathy: looking forward to 2030. Front. Endocrinol. 13, 1077669. doi:10.3389/fendo.2022.1077669
Tang, L., Xu, G.-T., and Zhang, J.-F. (2023). Inflammation in diabetic retinopathy: possible roles in pathogenesis and potential implications for therapy. Neural Regen. Res. 18 (5), 976–982. doi:10.4103/1673-5374.355743
Tan Y, Y., Huang, J., Li, D., Zou, C., Liu, D., and Qin, B. (2023). Single-cell RNA sequencing in dissecting microenvironment of age-related macular degeneration: challenges and perspectives. Ageing Res. Rev. 90, 102030. doi:10.1016/j.arr.2023.102030
Tan Z, Z., Chen, X., Zuo, J., Fu, S., Wang, J., and Wang, H. (2023). Integrating bulk and single-cell RNA sequencing reveals heterogeneity, tumor microenvironment, and immunotherapeutic efficacy based on sialylation-related genes in bladder cancer. J. Inflamm. Res. 16, 3399–3417. doi:10.2147/JIR.S418433
Temple, S. (2023). Advancing cell therapy for neurodegenerative diseases. Cell Stem Cell 30 (5), 512–529. doi:10.1016/j.stem.2023.03.017
Tolentino, M. J., Tolentino, A. J., Tolentino, E. M., Krishnan, A., and Genead, M. A. (2023). Sialic acid mimetic microglial sialic acid-binding immunoglobulin-like lectin agonism: potential to restore retinal homeostasis and regain visual function in age-related macular degeneration. Pharm. Basel, Switz. 16 (12), 1735. doi:10.3390/ph16121735
Tresenrider, A., Sridhar, A., Eldred, K. C., Cuschieri, S., Hoffer, D., Trapnell, C., et al. (2023). Single-cell sequencing of individual retinal organoids reveals determinants of cell-fate heterogeneity. Cell Rep. Methods 3 (8), 100548. doi:10.1016/j.crmeth.2023.100548
Uemura, A., Fruttiger, M., D'Amore, P. A., De Falco, S., Joussen, A. M., Sennlaub, F., et al. (2021). VEGFR1 signaling in retinal angiogenesis and microinflammation. Prog. Retin Eye Res. 84, 100954. doi:10.1016/j.preteyeres.2021.100954
Valecha, M., and Posada, D. (2022). Somatic variant calling from single-cell DNA sequencing data. Comput. Struct. Biotechnol. J. 20, 2978–2985. doi:10.1016/j.csbj.2022.06.013
Van Bergen, T., Etienne, I., Cunningham, F., Moons, L., Schlingemann, R. O., Feyen, J. H. M., et al. (2019). The role of placental growth factor (PlGF) and its receptor system in retinal vascular diseases. Prog. Retin. Eye Res. 69, 116–136. doi:10.1016/j.preteyeres.2018.10.006
Van de Sande, B., Lee, J. S., Mutasa-Gottgens, E., Naughton, B., Bacon, W., Manning, J., et al. (2023). Applications of single-cell RNA sequencing in drug discovery and development. Nat. Rev. Drug Discov. 22 (6), 496–520. doi:10.1038/s41573-023-00688-4
VanHorn, S., and Morris, S. A. (2021). Next-generation lineage tracing and fate mapping to interrogate development. Dev. Cell 56 (1), 7–21. doi:10.1016/j.devcel.2020.10.021
Van Hove, I., De Groef, L., Boeckx, B., Modave, E., Hu, T.-T., Beets, K., et al. (2020). Single-cell transcriptome analysis of the Akimba mouse retina reveals cell-type-specific insights into the pathobiology of diabetic retinopathy. Diabetologia 63 (10), 2235–2248. doi:10.1007/s00125-020-05218-0
Varughese, G. I., and Jacob, S. (2023). Sodium-glucose co-transporter 2 inhibitors and glucagon-like peptide 1 receptor agonists treatment: variable observations of sequelae on diabetic retinopathy. J. R. Soc. Med. 116 (12), 408. doi:10.1177/01410768231215999
Voight, B. F., Scott, L. J., Steinthorsdottir, V., Morris, A. P., Dina, C., Welch, R. P., et al. (2010). Twelve type 2 diabetes susceptibility loci identified through large-scale association analysis. Nat. Genet. 42 (7), 579–589. doi:10.1038/ng.609
Voigt, A. P., Mullin, N. K., Navratil, E. M., Flamme-Wiese, M. J., Lin, L.-C., Scheetz, T. E., et al. (2023). Gene expression within a human choroidal neovascular membrane using spatial transcriptomics. Investigative Ophthalmol. & Vis. Sci. 64 (13), 40. doi:10.1167/iovs.64.13.40
Voigt, A. P., Mullin, N. K., Stone, E. M., Tucker, B. A., Scheetz, T. E., and Mullins, R. F. (2021a). Single-cell RNA sequencing in vision research: insights into human retinal health and disease. Prog. Retin Eye Res. 83, 100934. doi:10.1016/j.preteyeres.2020.100934
Voigt, A. P., Mullin, N. K., Whitmore, S. S., DeLuca, A. P., Burnight, E. R., Liu, X., et al. (2021b). Human photoreceptor cells from different macular subregions have distinct transcriptional profiles. Hum. Mol. Genet. 30 (16), 1543–1558. doi:10.1093/hmg/ddab140
Voigt, A. P., Whitmore, S. S., Flamme-Wiese, M. J., Riker, M. J., Wiley, L. A., Tucker, B. A., et al. (2019). Molecular characterization of foveal versus peripheral human retina by single-cell RNA sequencing. Exp. Eye Res. 184, 234–242. doi:10.1016/j.exer.2019.05.001
Vu, T. N., Wills, Q. F., Kalari, K. R., Niu, N., Wang, L., Rantalainen, M., et al. (2016). Beta-Poisson model for single-cell RNA-seq data analyses. Bioinforma. Oxf. Engl. 32 (14), 2128–2135. doi:10.1093/bioinformatics/btw202
Wang, D., He, P., Wang, Z., Li, G., Majed, N., and Gu, A. Z. (2020). Advances in single cell Raman spectroscopy technologies for biological and environmental applications. Curr. Opin. Biotechnol. 64, 218–229. doi:10.1016/j.copbio.2020.06.011
Wang, J., Sun, H., Mou, L., Lu, Y., Wu, Z., Pu, Z., et al. (2024). Unveiling the molecular complexity of proliferative diabetic retinopathy through scRNA-seq, AlphaFold 2, and machine learning. Front. Endocrinol. 15, 1382896. doi:10.3389/fendo.2024.1382896
Wang, J.-H., Kumar, S., and Liu, G.-S. (2021). Bulk gene expression deconvolution reveals infiltration of M2 macrophages in retinal neovascularization. Investigative Ophthalmol. & Vis. Sci. 62 (14), 22. doi:10.1167/iovs.62.14.22
Wang, R., Yang, X., Chen, J., Zhang, L., Griffiths, J. A., Cui, G., et al. (2023). Time space and single-cell resolved tissue lineage trajectories and laterality of body plan at gastrulation. Nat. Commun. 14 (1), 5675. doi:10.1038/s41467-023-41482-5
Wang C, C., Yu, Q., Song, T., Wang, Z., Song, L., Yang, Y., et al. (2022). The heterogeneous immune landscape between lung adenocarcinoma and squamous carcinoma revealed by single-cell RNA sequencing. Signal Transduct. Target. Ther. 7 (1), 289. doi:10.1038/s41392-022-01130-8
Wang N, N., Wei, L., Liu, D., Zhang, Q., Xia, X., Ding, L., et al. (2022). Identification and validation of autophagy-related genes in diabetic retinopathy. Front. Endocrinol. 13, 867600. doi:10.3389/fendo.2022.867600
Wang Y, Y., Yang, X., Li, Q., Zhang, Y., Chen, L., Hong, L., et al. (2022). Single-cell RNA sequencing reveals the Müller subtypes and inner blood-retinal barrier regulatory network in early diabetic retinopathy. Front. Mol. Neurosci. 15, 1048634. doi:10.3389/fnmol.2022.1048634
Wang Y, Y., Yang, X., Zhang, Y., Hong, L., Xie, Z., Jiang, W., et al. (2024). Single-cell RNA sequencing reveals roles of unique retinal microglia types in early diabetic retinopathy. Diabetology & Metabolic Syndrome 16 (1), 49. doi:10.1186/s13098-024-01282-3
Weber, L. L., Sashittal, P., and El-Kebir, M. (2021). doubletD: detecting doublets in single-cell DNA sequencing data. Bioinforma. Oxf. Engl. 37 (Suppl. l_1), i214–i221. doi:10.1093/bioinformatics/btab266
Wimmers, F., Donato, M., Kuo, A., Ashuach, T., Gupta, S., Li, C., et al. (2021). The single-cell epigenomic and transcriptional landscape of immunity to influenza vaccination. Cell 184 (15), 3915–3935.e21. doi:10.1016/j.cell.2021.05.039
Wolf, J., Rasmussen, D. K., Sun, Y. J., Vu, J. T., Wang, E., Espinosa, C., et al. (2023). Liquid-biopsy proteomics combined with AI identifies cellular drivers of eye aging and disease in vivo. Cell 186 (22), 4868–4884.e12. doi:10.1016/j.cell.2023.09.012
Wu, H., Wang, M., Li, X., and Shao, Y. (2021). The metaflammatory and immunometabolic role of macrophages and microglia in diabetic retinopathy. Hum. Cell 34 (6), 1617–1628. doi:10.1007/s13577-021-00580-6
Wurl, J. A., Mac Nair, C. E., Dietz, J. A., Shestopalov, V. I., and Nickells, R. W. (2023). Contralateral astrocyte response to acute optic nerve damage is mitigated by PANX1 channel activity. Int. J. Mol. Sci. 24 (21), 15641. doi:10.3390/ijms242115641
Xia, M., Jiao, L., Wang, X.-H., Tong, M., Yao, M.-D., Li, X.-M., et al. (2023). Single-cell RNA sequencing reveals a unique pericyte type associated with capillary dysfunction. Theranostics 13 (8), 2515–2530. doi:10.7150/thno.83532
Xia, Y., Luo, Q., Chen, J., Huang, C., Jahangir, A., Pan, T., et al. (2022). Retinal astrocytes and microglia activation in diabetic retinopathy rhesus monkey models. Curr. Eye Res. 47 (2), 297–303. doi:10.1080/02713683.2021.1984535
Xiao, Y., Hu, X., Fan, S., Zhong, J., Mo, X., Liu, X., et al. (2021). Single-cell transcriptome profiling reveals the suppressive role of retinal neurons in microglia activation under diabetes mellitus. Front. Cell Dev. Biol. 9, 680947. doi:10.3389/fcell.2021.680947
Xiong, X., Kuang, H., Ansari, S., Liu, T., Gong, J., Wang, S., et al. (2019). Landscape of intercellular crosstalk in healthy and NASH liver revealed by single-cell secretome gene analysis. Mol. Cell 75 (3), 644–660. doi:10.1016/j.molcel.2019.07.028
Xu, C., Liu, G., Liu, X., and Wang, F. (2014). O-GlcNAcylation under hypoxic conditions and its effects on the blood-retinal barrier in diabetic retinopathy. Int. J. Mol. Med. 33 (3), 624–632. doi:10.3892/ijmm.2013.1597
Xu, L., Chang, C., Jiang, P., Wei, K., Zhang, R., Jin, Y., et al. (2022). Metabolomics in rheumatoid arthritis: advances and review. Front. Immunol. 13, 961708. doi:10.3389/fimmu.2022.961708
Xu, X., Crow, M., Rice, B. R., Li, F., Harris, B., Liu, L., et al. (2021). Single-cell RNA sequencing of developing maize ears facilitates functional analysis and trait candidate gene discovery. Dev. Cell 56 (4), 557–568.e6. doi:10.1016/j.devcel.2020.12.015
Xu, X., Zhang, C., Tang, G., Wang, N., and Feng, Y. (2023). Single-cell transcriptome profiling highlights the role of APP in blood vessels in assessing the risk of patients with proliferative diabetic retinopathy developing Alzheimer's disease. Front. Cell Dev. Biol. 11, 1328979. doi:10.3389/fcell.2023.1328979
Xu, Y., Huang, S., Li, Z., Dai, L., Wu, H., Wang, P., et al. (2023a). Single-cell RNA landscape of osteoimmune microenvironment in osteoporotic vertebral compression fracture and Kümmell's disease. Front. Cell Dev. Biol. 11, 1276098. doi:10.3389/fcell.2023.1276098
Xu, Y., Xiang, Z., E, W., Lang, Y., Huang, S., Qin, W., et al. (2023b). Single-cell transcriptomes reveal a molecular link between diabetic kidney and retinal lesions. Commun. Biol. 6 (1), 912. doi:10.1038/s42003-023-05300-4
Xu, Y., Zhang, Y., Qin, Y., Gu, M., Chen, R., Sun, Y., et al. (2023c). Multi-omics analysis of functional substances and expression verification in cashmere fineness. BMC Genomics 24 (1), 720. doi:10.1186/s12864-023-09825-0
Yang, C., Ma, Y., Yao, M., Jiang, Q., and Xue, J. (2024). Causal relationships between blood metabolites and diabetic retinopathy: a two-sample Mendelian randomization study. Front. Endocrinol. 15, 1383035. doi:10.3389/fendo.2024.1383035
Yang, J., and Liu, Z. (2022). Mechanistic pathogenesis of endothelial dysfunction in diabetic nephropathy and retinopathy. Front. Endocrinol. 13, 816400. doi:10.3389/fendo.2022.816400
Yang, Y., Sun, L., Chen, Z., Liu, W., Xu, Q., Liu, F., et al. (2023). The immune-metabolic crosstalk between CD3+C1q+TAM and CD8+T cells associated with relapse-free survival in HCC. Front. Immunol. 14, 1033497. doi:10.3389/fimmu.2023.1033497
Yang Z, Z., Liu, Y., Chen, X., Huang, S., Li, Y., Ye, G., et al. (2023). Empagliflozin targets Mfn1 and Opa1 to attenuate microglia-mediated neuroinflammation in retinal ischemia and reperfusion injury. J. Neuroinflammation 20 (1), 296. doi:10.1186/s12974-023-02982-9
Yao, M., Ren, T., Pan, Y., Xue, X., Li, R., Zhang, L., et al. (2022). A new generation of lineage tracing dynamically records cell fate choices. Int. J. Mol. Sci. 23 (9), 5021. doi:10.3390/ijms23095021
Yao, X., Zhao, Z., Zhang, W., Liu, R., Ni, T., Cui, B., et al. (2024). Specialized retinal endothelial cells modulate blood-retina barrier in diabetic retinopathy. Diabetes 73 (2), 225–236. doi:10.2337/db23-0368
Yao, Y., Chen, Z., Wu, Q., Lu, Y., Zhou, X., and Zhu, X. (2023). Single-cell RNA sequencing of retina revealed novel transcriptional landscape in high myopia and underlying cell-type-specific mechanisms. MedComm 4 (5), e372. doi:10.1002/mco2.372
Ye, C., Chen, Z., Liu, Z., Wang, F., and He, X. (2020). Defining endogenous barcoding sites for CRISPR/Cas9-based cell lineage tracing in zebrafish. J. Genet. Genomics = Yi Chuan Xue Bao 47 (2), 85–91. doi:10.1016/j.jgg.2019.11.012
Yeh, C.-F., Chen, Y.-H., Liu, S.-F., Kao, H.-L., Wu, M.-S., Yang, K.-C., et al. (2020). Mutual interplay of host immune system and gut microbiota in the immunopathology of atherosclerosis. Int. J. Mol. Sci. 21 (22), 8729. doi:10.3390/ijms21228729
Yi, W., Lu, Y., Zhong, S., Zhang, M., Sun, L., Dong, H., et al. (2021). A single-cell transcriptome atlas of the aging human and macaque retina. Natl. Sci. Rev. 8 (4), nwaa179. doi:10.1093/nsr/nwaa179
Yousri, N. A., Suhre, K., Yassin, E., Al-Shakaki, A., Robay, A., Elshafei, M., et al. (2022). Metabolic and metabo-clinical signatures of type 2 diabetes, obesity, retinopathy, and dyslipidemia. Diabetes 71 (2), 184–205. doi:10.2337/db21-0490
Yu, L., Shen, N., Shi, Y., Shi, X., Fu, X., Li, S., et al. (2022). Characterization of cancer-related fibroblasts (CAF) in hepatocellular carcinoma and construction of CAF-based risk signature based on single-cell RNA-seq and bulk RNA-seq data. Front. Immunol. 13, 1009789. doi:10.3389/fimmu.2022.1009789
Yuan, D., Xu, Y., Xue, L., Zhang, W., Gu, L., and Liu, Q. (2024). Resveratrol protects against diabetic retinal ganglion cell damage by activating the Nrf2 signaling pathway. Heliyon 10 (9), e30786. doi:10.1016/j.heliyon.2024.e30786
Zelniker, T. A., Wiviott, S. D., Raz, I., Im, K., Goodrich, E. L., Furtado, R. H. M., et al. (2019). Comparison of the effects of glucagon-like peptide receptor agonists and sodium-glucose cotransporter 2 inhibitors for prevention of major adverse cardiovascular and renal outcomes in type 2 diabetes mellitus. Circulation 139 (17), 2022–2031. doi:10.1161/CIRCULATIONAHA.118.038868
Zhan, L. (2023). Frontiers in understanding the pathological mechanism of diabetic retinopathy. Med. Sci. Monit. Int. Med. J. Exp. Clin. Res. 29, e939658. doi:10.12659/MSM.939658
Zhang, C., and Zhao, L. (2016). Strain-level dissection of the contribution of the gut microbiome to human metabolic disease. Genome Med. 8 (1), 41. doi:10.1186/s13073-016-0304-1
Zhang, J., Liu, R., Kuang, H.-Y., Gao, X.-Y., and Liu, H.-L. (2017). Protective treatments and their target retinal ganglion cells in diabetic retinopathy. Brain Res. Bull. 132, 53–60. doi:10.1016/j.brainresbull.2017.05.007
Zhang, J., Zhang, J., Zhang, C., Zhang, J., Gu, L., Luo, D., et al. (2022). Diabetic macular edema: current understanding, molecular mechanisms and therapeutic implications. Cells 11 (21), 3362. doi:10.3390/cells11213362
Zhang, P., Liu, J., Pei, S., Wu, D., Xie, J., Liu, J., et al. (2023). Mast cell marker gene signature: prognosis and immunotherapy response prediction in lung adenocarcinoma through integrated scRNA-seq and bulk RNA-seq. Front. Immunol. 14, 1189520. doi:10.3389/fimmu.2023.1189520
Zhang, Q., He, Y., Luo, N., Patel, S. J., Han, Y., Gao, R., et al. (2019). Landscape and dynamics of single immune cells in hepatocellular carcinoma. Cell 179 (4), 829–845. doi:10.1016/j.cell.2019.10.003
Zhang, R., Huang, C., Chen, Y., Li, T., and Pang, L. (2022). Single-cell transcriptomic analysis revealing changes in retinal cell subpopulation levels and the pathways involved in diabetic retinopathy. Ann. Transl. Med. 10 (10), 562. doi:10.21037/atm-22-1546
Zhang, X., Zhang, F., and Xu, X. (2024). Single-cell RNA sequencing in exploring the pathogenesis of diabetic retinopathy. Clin. Transl. Med. 14 (7), e1751. doi:10.1002/ctm2.1751
Zhang, Y., Bailey, T. S., Hittmeyer, P., Dubois, L. J., Theys, J., and Lambin, P. (2024). Multiplex genetic manipulations in Clostridium butyricum and Clostridium sporogenes to secrete recombinant antigen proteins for oral-spore vaccination. Microb. Cell Factories 23 (1), 119. doi:10.1186/s12934-024-02389-y
Zhang, Z., Cui, F., Lin, C., Zhao, L., Wang, C., and Zou, Q. (2021). Critical downstream analysis steps for single-cell RNA sequencing data. Briefings Bioinforma. 22 (5), bbab105. doi:10.1093/bib/bbab105
Zhang X, X., Chao, P., Zhang, L., Xu, L., Cui, X., Wang, S., et al. (2023). Single-cell RNA and transcriptome sequencing profiles identify immune-associated key genes in the development of diabetic kidney disease. Front. Immunol. 14, 1030198. doi:10.3389/fimmu.2023.1030198
Zhou, H., Zhang, L., Ding, C., Zhou, Y., and Li, Y. (2024). Upregulation of HMOX1 associated with M2 macrophage infiltration and ferroptosis in proliferative diabetic retinopathy. Int. Immunopharmacol. 134, 112231. doi:10.1016/j.intimp.2024.112231
Zhu, H., Chen, J., Liu, K., Gao, L., Wu, H., Ma, L., et al. (2023). Human PBMC scRNA-seq-based aging clocks reveal ribosome to inflammation balance as a single-cell aging hallmark and super longevity. Sci. Adv. 9 (26), eabq7599. doi:10.1126/sciadv.abq7599
Zhu, L., Zhang, D., Zhu, H., Zhu, J., Weng, S., Dong, L., et al. (2018). Berberine treatment increases Akkermansia in the gut and improves high-fat diet-induced atherosclerosis in Apoe-/- mice. Atherosclerosis 268, 117–126. doi:10.1016/j.atherosclerosis.2017.11.023
Glossary
ACER2 Alkaline Ceramidase 2
AD Alzheimer’s disease
AEBP1 Adipocyte Enhancer-Binding Protein 1
APP Amyloid-beta precursor protein
BC Bipolar cell
BCs Bipolar cells
BRB Blood-retina barrier
Col1a1 Collagen Type I Alpha 1 Chain
DEGs Differentially expressed genes
DEP Dielectrophoretic
DME Diabetic macular edema
DN Diabetic nephropathy
DRECs Diabetic Retinal Endothelial Cells
EC Endothelial cell
FACS Fluorescence-Activated Cell Sorting
FVM Fibrovascular membrane
GLP-1 Glucagon-like peptide-1
GLP-1 RA Glucagon-like peptide-1 receptor agonist
GWAS Population-based genome-wide association studies
ICAM1 Intercellular Adhesion Molecule 1
IEG Immediate early gene
INL Inner nuclear layer
IPL Inner plexiform layer
JUND JunD Proto-Oncogene, AP-1 Transcription Factor Subunit
LCM Laser Capture Microdissection
MACS Magnetic-Activated Cell Sorting
MC Mesangial cell
NDR Non-diabetic retinopathy
NK Natural killer
NPDR-NDME Non-proliferative diabetic retinopathy without diabetic macular edema
NR Neural retina
OIR Oxygen-induced retinopathy
ONL Outer nuclear layer
OPL Outer plexiform layer
PBMC Peripheral blood mononuclear cell
PDE6G Phosphodiesterase 6G
PDR Proliferative diabetic retinopathy
POSTN Periostin
RBC Retinal bipolar cell
RGC Retinal Ganglion Cell
RLBP1 Retinaldehyde Binding Protein 1
RNAi RNA interference
RPCs Retinal pericytes
RPE Retinal pigment epithelium
SCENIC Single-cell regulatory network inference and clustering
ST Spatial transcriptomics
STZ Streptozotocin
DM Diabetes mellitus
TAG Triglycerides
TSS Transcription start sites
VEGFR1 Vascular endothelial growth factor receptor
Keywords: diabetic retinopathy (DR), multi-omics, single-cell RNA sequencing(scRNA-seq), transcriptomics, genomics, metabolomic, lipidomic
Citation: Li X, Dong X, Zhang W, Shi Z, Liu Z, Sa Y, Li L, Ni N and Mei Y (2024) Multi-omics in exploring the pathophysiology of diabetic retinopathy. Front. Cell Dev. Biol. 12:1500474. doi: 10.3389/fcell.2024.1500474
Received: 23 September 2024; Accepted: 25 November 2024;
Published: 11 December 2024.
Edited by:
Venkaiah Betapudi, United States Department of Health and Human Services, United StatesReviewed by:
Rachel Basques Caligiorne, Grupo Santa Casa BH, BrazilLianqun Wu, Fudan University, China
Angela Cappello, University of Bari Aldo Moro, Italy
Copyright © 2024 Li, Dong, Zhang, Shi, Liu, Sa, Li, Ni and Mei. This is an open-access article distributed under the terms of the Creative Commons Attribution License (CC BY). The use, distribution or reproduction in other forums is permitted, provided the original author(s) and the copyright owner(s) are credited and that the original publication in this journal is cited, in accordance with accepted academic practice. No use, distribution or reproduction is permitted which does not comply with these terms.
*Correspondence: Yan Mei, bWVpa21AMTYzLmNvbQ==; Ninghua Ni, bm5oMTk3MkAxNjMuY29t