- Department of Physiology, Michigan State University, East Lansing, MI, United States
Traditionally viewed as a fixed and homogeneous machinery for protein synthesis, the ribosome is increasingly recognized for its heterogeneity, as indicated by emerging studies highlighting the functional relevance of specialized ribosomes. However, whether ribosome heterogeneity is merely an outcome limited to specific conditions or a pervasive cellular phenomenon remains unclear, and existing evidence on the extensive existence of ribosome heterogeneity is scant. Here, we leveraged existing proteomic data and employed ribosome ratio-omics (RibosomeR), which comprehensively analyzes ribosome protein stoichiometry across various biological samples exhibiting distinct functions, developmental stages, and pathological states. Using the 80S monosome proteomic data, RibosomeR analysis unveils significant ribosome heterogeneity across different tissues, including fat, spleen, liver, kidney, heart, and skeletal muscles. Furthermore, examination of testes at various stages of spermatogenesis reveals distinct RibosomeR signatures during tissue development. Analysis of the whole cell proteomic data finds that RibosomeR undergoes dynamic changes during in vitro neuronal maturation, indicating functional associations with specific molecular aspects of neurodevelopment. In pathological contexts, RibosomeR signatures in gastric tumors demonstrate functional links to pathways associated with tumorigenesis. Additionally, dynamic alterations in RibosomeR are observed in macrophages following immune challenges. Collectively, our investigation across a diverse array of biological samples underscores the presence of ribosome heterogeneity, while previous studies observed functional aspects of ribosome specialization, in cellular function, development, and disease. The RibosomeR barcode serves as a valuable tool for elucidating these complexities.
Introduction
The ribosome, a macromolecular complex essential for protein synthesis, comprises approximately 80 highly conserved ribosome proteins (RPs) and four rRNAs in eukaryotes. Traditionally, ribosome composition was viewed as predominantly fixed and homogeneous, with dynamic regulation of protein synthesis primarily mediated by activity-dependent alterations in RP-mRNA interactions, post-transcriptional and post-translational modifications of mRNA and RP, and epigenetic effects from non-coding RNAs (Gebauer and Hentze, 2004; Song et al., 2021).
However, the discovery of ribosomes with distinct morphologies suggests potential heterogeneity in composition and function (Norris et al., 2021). Emerging evidence indicates that while some core RPs are consistently incorporated into ribosomes, ribosomes lacking specific RPs remain functional (Shi et al., 2017; Genuth and Barna, 2018). Furthermore, studies involving particular RPs have highlighted the functional relevance of specialized ribosomes. Mutations in specific RPs, such as RPL5, RPL11, RPS17, RPS19, and RPS24, have been associated with Diamond Blackfan Anemia (DBA) (Horos and von Lindern, 2012). It is hypothesized that ribosomes containing distinct RPs may preferentially translate specific pools of mRNAs. Notably, knockdown of Rps19 or Rpl11 leads to reduced translation of Bag1 and Csde1 mRNA, protein levels of which are diminished in DBA patient samples (Horos et al., 2012). Additionally, specialized ribosomes may translate mRNA pools linked to distinct cellular functions more effectively. For example, ribosomes containing RPS25 and RPL10A exhibit enrichment of non-overlapping mRNA pools associated with functionally diverse Gene Ontology (GO) groups (Shi et al., 2017).
Despite these insights, previous studies have primarily focused on the impact of individual RPs on ribosome function, leaving uncertainties regarding whether ribosome heterogeneity represents limited outcomes under specific conditions or a widespread cellular phenomenon. Moreover, evidence of extensive ribosome heterogeneity and its associations with cell identity, dynamic development, and pathogenesis remains limited. We hypothesize that functional ribosome heterogeneity involves alterations in numerous RPs on a genome-wide scale.
To explore this hypothesis, we employed a novel bioinformatics approach to examine the expression ratio of every RP. Our analysis, termed ribosome ratio-omics (RibosomeR), reveals specific heterogeneity associated with tissue type, in vivo and in vitro development, and disease. The RibosomeR signature, serving as a genome-wide ribosome barcode, identifies a broad extent of ribosome heterogeneity and suggests its functional significance.
Results
RibosomeR reveals tissue-specific ribosome heterogeneity
The functionality of tissues is theoretically determined by the expression of tissue-specific genes. However, as the level of mRNA transcripts often does not correlate positively with the corresponding protein levels, tissue-specific and activity-dependent alterations in translation provide necessary control over gene expression. Leveraging proteomic data on the 80S ribosome (Li et al., 2022), we analyzed the stoichiometry of core ribosome proteins (RPs) in various tissues. Specifically, we selected functionally distinct tissues: fat (adipose tissue), spleen (immune tissue), liver and kidney (epithelial tissues), and heart and skeletal muscle (muscle tissues) (Figure 1A). Our analysis revealed that each tissue possesses a distinct RibosomeR, providing a holistic signature of the stoichiometry of detected RPs (Supplementary Table S1). Principal component analysis (PCA) of RibosomeR identified four functionally distinct groups: fat, kidney and liver, heart and skeletal muscle, and spleen (Figure 1B). Independent correlation analysis demonstrated that fat and spleen are distinctly separated from each other and from the other four tissues (Figure 1C). Additionally, the RibosomeR heatmap unveiled four prominent clusters, with fat, spleen, kidney and liver, and heart and skeletal muscle each forming a distinct cluster (Figure 1D). Within the epithelial tissue cluster, kidney and liver showed partial separation, while within the muscle cluster, heart and skeletal muscle exhibited partial separation (Figure 1D). Our novel bioinformatic approach illustrates that genome-wide RibosomeR detection unveils tissue-specific ribosome heterogeneity with functional relevance.
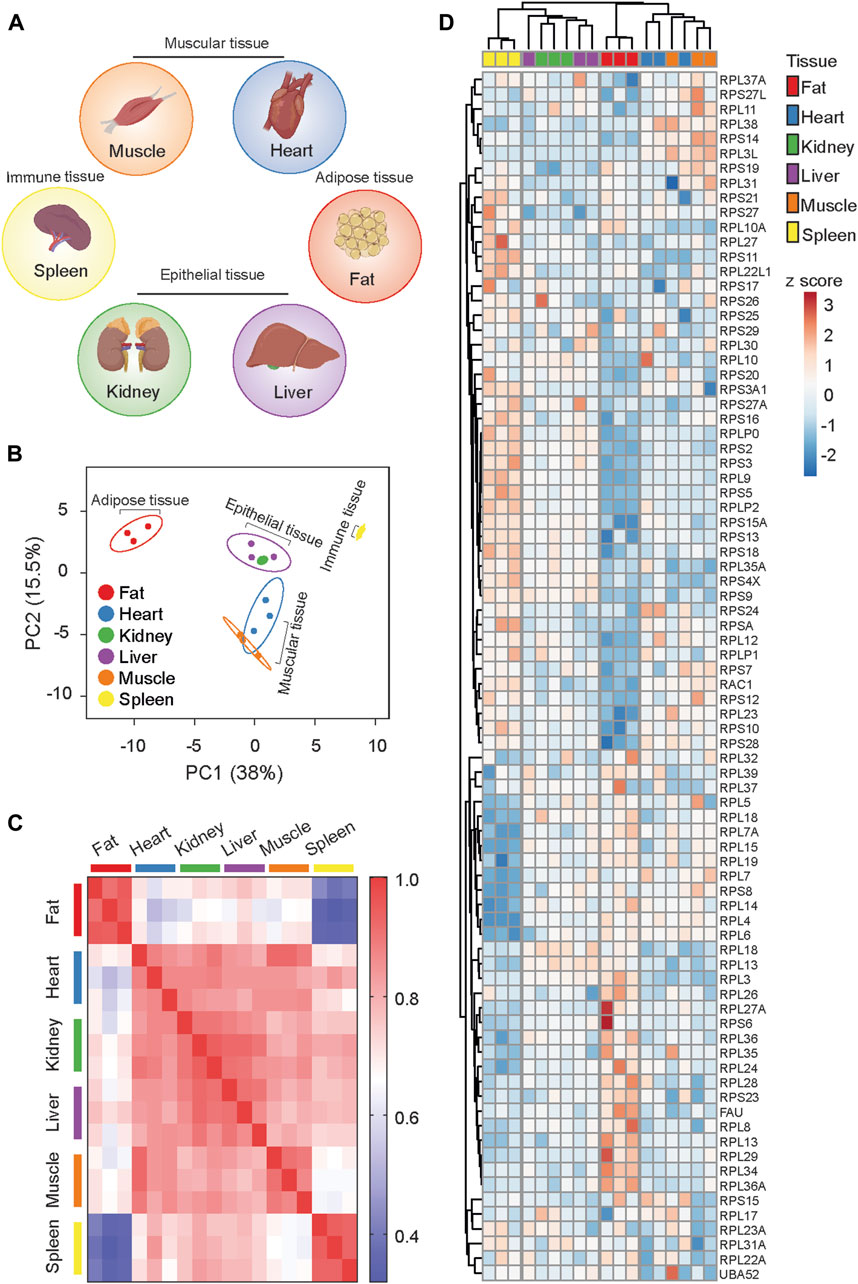
Figure 1. RibosomeR reveals ribosome heterogeneity in tissues with distinct physiological functions. (A) Tissues with specialized function. (B) Principal component analysis of the expression ratio of each RP in six mouse tissues (n = 3 for each tissue). The RibosomeR signatures of 82 RPs were compared. (C) Correlation matrix of the RibosomeR signatures in six mouse tissues. Correlation coefficient of >0.85 is observed within each tissue. The correlation coefficient between different tissues is expressed as color-coded. (D) Heatmap analysis of the RibosomeR signature in six mouse tissues. Rows (RP ratio) and columns (distinct tissues) are clustered using correlation distance and average linkage.
We further compared RibosomeR between fat and spleen, the most divergent tissues (Figure 1B), exhibiting the lowest correlation (Figure 1C). Interestingly, adipose tissue demonstrates a notably higher expression ratio predominantly in the large subunit RPs (Figures 2A–C) and a lower expression ratio in the small subunit RPs (Figures 2A, D, E). STRING analysis indicates functional interactions between MDM2 (murine double minute 2) and RACK1 (receptor for activated C kinase 1) and the RPs exhibiting an increased ratio in fat cells (Figure 2C). Furthermore, MRPL36, a mitochondrial RP, is predicted to interact with RPs showing a decreased ratio in fat (Figure 2E). Notably, RACK1 regulates adipogenesis (Kong et al., 2016), while MDM2 promotes the onset of fatty liver disease (Lin et al., 2022), both relevant to adipose tissue function.
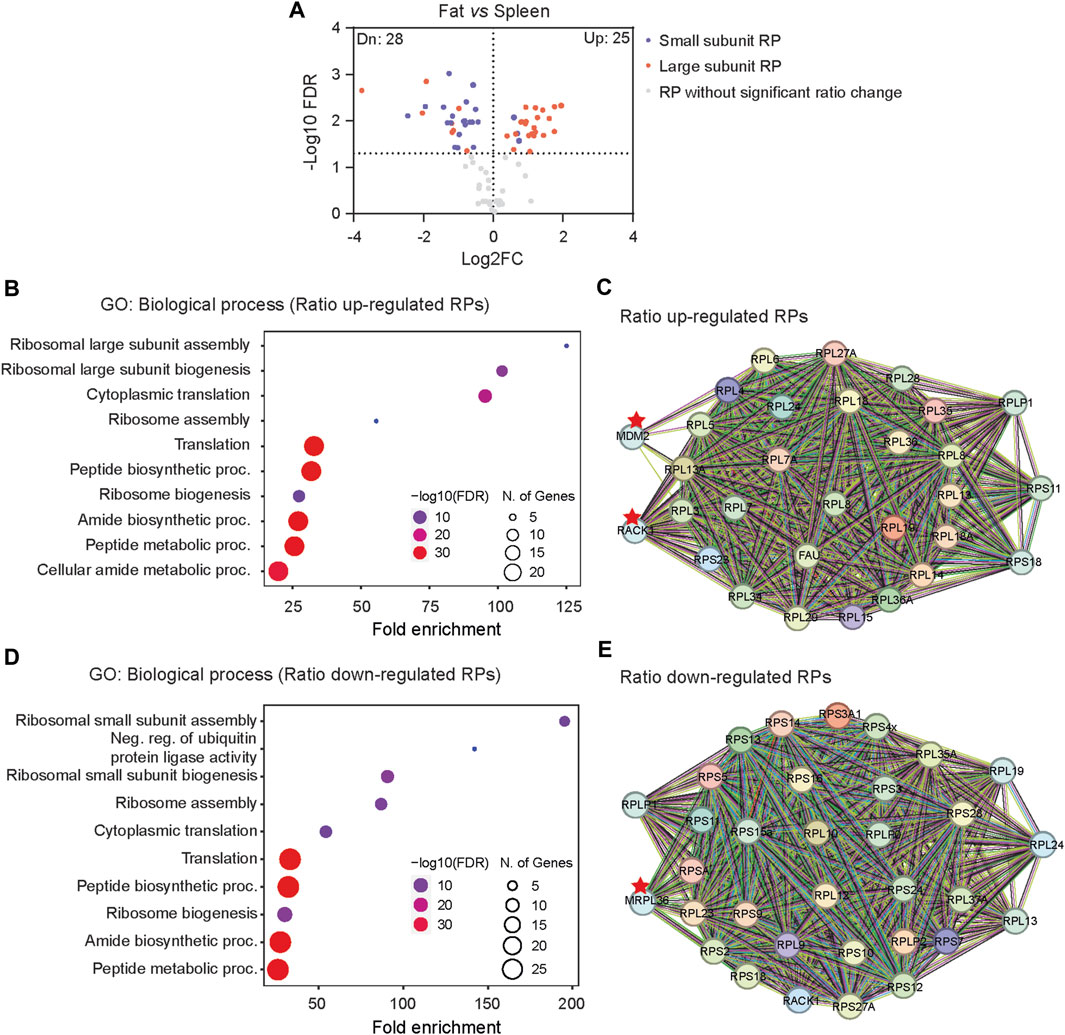
Figure 2. The distinct RibosomeR in fat suggests potential functional relevance to adipogenesis. (A) Volcano plot identifies up- and downregulated expression ratios of specific RPs in fat versus spleen. (B, D) The top 10 biological processes identified by GO (gene ontology) analysis with the RPs showing upregulated (B) and downregulated ratios (D) in fat versus spleen. (C, E) STRING analysis identifies the molecular and functional interaction with the RPs that show increased (C) and decreased (E) stoichiometry in fat versus spleen.
We further explored whether the actual expression levels of RP, rather than the expression ratio, could detect ribosome heterogeneity. Utilizing the iBAQ values of each RP as described by Li et al. (2022), our analysis identified differences between fat and spleen tissues (Supplementary Figure S1A). Moreover, the analysis revealed that, except for one RP protein (RPL37), the expression levels of all other RP proteins were downregulated in fat tissue (Supplementary Figure S1B). The findings suggest that fat cells may exhibit an overall lower abundance of ribosomes compared to spleen cells (assuming that the same sample weight contains an equivalent number of cells). However, the stoichiometry of RPs is not revealed through analysis using the iBAQ values of each RP.
RibosomeR reveals dynamic ribosome heterogeneity in developing testis
To explore dynamic changes in ribosome heterogeneity during development, we analyzed the RibosomeR of the 80S ribosome in mouse testes at various postnatal ages (Figure 3A). During spermatogenesis, the functional roles of testes vary with age. At 7 days postnatal, the testis supports spermatogonia genesis, characterized by 2N cells containing two copies of each chromosome. By 14 and 28 days, the testes support spermatocyte (4N cell) and spermatid (1N cell) development, respectively. The adult testis (∼60 days old) contains a mixture of these cells along with mature sperm cells. RibosomeR analysis of proteomic data (Li et al., 2022) revealed development-specific heterogeneity (Supplementary Table S2). PCA of RibosomeR segregated testes at different ages into four distinct groups (Figure 3B). Correlation analysis demonstrated stark differences in ribosome stoichiometry between adult testes and those in development at 7, 14, and 28 days of age (Figure 3C). The RibosomeR heatmap depicted four distinct clusters (Figure 3D). Notably, hierarchical clustering revealed that the RibosomeR signature in 7-day-old testes differed progressively from those at 14 days, 28 days, and adulthood (Figure 3D). We further compared the progressive alteration of RibosomeR in spermatogonia, spermatocyte and spermatid (Figures 3E, F). The expression ratios of RPL10L and PRL39L are higher in spermatocyte than in spermatogonia (Figure 3E). The expression ratio of RPL10L is further increased in spermatid (Figure 3F). These findings underscore the association of progressive alterations in ribosome heterogeneity with distinct stages of testis development. Such progressive RibosomeR alterations suggest an increase in the number of RPL39L-containing ribosomes from spermatogonia to spermatocyte. The number of RPL10L-containing ribosomes rises from spermatogonia to spermatocyte and further increases from spermatocyte to spermatid (Figure 3G). Interestingly, RPL10L is essential for meiotic processes (Jiang et al., 2017), while mutations in PPL10L lead to male infertility (Tu et al., 2020). Furthermore, deletion of RPL39L causes the mal-development of sperms with bent tails (Zou et al., 2021; Li et al., 2022), highlighting the indispensable role of RPL39L in spermiogenesis.
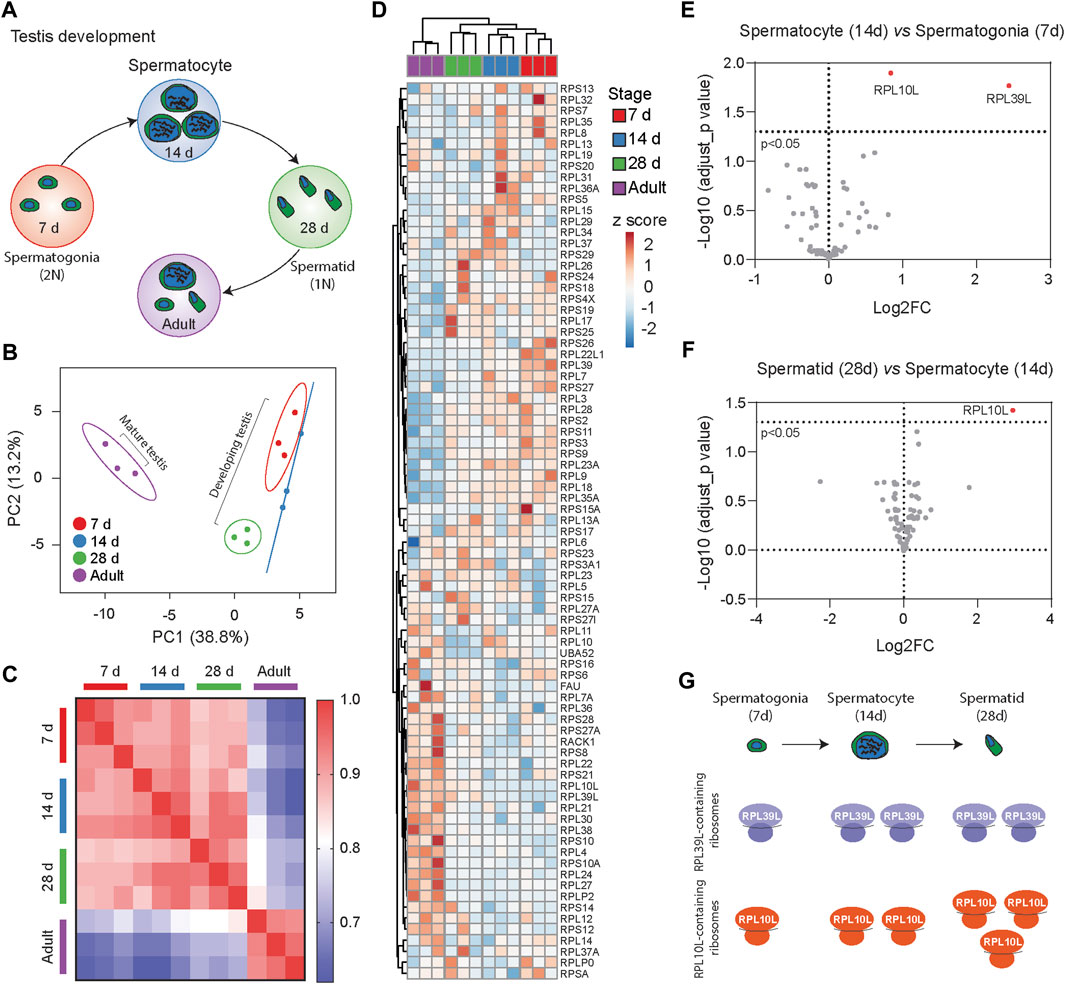
Figure 3. RibosomeR reveals distinct ribosome heterogeneity during testis development. (A) Testes at different development stages display distinct cell types during spermatogenesis. (B) Principal component analysis of RP ratios in developing and mature mouse testes (n = 3 for each group). RibosomeR signatures of 81 RPs were compared. (C) Correlation matrix of the RibosomeR signatures in developing and mature mouse testes. Correlation coefficient of >0.91 is detected within each group. The correlation coefficient between different tissues is expressed as color-coded. (D) Heatmap analysis of the RibosomeR signatures in developing and mature mouse testes. Rows are centered; unit variance scaling is applied to rows. Rows (RP ratio) and columns (7-day, 14-day, 28-day, and adult testis) are clustered using correlation distance and average linkage. (E) The volcano plot shows an upregulation in the expression ratios of RPL10L and PRL39L at day 14 compared to day 7 during testis development. (F) The volcano plot shows an upregulation in the expression ratio of RPL10L at day 28 compared to day 14 during testis development. (G) Analysis of RibosomeR signatures suggests an increase in the number of RPL39L-containing ribosomes from spermatogonia to spermatocyte. The number of RPL10L-containing ribosomes rises from spermatogonia to spermatocyte and further increases from spermatocyte to spermatid.
RibosomeR reveals dynamic ribosome heterogeneity during neuronal maturation
To elucidate dynamic changes in ribosome heterogeneity during the development of specific cell types, we analyzed RibosomeR in cultured cortical neurons (Supplementary Table S3). In the well-characterized in vitro maturation process, neurons at day in vitro (DIV) five exhibit moderately developed axons and dendrites with limited functional synapses, while those at DIV15 demonstrate elaborated axons and dendrites, full-scale synaptogenesis, and robust expression of synaptic proteins (Dotti et al., 1988; Lesuisse and Martin, 2002; Zhou et al., 2009) (Figure 4A).
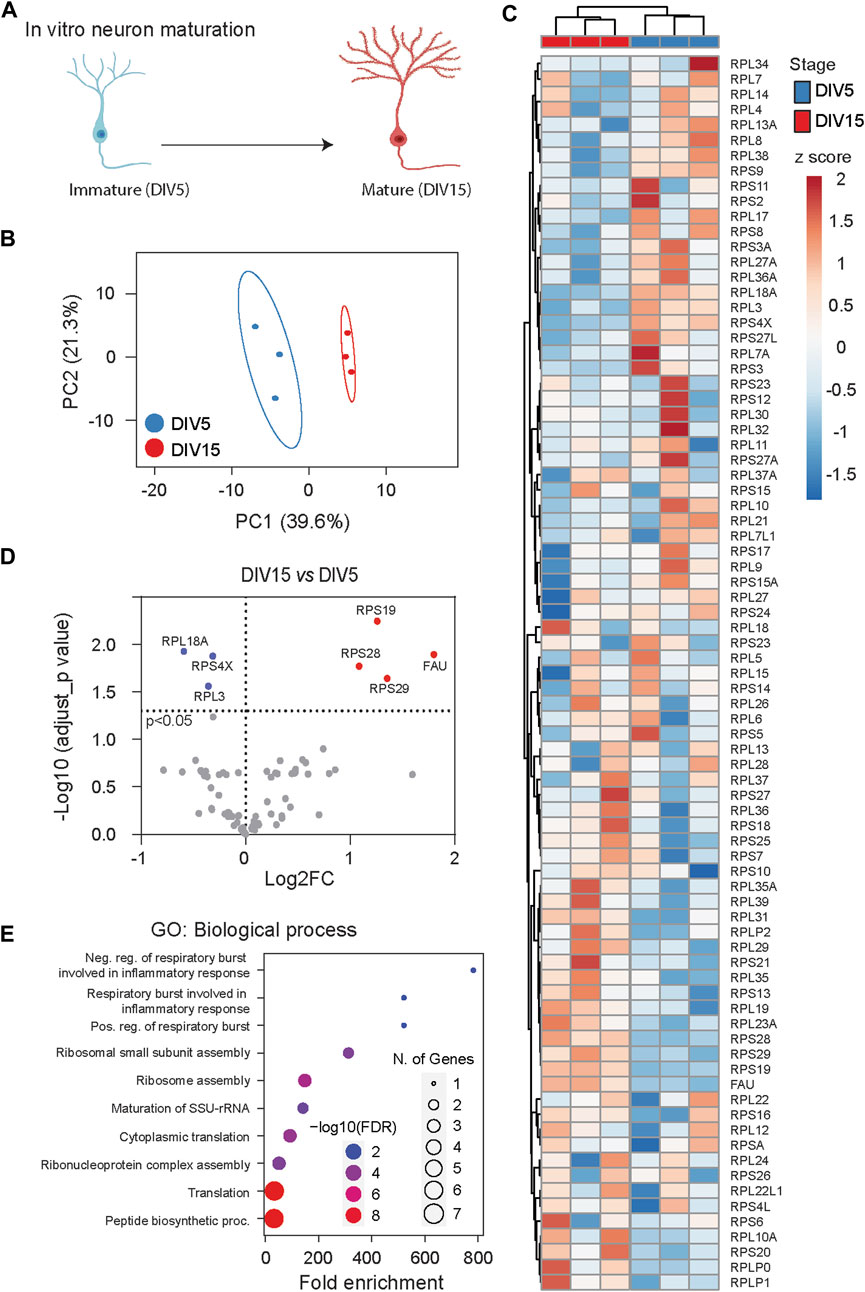
Figure 4. RibosomeR reveals ribosome heterogeneity during in vitro neuron maturation. (A) Cultured cortical neurons undergo morphological and functional maturation. From DIV (days in vitro) 5 to DIV 15, axons and dendrites become elongated and branched; functional synapses are formed. (B) Principal component analysis of RP ratios in DIV 5 and DIV 15 neurons (n = 3 for each group). RibosomeR signatures of 81 RPs were compared. (C) Heatmap analysis of the RibosomeR signatures in DIV 5 and DIV 15 neurons. Rows (RP ratio) and columns (DIV 5 and DIV 15 neurons) are clustered using correlation distance and average linkage. (D) Volcano plot identifies upregulated and downregulated expression ratios of specific RPs during in vitro maturation. (E) The top 10 biological processes identified by GO (gene ontology) analysis with the RPs that show ratio alteration during in vitro maturation.
We examined the whole-cell lysate proteomic data collected from DIV 5 and DIV 15 neurons (Sharma et al., 2015). PCA of RibosomeR revealed distinct ribosome compositions between immature DIV 5 neurons and mature DIV 15 neurons (Figure 4B). Heatmap analysis further highlighted distinct RibosomeR signatures in DIV 5 and DIV 15 neurons as separate clusters (Figure 4C). Employing the differential RibosomeR assay, we observed increased stoichiometry of four RPs and decreased stoichiometry of three RPs in mature neurons (Figure 4D). Gene ontology (GO) analysis with these seven RPs revealed that maturation-induced changes in ribosome heterogeneity are associated with respiratory burst among the top 10 biological processes (Figure 4E). Notably, respiratory burst is functionally relevant to the release of reactive oxygen species (ROS), dynamic alterations of which control synaptic plasticity and neural development (Massaad and Klann, 2011; Oswald M. C. et al., 2018; Oswald M. C. W. et al., 2018).
RibosomeR reveals ribosome heterogeneity in tumorigenesis
In comparison to normal tissue development, tumorigenesis is characterized by uncontrolled proliferation and cell immortalization. We investigated RibosomeR signatures using the whole-cell lysate proteomic data collected from 82 human normal gastric tissues and 58 human gastric tumor tissues (Figure 5A) (Ni et al., 2019). PCA of RibosomeR (Supplementary Table S4) revealed two partially separated groups (Figure 5B). Heatmap analysis unveiled two main clusters (Figure 5C). One cluster was enriched with normal gastric tissues (76 normal and 10 tumor tissues), while the other predominantly consisted of gastric tumor tissues (48 tumor and 6 normal tissues) (Figure 5C). In comparison with normal gastric tissues, tumor tissues exhibited increased expression ratios in 34 RPs and decreased ratios in 23 RPs (Figure 5D). GO analysis with these RPs identified neddylation among the top 10 biological processes (Figure 5E), potentially linking it to tumorigenesis (Zhou et al., 2019; Naik et al., 2020). Interestingly, among the two RPs associated with the Regulation of Protein Neddylation GO process, RPL11 could be neddylated and regulates the function of the tumor suppressor p53 (Sundqvist et al., 2009). RPL5 and RPL11 negatively regulate neddylation through interaction with MDM2 (Bailly et al., 2016). STRING prediction revealed that RPs with increased ratio changes interact with FBL (Fibrillarin), BYSL (Bystin or Bystin-Like), and MDM2 (Figure 5F), known to regulate tumorigenesis through p53 (Alarcon-Vargas and Ronai, 2002; Senturk and Manfredi, 2012). Additionally, STRING analysis with RPs showing decreased ratios suggested functional interactions with RACK1 (Figure 5G), known to regulate various aspects of ribosome function and tumorigenesis (Li and Xie, 2015).
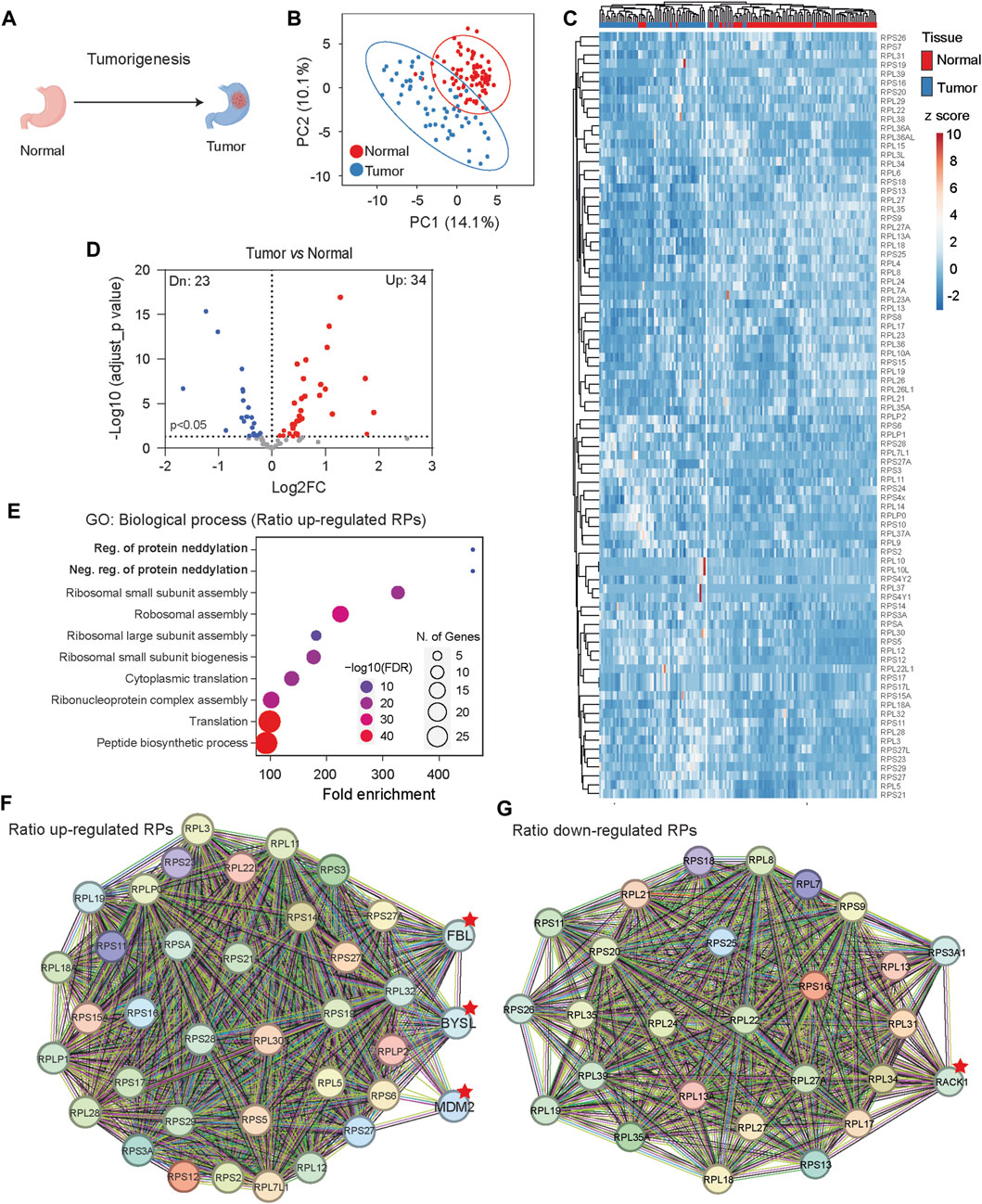
Figure 5. Gastric tissue tumorigenesis shows distinct RibosomeR signatures. (A) Tumorigenesis alters cell fate and function in gastric tissue. (B) Principal component analysis of RP ratios in human normal gastric tissues (n = 82) and gastric tumor tissues (n = 58). Proteomic data from normal and tumor human gastric tissues were analyzed. The RibosomeR signature of 86 RPs was compared. (C) Heatmap analysis of the RibosomeR signatures in normal and tumor tissues. Rows (RP ratio) and columns (normal and tumor gastric tissues) are clustered using correlation distance and average linkage. (D) Volcano plot identifies up- and downregulated expression ratios of specific RPs in tumor tissue. (E) The top 10 biological processes identified by GO (gene ontology) analysis with the RPs that show ratio alteration in the tumor tissues. (F, G) STRING analysis and identification of the potential molecular and functional interaction with the RPs that show increased (F) and decreased (G) stoichiometry in the tumor tissues.
RibosomeR reveals ribosome heterogeneity in acute macrophage activation
Macrophages, as early-responding immune cells, play a pivotal role in reacting to infection or inflammation and neutralizing pathogens (Figure 6A) (Zhang and Wang, 2014). We analyzed single-cell proteomic data collected from the murine macrophage cell line RAW 264.7 (Woo et al., 2022). These RAW 264.7 cells were treated with lipopolysaccharide (LPS), a main component of bacterial membranes and a potent reagent for macrophage activation. We compared single-cell RibosomeR signatures between 10 control cells and 38 LPS-challenged cells. The single-cell proteomic data provided reasonable coverage, detecting more than 50 RPs in each cell (Supplementary Table S5). PCA of RibosomeR revealed two groups with limited overlap (Figure 6B). Heatmap analysis unveiled two main clusters (Figure 6C). Hierarchical clustering demonstrated that one main cluster contained all control cells in one sub-cluster and a small fraction of LPS-challenged cells (i.e., three cells) in another sub-cluster (Figure 6C). The other main cluster comprised the remaining LPS-challenged cells (i.e., 35 cells) (Figure 6C). Compared to the 10 control cells, the 38 LPS-challenged cells exhibited increased stoichiometry in 9 RPs and decreased stoichiometry in nine other RPs (Figure 6D). GO analysis revealed that among the top 10 biological processes, the dynamic changes in ribosome heterogeneity were functionally associated with responses to infection (Figure 6E).
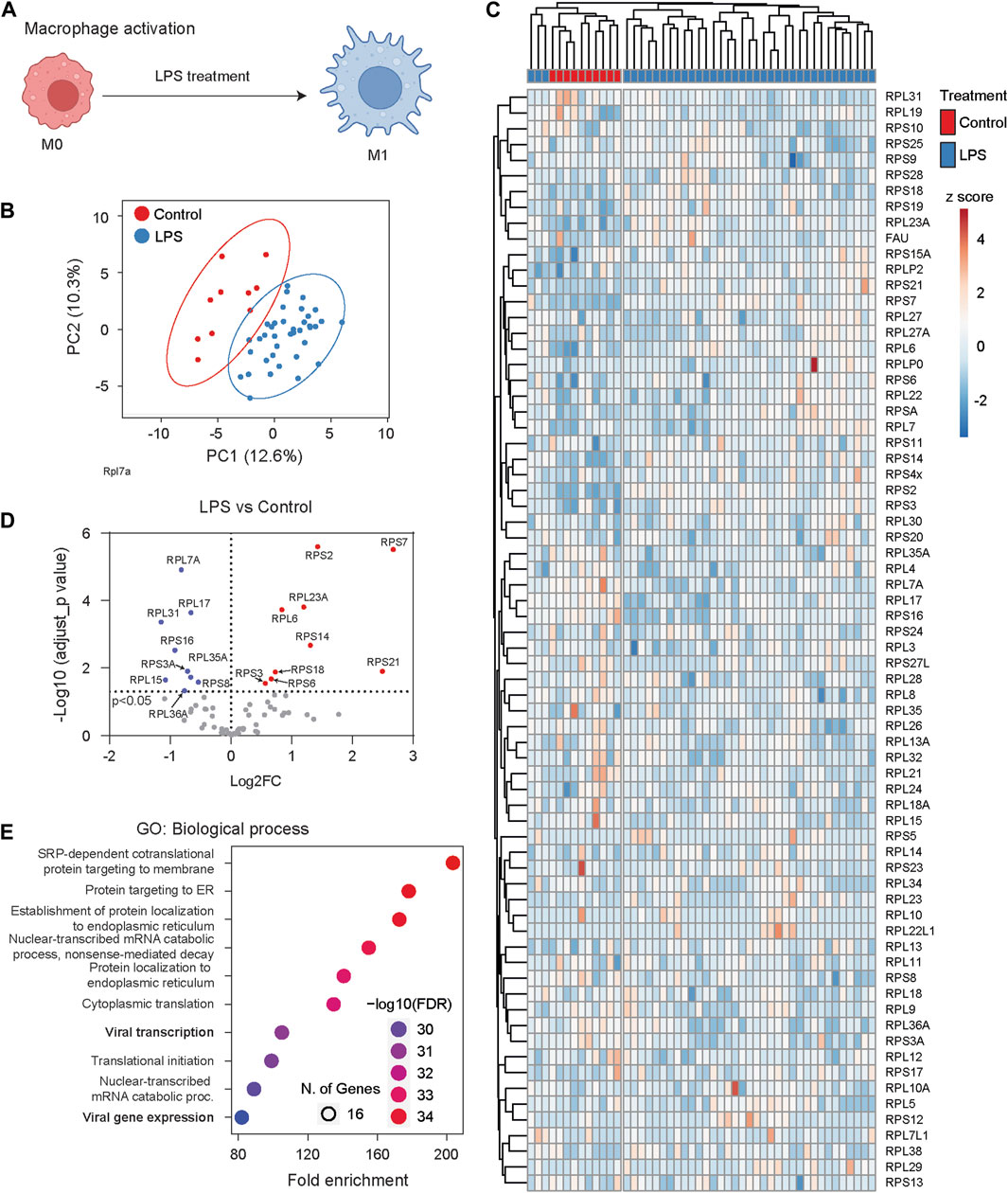
Figure 6. Macrophage cells undergo dynamic RibosomeR changes following activation. (A) The proinflammatory activator LPS (lipopolysaccharide) stimulates macrophage cells, leading to immune responses such as the secretion of cytokines. (B) Principal component analysis of RP ratios in control (n = 10) and LPS-treated macrophages (n = 38). The RibosomeR signature of 71 RPs was compared. (C) Heatmap analysis of RibosomeR signatures in control and LPS-treated macrophage cells. Rows (RP ratio) and columns (control and LPS-treated cells) are clustered using correlation distance and average linkage. (D) Volcano plot identifies upregulated and downregulated expression ratio of specific RPs in the LPS-treated cells. (E) The top 10 biological processes identified by GO (gene ontology) analysis with the RPs that show ratio alteration in the LPS-treated cells.
Discussion
Precise and dynamic regulation of gene expression is indispensable for cell function and development. The role of specific ribosome proteins (RPs) in mRNA translation and their association with pathology and disease underscore the proposed functional relevance of ribosome heterogeneity, although this remains a subject of debate. In this study, we conducted the first genome-wide and comprehensive characterization of ribosome composition in various tissues under physiological and pathological conditions. Using RibosomeR, we identified ribosome heterogeneity associated with tissue type and development, as well as alterations in RibosomeR under disease conditions. Our findings underscore the broad existence of ribosome heterogeneity and its functional significance. Moreover, we anticipate that RibosomeR, as an effective analytical method, will be instrumental in unraveling an essential and novel dimension of gene expression regulation.
Previous studies have noted a suggestive differential expression of specific ribosome protein (RP) mRNAs across various mammalian tissues (Bortoluzzi et al., 2001; Gupta and Warner, 2014; Guimaraes and Zavolan, 2016). Approximately 25% of RP genes exhibit tissue-specific mRNA expression (Guimaraes and Zavolan, 2016), although this observation is also debated (Gupta and Warner, 2014). The functional relevance of mRNA heterogeneity remains unclear, as mRNA expression profiles do not consistently segregate into distinct PCA groups based on tissue function. Hierarchical clustering analysis reveals that functionally similar tissues are not necessarily clustered together but rather exhibit some degree of separation. For instance, the RP mRNA signature in kidney (an epithelial tissue) shows greater similarity to adipose tissue than to liver (another epithelial tissue) (Guimaraes and Zavolan, 2016). In a study by Sugihara et al., ribosome proteomic data from liver, testis, and mammary gland revealed differential levels of several RP-like proteins but failed to detect tissue-specific ribosome heterogeneity (Sugihara et al., 2010). In our study, we utilize RibosomeR and, for the first time, identify functionally relevant and tissue-specific heterogeneity. Adipose, epithelial, muscular, and immune tissues exhibit distinct RibosomeR patterns. However, we observe that RibosomeR may not adequately detect significant heterogeneity among tissues of similar types. For instance, heart and skeletal muscle, as well as liver and kidney, exhibit slightly overlapping RibosomeR patterns. It remains unclear whether the presence of mixed cell types within each tissue compromises the resolution of RibosomeR. Comparing RibosomeR between two tissues with markedly different physiological functions—fat and spleen—we identify striking differences in ribosome composition. Our RibosomeR analysis further suggests the functional relevance of tissue-specific heterogeneity, exemplified by the involvement of RACK1 and MDM2 in adipogenesis and fatty liver disease.
If ribosome heterogeneity is indeed associated with biological function, it is reasonable to expect that ribosome composition in a specific tissue dynamically adjusts to functional changes during development. Even within tissues comprising different cell types, RibosomeR reveals the interaction of development and function across distinct developmental stages. For instance, in testis tissue, RibosomeR captures differences across four distinct development stages. Similarly, in cultured primary cells primarily composed of neurons rather than mixed cell types, RibosomeR distinguishes between immature and mature neurons. The ribosome changes associated with maturation detect gene ontology (GO) functions related to reactive oxygen species (ROS) production. Notably, ROS not only influences neural development and maturation (Wilson et al., 2018) but also exhibits excessive production in Diamond-Blackfan anemia (DBA) (Rio et al., 2019). Intriguingly, among the four ribosome proteins showing an increased ratio in mature DIV 15 neurons, RPS19, RPS28, and RPS29 mutations have been identified in DBA (Horos and von Lindern, 2012; Dokal et al., 2022). Causally, deficient RPS19, mutations of which are most frequent in DBA (Draptchinskaia et al., 1999), directly leads to anemia in mice (Debnath et al., 2017).
Ribosome alterations have been implicated in cancer and tumorigenesis (Guimaraes and Zavolan, 2016; Kang et al., 2021). Targeting ribosomes represents a potential avenue for cancer therapy (Gilles et al., 2020). Guimaraes and Zavolan analyzed The Cancer Genome Atlas (TCGA) data and found that certain ribosome protein (RP) genes, such as RPL39L, are commonly dysregulated in different cancer types; however, whether cancer cells and normal cells exhibit distinct ribosome heterogeneity remains unclear (Guimaraes and Zavolan, 2016). Our RibosomeR analysis, conducted with a large proteomic dataset comprising 82 normal and 58 gastric tumor tissues, revealed two separate clusters enriched with normal and tumor samples, respectively. Among the 34 RPs exhibiting an increased ratio in cancer, missense mutations in RPL11 and RPL5 occur in 73% and 66% of the 19,000 cancer samples across 49 cancer types (Orsolic et al., 2020). While an increase in RPS15A associates with the progression of various cancers (Guo et al., 2018), a decrease in RPS15A inhibits cancer cell proliferation (Zhao et al., 2015; Yao et al., 2016). Among the 23 RPs showing a decreased ratio in cancer, heterozygous deletion of RPL22 is observed in approximately 10% of T-acute lymphoblastic leukemia (T-ALL) cases (Rao et al., 2012). Various mutations in RPL22 are also found in solid cancers (Ferreira et al., 2014).
While it remains unclear whether tumor-specific ribosome heterogeneity regulates the translation of tumor-specific proteins or reflects a pathological outcome, STRING prediction suggests functional associations of RPs with an increased ratio with the MDM2/p53 axis, FBL, and BYSL. Among the RibosomeR -identified tumor-associated RPs with an increased ratio, RPL5, RPL11, RPS3, RPS14, RPS19, RPS27, RPS27A, and RPS27L have been found to physically interact with MDM2 (Kang et al., 2021), which inhibits the function of the tumor suppressor p53 (Alarcon-Vargas and Ronai, 2002; Senturk and Manfredi, 2012). Beyond its established role in regulating transcription, the MDM2/p53 axis, which affects and responds to nucleolar activity (Liu et al., 2016), may also participate in the ribosome assembly process. The interaction between RPs and FBL suggested by RibosomeR may imply altered ribosome biogenesis in gastric cancer (Nguyen Van Long et al., 2022). BYSL, involved in rRNA processing and 40S ribosome biogenesis during development and cancer cell proliferation (Adachi et al., 2007; Wang et al., 2009), could play a role in cancer progression.
STRING prediction suggests that RACK1, which functionally interacts with RPs showing a decreased ratio, stably associates with ribosomes (Johnson et al., 2019) and regulates the translation of specific mRNA pools (Majzoub et al., 2014; Thompson et al., 2016). Intriguingly, RACK1 affects cancer progression in a tissue-dependent manner; while it promotes breast and lung cancers (Li and Xie, 2015), it suppresses gastric tumors (Deng et al., 2012).
Single-cell RibosomeR analysis unveils dynamic ribosome alterations in macrophages following immune challenge. Intriguingly, RibosomeR responses to lipopolysaccharide (LPS) are not uniform across all cells. The three LPS-challenged cells exhibit distinct RibosomeR signatures compared to both the control cells and the remaining 35 LPS-challenged cells. This observation aligns with the emerging understanding that macrophages undergo epigenetic diversification (Ma et al., 2022). The different single-cell RibosomeR signatures in LPS-stimulated macrophages further suggest functional diversity.
In summary, RibosomeR identifies the broad existence of ribosome heterogeneity with functional relevance to development and disease. This analytical approach provides a novel strategy to assess ribosome composition and function.
Limitation of the study
The RibosomeR-identified heterogeneity in different tissues and during in vivo testis development relies on proteomic data obtained from purified 80S ribosomes. It's important to acknowledge that active translation primarily occurs via polyribosomes, albeit a recent study discovered that the 80S monosomes handle local mRNA translation at neuronal synapses (Biever et al., 2020). At present, it remains unclear whether polyribosomes and the 80S monosomes possess distinct ribosomal protein compositions.
The RibosomeR-identified heterogeneity during in vitro neuronal maturation, gastric tumorigenesis, and macrophage activation relies on proteomic data from whole-cell lysates of bulk tissues or single cells. It is uncertain if all the ribosomal proteins (RPs) detected within the whole-cell extracts are assembled into functional ribosomes. Thus, it is reasonable to suggest that the current RibosomeR analysis offers a remodeling potential of ribosome composition. Future experiments aimed at gathering proteomic data from purified polyribosomes and analyzing ribosomal protein expression ratios are crucial for advancing our understanding of the significance of ribosome heterogeneity.
We anticipate that RibosomeR will introduce a robust new analysis approach for detecting ribosome heterogeneity using current data. Moreover, we hope it will stimulate future validation efforts when polyribosome data becomes available.
Experimental procedures
Datasets
We used the published public proteomic data. The relative expression ratio of RPs in various tissues was analyzed with proteomic data of isolated 80S monosomes from fat, spleen, liver, kidney, heart, and skeletal muscle (Li et al., 2022). The relative expression ratio of RPs in developing testes was analyzed with proteomic data of isolated 80S monosomes from 7-day, 14-day, 28-day, and adult testes (Li et al., 2022). The relative expression ratio of RPs in cultured cortical neurons (Sharma et al., 2015) and human normal and tumor gastric tissues (Ni et al., 2019) was analyzed with whole-cell proteomic data. The relative expression ratio of RPs in control and activated macrophage cells was analyzed with single-cell proteomic data (Woo et al., 2022). The whole-cell extracts were obtained after sonication in lysis buffers containing detergent (2% SDS), reducing agent (5, or 10 or 100 mM DTT) at high temperatures (99°C, or 95°C, or 70°C) followed by alkylation with 10 mM iodoacetamide (Sharma et al., 2015; Ni et al., 2019; Woo et al., 2022).
Ribosome ratio-omics (RibosomeR)
We calculated the expression ratio of each RP to the level of all detected RPs. Depending on the isolation and proteomic detection method, most of the core RPs were detected. Data from various tissues and developing testes reported the expression of 82 and 81 RPs, respectively (Li et al., 2022). Data from cultured cortical neurons (Sharma et al., 2015), human normal and tumor gastric tissues (Ni et al., 2019), and control and activated macrophage cells (Woo et al., 2022) reported the expression of 81, 79, 86, and 71 RPs, respectively. The iBAQ algorithm was used for the ribosomal protein ratio analysis because the iBAQ values are proportional to the molar quantities of the proteins (Woo et al., 2022). The expression ratio of each RP was calculated by (individual RP iBAQ value)/(total RPs iBAQ value). The holistic ribosome ratio-omic (RibosomeR) values are reported in Supplementary Tables S1–S5.
Principal component analysis (PCA)
The principal component analysis was performed using ClustVis based on the pcaMethods R package (Metsalu and Vilo, 2015). Unit variance scaling is applied to rows; SVD (singular value decomposition) with imputation is used to calculate principal components. Prediction ellipses are such that, with a probability of 0.95, a new observation from the same group will fall inside the ellipse.
Correlation analysis
The correlation matrix, which captures the interrelationships among different samples, was computed utilizing the Pearson correlation coefficient within the R statistical environment. Subsequently, the correlation results were visually presented through plots generated using GraphPad Prism 9 software.
Heatmap clustering analysis
The Heatmap clustering analysis was performed using ClustVis (Metsalu and Vilo, 2015) based on the pheatmap R package (version 0.7.7). Rows are centered; unit variance scaling is applied to rows. Both rows and columns are clustered using correlation distance and average linkage.
Differential RibosomeR analysis and volcano plot
The two-tailed t-tests were performed to identify the RPs showing significantly different expression ratios. The Benjamini-Hochberg correction method was applied to adjust the obtained p values. A discernible difference in RP expression ratios is deemed significant if the adjusted p value falls below the conventional threshold of 0.05. The identified RPs are presented in the volcano plots utilizing GraphPad Prism 9 software, enabling visualization of the magnitude and significance of the observed changes in RP ratios.
Gene ontology (GO) analysis
The GO analysis and enrichment were performed using ShinyGO (Ge et al., 2020) with a false discovery rate (FDR) cutoff set at 0.05. The top 10 biological process terms were extracted and presented. The relevance of biological processes is listed in descending order based on their FDR significance and fold enrichment.
STRING analysis
The STRING database was used to predict potential molecular interactions with the RPs, which showed ratio alterations. Analysis was conducted using the default parameters within the STRING database. The RPs with altered expression ratios are placed in the center shell. The interacting proteins are placed in the outer shell.
Illustration graphs
Illustration graphs were made with BioRender.
One-sentence summary
Ratio-omics signature of ribosome deciphers functionally relevant heterogeneity in development and disease.
Data availability statement
The raw MS data of human stomach tissues presented in the study are deposited in the proteomeXchange via the iProX partner repository, accession number PXD011821.
Author contributions
YG: Conceptualization, Formal Analysis, Methodology, Writing–original draft, Writing–review and editing. HW: Conceptualization, Funding acquisition, Investigation, Project administration, Supervision, Writing–original draft, Writing–review and editing.
Funding
The authors declare that financial support was received for the research, authorship, and/or publication of this article. This research was supported by NIH grants R01MH124992 and R01MH119149 (to HW).
Conflict of interest
The authors declare that the research was conducted in the absence of any commercial or financial relationships that could be construed as a potential conflict of interest.
The authors declared that they were an editorial board member of Frontiers, at the time of submission. This had no impact on the peer review process and the final decision.
Publisher’s note
All claims expressed in this article are solely those of the authors and do not necessarily represent those of their affiliated organizations, or those of the publisher, the editors and the reviewers. Any product that may be evaluated in this article, or claim that may be made by its manufacturer, is not guaranteed or endorsed by the publisher.
Author disclaimer
The content is solely the responsibility of the authors and does not necessarily represent the official views of the National Institutes of Health.
Supplementary material
The Supplementary Material for this article can be found online at: https://www.frontiersin.org/articles/10.3389/fcell.2024.1414269/full#supplementary-material
SUPPLEMENTARY TABLE S1 | Expression ratio of RPs in different mouse tissues of fat, heart, skeletal muscle, kidney, liver, and spleen.
SUPPLEMENTARY TABLE S2 | Expression ratio of RPs in mouse testes at different ages.
SUPPLEMENTARY TABLE S3 | Expression ratio of RPs in DIV5 and DIV15 neurons.
SUPPLEMENTARY TABLE S4 | Expression ratio of RPs in human normal and tumor gastric tissues.
SUPPLEMENTARY TABLE S5 | Single-cell expression ratio of RPs in control and LPS-treated RAW 264.7 cells.
References
Adachi, K., Soeta-Saneyoshi, C., Sagara, H., and Iwakura, Y. (2007). Crucial role of Bysl in mammalian preimplantation development as an integral factor for 40S ribosome biogenesis. Mol. Cell Biol. 27 (6), 2202–2214. doi:10.1128/MCB.01908-06
Alarcon-Vargas, D., and Ronai, Z. (2002). p53-Mdm2--the affair that never ends. Carcinogenesis 23 (4), 541–547. doi:10.1093/carcin/23.4.541
Bailly, A., Perrin, A., Bou Malhab, L. J., Pion, E., Larance, M., Nagala, M., et al. (2016). The NEDD8 inhibitor MLN4924 increases the size of the nucleolus and activates p53 through the ribosomal-Mdm2 pathway. Oncogene 35 (4), 415–426. doi:10.1038/onc.2015.104
Biever, A., Glock, C., Tushev, G., Ciirdaeva, E., Dalmay, T., Langer, J. D., et al. (2020). Monosomes actively translate synaptic mRNAs in neuronal processes. Science 367 (6477), eaay4991. doi:10.1126/science.aay4991
Bortoluzzi, S., d'Alessi, F., Romualdi, C., and Danieli, G. A. (2001). Differential expression of genes coding for ribosomal proteins in different human tissues. Bioinformatics 17 (12), 1152–1157. doi:10.1093/bioinformatics/17.12.1152
Debnath, S., Jaako, P., Siva, K., Rothe, M., Chen, J., Dahl, M., et al. (2017). Lentiviral vectors with cellular promoters correct anemia and lethal bone marrow failure in a mouse model for diamond-blackfan anemia. Mol. Ther. 25 (8), 1805–1814. doi:10.1016/j.ymthe.2017.04.002
Deng, Y. Z., Yao, F., Li, J. J., Mao, Z. F., Hu, P. T., Long, L. Y., et al. (2012). RACK1 suppresses gastric tumorigenesis by stabilizing the beta-catenin destruction complex. Gastroenterology 142 (4), 812–823 e815. doi:10.1053/j.gastro.2011.12.046
Dokal, I., Tummala, H., and Vulliamy, T. (2022). Inherited bone marrow failure in the pediatric patient. Blood 140 (6), 556–570. doi:10.1182/blood.2020006481
Dotti, C. G., Sullivan, C. A., and Banker, G. A. (1988). The establishment of polarity by hippocampal neurons in culture. J. Neurosci. 8 (4), 1454–1468. doi:10.1523/JNEUROSCI.08-04-01454.1988
Draptchinskaia, N., Gustavsson, P., Andersson, B., Pettersson, M., Willig, T. N., Dianzani, I., et al. (1999). The gene encoding ribosomal protein S19 is mutated in Diamond-Blackfan anaemia. Nat. Genet. 21 (2), 169–175. doi:10.1038/5951
Ferreira, A. M., Tuominen, I., van Dijk-Bos, K., Sanjabi, B., van der Sluis, T., van der Zee, A. G., et al. (2014). High frequency of RPL22 mutations in microsatellite-unstable colorectal and endometrial tumors. Hum. Mutat. 35 (12), 1442–1445. doi:10.1002/humu.22686
Ge, S. X., Jung, D., and Yao, R. (2020). ShinyGO: a graphical gene-set enrichment tool for animals and plants. Bioinformatics 36 (8), 2628–2629. doi:10.1093/bioinformatics/btz931
Gebauer, F., and Hentze, M. W. (2004). Molecular mechanisms of translational control. Nat. Rev. Mol. Cell Biol. 5 (10), 827–835. doi:10.1038/nrm1488
Genuth, N. R., and Barna, M. (2018). The discovery of ribosome heterogeneity and its implications for gene regulation and organismal life. Mol. Cell 71 (3), 364–374. doi:10.1016/j.molcel.2018.07.018
Gilles, A., Frechin, L., Natchiar, K., Biondani, G., Loeffelholz, O. V., Holvec, S., et al. (2020). Targeting the human 80S ribosome in cancer: from structure to function and drug design for innovative adjuvant therapeutic strategies. Cells 9 (3), 629. doi:10.3390/cells9030629
Guimaraes, J. C., and Zavolan, M. (2016). Patterns of ribosomal protein expression specify normal and malignant human cells. Genome Biol. 17 (1), 236. doi:10.1186/s13059-016-1104-z
Guo, P., Wang, Y., Dai, C., Tao, C., Wu, F., Xie, X., et al. (2018). Ribosomal protein S15a promotes tumor angiogenesis via enhancing Wnt/β-catenin-induced FGF18 expression in hepatocellular carcinoma. Oncogene 37 (9), 1220–1236. doi:10.1038/s41388-017-0017-y
Gupta, V., and Warner, J. R. (2014). Ribosome-omics of the human ribosome. RNA 20 (7), 1004–1013. doi:10.1261/rna.043653.113
Horos, R., Ijspeert, H., Pospisilova, D., Sendtner, R., Andrieu-Soler, C., Taskesen, E., et al. (2012). Ribosomal deficiencies in Diamond-Blackfan anemia impair translation of transcripts essential for differentiation of murine and human erythroblasts. Blood 119 (1), 262–272. doi:10.1182/blood-2011-06-358200
Horos, R., and von Lindern, M. (2012). Molecular mechanisms of pathology and treatment in Diamond blackfan anaemia. Br. J. Haematol. 159 (5), 514–527. doi:10.1111/bjh.12058
Jiang, L., Li, T., Zhang, X., Zhang, B., Yu, C., Li, Y., et al. (2017). RPL10L is required for male meiotic division by compensating for RPL10 during meiotic sex chromosome inactivation in mice. Curr. Biol. 27 (10), 1498–1505. doi:10.1016/j.cub.2017.04.017
Johnson, A. G., Lapointe, C. P., Wang, J., Corsepius, N. C., Choi, J., Fuchs, G., et al. (2019). RACK1 on and off the ribosome. RNA 25 (7), 881–895. doi:10.1261/rna.071217.119
Kang, J., Brajanovski, N., Chan, K. T., Xuan, J., Pearson, R. B., and Sanij, E. (2021). Ribosomal proteins and human diseases: molecular mechanisms and targeted therapy. Signal Transduct. Target Ther. 6 (1), 323. doi:10.1038/s41392-021-00728-8
Kong, Q., Gao, L., Niu, Y., Gongpan, P., Xu, Y., Li, Y., et al. (2016). RACK1 is required for adipogenesis. Am. J. Physiol. Cell Physiol. 311 (5), C831–C836. doi:10.1152/ajpcell.00224.2016
Lesuisse, C., and Martin, L. J. (2002). Long-term culture of mouse cortical neurons as a model for neuronal development, aging, and death. J. Neurobiol. 51 (1), 9–23. doi:10.1002/neu.10037
Li, H., Huo, Y., He, X., Yao, L., Zhang, H., Cui, Y., et al. (2022). A male germ-cell-specific ribosome controls male fertility. Nature 612 (7941), 725–731. doi:10.1038/s41586-022-05508-0
Li, J. J., and Xie, D. (2015). RACK1, a versatile hub in cancer. Oncogene 34 (15), 1890–1898. doi:10.1038/onc.2014.127
Lin, H., Wang, L., Liu, Z., Long, K., Kong, M., Ye, D., et al. (2022). Hepatic MDM2 causes metabolic associated fatty liver disease by blocking triglyceride-VLDL secretion via ApoB degradation. Adv. Sci. (Weinh) 9 (20), e2200742. doi:10.1002/advs.202200742
Liu, Y., Deisenroth, C., and Zhang, Y. (2016). RP-MDM2-p53 pathway: linking ribosomal biogenesis and tumor surveillance. Trends Cancer 2 (4), 191–204. doi:10.1016/j.trecan.2016.03.002
Ma, R. Y., Black, A., and Qian, B. Z. (2022). Macrophage diversity in cancer revisited in the era of single-cell omics. Trends Immunol. 43 (7), 546–563. doi:10.1016/j.it.2022.04.008
Majzoub, K., Hafirassou, M. L., Meignin, C., Goto, A., Marzi, S., Fedorova, A., et al. (2014). RACK1 controls IRES-mediated translation of viruses. Cell 159 (5), 1086–1095. doi:10.1016/j.cell.2014.10.041
Massaad, C. A., and Klann, E. (2011). Reactive oxygen species in the regulation of synaptic plasticity and memory. Antioxid. Redox Signal 14 (10), 2013–2054. doi:10.1089/ars.2010.3208
Metsalu, T., and Vilo, J. (2015). ClustVis: a web tool for visualizing clustering of multivariate data using Principal Component Analysis and heatmap. Nucleic Acids Res. 43 (W1), W566–W570. doi:10.1093/nar/gkv468
Naik, S. K., Lam, E. W., Parija, M., Prakash, S., Jiramongkol, Y., Adhya, A. K., et al. (2020). NEDDylation negatively regulates ERRβ expression to promote breast cancer tumorigenesis and progression. Cell Death Dis. 11 (8), 703. doi:10.1038/s41419-020-02838-7
Nguyen Van Long, F., Lardy-Cleaud, A., Carene, D., Rossoni, C., Catez, F., Rollet, P., et al. (2022). Low level of Fibrillarin, a ribosome biogenesis factor, is a new independent marker of poor outcome in breast cancer. BMC Cancer 22 (1), 526. doi:10.1186/s12885-022-09552-x
Ni, X., Tan, Z., Ding, C., Zhang, C., Song, L., Yang, S., et al. (2019). A region-resolved mucosa proteome of the human stomach. Nat. Commun. 10 (1), 39. doi:10.1038/s41467-018-07960-x
Norris, K., Hopes, T., and Aspden, J. L. (2021). Ribosome heterogeneity and specialization in development. Wiley Interdiscip. Rev. RNA 12 (4), e1644. doi:10.1002/wrna.1644
Orsolic, I., Bursac, S., Jurada, D., Drmic Hofman, I., Dembic, Z., Bartek, J., et al. (2020). Cancer-associated mutations in the ribosomal protein L5 gene dysregulate the HDM2/p53-mediated ribosome biogenesis checkpoint. Oncogene 39 (17), 3443–3457. doi:10.1038/s41388-020-1231-6
Oswald, M. C., Brooks, P. S., Zwart, M. F., Mukherjee, A., West, R. J., Giachello, C. N., et al. (2018a). Reactive oxygen species regulate activity-dependent neuronal plasticity in Drosophila. Elife 7, e39393. doi:10.7554/eLife.39393
Oswald, M. C. W., Garnham, N., Sweeney, S. T., and Landgraf, M. (2018b). Regulation of neuronal development and function by ROS. FEBS Lett. 592 (5), 679–691. doi:10.1002/1873-3468.12972
Rao, S., Lee, S. Y., Gutierrez, A., Perrigoue, J., Thapa, R. J., Tu, Z., et al. (2012). Inactivation of ribosomal protein L22 promotes transformation by induction of the stemness factor, Lin28B. Blood 120 (18), 3764–3773. doi:10.1182/blood-2012-03-415349
Rio, S., Gastou, M., Karboul, N., Derman, R., Suriyun, T., Manceau, H., et al. (2019). Regulation of globin-heme balance in Diamond-Blackfan anemia by HSP70/GATA1. Blood 133 (12), 1358–1370. doi:10.1182/blood-2018-09-875674
Senturk, E., and Manfredi, J. J. (2012). Mdm2 and tumorigenesis: evolving theories and unsolved mysteries. Genes Cancer 3 (3-4), 192–198. doi:10.1177/1947601912457368
Sharma, K., Schmitt, S., Bergner, C. G., Tyanova, S., Kannaiyan, N., Manrique-Hoyos, N., et al. (2015). Cell type- and brain region-resolved mouse brain proteome. Nat. Neurosci. 18 (12), 1819–1831. doi:10.1038/nn.4160
Shi, Z., Fujii, K., Kovary, K. M., Genuth, N. R., Rost, H. L., Teruel, M. N., et al. (2017). Heterogeneous ribosomes preferentially translate distinct subpools of mRNAs genome-wide. Mol. Cell 67 (1), 71–83. doi:10.1016/j.molcel.2017.05.021
Song, P., Yang, F., Jin, H., and Wang, X. (2021). The regulation of protein translation and its implications for cancer. Signal Transduct. Target Ther. 6 (1), 68. doi:10.1038/s41392-020-00444-9
Sugihara, Y., Honda, H., Iida, T., Morinaga, T., Hino, S., Okajima, T., et al. (2010). Proteomic analysis of rodent ribosomes revealed heterogeneity including ribosomal proteins L10-like, L22-like 1, and L39-like. J. Proteome Res. 9 (3), 1351–1366. doi:10.1021/pr9008964
Sundqvist, A., Liu, G., Mirsaliotis, A., and Xirodimas, D. P. (2009). Regulation of nucleolar signalling to p53 through NEDDylation of L11. EMBO Rep. 10 (10), 1132–1139. doi:10.1038/embor.2009.178
Thompson, M. K., Rojas-Duran, M. F., Gangaramani, P., and Gilbert, W. V. (2016). The ribosomal protein Asc1/RACK1 is required for efficient translation of short mRNAs. Elife 5, e11154. doi:10.7554/eLife.11154
Tu, C., Meng, L., Nie, H., Yuan, S., Wang, W., Du, J., et al. (2020). A homozygous RPL10L missense mutation associated with male factor infertility and severe oligozoospermia. Fertil. Steril. 113 (3), 561–568. doi:10.1016/j.fertnstert.2019.10.029
Wang, H., Xiao, W., Zhou, Q., Chen, Y., Yang, S., Sheng, J., et al. (2009). Bystin-like protein is upregulated in hepatocellular carcinoma and required for nucleologenesis in cancer cell proliferation. Cell Res. 19 (10), 1150–1164. doi:10.1038/cr.2009.99
Wilson, C., Munoz-Palma, E., and Gonzalez-Billault, C. (2018). From birth to death: a role for reactive oxygen species in neuronal development. Semin. Cell Dev. Biol. 80, 43–49. doi:10.1016/j.semcdb.2017.09.012
Woo, J., Clair, G. C., Williams, S. M., Feng, S., Tsai, C. F., Moore, R. J., et al. (2022). Three-dimensional feature matching improves coverage for single-cell proteomics based on ion mobility filtering. Cell Syst. 13 (5), 426–434.e4. doi:10.1016/j.cels.2022.02.003
Yao, Y., Liu, Y., Lv, X., Dong, B., Wang, F., Li, J., et al. (2016). Down-regulation of ribosomal protein S15A inhibits proliferation of human glioblastoma cells in vivo and in vitro via AKT pathway. Tumour Biol. 37 (4), 4979–4990. doi:10.1007/s13277-015-4323-0
Zhang, L., and Wang, C. C. (2014). Inflammatory response of macrophages in infection. Hepatobiliary Pancreat. Dis. Int. 13 (2), 138–152. doi:10.1016/s1499-3872(14)60024-2
Zhao, X., Shen, L., Feng, Y., Yu, H., Wu, X., Chang, J., et al. (2015). Decreased expression of RPS15A suppresses proliferation of lung cancer cells. Tumour Biol. 36 (9), 6733–6740. doi:10.1007/s13277-015-3371-9
Zhou, L., Jiang, Y., Luo, Q., Li, L., and Jia, L. (2019). Neddylation: a novel modulator of the tumor microenvironment. Mol. Cancer 18 (1), 77. doi:10.1186/s12943-019-0979-1
Zhou, X., Moon, C., Zheng, F., Luo, Y., Soellner, D., Nunez, J. L., et al. (2009). N-methyl-D-aspartate-stimulated ERK1/2 signaling and the transcriptional up-regulation of plasticity-related genes are developmentally regulated following in vitro neuronal maturation. J. Neurosci. Res. 87 (12), 2632–2644. doi:10.1002/jnr.22103
Keywords: bioinformatics, cancer, development, macrophage, ribosome heterogeneity, stoichiometry
Citation: Gao Y and Wang H (2024) Ribosome heterogeneity in development and disease. Front. Cell Dev. Biol. 12:1414269. doi: 10.3389/fcell.2024.1414269
Received: 08 April 2024; Accepted: 24 June 2024;
Published: 17 July 2024.
Edited by:
Zemfira N. Karamysheva, Texas Tech University Health Sciences Center, United StatesReviewed by:
Kamil Filipek, University of Bologna, ItalyFederico Martinez-Seidel, Max Planck Institute of Molecular Plant Physiology, Germany
Copyright © 2024 Gao and Wang. This is an open-access article distributed under the terms of the Creative Commons Attribution License (CC BY). The use, distribution or reproduction in other forums is permitted, provided the original author(s) and the copyright owner(s) are credited and that the original publication in this journal is cited, in accordance with accepted academic practice. No use, distribution or reproduction is permitted which does not comply with these terms.
*Correspondence: Hongbing Wang, wangho@msu.edu