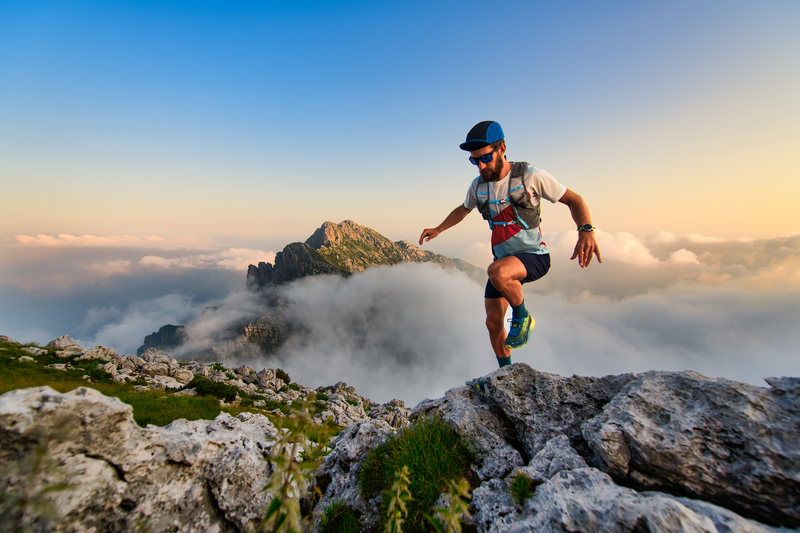
95% of researchers rate our articles as excellent or good
Learn more about the work of our research integrity team to safeguard the quality of each article we publish.
Find out more
ORIGINAL RESEARCH article
Front. Cell Dev. Biol. , 30 November 2023
Sec. Molecular and Cellular Pathology
Volume 11 - 2023 | https://doi.org/10.3389/fcell.2023.1247067
This article is part of the Research Topic Modelling Neurodevelopmental and Neurodegenerative Diseases for Prognosis, Diagnosis, and Therapies View all 7 articles
Introduction: Both low bone mineral density (BMD) and Alzheimer’s disease (AD) commonly co_occur in the older adult. Until now, the association between AD and BMD has been widely reported by observational studies. However, Mendelian randomization (MR) studies did not support the causal association between BMD and AD. We think that the lack of significant causal association between AD and BMD identified by recent MR studies may be caused by small number of potential instrumental variables.
Methods: We conduct a MR study to evaluate the causal effect of heel BMD on the risk of AD using 1,362 genome-wide significant and independent (p < 5.00E-08) heel BMD genetic variants as the potential instrumental variables, which are identified by a large-scale genome wide association study (GWAS) of heel BMD in 394,929 UK Biobank individuals. Using these 1,362 genome-wide significant and independent heel BMD genetic variants, we extracted their corresponding AD GWAS summary results in IGAP AD GWAS dataset (n = 63,926) and FinnGen AD GWAS dataset (n = 377,277). Five methods including inverse-variance weighted meta-analysis (IVW), weighted median, MR-Egger, MR-PRESSO, and MRlap were selected to perform the MR analysis. 951 of these 1,362 genetic variants are available in AD GWAS dataset.
Results: We observed statistically significant causal effect of heel BMD on the risk of AD using IVW in IGAP AD GWAS dataset (OR = 1.048, 95%CI: 1.002–1.095, p = 0.04) and FinnGen AD GWAS dataset (OR = 1.053, 95% CI:1.011–1.098, p = 0.011). Importantly, meta-analysis of IVW estimates from IGAP and FinnGen further supported the causal effect of heel BMD on the risk of AD (OR = 1.051, 95% CI: 1.02–1.083, p = 0.0013).
Discussion: Collectively, our current MR study supports heel BMD to be a risk factor of AD by analyzing the large-scale heel BMD and AD GWAS datasets. The potential mechanisms underlying the association between heel BMD and AD should be further evaluated in future.
Both low bone mineral density (BMD) and Alzheimer’s disease (AD) commonly co-occur in the older adult. Until now, the association between AD and BMD has been widely reported. The Rotterdam Study in 3,651 participants showed that participants with lower BMD at the femoral neck were associated with increased risk of all-cause dementia with Hazard ratio (HR) = 1.12, 95% confidence interval (CI): 1.02–1.23, and AD with HR = 1.14, 95% CI: 1.02–1.28 during the whole follow-up (Xiao et al., 2023). During the first 10 years follow-up, the lowest tertiles of femoral neck BMD, total body BMD, and trabecular bone score were associated with increased risk of dementia, with HR = 2.03, 95% CI: 1.39–2.96, HR = 1.42, 95% CI: 1.01–2.02, and HR = 1.59, 95% CI: 1.11–2.28, respectively (Xiao et al., 2023). Evidence from 71 early stage AD patients and 69 non-demented older adult controls showed the reduced BMD in early stage AD, which further contributed to brain atrophy and memory decline (Loskutova et al., 2009). Evidence from 150 AD cases and gender and age-matched 150 healthy controls further showed the reduced BMD in AD (Kumar et al., 2021). Evidence suggested significantly increased bone metabolic biomarkers and reduced BMD in early stage AD by analyzing the data from 42 male early stage AD and 40 age-matched healthy older controls (Pu et al., 2020). A community-based prospective cohort study in 987 participants including 610 women and 377 men showed significant relation between low femoral neck BMD and increased risk of AD (relative risk (RR) = 2.37) and all-cause dementia (RR = 2.24) in women but not in men (Tan et al., 2005). The Framingham Offspring Cohort study in 1905 participants further indicated that the increased femoral neck BMD contributed to better performance and lower white matter hyperintensity burden (Stefanidou et al., 2021).
In addition to these observational studies, several Mendelian randomization (MR) studies have also evaluated the bidirectional causal effect between BMD and AD by analyzing multiple large-scale genome wide association study (GWAS) datasets. Xu and others found no significant causal effect of AD on BMD at different sites including femoral neck, forearm, heel, lumbar spine, and total body, and different ages including 0–15, 15–30, 30–45, 45–60, and over 60 years (Hu et al., 2023). Meanwhile, Xu and others identified no evidence of causal effect of BMD at different sites (femoral neck, forearm, heel, lumbar spine, and total body) and different ages (0–15, 15–30, 30–45, 45–60, and over 60 years) on the risk of AD (Hu et al., 2023). MR findings supported the lack of significant causal effects of AD on BMD at different sites including femoral neck, lumbar spine, and forearm (Cui et al., 2020; Tang et al., 2023). However, Nethander and others found a significant causal effect of AD on hip fracture (OR = 1.07, 95% CI: 1.05–1.10, and p = 1.9 × 10−12, although lack of significant genetic correlation between AD and hip fracture (Nethander et al., 2022).
We think that the lack of significant causal association between AD and BMD identified by recent MR studies may be caused by small number of genetic variants as the potential instrumental variables. For example, Xu and others selected 68, 3, 16, 19 and 296 genetic variants as potential instrumental variables for total body, forearm, femoral neck, lumbar spine, and heel BMD, respectively (Hu et al., 2023). Meanwhile, Xu and others selected 7, 1, 9, 18 and 18 genetic variants as the potential instrumental variables for total body BMD at different ages including 0–15, 15–30, 30–45, 45–60, and over 60 years (Hu et al., 2023). Here, we conduct an updated MR study to evaluate the causal effect of heel BMD on the risk of AD using 1,362 genome-wide significant and independent heel BMD genetic variants (Kim, 2018).
Our current study design is based on a two-sample MR, which estimates the causal effect of an exposure (such as BMD) on an outcome (AD) only using genome-wide significant genetic variants and large-scale GWAS summary statistics. Importantly, two-sample MR must meet the instrumental variable assumptions to ensure the valid causal inference (Davies et al., 2018; de Leeuw et al., 2022; Wootton et al., 2022). First, relevance assumption: genetic variants must be significantly associated with BMD. Second, independence assumption: genetic variants must not be associated with confounders of the BMD-AD. Third, exclusion-restriction assumption: genetic variants must only be associated with the AD via BMD (Davies et al., 2018; de Leeuw et al., 2022; Wootton et al., 2022). Figure 1 provides the flow chart of our current study design.
FIGURE 1. Provides the flow chart of our current study design. (A) provides the instrumental variable assumptions; (B) provides the main analysis flow.
We selected 1,362 genome-wide significant (p < 5.00E-08) and independent (GCTA COJO r2 ≤ 0.1 for clumping) heel BMD genetic variants across 899 loci as the potential instrumental variables, which were identified by a large-scale GWAS of heel BMD (Kim, 2018). This GWAS is performed in UK Biobank participants of European ancestry (Kim, 2018). A total of 394,929 UK Biobank individuals were available for the final GWAS analysis with both genotype and phenotype data (Kim, 2018). Supplementary Table S1 provides the summary statistics for these 1,362 genome-wide significant and independent heel BMD genetic variants. The original study provides more detailed information about these 1,362 genetic variants (Kim, 2018).
In the discovery stage, we selected a large-scale AD GWAS dataset from the International Genomics of Alzheimer’s Project (IGAP) consisting of 21,982 autopsy-confirmed or clinically confirmed late-onset AD and 41,944 cognitively normal controls of European descent (Kunkle et al., 2019). The IGAP AD GWAS dataset is a meta-analysis of four available AD GWAS datasets. The fist AD GWAS dataset is from Alzheimer Disease Genetics Consortium (ADGC) including 14,428 autopsy-confirmed or clinically confirmed late-onset AD and 14,562 cognitively normal controls. The second AD GWAS dataset is from Cohorts for Heart and Aging Research in Genomic Epidemiology Consortium (CHARGE) consisting of 2,137 autopsy-confirmed or clinically confirmed late-onset AD and 13,474 cognitively normal controls. The third AD GWAS dataset is from European Alzheimer’s Disease Initiative (EADI), which includes 2,240 autopsy-confirmed or clinically confirmed late-onset AD and 6,631 cognitively normal controls. The fourth AD GWAS dataset is from Genetic and Environmental Risk in AD/Defining Genetic, Polygenic and Environmental Risk for Alzheimer’s Disease Consortium (GERAD/PERADES) including 3,177 autopsy-confirmed or clinically confirmed late-onset AD and 7,277 cognitively normal controls (Kunkle et al., 2019). Supplementary Table S2 provides a demographic description of the datasets in each consortium.
In the replication stage, we selected a large-scale AD GWAS dataset from FinnGen (release 9) consisting of 13,393 AD with wide definition and 363,884 controls of European descent (G6_AD_WIDE) (Kurki et al., 2023). FinnGen is a large public-private partnership that aims to collect and analyze genome and national health register data from 500,000 Finnish biobank participants (Kurki et al., 2023). The FinnGen AD GWAS summary statistics were downloaded from https://finngen.gitbook.io/documentation/data-download.
We conducted a MR analysis using three methods including inverse-variance weighted meta-analysis (IVW) (Bowden et al., 2016), weighted median (Bowden et al., 2016), and MR-Egger (Burgess and Thompson, 2017). IVW is widely used in MR analysis as the main analysis method, and IVW assumes all the selected genetic variants to be valid instrumental variables (Burgess et al., 2020). However, IVW will be biased even if only one of these selected genetic variants is invalid (Burgess et al., 2020). We selected weighted median, MR-Egger, MR-PRESSO, and MRlap as the sensitivity analysis methods (Verbanck et al., 2018; Burgess et al., 2020; Mounier and Kutalik, 2023). Weighted median assumes that the majority of genetic variants (>50%) are valid instrumental variables or the majority of weight (>50%) is from the valid instrumental variables (Burgess and Thompson, 2017). Compared with IVW and weighted median, MR-Egger not only tests the directional pleiotropy, but also provides a causal estimate by correcting for the pleiotropy (Burgess and Thompson, 2017). MR-PRESSO (Mendelian Randomization Pleiotropy RESidual Sum and Outlier) could evaluate (MR-PRESSO global test) and correct for (MR-PRESSO outlier test) horizontal pleiotropy using GWAS summary association statistics (Verbanck et al., 2018). MRlap could take into account the weak instrument bias and winner’s curse, and correct for potential sample overlap and the bias of IVW utilizing GWAS summary association statistics (Mounier and Kutalik, 2023). Meanwhile, we test statistical heterogeneity across the selected instrumental variables using Cochran’s Q statistic (Rucker et al., 2008). The significant causal effect of heel BMD on the risk of AD is provided by odds ratio (OR) as well as its corresponding 95% CI, which corresponds to 1 standard deviation (SD) in heel BMD levels. p < 0.05 is defined to be the statistical significant. R Version 4.0.3, R package “MendelianRandomization,” and R package “meta” (version 6.0–0) were used to conduct the MR analysis and meta-analysis (Yavorska and Burgess, 2017).
R2 is the proportion of the heel BMD variance that is explained by the instrumental variables, an indicator of power for MR (Burgess and Thompson, 2011; Pierce et al., 2011). F reflects the “strength” of an instrumental variable or a set of instrumental variables (Burgess and Thompson, 2011; Pierce et al., 2011). R2 could be calculated by the formula
Using 1,362 genome-wide significant (p < 5.00E-08) and independent heel BMD genetic variants, we extracted their corresponding GWAS summary statistics in IGAP AD GWAS dataset. 951 of these 1,362 genetic variants are available in AD GWAS dataset, which are provided in Supplementary Table S3. Using these 951 genetic variants as the potential instrumental variables, we observed statistically significant causal effect of heel BMD on AD risk using IVW method (OR = 1.048, 95% CI: 1.002–1.095, p = 0.04). However, weighted median method and MR-Egger method did not indicate any significant association between heel BMD and AD with OR = 0.99, 95% CI: 0.916–1.07, p = 0.797 and OR = 0.987, 95% CI: 0.908–1.073, p = 0.758, respectively. Table 1 provides the MR results about the association between heel BMD and AD. Figure 2 provides the single causal estimates about the effect of heel BMD on the risk of AD in IGAP dataset using IVW.
FIGURE 2. Single estimate about the causal effect of on heel BMD AD in IGAP dataset using IVW. The x-axis shows the single effect from each heel BMD genetic variant as the instrumental variable, and standard error, and the y-axis shows the single effect from each heel BMD genetic variant as the instrumental variable, and standard error on AD in IGAP. IVW, Inverse-variance weighted.
There is clear evidence of heterogeneity across these selected instrumental variables with Cochran’s Q statistic = 1,207.3781, degrees of freedom 950, p < 1.00E-04, and I^2 = 21.3%. MR-Egger intercept test showed no evidence of pleiotropy with intercept = 0.002, and p = 0.094. MR-PRESSO global test showed evidence of horizontal pleiotropy with p < 5.00E-04. MR-PRESSO outlier test and MRlap found suggestive and lack of significant association between genetically increased heel BMD and increased risk of AD with OR = 1.038, 95% CI: 0.994–1.084, p = 0.089 and OR = 1.011, 95% CI: 0.992–1.032, p = 0.262, respectively. Interestingly, MR estimates from MR-PRESSO outlier and MRlap are consistent in direction.
Using 1,362 genome-wide significant (p < 5.00E-08) and independent heel BMD genetic variants, we extracted their corresponding GWAS summary statistics in FinnGen AD GWAS dataset. 1,037 of these 1,362 genetic variants are available in FinnGen AD GWAS dataset, which are provided in Supplementary Table S4. Using these 1,103 genetic variants as the potential instrumental variables, we still observed statistically significant causal effect of heel BMD on AD risk using IVW method (OR = 1.053, 95% CI: 1.011–1.098, p = 0.011). Interestingly, both weighted median method (OR = 0.99, 95% CI: 0.916–1.07, p = 0.797) and MR-Egger method (OR = 0.99, 95% CI: 0.916–1.07, p = 0.797) suggested the same directions about the causal effect of heel BMD on AD risk, although lack of statistical significance. Table 1 provides the MR results about the association between heel BMD and AD. Figure 3 provides the single causal estimates about the effect of heel BMD on the risk of AD in FinnGen dataset using IVW.
FIGURE 3. Single estimate about the causal effect of on heel BMD AD in FinnGen dataset using IVW. The x-axis shows the single effect from each heel BMD genetic variant as the instrumental variable, and standard error, and the y-axis shows the single effect from each heel BMD genetic variant as the instrumental variable, and standard error on AD in FinnGen. IVW, Inverse-variance weighted.
There is clear evidence of heterogeneity across these selected instrumental variables with Cochran’s Q statistic = 1,338.1074, degrees of freedom 1,036, p < 1.00E-04, and I^2 = 22.6%. MR-Egger intercept test showed no evidence of pleiotropy with intercept = 0, and p = 0.761. MR-PRESSO global test showed evidence of horizontal pleiotropy with p < 5.00E-04. MR-PRESSO outlier test and MRlap found significant and lack of significant association between genetically increased heel BMD and increased risk of AD with OR = 1.042, 95% CI: 1.003–1.084, p = 0.037 and OR = 1.131, 95% CI: 0.415–3.079, p = 0.414, respectively. Interestingly, MR estimates from MR-PRESSO outlier and MRlap are also consistent in direction.
Collectively, IVW, MR-PRESSO, and MRlap identified consistent findings in direction about the causal effect of heel BMD on AD risk. We further performed a meta-analysis of IVW, MR-PRESSO, and MRlap estimates from IGAP and FinnGen datasets. Interestingly, meta-analysis of IVW estimates further supported the causal effect of genetically increased heel BMD on the risk of AD using both fixed effect model (OR = 1.051, 95% CI: 1.02–1.083, p = 0.0013) and random effects model (OR = 1.051, 95% CI: 1.02–1.083, p = 0.0013). Meanwhile, MR estimates from the meta-analysis of MR-PRESSO and MRlap estimates are consistent with MR estimate from the meta-analysis of IVW estimates, as provided in Table 1. Importantly, there is no evidence of heterogeneity across the IVW estimates (Q = 0.03, p = 0.8725, and I^2 = 0.0%), MR-PRESSO estimates (Q = 0.02, p = 0.8953, and I^2 = 0.0%), and MRlap estimate (Q = 0.05, p = 0.8270, and I^2 = 0.0%).
The sample size for heel BMD GWAS dataset is 394,929 (Kim, 2018). R2, the proportion of the heel BMD variance explained by these 1,362 instrumental variables, is 44.74%, as provided in Supplementary Table S1. F is 233.998, which shows that there is a strong correlation between these 1,362 instrumental variables and heel BMD, and avoids the potential bias from weak instruments in MR studies.
In this current study, we performed an updated MR analysis to determine the causal effect of heel BMD on the risk of AD by selecting 1,362 genome-wide significant (p < 5.00E-08) and independent heel BMD genetic variants as the potential instrumental variables (n = 394,929), and extracted the corresponding AD GWAS summary statistics in IGAP AD GWAS dataset (n = 63,926) and FinnGen AD GWAS dataset (n = 377,277). We found a statistically significant causal effect between increased heel BMD and increased risk of AD in both IGAP (OR = 1.048, 95% CI: 1.002–1.095, p = 0.04) and FinnGen (OR = 1.053, 95% CI: 1.011–1.098, p = 0.011). Importantly, meta-analysis of IVW estimates from IGAP and FinnGen further supported the causal effect of heel BMD on the risk of AD (OR = 1.051, 95% CI: 1.02–1.083, p = 0.0013).
It is known that calcium and vitamin D associates with BMD/fracture, although lack of sufficient clinical evidence to support the clear benefits and harms of alone or combined vitamin D and calcium supplementation to prevent the fractures in community-dwelling adults (Tai et al., 2015; Zhao et al., 2017; Grossman et al., 2018; Jin, 2018). In addition to BMD/fracture, MR studies have also evaluated the casual effect of calcium and vitamin D on the risk of AD. He and others selected 8 genome-wide significant and independent serum calcium genetic variants as the instrumental variables, and found that per 1 standard deviation increase in serum calcium level (about 0.5 mg/dL) contribute to a reduced risk of AD using IVW (OR = 0.57) (He et al., 2020). Shi and others further conducted an updated MR analysis in both discovery stage and replication stage (Shi et al., 2021). In the discovery stage, 14 independent but not genome-wide significant serum calcium genetic variants were selected as the instrumental variables (Shi et al., 2021). In the replication stage, 166 genome-wide significant and independent serum calcium genetic variants were selected as the instrumental variables (Shi et al., 2021). They identified the reduced trend of risk of AD as the serum calcium level increased (Shi et al., 2021). Meanwhile, several studies found reduced risk of AD as the 25OHD level increased (Larsson et al., 2017; Larsson et al., 2018; Wang et al., 2020).
In addition to AD, recent MR studies have also investigated the causal association between BMD and other neurological disease. Cui and others evaluated the causal effects of schizophrenia and bipolar disorder on BMD at different sites including femoral neck, lumbar spine, and forearm (Cui et al., 2020). However, they identified lack of significant causal effect of schizophrenia and bipolar disorder on BMD (Cui et al., 2020). Tang and others found no significant causal effect of schizophrenia, depression, Parkinson’s disease, and epilepsy on BMD at different sites including heel, forearm, lumbar spine, femoral neck, and total body, as well as fractures including leg fracture, arm fracture, heel fracture, spine fracture and osteoporotic fracture (Tang et al., 2023). Yao and others aimed to investigate the bidirectional causal association between BMD/fracture and the risk of MS using large-scale BMD, fracture, and MS GWAS datasets (Yao et al., 2022). However, they only highlighted significant association between fracture and reduced risk of MS (β = −0.375, p = 0.002) (Yao et al., 2022).
Compared with previous MR studies, our current MR analysis may increase the number of BMD genetic variants from 296 to 1,362 (Hu et al., 2023). Using 296 heel BMD genetic variants to be the potential instrumental variables, Xu and others found no statistically significant association between heel BMD and the risk of AD with OR = 0.991, 95% CI: 0.925–1.061, p = 0.794 (Hu et al., 2023). These findings show that the increase in the number of potential instrumental variables may contribute to identify more significant causal association.
However, some limitations still exist in our current MR study. The original GWAS identified 1,362 genome-wide significant and independent heel BMD genetic variants (Kim, 2018). However, only 951 and 1,037 genetic variants are available in IGAP and FinnGen AD GWAS dataset, which are provided in Supplementary Tables S3, S4. These missing genetic variants may influence our current MR results. Our current MR findings should be further verified by adding more heel BMD genetic variants as potential instrumental variables. Second, we only evaluated the effect of heel BMD on the risk of AD. It remains unclear about the effect of AD on the heel BMD, which deserves further investigation by additional studies. Third, we only focus on the heel BMD as the largest number of heel BMD genetic variants (N = 1,362) (Kim, 2018), compared to femoral neck (N = 16), lumbar spine (N = 19), total body (N = 68) and heel (N = 296) (Hu et al., 2023). Fourth, we did not perform the manual analysis to investigate the potential association of these heel BMD genetic variants with confounders for BMD-AD using Phenoscanner V2, a database of human genotype-phenotype associations (Kamat et al., 2019). We think that this kind of manual analysis only evaluate the known pleiotropy such as calcium and vitamin D levels, but not the unknown pleiotropy. Importantly, Phenoscanner V2 limits the largest number of input either 100 SNPs, 10 genes or 10 genomic regions (Kamat et al., 2019). Therefore, we test the pleiotropy using four statistical methods including MR-Egger intercept test, Cochran’s Q statistic, MR-PRESSO global test, and MRlap.
In summary, our current MR study supports heel BMD to be a risk factor of AD using large-scale GWAS datasets. The potential mechanisms underlying this association should be further evaluated in future.
The original contributions presented in the study are included in the article/Supplementary Material, further inquiries can be directed to the corresponding author.
Ethical approval was not required for the study involving humans in accordance with the local legislation and institutional requirements. Written informed consent to participate in this study was not required from the participants or the participants’ legal guardians/next of kin in accordance with the national legislation and the institutional requirements.
FG and HP designed this study, analyzed the data. All authors contributed to the article and approved the submitted version.
We thank the IGAP, FinnGen, and UK Biobank for providing us the AD and heel BMD GWAS datasets. We want to acknowledge the participants and investigators of the FinnGen study.
The authors declare that the research was conducted in the absence of any commercial or financial relationships that could be construed as a potential conflict of interest.
All claims expressed in this article are solely those of the authors and do not necessarily represent those of their affiliated organizations, or those of the publisher, the editors and the reviewers. Any product that may be evaluated in this article, or claim that may be made by its manufacturer, is not guaranteed or endorsed by the publisher.
The Supplementary Material for this article can be found online at: https://www.frontiersin.org/articles/10.3389/fcell.2023.1247067/full#supplementary-material
Bowden, J., Davey Smith, G., Haycock, P. C., and Burgess, S. (2016). Consistent estimation in mendelian randomization with some invalid instruments using a weighted median estimator. Genet. Epidemiol. 40, 304–314. doi:10.1002/gepi.21965
Burgess, S., Foley, C. N., Allara, E., Staley, J. R., and Howson, J. M. M. (2020). A robust and efficient method for Mendelian randomization with hundreds of genetic variants. Nat. Commun. 11, 376. doi:10.1038/s41467-019-14156-4
Burgess, S., and Thompson, S. G. (2011). Avoiding bias from weak instruments in Mendelian randomization studies. Int. J. Epidemiol. 40, 755–764. doi:10.1093/ije/dyr036
Burgess, S., and Thompson, S. G. (2017). Interpreting findings from Mendelian randomization using the MR-Egger method. Eur. J. Epidemiol. 32, 377–389. doi:10.1007/s10654-017-0255-x
Cui, Z., Meng, X., Zhuang, S., Liu, Z., Zhou, F., and Tian, Y. (2020). Schizophrenia, bipolar disorder, and alzheimer's disease are not causal factors of bone mineral density: a mendelian randomization analysis. Calcif. Tissue Int. 106, 131–146. doi:10.1007/s00223-019-00625-x
Davies, N. M., Holmes, M. V., and Davey Smith, G. (2018). Reading Mendelian randomisation studies: a guide, glossary, and checklist for clinicians. BMJ 362, k601. doi:10.1136/bmj.k601
De Leeuw, C., Savage, J., Bucur, I. G., Heskes, T., and Posthuma, D. (2022). Understanding the assumptions underlying Mendelian randomization. Eur. J. Hum. Genet. 30, 653–660. doi:10.1038/s41431-022-01038-5
Grossman, D. C., Curry, S. J., Owens, D. K., Barry, M. J., Caughey, A. B., Davidson, K. W., et al. (2018). Vitamin D, calcium, or combined supplementation for the primary prevention of fractures in community-dwelling adults: US preventive services task force recommendation statement. JAMA 319, 1592–1599. doi:10.1001/jama.2018.3185
He, Y., Zhang, H., Wang, T., Han, Z., Ni, Q. B., Wang, K., et al. (2020). Impact of serum calcium levels on alzheimer's disease: a mendelian randomization study. J. Alzheimers Dis. 76, 713–724. doi:10.3233/JAD-191249
Hu, H., Mei, J., Cai, Y., Ding, H., Niu, S., Zhang, W., et al. (2023). No genetic causal association between Alzheimer's disease and osteoporosis: a bidirectional two-sample Mendelian randomization study. Front. Aging Neurosci. 15, 1090223. doi:10.3389/fnagi.2023.1090223
Jin, J. (2018). Vitamin D and calcium supplements for preventing fractures. JAMA 319, 1630. doi:10.1001/jama.2018.3892
Kamat, M. A., Blackshaw, J. A., Young, R., Surendran, P., Burgess, S., Danesh, J., et al. (2019). PhenoScanner V2: an expanded tool for searching human genotype-phenotype associations. Bioinformatics 35, 4851–4853. doi:10.1093/bioinformatics/btz469
Kim, S. K. (2018). Identification of 613 new loci associated with heel bone mineral density and a polygenic risk score for bone mineral density, osteoporosis and fracture. PLoS One 13, e0200785. doi:10.1371/journal.pone.0200785
Kumar, S., Chandnani, A., Aung, N. H., Shahid, S., Bukhari, D., Shahzad, S., et al. (2021). Alzheimer's disease and its association with bone health: a case-control study. Cureus 13, e13772. doi:10.7759/cureus.13772
Kunkle, B. W., Grenier-Boley, B., Sims, R., Bis, J. C., Damotte, V., Naj, A. C., et al. (2019). Genetic meta-analysis of diagnosed Alzheimer's disease identifies new risk loci and implicates Aβ, tau, immunity and lipid processing. Nat. Genet. 51, 414–430. doi:10.1038/s41588-019-0358-2
Kurki, M. I., Karjalainen, J., Palta, P., Sipila, T. P., Kristiansson, K., Donner, K. M., et al. (2023). FinnGen provides genetic insights from a well-phenotyped isolated population. Nature 613, 508–518. doi:10.1038/s41586-022-05473-8
Larsson, S. C., Traylor, M., Malik, R., Dichgans, M., Burgess, S., Markus, H. S., et al. (2017). Modifiable pathways in Alzheimer's disease: Mendelian randomisation analysis. BMJ 359, j5375. doi:10.1136/bmj.j5375
Larsson, S. C., Traylor, M., Markus, H. S., and Michaelsson, K. (2018). Serum parathyroid hormone, 25-hydroxyvitamin D, and risk of alzheimer's disease: a mendelian randomization study. Nutrients 10, 1243. doi:10.3390/nu10091243
Locke, A. E., Kahali, B., Berndt, S. I., Justice, A. E., Pers, T. H., Day, F. R., et al. (2015). Genetic studies of body mass index yield new insights for obesity biology. Nature 518, 197–206. doi:10.1038/nature14177
Loskutova, N., Honea, R. A., Vidoni, E. D., Brooks, W. M., and Burns, J. M. (2009). Bone density and brain atrophy in early Alzheimer's disease. J. Alzheimers Dis. 18, 777–785. doi:10.3233/JAD-2009-1185
Mounier, N., and Kutalik, Z. (2023). Bias correction for inverse variance weighting Mendelian randomization. Genet. Epidemiol. 47, 314–331. doi:10.1002/gepi.22522
Nethander, M., Coward, E., Reimann, E., Grahnemo, L., Gabrielsen, M. E., Wibom, C., et al. (2022). Assessment of the genetic and clinical determinants of hip fracture risk: genome-wide association and Mendelian randomization study. Cell Rep. Med. 3, 100776. doi:10.1016/j.xcrm.2022.100776
Pierce, B. L., Ahsan, H., and Vanderweele, T. J. (2011). Power and instrument strength requirements for Mendelian randomization studies using multiple genetic variants. Int. J. Epidemiol. 40, 740–752. doi:10.1093/ije/dyq151
Pu, Z., Tang, X., Fei, Y., Hou, Q., Lin, Y., and Zha, X. (2020). Bone metabolic biomarkers and bone mineral density in male patients with early-stage Alzheimer's disease. Eur. Geriatr. Med. 11, 403–408. doi:10.1007/s41999-020-00289-z
Rucker, G., Schwarzer, G., Carpenter, J. R., and Schumacher, M. (2008). Undue reliance on I(2) in assessing heterogeneity may mislead. BMC Med. Res. Methodol. 8, 79. doi:10.1186/1471-2288-8-79
Shi, Y., Liu, R., Guo, Y., Li, Q., Zhou, H., Yu, S., et al. (2021). An updated mendelian randomization analysis of the association between serum calcium levels and the risk of alzheimer's disease. Front. Genet. 12, 731391. doi:10.3389/fgene.2021.731391
Stefanidou, M., O'donnell, A., Himali, J. J., Decarli, C., Satizabal, C., Beiser, A. S., et al. (2021). Bone mineral density measurements and association with brain structure and cognitive function: the framingham offspring cohort. Alzheimer Dis. Assoc. Disord. 35, 291–297. doi:10.1097/WAD.0000000000000453
Tai, V., Leung, W., Grey, A., Reid, I. R., and Bolland, M. J. (2015). Calcium intake and bone mineral density: systematic review and meta-analysis. BMJ 351, h4183. doi:10.1136/bmj.h4183
Tan, Z. S., Seshadri, S., Beiser, A., Zhang, Y., Felson, D., Hannan, M. T., et al. (2005). Bone mineral density and the risk of Alzheimer disease. Arch. Neurol. 62, 107–111. doi:10.1001/archneur.62.1.107
Tang, F., Wang, S., Zhao, H., Xia, D., and Dong, X. (2023). Mendelian randomization analysis does not reveal a causal influence of mental diseases on osteoporosis. Front. Endocrinol. (Lausanne) 14, 1125427. doi:10.3389/fendo.2023.1125427
Verbanck, M., Chen, C. Y., Neale, B., and Do, R. (2018). Detection of widespread horizontal pleiotropy in causal relationships inferred from Mendelian randomization between complex traits and diseases. Nat. Genet. 50, 693–698. doi:10.1038/s41588-018-0099-7
Wang, L., Qiao, Y., Zhang, H., Zhang, Y., Hua, J., Jin, S., et al. (2020). Circulating vitamin D levels and alzheimer's disease: a mendelian randomization study in the IGAP and UK biobank. J. Alzheimers Dis. 73, 609–618. doi:10.3233/JAD-190713
Wootton, R. E., Jones, H. J., and Sallis, H. M. (2022). Mendelian randomisation for psychiatry: how does it work, and what can it tell us? Mol. Psychiatry 27, 53–57. doi:10.1038/s41380-021-01173-3
Xiao, T., Ghatan, S., Mooldijk, S. S., Trajanoska, K., Oei, L., Gomez, M. M., et al. (2023). Association of bone mineral density and dementia: the rotterdam study. Neurology 100, e2125–e2133. doi:10.1212/WNL.0000000000207220
Yao, Y., Gao, F., Wu, Y., Zhang, X., Xu, J., Du, H., et al. (2022). Mendelian randomization analysis of the causal association of bone mineral density and fracture with multiple sclerosis. Front. Neurol. 13, 993150. doi:10.3389/fneur.2022.993150
Yavorska, O. O., and Burgess, S. (2017). MendelianRandomization: an R package for performing Mendelian randomization analyses using summarized data. Int. J. Epidemiol. 46, 1734–1739. doi:10.1093/ije/dyx034
Keywords: Mendelian randomization, Alzheimer’s disease, bone mineral density, genome-wide association studies, inverse-variance weighted meta-analysis
Citation: Gao F, Pan R, Fan T, Liu L and Pan H (2023) Identification of heel bone mineral density as a risk factor of Alzheimer’s disease by analyzing large-scale genome-wide association studies datasets. Front. Cell Dev. Biol. 11:1247067. doi: 10.3389/fcell.2023.1247067
Received: 25 June 2023; Accepted: 06 November 2023;
Published: 30 November 2023.
Edited by:
Suk Ling Ma, The Chinese University of Hong Kong, ChinaReviewed by:
Borbala Mifsud, Hamad Bin Khalifa University, QatarCopyright © 2023 Gao, Pan, Fan, Liu and Pan. This is an open-access article distributed under the terms of the Creative Commons Attribution License (CC BY). The use, distribution or reproduction in other forums is permitted, provided the original author(s) and the copyright owner(s) are credited and that the original publication in this journal is cited, in accordance with accepted academic practice. No use, distribution or reproduction is permitted which does not comply with these terms.
*Correspondence: Haile Pan, cGFuaGFpbGUyMDAyQDE2My5jb20=
Disclaimer: All claims expressed in this article are solely those of the authors and do not necessarily represent those of their affiliated organizations, or those of the publisher, the editors and the reviewers. Any product that may be evaluated in this article or claim that may be made by its manufacturer is not guaranteed or endorsed by the publisher.
Research integrity at Frontiers
Learn more about the work of our research integrity team to safeguard the quality of each article we publish.