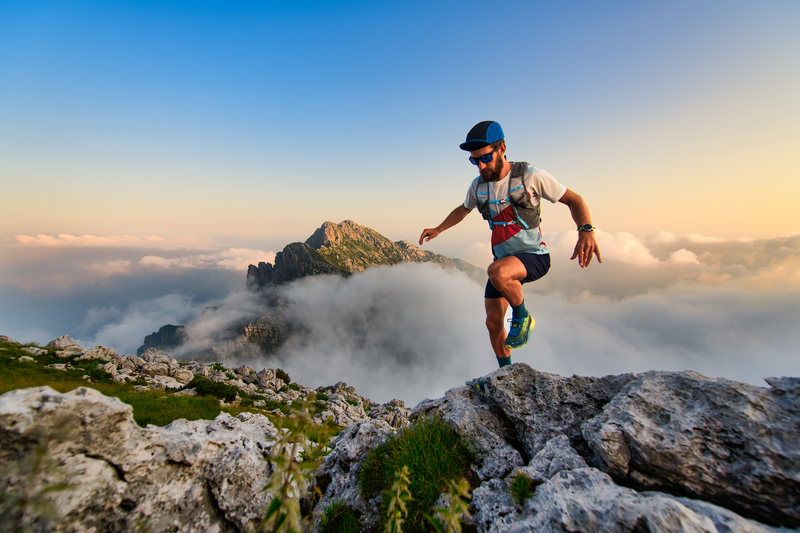
94% of researchers rate our articles as excellent or good
Learn more about the work of our research integrity team to safeguard the quality of each article we publish.
Find out more
ORIGINAL RESEARCH article
Front. Cell Dev. Biol. , 16 August 2023
Sec. Cancer Cell Biology
Volume 11 - 2023 | https://doi.org/10.3389/fcell.2023.1224069
This article is part of the Research Topic Exploring Genomic Instability Of Cancers: Applications In Diagnosis and Treatment View all 5 articles
Background: An increasing number of patients are being diagnosed with lung adenocarcinoma, but there remains limited progress in enhancing prognostic outcomes and improving survival rates for these patients. Genome instability is considered a contributing factor, as it enables other hallmarks of cancer to acquire functional capabilities, thus allowing cancer cells to survive, proliferate, and disseminate. Despite the importance of genome instability in cancer development, few studies have explored the prognostic signature associated with genome instability for lung adenocarcinoma.
Methods: In the study, we randomly divided 397 lung adenocarcinoma patients from The Cancer Genome Atlas database into a training group (n = 199) and a testing group (n = 198). By calculating the cumulative counts of genomic alterations for each patient in the training group, we distinguished the top 25% and bottom 25% of patients. We then compared their gene expressions to identify genome instability-related genes. Next, we used univariate and multivariate Cox regression analyses to identify the prognostic signature. We also performed the Kaplan–Meier survival analysis and the log-rank test to evaluate the performance of the identified prognostic signature. The performance of the signature was further validated in the testing group, in The Cancer Genome Atlas dataset, and in external datasets. We also conducted a time-dependent receiver operating characteristic analysis to compare our signature with established prognostic signatures to demonstrate its potential clinical value.
Results: We identified GULPsig, which includes IGF2BP1, IGF2BP3, SMC1B, CLDN6, and LY6K, as a prognostic signature for lung adenocarcinoma patients from 42 genome instability-related genes. Based on the risk score of the risk model with GULPsig, we successfully stratified the patients into high- and low-risk groups according to the results of the Kaplan–Meier survival analysis and the log-rank test. We further validated the performance of GULPsig as an independent prognostic signature and observed that it outperformed established prognostic signatures.
Conclusion: We provided new insights to explore the clinical application of genome instability and identified GULPsig as a potential prognostic signature for lung adenocarcinoma patients.
Lung cancer is one of the most frequently diagnosed cancer types and the leading cause of cancer-related deaths worldwide (Bray et al., 2018). Lung adenocarcinoma, the most prevalent subtype of lung cancer, accounts for 40% of all lung cancer types and continues to increase (Nagy-Mignotte et al., 2011; Myers and Wallen, 2023). However, there has been limited progress in improving prognostic outcomes and enhancing survival rates for lung adenocarcinoma patients (Travis et al., 2015). Therefore, a prognostic signature for lung adenocarcinoma would be highly valuable for clinical management.
Genome instability, one of the hallmarks of cancer, is regarded as a contributing factor for other hallmarks to acquire functional capabilities that allow cancer cells to survive, proliferate, and disseminate (Hanahan and Weinberg, 2011). Moreover, genome instability has been proved to be a vital prognostic factor associated with cancer progression and survival (Suzuki et al., 2003; Negrini et al., 2010). Signatures related to genome instability have been identified in certain types of cancers. For example, a prognostic signature including 11 genome instability-derived genes was identified for triple-negative breast cancer (Guo and Wang, 2021). A signature composed of five prognostic lncRNAs associated with genome instability was identified in endometrial cancer (Liu et al., 2022). Woo et al. (2021) identified circulating tumor DNA–genomic instability I-scores as a prognostic marker for pancreatic cancer survival, and Sun et al. (2021) found a prognostic signature composed of six lncRNAs associated with genome instability for gastric cancer. However, few studies have explored the association between genome instability and survival prediction in lung adenocarcinoma.
Genome instability is defined as an increased propensity for genomic alterations, including somatic mutations, copy number variations, and fusion genes (Shen, 2011). Patients with various genomic alterations may be affected differently. We assumed that there could be a signal associated with genome instability that plays a role in tumor development and drug resistance and that this potential signal could serve as a prognostic signature to predict the overall survival of lung adenocarcinoma patients. To identify the signature, we proposed comparing the gene expression of patients with various genomic alternations, which can be measured by integrating somatic mutations, copy number variations, and fusion genes.
In the study, we quantified genomic alternations by calculating the cumulative counts of somatic mutations, copy number variations, and fusion genes. We then selected the top 25% of patients as the genomic stable-like (GS) group and the bottom 25% as the genomic unstable-like (GU) group from the ranked cases in ascending order. By comparing the gene expressions between the GS and GU groups, we identified 42 genes associated with genome instability. Furthermore, from these 42 genes, we identified GULPsig, composed of IGF2BP1, IGF2BP3, SMC1B, CLDN6, and LY6K, as a prognostic signature for predicting overall survival. Subsequently, we constructed a risk model with GULPsig and divided the patients into high- and low-risk groups based on the score of the risk model to predict the overall survival of lung adenocarcinoma patients. We further evaluated the independence of GULPsig as a prognostic signature and compared its performance with established prognostic signatures. Overall, our study found GULPsig as an independent prognostic signature for overall survival prediction in clinical management for lung adenocarcinoma patients.
The genomic alterations, gene expression profiles, and corresponding clinical data of lung adenocarcinoma from The Cancer Genome Atlas (TCGA) Pan-Cancer Atlas dataset were obtained from the cBioPortal database (Cerami et al., 2012; Gao et al., 2013). The dataset includes somatic mutations and fusion genes of 566 patients, copy number variations of 511 patients, mRNA expressions of 510 patients, and clinical data of 566 patients. After data integration, data of 397 patients were used to construct and evaluate the prognostic risk model. The gene expression data were batch-normalized from Illumina HiSeq_RNASeq V2. In addition, we collected the expression data and corresponding clinical data of 133 patients in GSE42127 (Hight et al., 2020) and 85 patients in GSE30219 (Rousseaux et al., 2013) from the Gene Expression Omnibus (GEO) database to validate the performance of the risk model. To access the GEO data, we used the R package “GEOquery” (Davis and Meltzer, 2007).
To visualize the frequency of somatic mutations, copy number variations, and fusion genes for each gene in each patient, we developed a binary matrix with genes in rows and patients in columns to represent the genome events independently for the 397 patients in the TCGA dataset. Then, we computed the sum of the three binary matrices to obtain the total genome events for each patient. Based on the results, we sorted the patients and classified the top 25% as the genomic stable-like (GS) group and the bottom 25% as the genomic unstable-like (GU) group.
For the gene expression data, we used a log2 (x + 1)-transformed RSEM normalized count and then performed quantile normalization on the data. Only genes that had 10 counts or more in at least 10 samples were kept for differential expression analysis. The R package “limma” (Ritchie et al., 2015) was used to compare the gene expression between the GU and GS groups. The differentially expressed genes (DEGs) were identified with a Benjamini–Hochberg (BH)-adjusted p-value <0.05 and logFC (fold-change) > 2.
The functions enrichGO and enrichKEGG in the R package “clusterProfiler” (Wu et al., 2021) were applied for enrichment analysis and annotation of the Gene Ontology (GO) and Kyoto Encyclopedia of Genes and Genome (KEGG) pathways for DEGs. We identified the biological properties of the DEGs and visualized the DEG enrichment of molecular function (MF), cellular component (CC), biological process (BP), and KEGG pathways. A BH-adjusted p-value <0.05 was considered statistically significant.
The 397 patients in the TCGA dataset were randomly divided into a training group and a testing group, with a balanced proportion of living and deceased overall survival status. We used the 199 patients in the training group to identify the prognostic signature and construct the risk model. The univariate Cox proportional hazards regression analysis was applied to evaluate the relationship between the expression level of each gene and the overall survival of patients. Only a gene with a p-value of <0.05 was regarded as a statistically significant survival predictor. Next, we applied the multivariate Cox regression model to construct the risk model. To evaluate the risk of each patient, we used the following risk score formula:
where N is the total number of genes, Expr is the normalized gene expression value, and Coef is the estimated regression coefficient value of the gene. We calculated the ORS for each patient with this formula and then sorted them. To divide the patients into high- and low-risk groups based on ORS, we used the function “surv_cutpoint” in the R package “survminer” to determine the optimal cut-off threshold. The Kaplan–Meier (KM) survival analysis and a log-rank test were performed with the R package “survival” and “survminer.” Next, we used the R package “e1071” to train a support vector machine (SVM) classifier using the expression data of selected genes and the identified groups. The classifier’s performance was evaluated with 10-fold cross-validation using the R package “caret.” Similarly, the aforementioned operations were conducted on the 198 patients in the testing group and 397 patients in the TCGA dataset. To further validate the performance of the risk model, we applied the same operations to 133 patients in GSE42127 (Tang et al., 2013; Hight et al., 2020) and 85 patients in GSE30219 (Rousseaux et al., 2013). Additionally, we performed a univariate and multivariate Cox proportional hazards regression, as well as data stratification analysis, to identify the independence of ORS as an independent prognostic indicator.
We applied the TCGA dataset to construct the established benchmarks following the instructions in the respective publications. The R package “survivalROC” was used to conduct a time-dependent receiver operating characteristic (ROC) analysis and calculate the area under the curve (AUC). The performance of the risk model was assessed by measuring the AUC.
A Wilcoxon rank-sum test was performed on the continuous variables, and a chi-square test was performed for categorical data to compare the differences, as appropriate. Statistical significance was defined when p < 0.05. Statistical analysis and visualization were performed with R version 4.2.1.
Figure 1 illustrates the methodology of the study. We involved 397 patients in the TCGA dataset and briefly addressed the clinical characteristics in Table 1. Furthermore, we calculated the cumulative counts of genomic alterations for each patient, consisting of somatic mutations (Figure 2A), copy number variations (Figure 2B), and fusion genes (Figure 2C). We also summarized the genomic alterations and sorted the counts in ascending order. The top 25% of the lung adenocarcinoma patients (n = 99) were defined as the GS group, and the bottom 25% (n = 99) were defined as the GU group. We performed a statistical analysis of the clinical characteristics of the GU and GS groups and found no association (Supplementary Table S1, p > 0.05).
FIGURE 2. Identification of genome instability-related genes in lung adenocarcinoma. (A–C) Boxplots of cumulative counts for somatic mutations, copy number variations, and fusion genes between the GS and GU groups. (D) Hierarchical cluster of the patients in the GS and GU groups with the expression of 42 genome instability-related genes. (E) GO terms for functional enrichment of the 42 genome instability-related genes.
Subsequently, we explored the differences in mRNA expression between the GU and GS groups and found 42 differentially expressed genes (Figure 2D). Among these genes, ROS1 was reported as an oncogenic driver in lung cancer (Rikova et al., 2007), and tyrosine kinase inhibitors (TKIs) targeting ROS1 have been found to block tumor growth and provide clinical benefits for patients (D'Angelo et al., 2020; Shaw et al., 2014). MAGEA2, MAGEA3, MAGEA4, MAGEA6, and MEGEA12, members of the MAGE family, have been studied for their potential for cancer immunotherapy (Barker and Salehi, 2002). MAGEs were observed to be broadly expressed in many tumor types, such as lung cancer (Tajima et al., 2003; Gure et al., 2005; Kim et al., 2012), melanoma (Brasseur et al., 1995; Barrow et al., 2006), brain cancer (Scarcella et al., 1999), colorectal carcinoma (Mori et al., 1996), prostate cancer (Karpf et al., 2009), and breast cancer (Otte et al., 2001). To identify the potential functions of these 42 genes, we performed functional enrichment analysis, which indicated that these genes are involved mainly in the respiratory gaseous exchange by respiratory system (BP), histone deacetylase binding (MF) and endocytic vesicle, collagen trimer, lamellar body, multivesicular body, and clathrin-coated endocytic vesicle (CC) (Figure 2E).
To explore the potential prognostic value of the 42 genome instability-related genes, we randomly divided all 397 samples into a training group (n = 199) and a testing group (n = 198) with the same size of living and deceased overall survival status in each group. Next, we conducted a univariate Cox regression analysis with samples in the training group to identify the prognostic-related genes (Supplementary Table S2). Among the genes, IGF2BP3, SMC1B, IGF2BP1, CLDN6, and LY6K were found to be closely associated with overall survival (Table 2, p < 0.05). The combined signature of the five genes was named GULPsig. IGF2BP1 and IGF2BP3 are both members of the IGF2BP family (Bell et al., 2013). IGF2BP1 was found to promote proliferation (Rebucci et al., 2015), invasion (Zhou et al., 2015), and chemoresistance (Faye et al., 2015). In addition, its overexpression was associated with poor prognosis (Gu et al., 2004; Bell et al., 2015) in various types of cancer. There were also reports that the overexpression of IGF2BP3 was closely related to poor prognosis in endometrial carcinoma (Fadare et al., 2013), oral squamous cell carcinoma (Lin et al., 2011), colorectal cancer (Shantha Kumara et al., 2015), ovarian cancer (Hsu et al., 2015), and lung adenocarcinoma (Guo et al., 2021). The overexpression of SMC1B was found to be associated with poor overall survival in hepatocellular carcinoma (Nie et al., 2021). CLDN6 was also found to play a role in cancer cell migration and invasion in breast cancer (Song et al., 2019), hepatocellular carcinoma (Lu et al., 2021), and gastric cancer (Yu et al., 2019). LY6K was found to be a molecular marker for non-small-cell lung carcinoma (Ishikawa et al., 2007), head-and-neck squamous cell carcinoma (de Nooij-van Dalen et al., 2003), breast cancer (Kong et al., 2012), bladder cancer (Matsuda et al., 2011), and esophageal squamous cell carcinoma (Zhang et al., 2012).
We performed a multivariate Cox regression analysis (Figure 3A) and constructed a risk model based on the expression of GULPsig and the corresponding coefficients of multivariate Cox regression to evaluate the prognostic potential of GULPsig. The formula of the risk model is ORS = (0.141 × expression IGF2BP3) + (0.009 × expression SMC1B) + (0.053 × expression IGF2BP1) + (0.034 × expression CLDN6) + (0.069 × expression LY6K). The coefficients of GULPsig were all positive, suggesting that upregulated expressions of GULPsig are associated with poor prognosis. Then, we divided these patients into high- and low-risk groups according to the optimal cut-off threshold of 2.124. Patients whose scores were higher than the threshold were classified as high risk, and those whose scores were equal to or lower than the threshold were classified as low risk. We performed a chi-square test of association for the clinical characteristics between the high- and low-risk groups in the training group. A statistically significant association (p < 0.001) was found between the overall survival status and the risk groups, suggesting an association between the overall survival status and the risk groups (Supplementary Table S3).
FIGURE 3. Identification of GULPsig for overall survival prediction. (A) Forest plot to show the hazard ratio for each gene in GULPsig. (B) Survival curve for the high- and low-risk groups. (C) Expression of each gene in GULPsig between the high- and low-risk groups. (D) 1-, 3-, and 5-year ROC curves for GULPsig.
To evaluate the performance of the prognostic risk model, we conducted the KM survival analysis to estimate the survival probability and the log-rank test to compare the survival curves of the high- and low-risk groups (Figure 3B). We found that the overall survival of the low-risk group was significantly better than that of the high-risk group (p < 0.001). We also checked the expression of each gene within GULPsig for both the high- and low-risk groups. The results showed that the expression of each gene in the high-risk group was significantly higher than that in the low-risk group (Figure 3C).
We assessed the specificity and sensitivity of GULPsig using ROC curve analysis and calculated AUC to evaluate its performance in overall survival prediction at 1, 3, and 5 years. The AUC for 1, 3, and 5 years was 0.661, 0.705, and 0.715, respectively, suggesting that GULPsig is better for predicting overall survival at 5 years (Figure 3D). In addition, the results of SVM and 10-fold cross-validation showed that GULPsig is effective in classifying patients into high- and low-risk groups (Supplementary Figure S3D).
To further evaluate the risk model with GULPsig, we divided the testing group into high- and low-risk groups according to the optimal cut-off threshold. In the testing group, 76 patients were classified into the high-risk group and 122 patients into the low-risk group. The chi-square test of association showed a significant association between the overall survival status and the risk groups (Supplementary Table S3, p < 0.001). Moreover, the results of the KM survival analysis and the log-rank test showed a significant difference between the high- and low-risk groups (Figure 4A, p < 0.01). The expression of each gene in GULPsig was significantly higher in the high-risk group than in the low-risk group (Figure 4C, p < 0.01). Moreover, the AUC for 1, 3, and 5 years in the testing group was 0.672, 0.558, and 0.686, respectively, suggesting that GULPsig was more effective in predicting overall survival at 5 years (Figure 4B). Additionally, the results of SVM and 10-fold cross-validation suggest the effectiveness of GULPsig in classifying patients into high- and low-risk groups in the testing dataset (Supplementary Figure S2B).
FIGURE 4. Validation of GULPsig as a prognostic signature. (A) Survival curve for the high- and low-risk groups in the testing dataset. (B) 1-, 3-, and 5-year ROC curves for GULPsig. (C) Expression of each gene in GULPsig between the high- and low-risk groups in the testing dataset. (D) Survival curve for the high- and low-risk groups in GSE42127. (E) Survival curve for the high- and low-risk groups in GSE30219.
We also validated the prognostic value of the risk model with GULPsig on the TCGA dataset. Based on the optimal cut-off threshold, we classified 143 patients in the high-risk group and 254 patients in the low-risk group. We observed a significant association between the overall survival status and the risk groups (Supplementary Table S3, p < 0.001). The KM survival analysis and the log-rank test demonstrated that patients in the low-risk group had a significantly better prognosis than those in the high-risk group (Supplementary Figure S3A, p < 0.001). Furthermore, the expression of each gene in GULPsig was significantly higher in the high-risk group than in the low-risk group (Supplementary Figure S3B, p < 0.001). The AUC for 1, 3, and 5 years was 0.677, 0.634, and 0.697, respectively, indicating that GULPsig is better at predicting overall survival at 5 years (Supplementary Figure S3C). Moreover, SVM and 10-fold cross-validation showed the robustness of GULPsig for classifying patients into high- and low-risk groups (Supplementary Figure S3D). We used GSE42127 and GSE30219 to further validate the performance of GULPsig. When we applied the risk model to GSE42127, it classified 49 patients as high risk and 84 patients as low risk. The KM survival analysis and the log-rank test showed high significance between the high- and low-risk groups (Figure 4D, p < 0.001). For GSE30219, the model classified 11 patients as high risk and 74 as low risk. Similarly, a significant difference between the high-risk and low-risk groups was observed in the results of the KM analysis and the log-rank test (Figure 4E, p < 0.001).
To explore whether GULPsig was an independent prognostic signature from traditional clinical characteristics, we performed univariate and multivariate Cox regression analyses using the TCGA dataset. The results indicated that GULPsig (p < 0.001) is significantly associated with overall survival when adjustments are made for age, sex, tumor stage, T stage, M stage, and N stage (Table 3). Additionally, we divided the TCGA dataset into two groups based on age (>65 and ≤65), sex (male and female), and tumor stage (I/II and III/IV). Then, we used the risk model and categorized the patients in each group into high- and low-risk categories independently. The results demonstrated a significant difference between the high- and low-risk groups at age >65 (Figure 5A, p < 0.01), age ≤65 (Figure 5B, p < 0.01), male (Figure 5C, p < 0.05), female (Figure 5D, p < 0.01), tumor stage I/II (Figure 5E, p < 0.01), and tumor stage III/IV (Figure 5F, p < 0.01). These results demonstrate the independence of GULPsig as a prognostic signature, regardless of age, sex, and tumor stage.
FIGURE 5. Assessment of GULPsig as an independent prognostic signature. (A, B) Survival curve for the high- and low-risk groups among patients aged >65 and ≤65. (C, D) Survival curve for the high- and low-risk groups among males and females. (E, F) Survival curve for the high- and low-risk groups among patients at tumor stage I/II and tumor stage III/IV.
To further evaluate the performance of GULPsig, we compared GULPsig with traditional clinical characteristics, including age, sex, tumor stage, T stage, M stage, and N stage. By assessing the specificity and sensitivity using the ROC curve analysis and calculating the AUC, we found that the AUC of GULPsig (0.697) was larger than that of age (0.541), sex (0.457), tumor stage (0.664), T stage (0.567), M stage (0.549), and N stage (0.615), suggesting the better performance of GULPsig (Figure 6A). We also compared the performance of GULPsig with that of the state-of-the-art prognostic signatures, including the 7-gene signature (Al-Dherasi et al., 2021), 10-gene signature (Jiang et al., 2021), 4-gene signature (Liu et al., 2019), 9-gene signature (Zhang et al., 2019), and 6-gene signature (Xie and Xie, 2019). Still, the AUC of GULPsig (0.697) was larger than that of the 7-gene signature (0.609), 10-gene signature (0.524), 4-gene signature (0.654), 9-gene signature (0.655), and 6-gene signature (0.577), indicating that GULPsig performed better at overall survival prediction than the state-of-the-art prognostic signatures (Figure 6B).
FIGURE 6. Comparison of GULPsig with established prognostic signatures. (A) ROC curves comparing GULPsig with traditional clinical characteristics. (B) ROC curves comparing GULPsig with the state-of-the-art prognostic signatures.
Traditional prognostic signatures for lung adenocarcinoma patients, such as age (Tas et al., 2013), sex (Radkiewicz et al., 2019), TNM stage, and tumor stage (Goldstraw et al., 2007), have provided valuable insights. However, the absence of molecular characteristics limits the comprehensiveness and accuracy of prognostic signatures. As our study has shown, incorporating genome instability-derived genes can substantially improve the accuracy of overall survival prediction for lung adenocarcinoma patients. We initially identified 42 genome instability-related genes by analyzing the gene expression profiles of patients with various genomic alternations. Using functional analysis, we found that these genes focused mainly on the biological process of the respiratory gaseous exchange by the respiratory system and the molecular function of histone deacetylase binding, which play vital roles in studies of molecular mechanisms for lung cancer (Valavanidis et al., 2013; Sanaei and Kavoosi, 2019; Rolfo et al., 2022; Seguin et al., 2022). Furthermore, we identified GULPsig, which includes IGF2BP1, IGF2BP3, SMC1B, CLDN6, and LY6K, from these 42 genome instability-related genes. Each gene in GULPsig has been reported to be involved in tumor development (Bell et al., 2013; Du et al., 2021; Nie et al., 2021; Guo D et al., 2022). We also constructed a risk model with GULPsig and stratified the patients into high- and low-risk groups based on the optimal cut-off threshold. Then, we validated the performance of GULPsig in various cohorts, including the testing group, TCGA dataset, and external datasets GSE42127 and GSE30219. These results further corroborated the effectiveness of GULPsig as a reliable and robust prognostic signature for lung adenocarcinoma patients. As we continued to evaluate the independence of GULPsig as a prognostic signature, irrespective of factors such as age, gender, and tumor stage, we embarked on a more comprehensive investigation of its potential clinical implications. By comparing it with established prognostic signatures, our findings revealed that GULPsig surpassed both traditional clinical characteristics and state-of-the-art prognostic signatures in performance, highlighting its potential for enhanced clinical applications in lung adenocarcinoma prognosis.
To the best of our knowledge, this study is the first to integrate somatic mutations, copy number variations, and fusion genes to comprehensively measure genome instability. Prior studies typically considered only one or two genomic alternations (Guo and Wang, 2021; Song et al., 2021; Chen et al., 2022). Our approach offers a more holistic representation of genome instability and its impact on prognostic signatures. Furthermore, while Guo C R et al. (2022) and Peng et al. (2021) identified genome instability-derived lncRNA signatures for lung adenocarcinoma patients, our study focused on mRNA-based signatures. An mRNA-based signature allows for a more direct assessment of gene expression, which, in turn, provides valuable insights into the functional consequences of genome instability. Additionally, focusing on mRNA can facilitate the identification of novel therapeutic targets and enrich our understanding of the molecular mechanisms driving tumor progression. This distinct approach underscores the unique contribution of our study to the field of lung adenocarcinoma prognostic research.
Our study has some limitations. The method of quantifying genome instability using genomic alterations needs further exploration. While we have pinpointed GULPsig as a potential prognostic signature for overall survival prediction, the hidden biological mechanisms related to GULPsig are still unclear, so further investigation is needed.
In conclusion, we identified GULPsig, consisting of IGF2BP1, IGF2BP3, SMC1B, CLDN6, and LY6K, as a potential prognostic signature for lung adenocarcinoma patients. However, further studies are required for clinical applications, such as the further validation of biological experiments and the exploration of hidden biological mechanisms.
The original contributions presented in the study are included in the article/Supplementary Material; further inquiries can be directed to the corresponding author.
XZ and H-FT conceptualized the study. XZ formulated the methodology, performed the investigation and visualization, and wrote the original draft. H-FT and T-WL reviewed and edited the manuscript. H-FT supervised the study and secured the funding. All authors contributed to the article and approved the submitted version.
This study was supported by a Hong Kong GRF grant (HKU17208019).
The authors would like to thank the Information Technology Services of the University of Hong Kong for providing the High-Performance Computing Cluster resources and facilities for the study.
The authors declare that the research was conducted in the absence of any commercial or financial relationships that could be construed as a potential conflict of interest.
All claims expressed in this article are solely those of the authors and do not necessarily represent those of their affiliated organizations, or those of the publisher, the editors, and the reviewers. Any product that may be evaluated in this article, or claim that may be made by its manufacturer, is not guaranteed or endorsed by the publisher.
The Supplementary Material for this article can be found online at: https://www.frontiersin.org/articles/10.3389/fcell.2023.1224069/full#supplementary-material
Al-Dherasi, A., Huang, Q. T., Liao, Y. W., Al-Mosaib, S., Hua, R. L., Wang, Y. C., et al. (2021). A seven-gene prognostic signature predicts overall survival of patients with lung adenocarcinoma (LUAD). Cancer Cell Int. 21, 294. doi:10.1186/s12935-021-01975-z
Barker, P. A., and Salehi, A. (2002). The MAGE proteins: emerging roles in cell cycle progression, apoptosis, and neurogenetic disease. J. Neurosci. Res. 67, 705–712. doi:10.1002/jnr.10160
Barrow, C., Browning, J., Macgregor, D., Davis, I. D., Sturrock, S., Jungbluth, A. A., et al. (2006). Tumor antigen expression in melanoma varies according to antigen and stage. Clin. Cancer Res. 12, 764–771. doi:10.1158/1078-0432.CCR-05-1544
Barta, J. A., Powell, C. A., and Wisnivesky, J. P. (2019). Global epidemiology of lung cancer. Ann. Glob. Health 85, 8. doi:10.5334/aogh.2419
Bell, J. L., Turlapati, R., Liu, T., Schulte, J. H., and Huttelmaier, S. (2015). IGF2BP1 harbors prognostic significance by gene gain and diverse expression in neuroblastoma. J. Clin. Oncol. 33, 1285–1293. doi:10.1200/JCO.2014.55.9880
Bell, J. L., Wachter, K., Muhleck, B., Pazaitis, N., Kohn, M., Lederer, M., et al. (2013). Insulin-like growth factor 2 mRNA-binding proteins (IGF2BPs): post-transcriptional drivers of cancer progression? Cell Mol. Life Sci. 70, 2657–2675. doi:10.1007/s00018-012-1186-z
Brasseur, F., Rimoldi, D., Lienard, D., Lethe, B., Carrel, S., Arienti, F., et al. (1995). Expression of MAGE genes in primary and metastatic cutaneous melanoma. Int. J. Cancer 63, 375–380. doi:10.1002/ijc.2910630313
Bray, F., Ferlay, J., Soerjomataram, I., Siegel, R. L., Torre, L. A., and Jemal, A. (2018). Global cancer statistics 2018: GLOBOCAN estimates of incidence and mortality worldwide for 36 cancers in 185 countries. CA Cancer J. Clin. 68, 394–424. doi:10.3322/caac.21492
Cerami, E., Gao, J., Dogrusoz, U., Gross, B. E., Sumer, S. O., Aksoy, B. A., et al. (2012). The cBio cancer genomics portal: an open platform for exploring multidimensional cancer genomics data. Cancer Discov. 2, 401–404. doi:10.1158/2159-8290.CD-12-0095
Chen, X., Tian, F., and Wu, Z. (2022). A genomic instability-associated prognostic signature for glioblastoma patients. World Neurosurg. 167, e515–e526. doi:10.1016/j.wneu.2022.08.049
D'Angelo, A., Sobhani, N., Chapman, R., Bagby, S., Bortoletti, C., Traversini, M., et al. (2020). Focus on ROS1-positive non-small cell lung cancer (NSCLC): crizotinib, resistance mechanisms and the newer generation of targeted therapies. Cancers (Basel) 12, 3293. doi:10.3390/cancers12113293
Davis, S., and Meltzer, P. S. (2007). GEOquery: A bridge between the gene expression Omnibus (GEO) and BioConductor. Bioinformatics 23, 1846–1847. doi:10.1093/bioinformatics/btm254
De Nooij-Van Dalen, A. G., Van Dongen, G. A., Smeets, S. J., Nieuwenhuis, E. J., Stigter-Van Walsum, M., Snow, G. B., et al. (2003). Characterization of the human Ly-6 antigens, the newly annotated member Ly-6K included, as molecular markers for head-and-neck squamous cell carcinoma. Int. J. Cancer 103, 768–774. doi:10.1002/ijc.10903
Du, H., Yang, X., Fan, J., and Du, X. (2021). Claudin 6: therapeutic prospects for tumours, and mechanisms of expression and regulation (Review). Mol. Med. Rep. 24, 677. doi:10.3892/mmr.2021.12316
Fadare, O., Liang, S. X., Crispens, M. A., Jones, H. W., 3R. D., Khabele, D., Gwin, K., et al. (2013). Expression of the oncofetal protein IGF2BP3 in endometrial clear cell carcinoma: assessment of frequency and significance. Hum. Pathol. 44, 1508–1515. doi:10.1016/j.humpath.2012.12.003
Faye, M. D., Beug, S. T., Graber, T. E., Earl, N., Xiang, X., Wild, B., et al. (2015). IGF2BP1 controls cell death and drug resistance in rhabdomyosarcomas by regulating translation of cIAP1. Oncogene 34, 1532–1541. doi:10.1038/onc.2014.90
Goldstraw, P., Crowley, J., Chansky, K., Giroux, D. J., Groome, P. A., Rami-Porta, R., et al. (2007). The IASLC lung cancer staging project: proposals for the revision of the TNM stage groupings in the forthcoming (seventh) edition of the TNM classification of malignant tumours. J. Thorac. Oncol. 2, 706–714. doi:10.1097/JTO.0b013e31812f3c1a
Gao, J., Aksoy, B. A., Dogrusoz, U., Dresdner, G., Gross, B., Sumer, S. O., et al. (2013). Integrative analysis of complex cancer genomics and clinical profiles using the cBioPortal. Sci. Signal 6, pl1. doi:10.1126/scisignal.2004088
Gu, L. J., Shigemasa, K., and Ohama, K. (2004). Increased expression of IGF II mRNA-binding protein 1 mRNA is associated with an advanced clinical stage and poor prognosis in patients with ovarian cancer. Int. J. Oncol. 24, 671–678. doi:10.3892/ijo.24.3.671
Guo, C. R., Mao, Y., Jiang, F., Juan, C. X., Zhou, G. P., and Li, N. (2022). Computational detection of a genome instability-derived lncRNA signature for predicting the clinical outcome of lung adenocarcinoma. Cancer Med. 11, 864–879. doi:10.1002/cam4.4471
Guo, D., Liu, Y., Jiang, Y., Zheng, S., Xu, T., Zhu, J., et al. (2022). A narrative review of the emerging role of lymphocyte antigen 6 complex locus K in cancer: from basic research to clinical practice. Ann. Transl. Med. 10, 26. doi:10.21037/atm-21-5831
Guo, M., and Wang, S. M. (2021). Genome instability-derived genes are novel prognostic biomarkers for triple-negative breast cancer. Front. Cell Dev. Biol. 9, 701073. doi:10.3389/fcell.2021.701073
Guo, W., Huai, Q., Wan, H., Guo, L., Song, P., Gao, S., et al. (2021). Prognostic impact of IGF2BP3 expression in patients with surgically resected lung adenocarcinoma. DNA Cell Biol. 40, 316–331. doi:10.1089/dna.2020.6136
Gure, A. O., Chua, R., Williamson, B., Gonen, M., Ferrera, C. A., Gnjatic, S., et al. (2005). Cancer-testis genes are coordinately expressed and are markers of poor outcome in non-small cell lung cancer. Clin. Cancer Res. 11, 8055–8062. doi:10.1158/1078-0432.CCR-05-1203
Hanahan, D., and Weinberg, R. A. (2011). Hallmarks of cancer: the next generation. Cell 144, 646–674. doi:10.1016/j.cell.2011.02.013
Hight, S. K., Mootz, A., Kollipara, R. K., Mcmillan, E., Yenerall, P., Otaki, Y., et al. (2020). An in vivo functional genomics screen of nuclear receptors and their co-regulators identifies FOXA1 as an essential gene in lung tumorigenesis. Neoplasia 22, 294–310. doi:10.1016/j.neo.2020.04.005
Hsu, K. F., Shen, M. R., Huang, Y. F., Cheng, Y. M., Lin, S. H., Chow, N. H., et al. (2015). Overexpression of the RNA-binding proteins Lin28B and IGF2BP3 (IMP3) is associated with chemoresistance and poor disease outcome in ovarian cancer. Br. J. Cancer 113, 414–424. doi:10.1038/bjc.2015.254
Ishikawa, N., Takano, A., Yasui, W., Inai, K., Nishimura, H., Ito, H., et al. (2007). Cancer-testis antigen lymphocyte antigen 6 complex locus K is a serologic biomarker and a therapeutic target for lung and esophageal carcinomas. Cancer Res. 67, 11601–11611. doi:10.1158/0008-5472.CAN-07-3243
Jiang, W., Xu, J., Liao, Z., Li, G., Zhang, C., and Feng, Y. (2021). Prognostic signature for lung adenocarcinoma patients based on cell-cycle-related genes. Front. Cell Dev. Biol. 9, 655950. doi:10.3389/fcell.2021.655950
Karpf, A. R., Bai, S., James, S. R., Mohler, J. L., and Wilson, E. M. (2009). Increased expression of androgen receptor coregulator MAGE-11 in prostate cancer by DNA hypomethylation and cyclic AMP. Mol. Cancer Res. 7, 523–535. doi:10.1158/1541-7786.MCR-08-0400
Kim, Y. D., Park, H. R., Song, M. H., Shin, D. H., Lee, C. H., Lee, M. K., et al. (2012). Pattern of cancer/testis antigen expression in lung cancer patients. Int. J. Mol. Med. 29, 656–662. doi:10.3892/ijmm.2012.896
Kong, H. K., Yoon, S., and Park, J. H. (2012). The regulatory mechanism of the LY6K gene expression in human breast cancer cells. J. Biol. Chem. 287, 38889–38900. doi:10.1074/jbc.M112.394270
Lin, C. Y., Chen, S. T., Jeng, Y. M., Yeh, C. C., Chou, H. Y., Deng, Y. T., et al. (2011). Insulin-like growth factor II mRNA-binding protein 3 expression promotes tumor formation and invasion and predicts poor prognosis in oral squamous cell carcinoma. J. Oral Pathol. Med. 40, 699–705. doi:10.1111/j.1600-0714.2011.01019.x
Liu, C., Li, Y., Wei, M., Zhao, L., Yu, Y., and Li, G. (2019). Identification of a novel glycolysis-related gene signature that can predict the survival of patients with lung adenocarcinoma. Cell Cycle 18, 568–579. doi:10.1080/15384101.2019.1578146
Liu, J., Cui, G., Ye, J., Wang, Y., Wang, C., and Bai, J. (2022). Comprehensive analysis of the prognostic signature of mutation-derived genome instability-related lncRNAs for patients with endometrial cancer. Front. Cell Dev. Biol. 10, 753957. doi:10.3389/fcell.2022.753957
Lu, Y., Dang, Q., Bo, Y., Su, X., Wang, L., Sun, J., et al. (2021). The expression of CLDN6 in hepatocellular carcinoma tissue and the effects of CLDN6 on biological phenotypes of hepatocellular carcinoma cells. J. Cancer 12, 5454–5463. doi:10.7150/jca.55727
Matsuda, R., Enokida, H., Chiyomaru, T., Kikkawa, N., Sugimoto, T., Kawakami, K., et al. (2011). LY6K is a novel molecular target in bladder cancer on basis of integrate genome-wide profiling. Br. J. Cancer 104, 376–386. doi:10.1038/sj.bjc.6605990
Mori, M., Inoue, H., Mimori, K., Shibuta, K., Baba, K., Nakashima, H., et al. (1996). Expression of MAGE genes in human colorectal carcinoma. Ann. Surg. 224, 183–188. doi:10.1097/00000658-199608000-00011
Nagy-Mignotte, H., Guillem, P., Vesin, A., Toffart, A. C., Colonna, M., Bonneterre, V., et al. (2011). Primary lung adenocarcinoma: characteristics by smoking habit and sex. Eur. Respir. J. 38, 1412–1419. doi:10.1183/09031936.00191710
Negrini, S., Gorgoulis, V. G., and Halazonetis, T. D. (2010). Genomic instability--an evolving hallmark of cancer. Nat. Rev. Mol. Cell Biol. 11, 220–228. doi:10.1038/nrm2858
Nie, H., Wang, Y., Yang, X., Liao, Z., He, X., Zhou, J., et al. (2021). Clinical significance and integrative analysis of the SMC family in hepatocellular carcinoma. Front. Med. (Lausanne) 8, 727965. doi:10.3389/fmed.2021.727965
Otte, M., Zafrakas, M., Riethdorf, L., Pichlmeier, U., Loning, T., Janicke, F., et al. (2001). MAGE-A gene expression pattern in primary breast cancer. Cancer Res. 61, 6682–6687.
Peng, B., Li, H., Na, R., Lu, T., Li, Y., Zhao, J., et al. (2021). Identification of a novel prognostic signature of genome instability-related LncRNAs in early stage lung adenocarcinoma. Front. Cell Dev. Biol. 9, 706454. doi:10.3389/fcell.2021.706454
Radkiewicz, C., Dickman, P. W., Johansson, A. L. V., Wagenius, G., Edgren, G., and Lambe, M. (2019). Sex and survival in non-small cell lung cancer: A nationwide cohort study. PLoS One 14, e0219206. doi:10.1371/journal.pone.0219206
Rebucci, M., Sermeus, A., Leonard, E., Delaive, E., Dieu, M., Fransolet, M., et al. (2015). miRNA-196b inhibits cell proliferation and induces apoptosis in HepG2 cells by targeting IGF2BP1. Mol. Cancer 14, 79. doi:10.1186/s12943-015-0349-6
Rikova, K., Guo, A., Zeng, Q., Possemato, A., Yu, J., Haack, H., et al. (2007). Global survey of phosphotyrosine signaling identifies oncogenic kinases in lung cancer. Cell 131, 1190–1203. doi:10.1016/j.cell.2007.11.025
Ritchie, M. E., Phipson, B., Wu, D., Hu, Y., Law, C. W., Shi, W., et al. (2015). Limma powers differential expression analyses for RNA-sequencing and microarray studies. Nucleic Acids Res. 43, e47. doi:10.1093/nar/gkv007
Rolfo, C., Meshulami, N., Russo, A., Krammer, F., Garcia-Sastre, A., Mack, P. C., et al. (2022). Lung cancer and severe acute respiratory syndrome coronavirus 2 infection: identifying important knowledge gaps for investigation. J. Thorac. Oncol. 17, 214–227. doi:10.1016/j.jtho.2021.11.001
Rousseaux, S., Debernardi, A., Jacquiau, B., Vitte, A. L., Vesin, A., Nagy-Mignotte, H., et al. (2013). Ectopic activation of germline and placental genes identifies aggressive metastasis-prone lung cancers. Sci. Transl. Med. 5, 186ra66. doi:10.1126/scitranslmed.3005723
Sanaei, M., and Kavoosi, F. (2019). Histone deacetylases and histone deacetylase inhibitors: molecular mechanisms of action in various cancers. Adv. Biomed. Res. 8, 63. doi:10.4103/abr.abr_142_19
Scarcella, D. L., Chow, C. W., Gonzales, M. F., Economou, C., Brasseur, F., and Ashley, D. M. (1999). Expression of MAGE and GAGE in high-grade brain tumors: a potential target for specific immunotherapy and diagnostic markers. Clin. Cancer Res. 5, 335–341.
Seguin, L., Durandy, M., and Feral, C. C. (2022). Lung adenocarcinoma tumor origin: a guide for personalized medicine. Cancers (Basel) 14, 1759. doi:10.3390/cancers14071759
Shantha Kumara, H., Kirchoff, D., Caballero, O. L., Su, T., Ahmed, A., Herath, S. A., et al. (2015). Expression of the cancer testis antigen IGF2BP3 in colorectal cancers; IGF2BP3 holds promise as a specific immunotherapy target. Oncoscience 2, 607–614. doi:10.18632/oncoscience.174
Shaw, A. T., Ou, S. H., Bang, Y. J., Camidge, D. R., Solomon, B. J., Salgia, R., et al. (2014). Crizotinib in ROS1-rearranged non-small-cell lung cancer. N. Engl. J. Med. 371, 1963–1971. doi:10.1056/NEJMoa1406766
Shen, Z. (2011). Genomic instability and cancer: an introduction. J. Mol. Cell Biol. 3, 1–3. doi:10.1093/jmcb/mjq057
Song, P., Li, Y., Dong, Y., Liang, Y., Qu, H., Qi, D., et al. (2019). Estrogen receptor beta inhibits breast cancer cells migration and invasion through CLDN6-mediated autophagy. J. Exp. Clin. Cancer Res. 38, 354. doi:10.1186/s13046-019-1359-9
Song, Z. B., Yu, Y., Zhang, G. P., and Li, S. Q. (2021). Genomic instability of mutation-derived gene prognostic signatures for hepatocellular carcinoma. Front. Cell Dev. Biol. 9, 728574. doi:10.3389/fcell.2021.728574
Sun, J., Jiang, Q., Chen, H., Zhang, Q., Zhao, J., Li, H., et al. (2021). Genomic instability-associated lncRNA signature predicts prognosis and distinct immune landscape in gastric cancer. Ann. Transl. Med. 9, 1326. doi:10.21037/atm-21-3569
Suzuki, K., Ohnami, S., Tanabe, C., Sasaki, H., Yasuda, J., Katai, H., et al. (2003). The genomic damage estimated by arbitrarily primed PCR DNA fingerprinting is useful for the prognosis of gastric cancer. Gastroenterology 125, 1330–1340. doi:10.1016/j.gastro.2003.07.006
Tajima, K., Obata, Y., Tamaki, H., Yoshida, M., Chen, Y. T., Scanlan, M. J., et al. (2003). Expression of cancer/testis (CT) antigens in lung cancer. Lung Cancer 42, 23–33. doi:10.1016/s0169-5002(03)00244-7
Tang, H., Xiao, G., Behrens, C., Schiller, J., Allen, J., Chow, C. W., et al. (2013). A 12-gene set predicts survival benefits from adjuvant chemotherapy in non-small cell lung cancer patients. Clin. Cancer Res. 19, 1577–1586. doi:10.1158/1078-0432.CCR-12-2321
Tas, F., Ciftci, R., Kilic, L., and Karabulut, S. (2013). Age is a prognostic factor affecting survival in lung cancer patients. Oncol. Lett. 6, 1507–1513. doi:10.3892/ol.2013.1566
Travis, W. D., Brambilla, E., Nicholson, A. G., Yatabe, Y., Austin, J. H. M., Beasley, M. B., et al. (2015). The 2015 world health organization classification of lung tumors: impact of genetic, clinical and radiologic advances since the 2004 classification. J. Thorac. Oncol. 10, 1243–1260. doi:10.1097/JTO.0000000000000630
Valavanidis, A., Vlachogianni, T., Fiotakis, K., and Loridas, S. (2013). Pulmonary oxidative stress, inflammation and cancer: respirable particulate matter, fibrous dusts and ozone as major causes of lung carcinogenesis through reactive oxygen species mechanisms. Int. J. Environ. Res. Public Health 10, 3886–3907. doi:10.3390/ijerph10093886
Woo, S. M., Kim, M. K., Park, B., Cho, E. H., Lee, T. R., Ki, C. S., et al. (2021). Genomic instability of circulating tumor dna as a prognostic marker for pancreatic cancer survival: A prospective cohort study. Cancers 13, 5466. doi:10.3390/cancers13215466
Wu, T., Hu, E., Xu, S., Chen, M., Guo, P., Dai, Z., et al. (2021). ClusterProfiler 4.0: A universal enrichment tool for interpreting omics data. Innov. (Camb) 2, 100141. doi:10.1016/j.xinn.2021.100141
Xie, H., and Xie, C. (2019). A six-gene signature predicts survival of adenocarcinoma type of non-small-cell lung cancer patients: A comprehensive study based on integrated analysis and weighted gene coexpression network. Biomed. Res. Int. 2019, 4250613. doi:10.1155/2019/4250613
Yu, S., Zhang, Y., Li, Q., Zhang, Z., Zhao, G., and Xu, J. (2019). CLDN6 promotes tumor progression through the YAP1-snail1 axis in gastric cancer. Cell Death Dis. 10, 949. doi:10.1038/s41419-019-2168-y
Zhang, B., Zhang, Z., Zhang, X., Gao, X., Kernstine, K. H., and Zhong, L. (2012). Serological antibodies against LY6K as a diagnostic biomarker in esophageal squamous cell carcinoma. Biomarkers 17, 372–378. doi:10.3109/1354750X.2012.680609
Zhang, L., Zhang, Z., and Yu, Z. (2019). Identification of a novel glycolysis-related gene signature for predicting metastasis and survival in patients with lung adenocarcinoma. J. Transl. Med. 17, 423. doi:10.1186/s12967-019-02173-2
Keywords: genome instability, lung adenocarcinoma, somatic mutations, copy number variation, fusion genes, overall survival prediction
Citation: Zhang X, Lam T-W and Ting H-F (2023) Genome instability-derived genes as a novel prognostic signature for lung adenocarcinoma. Front. Cell Dev. Biol. 11:1224069. doi: 10.3389/fcell.2023.1224069
Received: 17 May 2023; Accepted: 31 July 2023;
Published: 16 August 2023.
Edited by:
Dong Zhang, New York Institute of Technology, United StatesReviewed by:
Guozhong Jiang, First Affiliated Hospital of Zhengzhou University, ChinaCopyright © 2023 Zhang, Lam and Ting. This is an open-access article distributed under the terms of the Creative Commons Attribution License (CC BY). The use, distribution or reproduction in other forums is permitted, provided the original author(s) and the copyright owner(s) are credited and that the original publication in this journal is cited, in accordance with accepted academic practice. No use, distribution or reproduction is permitted which does not comply with these terms.
*Correspondence: Hing-Fung Ting, aGZ0aW5nQGNzLmhrdS5oaw==
Disclaimer: All claims expressed in this article are solely those of the authors and do not necessarily represent those of their affiliated organizations, or those of the publisher, the editors and the reviewers. Any product that may be evaluated in this article or claim that may be made by its manufacturer is not guaranteed or endorsed by the publisher.
Research integrity at Frontiers
Learn more about the work of our research integrity team to safeguard the quality of each article we publish.