- 1INSERM U1169, Bicêtre Hospital, Paris Sud University, Le Kremlin-Bicêtre, Paris, France
- 2The Laboratory for Epigenetics and Environment, Centre National de Recherche en Genomique Humaine, CEA-Institut de Biologie Francois Jacob, Université Paris-Saclay, Evry, France
- 3Centre National de Recherche en Génomique Humaine, Institut de Biologie François Jacob, CEA, Université Paris-Saclay, Evry, France
- 4Child and Perinatal Psychiatric Department, Le Havre University Hospital, University Rouen Normandie, Le Havre, France
- 5Department of Obstetrics and Gynecology, DMU Santé des Femmes et des Nouveau-nés, Assistance Publique Hôpitaux de Paris, Antoine Beclere Hospital, Université Paris-Saclay, Paris, France
Pregnancy is a state of multiple physiological adaptations. Since methylation of DNA is an epigenetic mechanism that regulates gene expression and contributes to adaptive phenotypic variations, we investigated methylation changes in maternal blood of a longitudinal cohort of pregnant women from the first trimester of gestation to the third. Interestingly, during pregnancy, we found a gain of methylation in genes involved in morphogenesis, such as ezrin, while we identified a loss of methylation in genes promoting maternal-infant bonding (AVP and PPP1R1B). Together, our results provide insights into the biological mechanisms underlying physiological adaptations during pregnancy.
Introduction
DNA methylation, the addition of a CH3 group to the carbon-5 position of cytosine bases in the context of CpG dinucleotides, has been associated with multiple adverse health conditions; however, in non-pathological states, DNA methylation is a key determinant of plasticity and adaptation while maintaining normal cellular function and gene expression. DNA methylation is sensitive to numerous extrinsic and intrinsic factors, such as nutrition, stress, sex, or aging (Lam et al., 2012). Some studies also showed that DNA methylation in peripheral blood cells varies over time (Bjornsson et al., 2008; Feinberg et al., 2010; Flanagan et al., 2015). However, little is known about the dynamics of maternal DNA methylation status during pregnancy, even if this period is a well-known state of intense physiological changes. In this sense, DNA methylation would modulate gene expression and thus participate in the gestational adaptation of almost all maternal organs.
This study aimed to fill this gap by investigating DNA methylation changes in maternal blood from early to late pregnancy (up to week 18 of amenorrhea compared with week 35 and later) in 36 Caucasian women.
Methods
Pregnant women were included in the study in accordance with French bioethics law, with patients being carefully informed and having signed a detailed informed consent. All protocols were approved by the French ethic councils (CPP C0-14-001, CNIL No. C13–61). DNA was extracted from whole blood cells using the Gentra DNA extraction kit (Qiagen). Genomic DNA (1 μg) from each of the 36 pregnant women was bisulfite-converted using the EpiTect® Fast 96 DNA Bisulfite Kit (Qiagen), and the DNA was analyzed using the Illumina Infinium MethylationEPIC (850K) BeadChip at the Centre National de Recherche en Genomique Humaine (CNRGH, CEA, Evry, France). Preprocessing and normalization were performed using the ChAMP pipeline and involved steps of probe filtering, color bias correction, background subtraction, and beta-mixture quantile normalization method (Tian et al., 2017). Array and slide effects were corrected using the ComBat function implemented in the ChAMP package as well (Tian et al., 2017). Cell-type heterogeneity corrected beta matrix and cell-type-specific proportion in each blood sample were obtained using the champ.refbase function again implemented in the ChAMP package. Finally, we used this same package to identify differentially methylated CpG (DMP) and differentially methylated regions (DMRs) using the BumpHunter algorithm (Tian et al., 2017). Functional enrichment analysis was conducted on the GSEA website (http://www.gsea-msigdb.org/).
Results
DNA methylation variation was explored in 36 pregnant women. The age of the women ranged from 24.1 to 38.1 years (median 30.31), with a first-trimester BMI of 23.45 ± 3.42 kg/m2 and a weight gain of 8.91 ± 0.2 kg during pregnancy (Supplemental Figure 1). We analyzed DNA methylation on the Infinium Human MethylationEPIC Beadchip (Illumina) that covers 846,604 CpG loci at single-nucleotide resolution.
As cellular heterogeneity strongly influences methylation profiles and can drive some of the methylation differences detectable across individual blood samples, we first estimated the cell-type compositions in each sample. We found that cellular composition varies across individuals and across the first and third trimesters, so we then used the corrected beta matrix to conduct our analyses and avoid those differences in methylation resulting from differences in cellular heterogeneity.
After correction for cellular heterogeneity and multiple testing, methylation levels changed at 57 CpGs between the first and third trimesters of pregnancy (Table 1; Supplementary Table S1). These CpGs are annotated to 31 genes, and five showed an increasing methylation level during pregnancy, whereas all others decreased. Among them, MIR10A showed methylation changes at four distinct CpGs (Figure 1A). No hypermethylated CpGs were located in shore or shelf regions, whereas we observed an enrichment of these CpGs over the CpG islands (CGIs) or the opensea regions (Figure 1B). The genomic distribution of the 57 CpGs in comparison to all the probes located on the 850K BeadChip array with respect to the gene structure showed an enrichment of hypermethylated CpGs inside the gene body or exon binding regions (Figure 1C). We found no correlation between these DNA methylation changes and age or BMI in our cohort (Supplementary Figure S2).
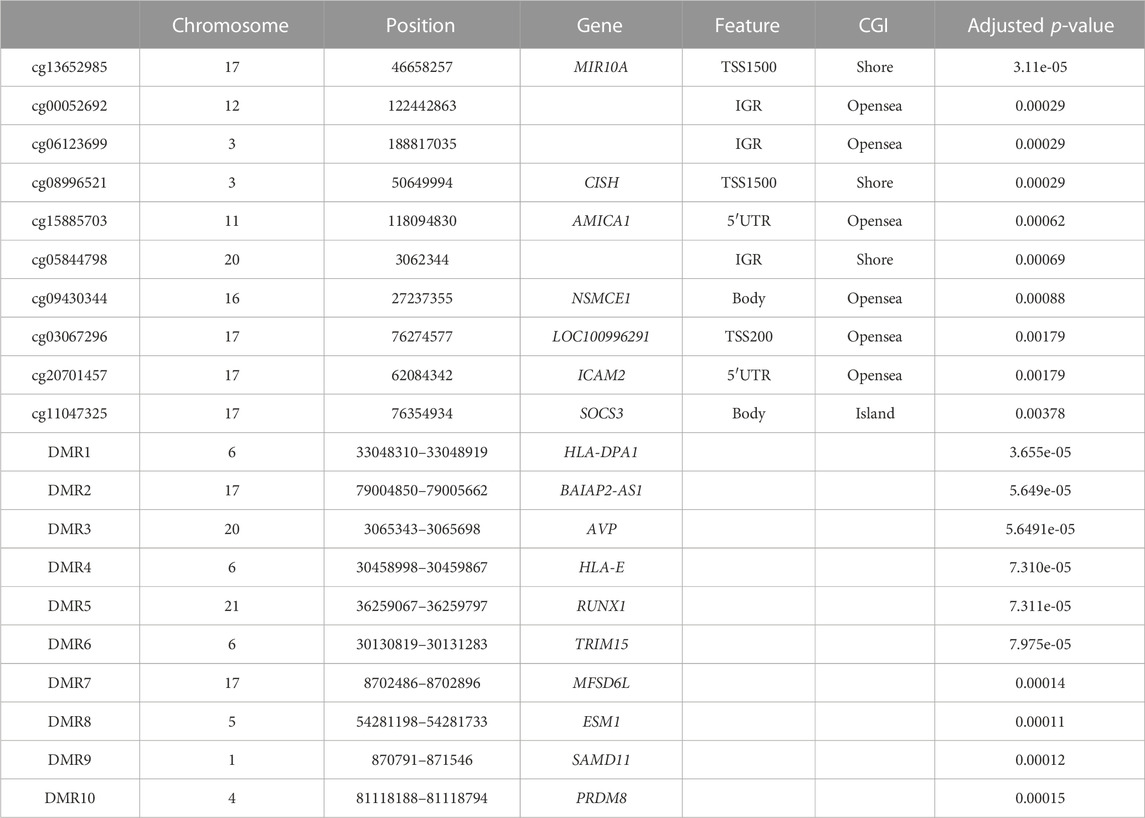
TABLE 1. Top 10 of the most significant differentially methylated CpGs and the most significant differentially methylated regions (DMRs) during pregnancy.
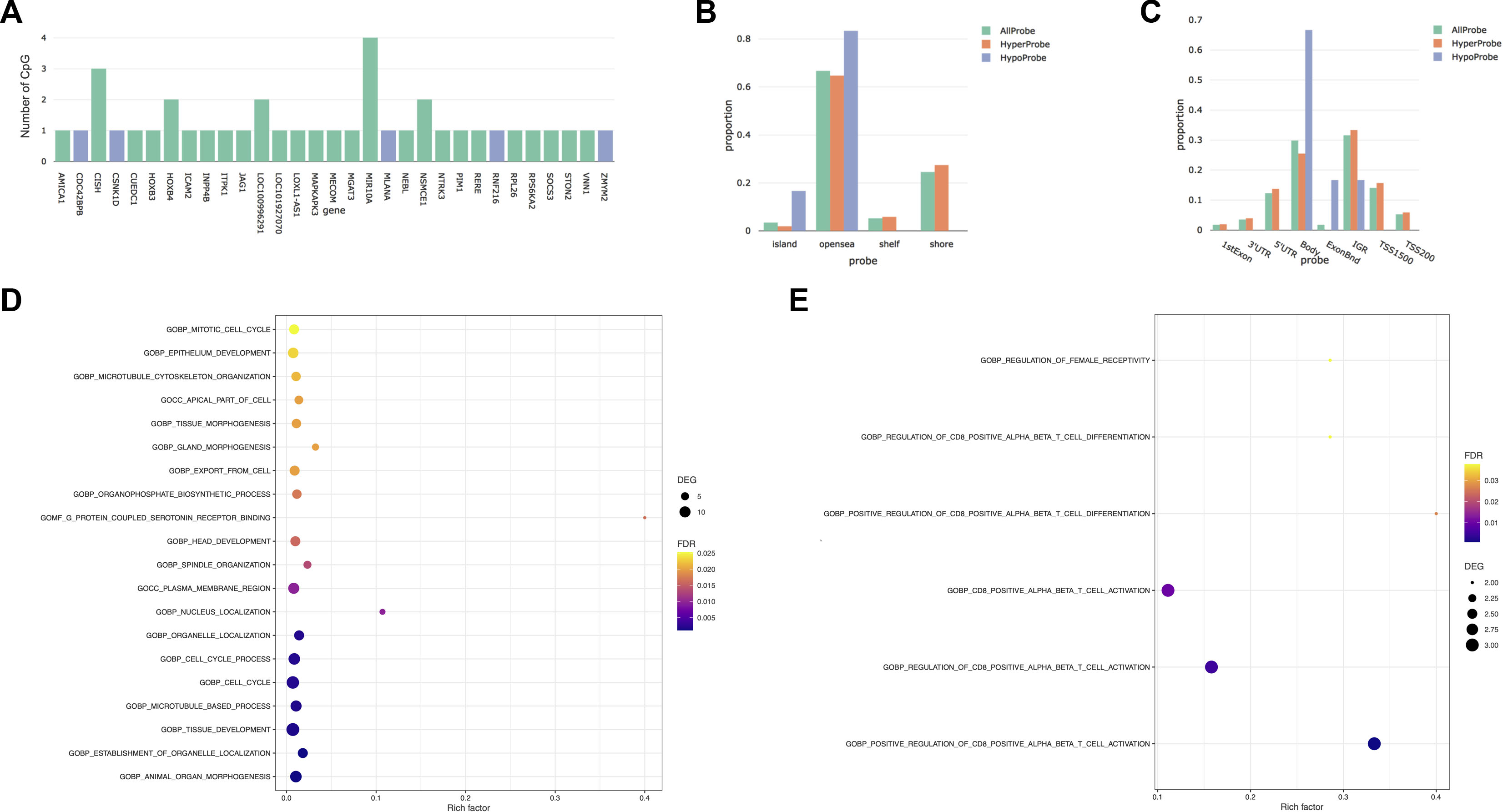
FIGURE 1. Differentially methylated CpGs between first and third trimesters. (A) Number of differentially methylated CpGs by genes. Blue represents the hypomethylated genes, while green represents the hypermethylated genes. (B) Distribution of differentially methylated CpGs versus all CpG sites on the EPIC array in relation to the CpG island annotation. (C) Distribution of differentially methylated CpGs versus all CpG sites on the EPIC array in relation to the nearest gene regions. ExonBnd, exon binding region; IGR, intergenic region; TSS200, 200 base pairs (bps) around the transcriptional start site; TSS1500, 1500 bps around the transcriptional start site. (D) Bubble diagram of Gene Ontology (GO) analysis of DMRs that gained methylation across pregnancy. (E) Bubble diagram of Gene Ontology (GO) analysis of DMRs that lose methylation during pregnancy. The Y-axis label represents the pathway, and the X-axis label represents the rich factor (rich factor = amount of differentially expressed genes enriched in the pathway/amount of all genes in the background gene set). Size and color of the bubble represent the amount of differentially expressed genes enriched in the pathway and enrichment significance, respectively. DEGs, differentially expressed genes; FDR, false discovery rate.
Next, we analyzed our DNA methylation data to identify DMRs, since it has been shown that the identification of regional differences across several CpGs provides more robust findings and is more likely to be replicated than individual CpG differences. We identified 157 DMRs between the first and third trimesters of pregnancy (Table 1; Supplementary Table S2); 98 DMRs showed a gain of methylation, while 59 DMRs showed a decrease. Gene Ontology (GO) analysis identified enrichment in several pathways involved in tissue development, morphogenesis, and cell cycle in the 98 DMRs that gained methylation during pregnancy (Figure 1D), whereas those that lost methylation were involved in immune cell functions (Figure 1E).
Discussion
Our study supports evidence that DNA methylation changes in maternal blood cells during pregnancy, which may be important for maternal adaptation to gestation and labor. Indeed, maternal systems progressively adapt during human pregnancy to accommodate the increasing demands of fetal growth and development and prepare for parturition. These physiological adaptations seem to correlate with DNA methylation changes as a master regulator of gene expression.
We identified 39 individual CpGs and 157 regions showing DNA methylation changes across pregnancy after correction for multiple testing. Biological pathway analysis revealed that CpGs that gained methylation included genes involved in organ morphogenesis, epithelium development, and cell cycle, among others. These genes are needed during the formation of the placenta or the early stages of pregnancy. Among them, ezrin (EZR) is an interesting candidate since it is a cytoskeletal linker protein that facilitates uterine receptivity and embryo–endometrium physical attachment (Lecce et al., 2011). Its expression is consequently necessary at the beginning of the pregnancy and seems to be regulated by DNA methylation (Turco et al., 2018).
Concerning CpGs that lose DNA methylation during pregnancy, they belong mainly to genes involved in immune functions. It is indeed well described that the maintenance of pregnancy relies on finely tuned immune adaptations since the maternal immune system should tolerate the fetus while preserving its abilities against microbial or viral attacks. We interestingly identified a loss of DNA methylation in HLA-E (human leukocyte antigen E). This gene was initially recognized as HLA-6.2 and is a ligand of KIR (killer cell immunoglobulin-like receptor) of NK (natural killer) cells, which leads to downregulation of immune response and helps in the maintenance of pregnancy. In this context, Tripathi et al. (2006) found that expression, affinity, and stability of some HLA-E alleles were associated with the success of pregnancy.
Finally, we identified two genes (AVP, arginine vasopressin and PPP1R1B, protein phosphatase 1 regulatory (inhibitor) subunit 1B) which lose DNA methylation during pregnancy and are linked to “regulation of female receptivity.” These genes are indeed fascinating since they are associated with maternal-infant bonding. Indeed, AVP is a neuropeptide with crucial regulatory functions in a wide spectrum of socio-emotional and cognitive processes in humans and animals. Research on prairie voles has highlighted the role of AVP in attachment and has also shown its epigenetic regulation (Sadino and Donaldson, 2018). PPP1R1B, also called DARPP-32, plays an essential role in mediating dopamine effects. Moreover, maternal neglect was linked to dysregulation of dopamine transmission, associated itself with a modified DARPP-32 phosphorylation pattern (Scheggi et al., 2018). The demethylation of these two genes during pregnancy indicates that they are probably highly expressed before delivery to enhance the capacity for nursing and maternal care of the newborn. It is important to note, however, that our observations were made in blood, so we can only speculate that this is also true in the brain, where these two genes are expressed.
Several studies have previously identified age-associated changes in DNA methylation in blood cells (Hannum et al., 2013; Horvath, 2013; Johansson et al., 2013; Florath et al., 2014), which led to the development of epigenetic clocks (Horvath and Raj, 2018). The small age range used in our study likely explains the lack of correlation observed in our cohort between age and DNA methylation since 19 of our 57 identified CpGs have previously been associated with chronological age in a large cohort of individuals aged 14–94 years (Johansson et al., 2013). Similarly, many studies have reported BMI-related differential methylation CpG sites, whereas no correlation was found in our cohort (Dick et al., 2014; Aslibekyan et al., 2015; Demerath et al., 2015; Huang et al., 2015; Fradin et al., 2017; Mendelson et al., 2017). Only cg17501210, associated with methylation changes during pregnancy in our study, was linked to BMI in three independent cohorts (ARIC: Atherosclerosis Risk in Communities, GOLDN: Genetics of Lipid Lowering Drugs and Diet Network, and PIVUS: Prospective Investigation of the Vasculature in Uppsala Seniors) (Mendelson et al., 2017).
Consistently, using the same EPIC BeadChip technology, Gruzieva et al. (2019) also observed DNA methylation changes at several CpGs within MIR10A, LOC100996291, and AVP in 21 pregnant women. Only one other study investigated DNA methylation in maternal blood during pregnancy and, by contrast, showed that DNA methylation becomes stable over time. However, they analyzed approximately 200 000 CpGs (Chen et al., 2017). We cannot exclude the possibility that our identified CpGs were not present in their less covering array.
Our study has several limitations. The first limitation is the small sample size, which can produce false-positive results. However, the fact that some identified CpGs were previously described in the only other study investigating DNA methylation changes in maternal blood during pregnancy reinforces our results. The second limitation is that we conducted our analysis by considering the blood cell heterogeneity but not fetal cells that may be present in maternal circulation, although in very low proportions.
Altogether, our results showed that DNA methylation may play a role not only in maternal adaptations during pregnancy and preparation for delivery but also in adaptation to care for the newborn.
Data availability statement
The datasets presented in this study can be found in online repositories. The names of the repository/repositories and accession number(s) can be found at: https://www.ncbi.nlm.nih.gov/geo/, GSE224339.
Ethics statement
The studies involving human participants were reviewed and approved by CPP C0-14-001, CNIL No. C13–61. The patients/participants provided their written informed consent to participate in this study.
Author contributions
FL, GA, and AB recruited pregnant women and retrieved blood samples and clinical data. CM performed sample preparations. JT and FB performed DNA methylation arrays and quality step analyses. DF carried out DNA methylation analysis with the help of JT. DF wrote the main manuscript text and prepared figures. JT, FB, AB, and GA provided intellectual input for the discussion and critically revised the manuscript. All authors contributed to the article and approved the submitted version.
Funding
This study was funded by the Agence Nationale de la Recherche (Project-ANR-12-DSSA-0006 Epichild).
Conflict of interest
The authors declare that the research was conducted in the absence of any commercial or financial relationships that could be construed as a potential conflict of interest.
Publisher’s note
All claims expressed in this article are solely those of the authors and do not necessarily represent those of their affiliated organizations, or those of the publisher, the editors, and the reviewers. Any product that may be evaluated in this article, or claim that may be made by its manufacturer, is not guaranteed or endorsed by the publisher.
Supplementary material
The Supplementary Material for this article can be found online at: https://www.frontiersin.org/articles/10.3389/fcell.2023.1185311/full#supplementary-material
References
Aslibekyan, S., Demerath, E. W., Mendelson, M., Zhi, D., Guan, W., Liang, L., et al. (2015). Epigenome-wide study identifies novel methylation loci associated with body mass index and waist circumference. Obesity 23, 1493–1501. doi:10.1002/oby.21111
Bjornsson, H. T., Sigurdsson, M. I., Fallin, M. D., Irizarry, R. A., Aspelund, T., Cui, H., et al. (2008). Intra-individual change over time in DNA methylation with familial clustering. JAMA 299, 2877–2883. [pii]. doi:10.1001/jama.299.24.2877
Chen, S., Mukherjee, N., Janjanam, V. D., Arshad, S. H., Kurukulaaratchy, R. J., Holloway, J. W., et al. (2017). Consistency and variability of DNA methylation in women during puberty, young adulthood, and pregnancy. Genet. Epigenet 9, 1179237X17721540. doi:10.1177/1179237X17721540
Demerath, E. W., Guan, W., Grove, M. L., Aslibekyan, S., Mendelson, M., Zhou, Y.-H., et al. (2015). Epigenome-wide association study (EWAS) of BMI, BMI change and waist circumference in African American adults identifies multiple replicated loci. Hum. Mol. Genet. 24, 4464–4479. doi:10.1093/hmg/ddv161
Dick, K. J., Nelson, C. P., Tsaprouni, L., Sandling, J. K., Aissi, D., Wahl, S., et al. (2014). DNA methylation and body-mass index: A genome-wide analysis. Lancet 383, 1990–1998. doi:10.1016/S0140-6736(13)62674-4
Feinberg, A. P., Irizarry, R. A., Fradin, D., Aryee, M. J., Murakami, P., Aspelund, T., et al. (2010). Personalized epigenomic signatures that are stable over time and covary with body mass index. Sci. Transl. Med. 2, 49ra67. doi:10.1126/scitranslmed.3001262
Flanagan, J. M., Brook, M. N., Orr, N., Tomczyk, K., Coulson, P., Fletcher, O., et al. (2015). Temporal stability and determinants of white blood cell DNA methylation in the breakthrough generations study. Cancer Epidemiol. Biomarkers Prev. 24, 221–229. doi:10.1158/1055-9965.EPI-14-0767
Florath, I., Butterbach, K., Müller, H., Bewerunge-Hudler, M., and Brenner, H. (2014). Cross-sectional and longitudinal changes in DNA methylation with age: An epigenome-wide analysis revealing over 60 novel age-associated CpG sites. Hum. Mol. Genet. 23, 1186–1201. doi:10.1093/hmg/ddt531
Fradin, D., Boëlle, P.-Y., Belot, M.-P., Lachaux, F., Tost, J., Besse, C., et al. (2017). Genome-wide methylation analysis identifies specific epigenetic marks in severely obese children. Sci. Rep. 7, 46311. doi:10.1038/srep46311
Gruzieva, O., Merid, S. K., Chen, S., Mukherjee, N., Hedman, A. M., Almqvist, C., et al. (2019). DNA methylation trajectories during pregnancy. Epigenet Insights 12, 2516865719867090. doi:10.1177/2516865719867090
Hannum, G., Guinney, J., Zhao, L., Zhang, L., Hughes, G., Sadda, S., et al. (2013). Genome-wide methylation profiles reveal quantitative views of human aging rates. Mol. Cell 49, 359–367. doi:10.1016/j.molcel.2012.10.016
Horvath, S. (2013). DNA methylation age of human tissues and cell types. Genome Biol. 14, R115. doi:10.1186/gb-2013-14-10-r115
Horvath, S., and Raj, K. (2018). DNA methylation-based biomarkers and the epigenetic clock theory of ageing. Nat. Rev. Genet. 19, 371–384. doi:10.1038/s41576-018-0004-3
Huang, R. C., Garratt, E. S., Pan, H., Wu, Y., Davis, E. A., Barton, S. J., et al. (2015). Genome-wide methylation analysis identifies differentially methylated CpG loci associated with severe obesity in childhood. Epigenetics 10, 995–1005. doi:10.1080/15592294.2015.1080411
Johansson, Å., Enroth, S., and Gyllensten, U. (2013). Continuous aging of the human DNA methylome throughout the human lifespan. PLOS ONE 8, e67378. doi:10.1371/journal.pone.0067378
Lam, L. L., Emberly, E., Fraser, H. B., Neumann, S. M., Chen, E., Miller, G. E., et al. (2012). Factors underlying variable DNA methylation in a human community cohort. Proc. Natl. Acad. Sci. U. S. A. 109, 17253–17260. doi:10.1073/pnas.1121249109
Lecce, L., Lindsay, L. A., and Murphy, C. R. (2011). Ezrin and EBP50 redistribute apically in rat uterine epithelial cells at the time of implantation and in response to cell contact. Cell Tissue Res. 343, 445–453. doi:10.1007/s00441-010-1088-z
Mendelson, M. M., Marioni, R. E., Joehanes, R., Liu, C., Hedman, Å. K., Aslibekyan, S., et al. (2017). Association of body mass index with DNA methylation and gene expression in blood cells and relations to cardiometabolic disease: A mendelian randomization approach. PLOS Med. 14, e1002215. doi:10.1371/journal.pmed.1002215
Sadino, J. M., and Donaldson, Z. R. (2018). Prairie voles as a model for understanding the genetic and epigenetic regulation of attachment behaviors. ACS Chem. Neurosci. 9, 1939–1950. doi:10.1021/acschemneuro.7b00475
Scheggi, S., De Montis, M. G., and Gambarana, C. (2018). DARPP-32 in the orchestration of responses to positive natural stimuli. J. Neurochem. 147, 439–453. doi:10.1111/jnc.14558
Tian, Y., Morris, T. J., Webster, A. P., Yang, Z., Beck, S., Feber, A., et al. (2017). ChAMP: Updated methylation analysis pipeline for Illumina BeadChips. Bioinformatics 33, 3982–3984. doi:10.1093/bioinformatics/btx513
Tripathi, P., Naik, S., and Agrawal, S. (2006). HLA-E and immunobiology of pregnancy. Tissue Antigens 67, 207–213. doi:10.1111/j.1399-0039.2005.00550.x
Keywords: DNA methylation, pregnancy, changes, bonding, immunity
Citation: Fradin D, Tost J, Busato F, Mille C, Lachaux F, Deleuze J-F, Apter G and Benachi A (2023) DNA methylation dynamics during pregnancy. Front. Cell Dev. Biol. 11:1185311. doi: 10.3389/fcell.2023.1185311
Received: 13 March 2023; Accepted: 04 May 2023;
Published: 22 May 2023.
Edited by:
Hehuang Xie, Virginia Tech, United StatesReviewed by:
Jacob Peedicayil, Christian Medical College & Hospital, IndiaMichelle Holland, King’s College London, United Kingdom
Copyright © 2023 Fradin, Tost, Busato, Mille, Lachaux, Deleuze, Apter and Benachi. This is an open-access article distributed under the terms of the Creative Commons Attribution License (CC BY). The use, distribution or reproduction in other forums is permitted, provided the original author(s) and the copyright owner(s) are credited and that the original publication in this journal is cited, in accordance with accepted academic practice. No use, distribution or reproduction is permitted which does not comply with these terms.
*Correspondence: Delphine Fradin, ZGVscGhpbmUuZnJhZGluQGluc2VybS5mcg==
†Present address: Delphine Fradin, Nantes Université, INSERM UMR 1307, CNRS UMR 6075, Université d’Angers, CRCI2NA, Nantes, France