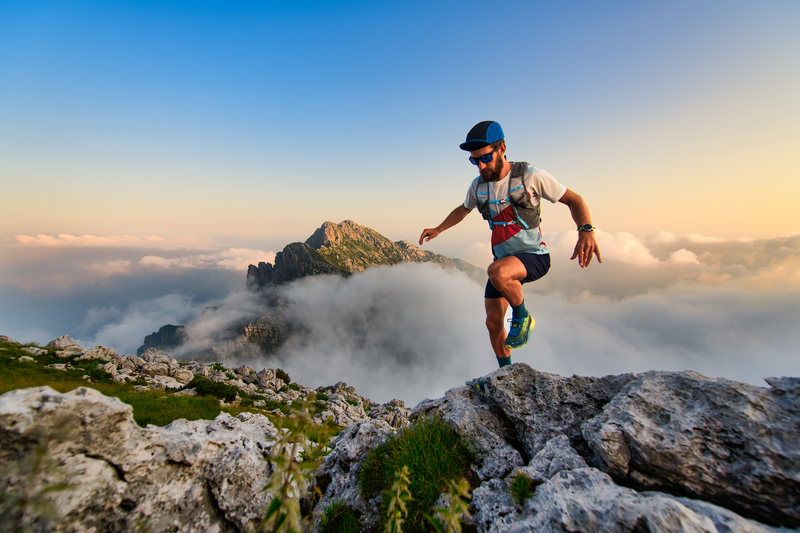
94% of researchers rate our articles as excellent or good
Learn more about the work of our research integrity team to safeguard the quality of each article we publish.
Find out more
ORIGINAL RESEARCH article
Front. Cell Dev. Biol. , 15 May 2023
Sec. Molecular and Cellular Pathology
Volume 11 - 2023 | https://doi.org/10.3389/fcell.2023.1174936
This article is part of the Research Topic Artificial Intelligence Applications in Chronic Ocular Diseases View all 31 articles
Background: Artificial intelligence (AI) is used in ophthalmological disease screening and diagnostics, medical image diagnostics, and predicting late-disease progression rates. We reviewed all AI publications associated with macular edema (ME) research Between 2011 and 2022 and performed modeling, quantitative, and qualitative investigations.
Methods: On 1st February 2023, we screened the Web of Science Core Collection for AI applications related to ME, from which 297 studies were identified and analyzed (2011–2022). We collected information on: publications, institutions, country/region, keywords, journal name, references, and research hotspots. Literature clustering networks and Frontier knowledge bases were investigated using bibliometrix-BiblioShiny, VOSviewer, and CiteSpace bibliometric platforms. We used the R “bibliometrix” package to synopsize our observations, enumerate keywords, visualize collaboration networks between countries/regions, and generate a topic trends plot. VOSviewer was used to examine cooperation between institutions and identify citation relationships between journals. We used CiteSpace to identify clustering keywords over the timeline and identify keywords with the strongest citation bursts.
Results: In total, 47 countries published AI studies related to ME; the United States had the highest H-index, thus the greatest influence. China and the United States cooperated most closely between all countries. Also, 613 institutions generated publications - the Medical University of Vienna had the highest number of studies. This publication record and H-index meant the university was the most influential in the ME field. Reference clusters were also categorized into 10 headings: retinal Optical Coherence Tomography (OCT) fluid detection, convolutional network models, deep learning (DL)-based single-shot predictions, retinal vascular disease, diabetic retinopathy (DR), convolutional neural networks (CNNs), automated macular pathology diagnosis, dry age-related macular degeneration (DARMD), class weight, and advanced DL architecture systems. Frontier keywords were represented by diabetic macular edema (DME) (2021–2022).
Conclusion: Our review of the AI-related ME literature was comprehensive, systematic, and objective, and identified future trends and current hotspots. With increased DL outputs, the ME research focus has gradually shifted from manual ME examinations to automatic ME detection and associated symptoms. In this review, we present a comprehensive and dynamic overview of AI in ME and identify future research areas.
Macular edema (ME) is a common, critical disease caused by retinal vein occlusion, diabetic retinopathy (DR), chronic uveitis, and eye injury, of which, macular lesions are the leading cause of disease. Clinically significant ME is manifested by retinal thickening which impacts the macula center, is defined by central retinal thickness >250–300 μm, and examined using Optical Coherence Tomography (OCT) (Hee et al., 1995). ME also involves fluid accumulation in retinal layers which is a common morphological manifestation in different retinal diseases (Daruich et al., 2018). Therefore, it is vital to quantitatively analyze ME research areas, the disease status quo, and future prospects related to disease progression.
Bibliometrics is used to analyze different knowledge carriers using mathematics and statistics (Cancino et al., 2017). It evaluates development trends in target disciplines/scientific fields by analyzing database and document characteristics to identify research hotspots and key research directions. In recent years, bibliometric analysis have been successfully used in orthopedics, ophthalmology, and gynecology (Qiu et al., 2018; Huang et al., 2020; He J. et al., 2021). Additionally, the approach is invaluable for writing guidelines, making clinical decisions, and importantly, treating different diseases. However, bibliometric analyses related to ME in ophthalmology remains under-studied (Narotsky et al., 2012; Seriwala et al., 2015; Khan et al., 2016), therefore, we systematically investigated this research area to characterize the status quo and identify research hotspots.
On 1st February 2023, we downloaded data from the Web of Science Core Collection (2011–2022) using: “machine learning” OR “deep learning” OR “convolutional neural network*” OR “CNN*” OR “Recurrent neural network*” OR “RNN” OR “Fully Convolutional Network*” OR “FCN*” search terms. The parallel search subject was ME and relevant studies included basic information on: authors, abstracts, keywords, titles, institutions, journals, countries/regions, and references. Indexed database studies were included, but meeting abstracts, book chapters, data papers, proceedings, editorials, and repeat articles, and unpublished studies containing limited data were excluded. A summary of this process is shown (Figure 1).
FIGURE 1. Study flow chart showing bibliometric analyses and selection criteria of macular edema studies.
We also examined publication characteristics: keywords, institutions, countries/regions, and journals. We also used the H-index which evaluates the scientific value of research and measures author/journal scientific productivity (Eyre-Walker and Stoletzki, 2013). To represent collaborative networks across journals/institutes/countries/keywords and facilitate co-occurrence investigations, we used R language bibliometrics software (Massimo Aria and Corrado Cuccurullo), CiteSpace (Drexel University, PA, United States), and VOSviewer (Leiden University, Holland). The R language bibliometric package is widely used in statistical computation and graphics (Aria and Cuccurullo, 2017) and was used to extract the top 10 keywords and cluster them into themes/evolution/hierarchical clustering/topic trends. From collaborative data, we used the VOSviewer to provide a comprehensive and detailed view of bibliometric maps. We also generated a cooperation relationship diagram between institutions and also a reference relationship diagram between foresight to analyze cooperation outputs between institutions and reference relationships between disciplines. CiteSpace was used to investigate knowledge from the literature and visualize data (Chen, 2004). We also generated knowledge maps, performed discipline evolution analyses, and determined burst keywords (BKs) to identify recurrent keywords.
We observed that AI in ME research commenced in 2011. From 2011 to 2022, we identified 297 papers and identified AI-associated ME publication trends (Figure 2). While this type of research emerged in 2011, it fell silent from 2012 to 2015. However, from 2016, in-depth learning approaches combined with ophthalmology led to increased ME research outputs, and paper outputs increased year on year suggesting an important research trend had been established.
Forty-seven countries/regions published ME studies - the top 10 countries (Table 1) and collaborations (Figures 3, 4) are indicated. China published the most studies (101), then the United States (73), India (48), and the United Kingdom (23). Some countries (United States, China, and the United Kingdom) showed high centrality (dark blue—Figure 3) suggesting important regional roles in/contributions to ME research. H index is a mixed quantitative index, which can be used to evaluate the quantity and level of academic output of a country or institution. Because the United States has the highest H-index, it has the greatest influence in the field of macular edema.
FIGURE 3. Collaboration map showing how countries/regions contributed to/collaborated on macular edema publications.
FIGURE 4. Diagram showing how the most cited countries/regions contributed to/collaborated on macular edema publications.
In total, 613 institutes generated ME publications; the top ten are shown (Table 1). Institutions are also outlined (Figure 5). The Medical University of Vienna had the most publications (15), followed by the National University of Singapore (12), the University of California (12), and the Singapore National Eye Center (11).
We also cataloged research institutions with outstanding contributions to the ME field (Figure 5) using VOSviewer. Regional institutional distributions showed distinct aggregation effects, indicating that academic research was concentrated to a few countries. From a literature perspective, most institutions were based in universities and scientific research institutions and generally reflected the ME research status. A possible reason could be that the ME research field is highly academic in nature and not currently economically feasible, thus enterprises and other institutions may currently eschew the field. The Medical University of Vienna and the National University of Singapore were major prominent organizations which had significant ME research outputs.
Across all academic fields, knowledge exchange in/between fields is often reflected in reference relationships between academic journals. Citing papers are knowledge frontiers, while referenced papers are knowledge bases. As indicated (Figure 6; Table 2), the major journals contributing to ME research included, Biomed Opt Express, Ieee T Med Imaging, Ophthalmology, Med Image Anal, and Invest Ophth Vis Sci—with high centrality, these were the most popular journals publishing ME research.
A journal dual-map overlay (Figure 7) showed citing (left) and cited (right) journals, while citation associations were indicated by colored lines—these investigations demonstrated that studies in computer/medicine/molecular journals were typically cited in ophthalmology/mathematics/clinical journals.
Reference analysis is an important index in bibliometric—typically, often-cited studies significantly impact certain research areas. Therefore, we used Citespace to cluster references from data to generate a reference clustering diagram (with a timeline) to analyze ME research.
A co-citation reference network was used to assess the relevance of studies (Figure 8). Cluster setting: g-index K = 5 and #years/slice = 1. The modularity Q score was 0.8306 (>0.5), thus the network was adequately split into loosely coupled clusters. The weighted mean silhouette score was 0.9621 (>0.5), thus cluster homogeneity was reasonable.
Index items, as cluster markers, were extracted from studies. The largest clusters were cluster #0 “retinal oct fluid detection” (Lee et al., 2017; Venhuizen et al., 2018; Girish et al., 2019), cluster #1 “convolutional network model” (Gargeya and Leng, 2017; Porwal et al., 2020; Singh and Gorantla, 2020; Dai et al., 2021), cluster #2 “deep learning-based single-shot prediction” (Rasti et al., 2018; Das et al., 2019; Tsuji et al., 2020), cluster #3 “retinal vascular disease” (Karri et al., 2017; Ehlers et al., 2019; Figueiredo et al., 2020; Rasti et al., 2020), cluster #4 “diabetic retinopathy” (Ting et al., 2017; Raumviboonsuk et al., 2019; Ting et al., 2019), cluster #5 “convolutional neural network” (Kermany et al., 2018; Hwang et al., 2019), cluster #6 “automated macular pathology diagnosis” (Chang and Lin, 2011), cluster #7 “dry age-related macular degeneration” (Kafieh et al., 2013; Srinivasan et al., 2014a; Rathke et al., 2014; Karri et al., 2016), cluster #8 “class weight”(Wan et al., 2018; Li et al., 2019b; Huang et al., 2019), and cluster #9 “recent advanced deep learning architecture” (Schmidt-Erfurth et al., 2018; Bogunovic et al., 2019; Gu et al., 2019; Lu et al., 2019).
Keyword analyses help summarize research themes and explore research hotspots and trends in a given field. The top 20 keywords from ME studies are shown (Table 3). Temporal trend/hotspot shifts (from seven keywords with the strongest citation burst) in 2016–2019; BKs were Image Analysis (2016–2019), OCT Imaging (2017–2019), Layer Segmentation (2017–2019), and Age Related Macular Degeneration (AMD) (2017–2019). BKs in 2020–2022 were validation (2020), system (2020), and the hotspot, Diabetic Macular Edema (DME) (2021–2022). (Figure 9).
Between 2011 and 2022, 297 ME studies, conforming to inclusion/exclusion criteria and search terms, were identified. China generated the most studies (101, 34.007%), with the United States in second place (73, 24.579%). Two of the top ten institutions were in China. The most common ME publication journal, and the major contributor to ME research, was BIOMEDICAL OPTICS EXPRESS. The top study (cited 2,936 times) was by Gulshan et al. in JAMA-JOURNAL OF THE AMERICAN MEDICAL ASSOCIATION (Gulshan et al., 2016). The second top study (cited 1,474 times) was by Kermany et al. in CELL (Kermany et al., 2018).
Previously DL-related technologies and associations with ME generated several major achievements. When co-cited references were clustered (Figure 8), key clustering nodes were used to identify knowledge bases in ME research: #0 “retinal oct fluid detection,” #1 “convolutional network model,” #2 “deep learning-based single-shot prediction,” #3 “retinal vascular disease,” #4 “diabetic retinopathy,” #5 “convolutional neural network,” #6 “automated macular pathology diagnosis,” #7 “dry age-related macular degeneration,” #8 “class weight,” and #9 “recent advanced deep learning architecture.” In the following sections, we outline knowledge bases according to different clusters.
#0 “Retinal OCT fluid detection”; Lee et al. (2017) generated a model formulated on encoding and decoding mechanism and outlined improved segmentation intraretinal fluid (IRF) methods, which showed good IRF segmentation results in OCT images. Roy et al. (2017) developed an AI approach (Relay Net) which segmented multiple retinal layers and generated fluid bag descriptions in OCT eye images. The model displayed excellent performance in object segmentation. Fundus dropsy can lead to ME. These methods were used to segment retinal fundus dropsy and automatically detected ME in OCT and affected segmented parts.
#1 “Convolutional network model”; Gargeya and Leng. (2017) designed a convolution network model to facilitate automatic DR recognition. Abramoff et al. (2016) compared this convolutional network with other automatic detection methods (IDx-DR X2.1) to automatically detect DR, mainly evaluating the analysis software that IDx-DR X2.1 runs on the server maintained and controlled by IDx. Porwal et al. (2020) generated a dataset for Indian populations with DR which provided normal retinal and typical DR structures at pixel levels. Image information was also provided for DR and DME severity, and facilitated image algorithm development and evaluations for early DR detection. DR is one of the most common diabetic microvascular complications. Retinal microvascular leakage and occlusions caused by chronic progressive diabetes causes different fundus diseases. DR is one of the main inducements of ME. Importantly, automated DR screening combined with a convolutional network model can effectively and economically prevent ME.
#2 “Deep learning-based single-shot prediction”; Rasti et al. (2018) used a DL-based single-shot prediction method (MCME) to predict macular OCT categories. The model performed category predictions based on minimum preprocessing requirements and helped to automatically classify macular OCT in clinical settings. The method by Srinivasan et al. (2014b) automatically detected diabetic ME and DARMD in OCT images. Using Histogram of Oriented Gradient descriptors and SVMs to classify spectral domain-OCT images, the method may be used to remotely diagnose some ophthalmic diseases.
#3 “Retinal vascular disease”; Karri et al. (2017) used a DL technique to identify DME or DARMD in retinal vascular diseases from OCT images. The strategy used transfer learning and the pre-trained GoogLeNet as a classification model to allow for faster convergence with less data. Li et al. (2019a) combined four classification models to automatically detect four retinal vascular diseases in OCT images: choroidal neovascularization, DME, DRUSEN, and NORMAL. The method had a classification accuracy = 0.973, which met or exceeded ophthalmologist expectations.
#4 “Diabetic retinopathy”; a DL system by Ting et al. (2017) was used to rapidly and accurately screen DR and related eye diseases. Abramoff et al. (2018) diagnostically evaluated an autonomous AI system (mtmDR) to automatically detect DR and DME; the approach improved early DR detection rates and reduced pain induced by vision loss and blindness. Thus, to some extent, these methods helped limit ME.
#5 “CNN” is one of the representative DL algorithms (Gu et al., 2018). Gulshan et al. (2016) developed a CNN algorithm to detect DR in retinal fundus images and detect referential diagnostic retinopathy. Kermany et al. (2018) formulated an effective transfer learning algorithm which processed medical images and identified key pathology traits in images. The algorithm was primarily used to analyze retinal OCT images, while combination with a CNN helped clinicians effectively diagnose ME.
#6 “Automated macular pathology diagnosis”; Chang and Lin (2011) introduced a software package for SVM algorithms—the LIBSVM library—which is one of the most widely used SVM software programs. The algorithm effectively supported automatic macular pathological diagnoses using SVM.
#7 “Dry age-related macular degeneration”; Chiu et al. (2015) designed a method to automatically segment diabetic ME in OCT images. The authors first estimated fluid and retinal layer positions using a classification method based on kernel regression, and then used classification estimates to accurately segment retinal layer boundaries using dynamic programming frameworks and graph theory. The method was the first to be validated, fully-automated, seven-layered, and fluid segmented for analyzing severe real-world DME images. Karri et al. (2016) generated a structured learning algorithm which enhanced layer specific-edge detection in OCT retinal images. Simultaneously, the algorithm identified layers and corresponding edges so that layer-specific edge computation were calculated to within 1 s.
#8 “Class weight”; Huang et al. (2019) formulated a layer-guided CNN to classify OCT retinal images. The method was divided into; 1), a segmentation network (ReLayNet) extracted segmentation maps from retinal layers, and 2), two disease related layers (RPE-BrM and ILM-RPE) were taken from layer segmentation graphs. The network may be applied to other retinal diseases (macular hole and macular telangiectasia) and also retinopathy detection and segmentation.
#9 “Recent advances in deep learning architecture”; Bogunovic et al. (2019) reviewed the standards and models used in retinal OCT fluid detection and segmentation, and showed that >50% of clinical teams selected UNet and its derivative model structure as a basic network architecture to segment OCT images. Schlegl et al. (2018) generated a DL strategy to automatically quantify and detect subretinal fluid (SRF) and intra-retinal cystic fluid (IRC). The method included a CNN with encoder/decoder architecture, which identified IRC and SRF. In the ME research field, codec structures (similar to UNet) have become popular DL network architecture approaches.
Keywords typically highlight research ideas, while BKs reflect research frontiers and trends. Citespace captured BKs and identified ME research frontiers; e.g., DME in 2021–2022. We forecast these words will highlight future research frontiers ME research.
DME represents retinal thickening or hard exudative deposition caused by extracellular fluid accumulation in the optic disc diameter, in the macular fovea. OCT image are important tools for diagnosing diabetic macular disease, and AI-related methods for identifying and segmenting disease related ME diabetes in OCT image are key modalities for clinicians who treat and screen diseases and help reduce medical costs.
When AI correlation methods were used to assess OCT images, (Sunija et al., 2021; Nazir et al., 2021; Tayal et al., 2021; Wu et al., 2021; Atteia et al., 2021) used DL to automatically recognize ME-related lesions in OCT images. Similarly, He et al. provided accurate image support for doctors when diagnosing ME by layering retinas in images.
Sunija et al. (2021) used a lightweight DL algorithms to determine if patients had DME from OCT images. The algorithm network comprised six deep CNN layers and had accuracy and recall rates of 99.69% and 99.69%, respectively.
He Y. et al. (2021) formulated a unified framework for segmenting structured layer surfaces which generated continuous structured and smooth layer surfaces, with ordered topology, in an end-to-end DL strategy. DME was effectively observed by layering the retinal surface, and generating sub-pixel surface positions in single feed-forward propagation with full connection layers, thereby improving segmentation accuracy.
Nazir et al. (2021) proposed an automatic DR and DME screening method. The algorithm used DenseNet-100 as the basic CNN architecture and was greatly improved. The approach also extracted representative information from low-intensity/noisy images and accurately classified them.
The diagnostic method by Tayal et al. (2021) automatically detected DME and used three different CNN models (five, seven, and nine layer approaches) to classify and recognize four eye diseases. The strategy generated high F1 scores, accuracy and sensitivity outputs, and greatly reduced detection times.
Wu et al. (2021) developed a DL model to detect morphological DME patterns based on OCT images using a VGG-16 network strategy. The model was trained using ME manifestations in OCT images (diffused retinal thickening, cystoid ME, and serous retinal detachment) and greatly facilitated disease diagnostics.
Atteia et al. (2021) formulated a transfer-based stacked autoencoder neural network system, which used four standard pre-training depth networks to extract information from small input datasets. With a maximum classification accuracy = 96.8% and specificity = 95.5%, the approach allowed clinicians to automatically detect and diagnose DME.
We performed a bibliometric investigation of ME research related to DL, machine learning, FCN, CNN, RNN, and other AI fields. We identified the ME knowledge base, future trends, and current research hotspots. The knowledge base included: retinal OCT fluid detection, convolutional network models, DL-based single-shot predictions, retinal vascular disease, DR, CNNs, automated macular pathology diagnosis, DARMD, class weight, and recent advances in DL architecture. DME was also identified as a future research trend and Frontier. The current research focuses on disease classification in OCT images, segmentation and segmentation of disease regions based on OCT images.
Our study had some limitations; we only identified studies between 2011 and 2022, therefore, some research may have been missed, thus we possibly and inadvertently introduced publication bias into our investigation which impacted our conclusion.
The datasets presented in this study can be found in online repositories. The names of the repository/repositories and accession number(s) can be found in the article/Supplementary Material.
WY, SZ, ZZ, HF, and YL conceived the research and designed the research method. HF and JC have prepared and standardized the contents and chapters. JC searched the references cited in the article. All authors contributed to the article and approved the submitted version.
Supported by the Shenzhen Fund for Guangdong Provincial High-level Clinical Key Specialties (SZGSP014), Sanming Project of Medicine in Shenzhen (SZSM202011015) and Shenzhen Science and Technology Planning Project (KCXFZ20211020163813019).
The authors declare that the research was conducted in the absence of any commercial or financial relationships that could be construed as a potential conflict of interest.
All claims expressed in this article are solely those of the authors and do not necessarily represent those of their affiliated organizations, or those of the publisher, the editors and the reviewers. Any product that may be evaluated in this article, or claim that may be made by its manufacturer, is not guaranteed or endorsed by the publisher.
The Supplementary Material for this article can be found online at: https://www.frontiersin.org/articles/10.3389/fcell.2023.1174936/full#supplementary-material
Abramoff, M. D., Lavin, P. T., Birch, M., Shah, N., and Folk, J. C. (2018). Pivotal trial of an autonomous AI-based diagnostic system for detection of diabetic retinopathy in primary care offices. NPJ Digit. Med. 1, 39. doi:10.1038/s41746-018-0040-6
Abramoff, M. D., Lou, Y., Erginay, A., Clarida, W., Amelon, R., Folk, J. C., et al. (2016). Improved automated detection of diabetic retinopathy on a publicly available dataset through integration of deep learning. Invest. Ophthalmol. Vis. Sci. 57 (13), 5200–5206. doi:10.1167/iovs.16-19964
Aria, M., and Cuccurullo, C. (2017). Bibliometrix: An R-tool for comprehensive science mapping analysis. J. Inf. 11 (4), 959–975. doi:10.1016/j.joi.2017.08.007
Atteia, G., Abdel Samee, N., and Zohair Hassan, H. (2021). DFTSA-Net: Deep feature transfer-based stacked autoencoder network for DME diagnosis. Entropy (Basel) 23 (10), 1251. doi:10.3390/e23101251
Bogunovic, H., Venhuizen, F., Klimscha, S., Apostolopoulos, S., Bab-Hadiashar, A., Bagci, U., et al. (2019). Retouch: The retinal OCT fluid detection and segmentation benchmark and challenge. IEEE Trans. Med. Imaging 38 (8), 1858–1874. doi:10.1109/TMI.2019.2901398
Cancino, C., Merigó, J. M., Coronado, F., Dessouky, Y., and Dessouky, M. (2017). Forty years of computers and industrial engineering: A bibliometric analysis. Comput. Industrial Eng. 113, 614–629. doi:10.1016/j.cie.2017.08.033
Chang, C.-C., Lin, C.-J., McQuoid, D. R., Messer, D. F., Taylor, W. D., Singh, K., et al. (2011). Reduction of dorsolateral prefrontal cortex gray matter in late-life depression. Psychiatry Res. 193 (3), 1–6. doi:10.1016/j.pscychresns.2011.01.003
Chen, C. (2004). Searching for intellectual turning points: Progressive knowledge domain visualization. Proc. Natl. Acad. Sci. U. S. A. 101, 5303–5310. doi:10.1073/pnas.0307513100
Chiu, S. J., Allingham, M. J., Mettu, P. S., Cousins, S. W., Izatt, J. A., and Farsiu, S. (2015). Kernel regression based segmentation of optical coherence tomography images with diabetic macular edema. Biomed. Opt. Express 6 (4), 1172–1194. doi:10.1364/BOE.6.001172
Dai, L., Wu, L., Li, H., Cai, C., Wu, Q., Kong, H., et al. (2021). A deep learning system for detecting diabetic retinopathy across the disease spectrum. Nat. Commun. 12 (1), 3242. doi:10.1038/s41467-021-23458-5
Daruich, A., Matet, A., Moulin, A., Kowalczuk, L., Nicolas, M., Sellam, A., et al. (2018). Mechanisms of macular edema: Beyond the surface. Prog. Retin Eye Res. 63, 20–68. doi:10.1016/j.preteyeres.2017.10.006
Das, V., Dandapat, S., and Bora, P. K. (2019). Multi-scale deep feature fusion for automated classification of macular pathologies from OCT images. Biomed. Signal Process. Control 54, 101605. doi:10.1016/j.bspc.2019.101605
Ehlers, J. P., Jiang, A. C., Boss, J. D., Hu, M., Figueiredo, N., Babiuch, A., et al. (2019). Quantitative ultra-widefield angiography and diabetic retinopathy severity: An assessment of panretinal leakage index, ischemic index and microaneurysm count. Ophthalmology 126 (11), 1527–1532. doi:10.1016/j.ophtha.2019.05.034
Eyre-Walker, A., and Stoletzki, N. (2013). The assessment of science: The relative merits of post-publication review, the impact factor, and the number of citations. PLoS Biol. 11 (10), e1001675. doi:10.1371/journal.pbio.1001675
Figueiredo, N., Srivastava, S. K., Singh, R. P., Babiuch, A., Sharma, S., Rachitskaya, A., et al. (2020). Longitudinal panretinal leakage and ischemic indices in retinal vascular disease after aflibercept therapy: The PERMEATE study. Ophthalmol. Retina 4 (2), 154–163. doi:10.1016/j.oret.2019.09.001
Gargeya, R., and Leng, T. (2017). Automated identification of diabetic retinopathy using deep learning. Ophthalmology 124 (7), 962–969. doi:10.1016/j.ophtha.2017.02.008
Girish, G. N., Thakur, B., Chowdhury, S. R., Kothari, A. R., and Rajan, J. (2019). Segmentation of intra-retinal cysts from optical coherence tomography images using a fully convolutional neural network model. IEEE J. Biomed. Health Inf. 23 (1), 296–304. doi:10.1109/JBHI.2018.2810379
Gu, J., Wang, Z., Kuen, J., Ma, L., Shahroudy, A., Shuai, B., et al. (2018). Recent advances in convolutional neural networks. Pattern Recognit. 77, 354–377. doi:10.1016/j.patcog.2017.10.013
Gu, Z., Cheng, J., Fu, H., Zhou, K., Hao, H., Zhao, Y., et al. (2019). CE-Net: Context encoder network for 2D medical image segmentation. IEEE Trans. Med. Imaging 38 (10), 2281–2292. doi:10.1109/TMI.2019.2903562
Gulshan, V., Peng, L., Coram, M., Stumpe, M. C., Wu, D., Narayanaswamy, A., et al. (2016). Development and validation of a deep learning algorithm for detection of diabetic retinopathy in retinal fundus photographs. JAMA 316 (22), 2402–2410. doi:10.1001/jama.2016.17216
He, J., He, L., Geng, B., and Xia, Y. (2021a). Bibliometric analysis of the top-cited articles on unicompartmental knee arthroplasty. J. Arthroplasty 36 (5), 1810–1818.e3. doi:10.1016/j.arth.2020.11.038
He, Y., Carass, A., Liu, Y., Jedynak, B. M., Solomon, S. D., Saidha, S., et al. (2021b). Structured layer surface segmentation for retina OCT using fully convolutional regression networks. Med. Image Anal. 68, 101856. doi:10.1016/j.media.2020.101856
Hee, M. R., Puliafito, C. A., Wong, C., Duker, J. S., Reichel, E., Rutledge, B., et al. (1995). Quantitative assessment of macular edema with optical coherence tomography. Arch. Ophthalmol. 113 (8), 1019–1029. doi:10.1001/archopht.1995.01100080071031
Huang, L., He, X., Fang, L., Rabbani, H., and Chen, X. (2019). Automatic classification of retinal optical coherence tomography images with layer guided convolutional neural network. IEEE Signal Process. Lett. 26 (7), 1026–1030. doi:10.1109/lsp.2019.2917779
Huang, X., Liu, X., Shang, Y., Qiao, F., and Chen, G. (2020). Current trends in research on bone regeneration: A bibliometric analysis. Biomed. Res. Int. 2020, 8787394. doi:10.1155/2020/8787394
Hwang, D. K., Hsu, C. C., Chang, K. J., Chao, D., Sun, C. H., Jheng, Y. C., et al. (2019). Artificial intelligence-based decision-making for age-related macular degeneration. Theranostics 9 (1), 232–245. doi:10.7150/thno.28447
Kafieh, R., Rabbani, H., Abramoff, M. D., and Sonka, M. (2013). Intra-retinal layer segmentation of 3D optical coherence tomography using coarse grained diffusion map. Med. Image Anal. 17 (8), 907–928. doi:10.1016/j.media.2013.05.006
Karri, S. P., Chakraborthi, D., and Chatterjee, J. (2016). Learning layer-specific edges for segmenting retinal layers with large deformations. Biomed. Opt. Express 7 (7), 2888–2901. doi:10.1364/BOE.7.002888
Karri, S. P., Chakraborty, D., and Chatterjee, J. (2017). Transfer learning based classification of optical coherence tomography images with diabetic macular edema and dry age-related macular degeneration. Biomed. Opt. Express 8 (2), 579–592. doi:10.1364/BOE.8.000579
Kermany, D. S., Goldbaum, M., Cai, W., Valentim, C. C. S., Liang, H., Baxter, S. L., et al. (2018). Identifying medical diagnoses and treatable diseases by image-based deep learning. Cell 172 (5), 1122–1131. doi:10.1016/j.cell.2018.02.010
Khan, M. S., Ullah, W., Riaz, I. B., Bhulani, N., Manning, W. J., Tridandapani, S., et al. (2016). Top 100 cited articles in cardiovascular magnetic resonance: A bibliometric analysis. J. Cardiovasc Magn. Reson 18 (1), 87. doi:10.1186/s12968-016-0303-9
Lee, C. S., Tyring, A. J., Deruyter, N. P., Wu, Y., Rokem, A., and Lee, A. Y. (2017). Deep-learning based, automated segmentation of macular edema in optical coherence tomography. Biomed. Opt. Express 8 (7), 3440–3448. doi:10.1364/BOE.8.003440
Li, F., Chen, H., Liu, Z., Zhang, X.-d., Jiang, M.-s., Wu, Z.-z., et al. (2019a). Deep learning-based automated detection of retinal diseases using optical coherence tomography images. Biomed. Opt. Express 10 (12), 6204–6226. doi:10.1364/boe.10.006204
Li, F., Chen, H., Liu, Z., Zhang, X., and Wu, Z. (2019b). Fully automated detection of retinal disorders by image-based deep learning. Graefes Arch. Clin. Exp. Ophthalmol. 257 (3), 495–505. doi:10.1007/s00417-018-04224-8
Lu, D., Heisler, M., Lee, S., Ding, G. W., Navajas, E., Sarunic, M. V., et al. (2019). Deep-learning based multiclass retinal fluid segmentation and detection in optical coherence tomography images using a fully convolutional neural network. Med. Image Anal. 54, 100–110. doi:10.1016/j.media.2019.02.011
Narotsky, D., Green, P. H., and Lebwohl, B. (2012). Temporal and geographic trends in celiac disease publications: A bibliometric analysis. Eur. J. Gastroenterol. Hepatol. 24 (9), 1071–1077. doi:10.1097/MEG.0b013e328355a4ab
Nazir, T., Nawaz, M., Rashid, J., Mahum, R., Masood, M., Mehmood, A., et al. (2021). Detection of diabetic eye disease from retinal images using a deep learning based CenterNet model. Sensors (Basel) 21 (16), 5283. doi:10.3390/s21165283
Porwal, P., Pachade, S., Kokare, M., Deshmukh, G., Son, J., Bae, W., et al. (2020). IDRiD: Diabetic retinopathy - segmentation and grading challenge. Med. Image Anal. 59, 101561. doi:10.1016/j.media.2019.101561
Qiu, Y., Yang, W., Wang, Q., Yan, S., Li, B., and Zhai, X. (2018). Osteoporosis in postmenopausal women in this decade: A bibliometric assessment of current research and future hotspots. Arch. Osteoporos. 13 (1), 121. doi:10.1007/s11657-018-0534-5
Rasti, R., Allingham, M. J., Mettu, P. S., Kavusi, S., Govind, K., Cousins, S. W., et al. (2020). Deep learning-based single-shot prediction of differential effects of anti-VEGF treatment in patients with diabetic macular edema. Biomed. Opt. Express 11 (2), 1139–1152. doi:10.1364/BOE.379150
Rasti, R., Rabbani, H., Mehridehnavi, A., and Hajizadeh, F. (2018). Macular OCT classification using a multi-scale convolutional neural network ensemble. IEEE Trans. Med. Imaging 37 (4), 1024–1034. doi:10.1109/TMI.2017.2780115
Rathke, F., Schmidt, S., and Schnorr, C. (2014). Probabilistic intra-retinal layer segmentation in 3-D OCT images using global shape regularization. Med. Image Anal. 18 (5), 781–794. doi:10.1016/j.media.2014.03.004
Raumviboonsuk, P., Krause, J., Chotcomwongse, P., Sayres, R., Raman, R., Widner, K., et al. (2019). Deep learning versus human graders for classifying diabetic retinopathy severity in a nationwide screening program. NPJ Digit. Med. 2, 25. doi:10.1038/s41746-019-0099-8
Roy, A. G., Conjeti, S., Karri, S. P. K., Sheet, D., Katouzian, A., Wachinger, C., et al. (2017). ReLayNet: Retinal layer and fluid segmentation of macular optical coherence tomography using fully convolutional networks. Biomed. Opt. Express 8 (8), 3627–3642. doi:10.1364/BOE.8.003627
Schlegl, T., Waldstein, S. M., Bogunovic, H., Endstrasser, F., Sadeghipour, A., Philip, A. M., et al. (2018). Fully automated detection and quantification of macular fluid in OCT using deep learning. Ophthalmology 125 (4), 549–558. doi:10.1016/j.ophtha.2017.10.031
Schmidt-Erfurth, U., Sadeghipour, A., Gerendas, B. S., Waldstein, S. M., and Bogunovic, H. (2018). Artificial intelligence in retina. Prog. Retin Eye Res. 67, 1–29. doi:10.1016/j.preteyeres.2018.07.004
Seriwala, H. M., Khan, M. S., Shuaib, W., and Shah, S. R. (2015). Bibliometric analysis of the top 50 cited respiratory articles. Expert Rev. Respir. Med. 9 (6), 817–824. doi:10.1586/17476348.2015.1103649
Singh, R. K., and Gorantla, R. (2020). DMENet: Diabetic macular edema diagnosis using hierarchical ensemble of CNNs. PLoS One 15 (2), e0220677. doi:10.1371/journal.pone.0220677
Srinivasan, P. P., Heflin, S. J., Izatt, J. A., Arshavsky, V. Y., and Farsiu, S. (2014a). Automatic segmentation of up to ten layer boundaries in SD-OCT images of the mouse retina with and without missing layers due to pathology. Biomed. Opt. Express 5 (2), 348–365. doi:10.1364/BOE.5.000348
Srinivasan, P. P., Kim, L. A., Mettu, P. S., Cousins, S. W., Comer, G. M., Izatt, J. A., et al. (2014b). Fully automated detection of diabetic macular edema and dry age-related macular degeneration from optical coherence tomography images. Biomed. Opt. Express 5 (10), 3568–3577. doi:10.1364/BOE.5.003568
Sunija, A. P., Kar, S., S, G., Gopi, V. P., and Palanisamy, P. (2021). OctNET: A lightweight CNN for retinal disease classification from optical coherence tomography images. Comput. Methods Programs Biomed. 200, 105877. doi:10.1016/j.cmpb.2020.105877
Tayal, A., Gupta, J., Solanki, A., Bisht, K., Nayyar, A., and Masud, M. (2021). DL-CNN-based approach with image processing techniques for diagnosis of retinal diseases. Multimed. Syst. 28 (4), 1417–1438. doi:10.1007/s00530-021-00769-7
Ting, D. S. W., Cheung, C. Y., Lim, G., Tan, G. S. W., Quang, N. D., Gan, A., et al. (2017). Development and validation of a deep learning system for diabetic retinopathy and related eye diseases using retinal images from multiethnic populations with diabetes. JAMA 318 (22), 2211–2223. doi:10.1001/jama.2017.18152
Ting, D. S. W., Pasquale, L. R., Peng, L., Campbell, J. P., Lee, A. Y., Raman, R., et al. (2019). Artificial intelligence and deep learning in ophthalmology. Br. J. Ophthalmol. 103 (2), 167–175. doi:10.1136/bjophthalmol-2018-313173
Tsuji, T., Hirose, Y., Fujimori, K., Hirose, T., Oyama, A., Saikawa, Y., et al. (2020). Classification of optical coherence tomography images using a capsule network. BMC Ophthalmol. 20 (1), 114. doi:10.1186/s12886-020-01382-4
Venhuizen, F. G., van Ginneken, B., Liefers, B., van Asten, F., Schreur, V., Fauser, S., et al. (2018). Deep learning approach for the detection and quantification of intraretinal cystoid fluid in multivendor optical coherence tomography. Biomed. Opt. Express 9 (4), 1545–1569. doi:10.1364/BOE.9.001545
Wan, S., Liang, Y., and Zhang, Y. (2018). Deep convolutional neural networks for diabetic retinopathy detection by image classification. Comput. Electr. Eng. 72, 274–282. doi:10.1016/j.compeleceng.2018.07.042
Keywords: bibliometric analysis, deep learning, artificial intelligence, macular edema, ophthalmology, machine learning
Citation: Feng H, Chen J, Zhang Z, Lou Y, Zhang S and Yang W (2023) A bibliometric analysis of artificial intelligence applications in macular edema: exploring research hotspots and Frontiers. Front. Cell Dev. Biol. 11:1174936. doi: 10.3389/fcell.2023.1174936
Received: 14 April 2023; Accepted: 02 May 2023;
Published: 15 May 2023.
Edited by:
Xiaomeng Li, Hong Kong University of Science and Technology, Hong Kong, SAR ChinaCopyright © 2023 Feng, Chen, Zhang, Lou, Zhang and Yang. This is an open-access article distributed under the terms of the Creative Commons Attribution License (CC BY). The use, distribution or reproduction in other forums is permitted, provided the original author(s) and the copyright owner(s) are credited and that the original publication in this journal is cited, in accordance with accepted academic practice. No use, distribution or reproduction is permitted which does not comply with these terms.
*Correspondence: Weihua Yang, YmVuYmVuMDYwNkAxMzkuY29t; Shaochong Zhang, emhhbmdzaGFvY2hvbmdAZ3p6b2MuY29t; Yan Lou, eWxvdTA0QGNtdS5lZHUuY24=
Disclaimer: All claims expressed in this article are solely those of the authors and do not necessarily represent those of their affiliated organizations, or those of the publisher, the editors and the reviewers. Any product that may be evaluated in this article or claim that may be made by its manufacturer is not guaranteed or endorsed by the publisher.
Research integrity at Frontiers
Learn more about the work of our research integrity team to safeguard the quality of each article we publish.