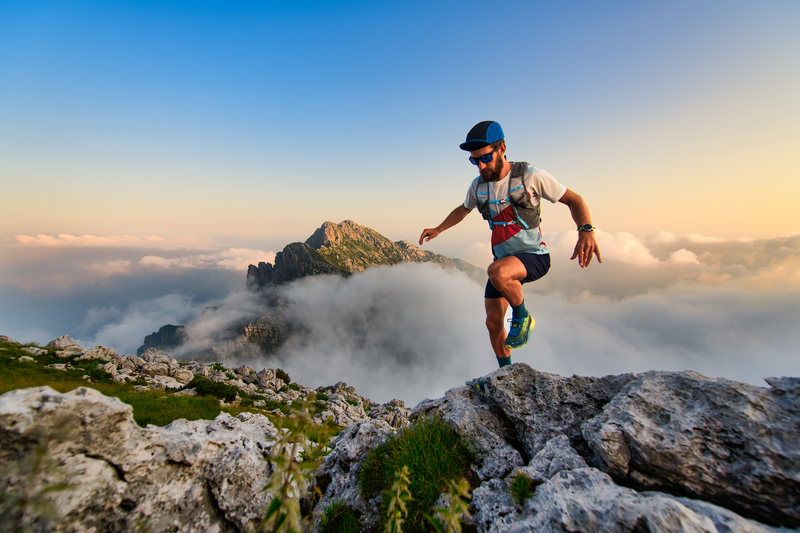
94% of researchers rate our articles as excellent or good
Learn more about the work of our research integrity team to safeguard the quality of each article we publish.
Find out more
ORIGINAL RESEARCH article
Front. Cell Dev. Biol. , 28 February 2023
Sec. Molecular and Cellular Pathology
Volume 11 - 2023 | https://doi.org/10.3389/fcell.2023.1021920
This article is part of the Research Topic Pathogenic Mechanisms in Neurodevelopmental Disorders: Advances in Cellular Models and Multi-omics Approaches View all 9 articles
Purpose: Multi-omics offer worthwhile and increasingly accessible technologies to diagnostic laboratories seeking potential second-tier strategies to help patients with unresolved rare diseases, especially patients clinically diagnosed with a rare OMIM (Online Mendelian Inheritance in Man) disease. However, no consensus exists regarding the optimal diagnostic care pathway to adopt after negative results with standard approaches.
Methods: In 15 unsolved individuals clinically diagnosed with recognizable OMIM diseases but with negative or inconclusive first-line genetic results, we explored the utility of a multi-step approach using several novel omics technologies to establish a molecular diagnosis. Inclusion criteria included a clinical autosomal recessive disease diagnosis and single heterozygous pathogenic variant in the gene of interest identified by first-line analysis (60%–9/15) or a clinical diagnosis of an X-linked recessive or autosomal dominant disease with no causative variant identified (40%–6/15). We performed a multi-step analysis involving short-read genome sequencing (srGS) and complementary approaches such as mRNA sequencing (mRNA-seq), long-read genome sequencing (lrG), or optical genome mapping (oGM) selected according to the outcome of the GS analysis.
Results: SrGS alone or in combination with additional genomic and/or transcriptomic technologies allowed us to resolve 87% of individuals by identifying single nucleotide variants/indels missed by first-line targeted tests, identifying variants affecting transcription, or structural variants sometimes requiring lrGS or oGM for their characterization.
Conclusion: Hypothesis-driven implementation of combined omics technologies is particularly effective in identifying molecular etiologies. In this study, we detail our experience of the implementation of genomics and transcriptomics technologies in a pilot cohort of previously investigated patients with a typical clinical diagnosis without molecular etiology.
Rare genetic diseases, which individually affect a relatively small number of people, collectively represent a public health concern, with more than 300 million people affected worldwide (Boycott et al., 2019). The molecular diagnosis of such conditions has a profound public impact involving genetic counseling and treatment to improve disease management (Sawyer et al., 2016; Hartley et al., 2018; Boycott et al., 2019). Amongst Mendelian disorders, developmental diseases with or without intellectual disability are highly heterogeneous and require extensive workup to establish a molecular diagnosis.
Exome sequencing (ES), the current gold-standard molecular test used for heterogeneous diseases with strong evidence of a genetic etiology, has a 30%–40% diagnostic yield, after negative cytogenetic tests including conventional karyotyping, sub-telomeric screening, array comparative genomic hybridization (array–CGH), and fragile X testing (Clark et al., 2018). Several limitations may explain a high percentage of undetected causative variants, such as poorly enriched exonic regions, pseudogenes, and non-coding variants within untranslated, intronic, and intergenic regions. In addition, inherited variants with incomplete penetrance and variable expressivity may further complicate the correct interpretation of their clinical significance (Bruel et al., 2020).
Short-read genome sequencing (srGS) has been considered a powerful second-tier approach for the molecular diagnosis of unsolved patients (Hartley et al., 2020). Several countries (e.g. the United States, Canada, UK, and France) have now invested in national human genome projects programs to identify the genetic causes of rare and common diseases as well as cancer and population genetics studies (Marshall et al., 2020; Sanlaville et al., 2021; Smedley et al., 2021).
As compared to ES, srGS discovers around two orders of magnitude more variants and requires the implementation of complementary approaches, including split-reads analysis to identify balanced structural variants (SVs), combined with read-depth analysis, to identify unbalanced structural variants below the detection limit of array-CGH (Caspar et al., 2018). However, it remains underused as a routine diagnostic laboratory approach in clinical settings due to the need for tailored bioinformatics pipelines, interpretation tools, and associated costs (Belkadi et al., 2015; Lelieveld et al., 2015; Meienberg et al., 2016). In the last 5 years, several publications have reported that, on average, GS improves diagnostic yield (10%–20%) compared to ES primarily by extending the analysis to non-ES-enriched coding and non-coding regions. Thus, srGS is effective in detecting variants within poorly-enriched exonic regions, and balanced and unbalanced structural variants not observed by the abovementioned techniques (Belkadi et al., 2015; Lelieveld et al., 2015). However, srGS cannot identify structural variants with breakpoints lying within repetitive DNA and GC-rich regions, hampering its ability to fully characterize complex structural variants (Bose et al., 2014). In this respect, low-depth (10–20X) long-read genome sequencing (lrGS) overcomes this problem by generating reads longer than 5 kilobases (Kb) in length, and up to 1–2 megabases (Mb) depending on the technology used, spanning repeated DNA or GC-rich regions, thus facilitating de novo genome assembly, SV calling and haplotype phasing using tailored bioinformatics pipelines (Sedlazeck et al., 2018; Chaisson et al., 2019; Amarasinghe et al., 2020; Mitsuhashi and Matsumoto, 2020). Optical genome mapping is a suitable alternative to lrGS. Using this approach SVs are identified by reconstructing high-resolution genomic maps obtained by detecting specific sequence motifs (fingerprints) along multiple single and extremely long fluorescently-labeled DNA fragments (ranging from 150 Kb up to 2 Mb).
Bionano Genomics optical mapping currently offers a resolution of up to 500 base pairs, thus facilitating first-line genome scaffolding and the detection of large-scale structural variations (Yuan et al., 2020).
However, this method requires additional investigations to achieve nucleotide resolution for the identification of breakpoints, and phase single nucleotide variants/small indels haplotypes.
Genetic information alone is sometimes insufficient to predict the impact of candidate variants on gene expression, transcription or RNA splicing. Interest in RNA sequencing is also increasing in the area of translational research to help identify or prioritize genetic variants (Cummings et al., 2020; Murdock et al., 2021; Yépez et al., 2022). In particular, RNA-seq analysis identifies aberrant splicing events, expression outliers and monoallelic expressions (Kremer et al., 2017; Peymani et al., 2022). Recent studies in clinically accessible sample tissues (i.e. blood, fibroblast or lymphoblastoid cell lines, bone marrow or skeletal muscle) reported a highly variable diagnostic yield of RNA-seq, in combination with ES or GS, between 7.5%–36% in defined cohorts of patients presenting primarily with neuromuscular, mitochondrial or metabolic diseases (Cummings et al., 2017; Kremer et al., 2017; Kremer et al., 2018; Frésard et al., 2019; Gonorazky et al., 2019; Hamanaka et al., 2019; Lee et al., 2020; Rentas et al., 2020; Stenton and Prokisch, 2020; Murdock et al., 2021; Yépez et al., 2022). Interestingly, clinically accessible samples are heterogeneous in terms of gene and transcript expression (GTEx - https://gtexportal.org/home/), (Lonsdale et al., 2013), indicating that their relevance for RNA studies in Mendelian disorders may not be the same, meaning their selection could impact the diagnostic outcome of RNA-seq testing (Rentas et al., 2020; Murdock et al., 2021). Although the interest in using GS, long-read sequencing, optical mapping and RNA-seq for establishing diagnoses in unsolved rare-disease patients, no standard consensus exists regarding the order of applying these approaches after negative or inconclusive routine results in patients with a typical clinical diagnosis.
To address this issue, we used 15 molecularly unexplained individuals diagnosed with an OMIM syndrome. Some patients presented with a recognizable syndrome (i.e., a genetic condition associated with distinctive features, cardinal symptoms, and signs), whose pathogenic variants could be expected to be identified within a relatively short list of well-known OMIM morbid genes. Clinical diagnostic indications of recognizable syndromes are a valuable aid in selecting the most cost-effective molecular approach to identify causative genetic variants. Depending on the possible genetic heterogeneity associated with the investigated disease, several first-line genetic tests, including targeted Sanger sequencing, gene panel testing or ES/GS, can be used to establish a molecular diagnosis before turning to more expensive pan-genomic analyses. Standard approaches, however, may sometimes miss causative variants. The individuals had been previously tested via gold standard diagnostic approaches including Sanger sequencing, gene panel sequencing, ES or array-CGH/SNP-array. Individuals had been clinically diagnosed with: 1) an autosomal recessive disease with a single known pathogenic variant identified, 2) an X-linked recessive disease or 3) a dominant disease. We performed srGS followed by RNA sequencing in clinically accessible samples selected based on the expression pattern of the expected causal genes, in cases with negative or inconclusive GS results. Optical mapping or long-read sequencing (lrGS) were used to resolve structural variants unable to be characterized by srGS alone.
The French AnDDI-rares network recruited affected individuals from eight investigating centers in Dijon, Rouen, Nantes, Rennes, Angers, Strasbourg, Reims in France, and Liège in Belgium. We obtained written informed consent for research purposes for the testing from all subjects or their legal representatives. A clinical description of all individuals is available in the Supplementary Data section.
DNA was extracted from blood collected in EDTA tubes. We incubated 3–5 mL of whole blood for 10 min in RBC lysis buffer (Qiagen GmbH, Hilden, Germany) and then centrifuged it for 2 min at 2000 rpm to pellet white blood cells. The pellet was resuspended in 180 µL of residual supernatant and 20 µL of RNAse A (Qiagen GmbH, Hilden, Germany). DNA was then purified using the QiAamp DNA Blood mini kit on a QiaCube extraction device following the standard protocol.
DNA was quantified using the Qubit dsDNA HS Assay (Life Technologies, CA, United States) and qualified via gel electrophoresis. DNA purity was checked by evaluating the absorbance ratio A260/A280, to evaluate protein contamination, and the A260/A230 ratio, to evaluate organic solvent contamination, using a Multiskan Go device (Thermo Scientific, Waltham, MA, United States).
A minimum of 4 µg of DNA was needed per sample for quality control purposes before sequencing at the CNRGH platform and to potentially prepare a second library in the event of technical problems. The center was asked to provide another sample in the event of insufficient DNA quantity or quality.
Standard methods (described in Supplementary Methods) were used for data extraction. Genomic DNA libraries were prepared in accordance with the TruSeq DNA PCR-free protocol (Illumina, CA, United States). A minimum of 1 µg of genomic DNA was sheared by sonication and then purified. Oligonucleotide adaptors to sequence both ends were ligated on end-repaired fragments and then purified. DNA libraries were barcoded (indexed) and then multiplexed. GS was performed at the Centre National de Recherche en Génomique Humaine (CNRGH, CEA) using the Illumina NovaSeq6000 platform (Illumina, CA, United States), generating 150 base pairs paired-end reads. Data sequencing was required to meet minimum quality standards, with an average of over ×35 depth of coverage and over 97% of the genome covered by at least 10 reads.
Variants were identified using a computational platform of the FHU Translad, hosted by the University of Burgundy Computing Cluster (CCuB). Raw data quality was evaluated by FastQC software (v0.11.4—see web resources). Reads were aligned to the GRCh37/hg19 human genome reference sequence using the Burrows-Wheeler Aligner (v0.7.15) (Li and Durbin, 2009). Aligned read data underwent the following steps: (a) duplicate paired-end reads were marked by Picard software (v2.4.1—see web resources), and (b) base quality score was recalibrated using the Genome Analysis Toolkit (GATK v3.8) Base recalibrator (DePristo et al., 2011). Using GATK Haplotype Caller, Single Nucleotide Variants with a quality score >30 and an alignment quality score >20 were annotated with SNPEff (v4.3) (Cingolani et al., 2012). Rare variants were identified by focusing on non-synonymous changes at a frequency of less than 1% in the gnomAD database. Copy Number Variants (CNV) were detected using two approaches based on read-depth analysis using Control-FREEC (v11.4) (Boeva et al., 2012) and based on read-pair anomalies combined with split-read detection using Lumpy (v0.2.12) (Layer et al., 2014). The resulting CNV and SV were annotated using in-house python scripts and filtered in terms of their frequency in public databases (DGV, ISCA, DDD).
HMW DNA extractions and sequencing were performed by the Centre National de Recherche en Génomique Humaine (CNRGH, CEA). DNA was extracted from frozen PBMCs or fibroblasts (2 to 3 million cells) using Circulomics Nanobind CBB DNA kits (Circulomics Inc., Baltimore, MD), following the standard protocol of the manufacturer. Extracted HMW gDNA was quantified using the Qubit dsDNA HS Assay (Life Technologies, CA, United States), and the quality of the HMW DNA assessed by migration of a small aliquot onto a Femto Pulse System (Agilent Technologies, Santa Clara, CA, United States). HMW DNA purity was verified by evaluating the absorbance ratios A260/A280 and A260/A230 using a NanoDropTM ND-1000 device (Thermo Scientific, Waltham, MA, United States). After gDNA fragmentation to a size of ∼20 kb using a Megaruptor®-2 (Diagenode) and size validation on a TapeStation 4200 (Agilent technologies, Santa Clara, CA, United States), libraries were prepared using an input of 1 µg 20 kb-fragmented-gDNA, following the Oxford Nanopore “Genomic DNA by Ligation” protocol, using the NEBNext® Companion Module for OxfordNanopore Technologies® LigationSequencing (cat #E7180S New England Biolabs) and the Oxford Nanopore Ligation Sequencing kit (cat # SQK-LSK109 or SQK-LSK110, ONT®). Each library was then sequenced on R9.4.1 flowcell using PromethION (ONT®). Base-calling on PromethION was performed using the Guppy version available at the time of processing the samples (Guppy 3.2.6 in 2020 and Guppy 4.3.4 in 2021).
We analyzed the rearrangements in the region targeted by the GS. Reads were aligned to the GRCh37/hg19 human genome reference sequence using the Minimap2 Aligner (v2.11) (39) and were extracted by samtools (version 1.9) (Li et al., 2009) for the chromosome of interest. In line with the recommendations of the official pipeline, chromosome-specific reads were aligned to the genome using Last (version 1080) (Frith et al., 2010). Rearrangements were identified by dnarrange (Mitsuhashi et al., 2020) and five controls. We retained rearrangements supported by at least three reads and grouped the reads with the same rearrangement into a consensus sequence using dnarrange-merge and lamassemble (Frith et al., 2021). Pictures of each consensus sequence were created using last-multiplot and last-dotplot (Frith et al., 2010). For genes of interest, we manually analyzed each group to reconstruct the complex rearrangement.
RNA was extracted from cultured cells or Blood PAXgene tubes. Cultured cells were washed in PBS and lysed in 1 mL Trizol (Ambion); 200 µL of chloroform was added, mixed, and incubated at room temperature (RT) for 3 min before centrifugation for 15 min at 4°C at 12 000 G. The RNA-containing supernatant was then retrieved, and 500 µL of isopropanol was added and mixed before being incubated for 15 min at room temperature and then centrifugated at ×12000 g, 4°C. The RNA-containing pellet was then washed twice with 75% ethanol and centrifugated for 5 min, ×7500 g, 4°C. Ethanol was then carefully eliminated, and the resulting dried-up pellet resuspended in 20 µL of nuclease-free water. For PAXgene Blood tubes, total RNA was extracted from whole blood collected in PAXgene tubes (Preanalytics GmbH, Hombrechtikon, Switzerland) using the PAXgene Blood RNA kit (Preanalytics GmbH, Hombrechtikon, Switzerland) automated on a QiaCube extraction device (Qiagen GmbH, Hilden, Germany) following the standard protocol. RNA was then quantified by absorbance measurement on a Nanodrop device. Quality was assessed by determining the RNA Integrity Number (RIN) on a bioanalyzer device (Agilent Technologies, Santa Clara, CA, United States). For RNA-seq, RNA with an RNA integrity Number (RIN) ≥7 was used.
RNAseq sequencing was carried out at the CNRGH (CEA). After an RNA quality control on each sample (duplicate quantification on a NanoDrop™ 8000 spectrophotometer and RNA6000 Nano LabChip analysis on Bioanalyzer from Agilent), libraries were prepared using either the “TruSeq Total stranded RNA Library Prep Gold” from Illumina (individual 9085), or the “TruSeq Stranded mRNA Library Prep Kit” from Illumina (individual 13158). All libraries were prepared on an automated platform, using an input of 1 µg of total RNA, in accordance with the instructions of the manufacturer. Library quality was checked on a LabGx (Perkin Elmer) for profile analysis and quantification. Sample libraries were pooled before sequencing to reach the expected sequencing depth. Sequencing was performed on an Illumina HiSeq4000 as paired-end 100 bp reads, using dedicated Illumina sequencing reagents. Libraries were pooled using four samples per lane. Fastq files produced after RNA-seq sequencing were then processed using in-house CNRGH tools to assess the quality of raw and genomic-aligned nucleotides.
Aberrant splice events and expression outliers were identified using a computational platform of FHU Translad. Raw data quality was evaluated by FastQC software (v0.11.4.—see web resources). Reads were aligned to the GRCh37/hg19 human genome reference sequence using the STAR2 Aligner (v2.5.2b) (Dobin et al., 2013) with the 2-pass mapping method using the human RefSeq genome annotation (Build GCF_000001405.25[YD1]). Read counts were also collected using STAR2. Uniquely mapped reads were counted while overlapping only one gene. Outlier expressed genes were detected using two parallel methods: DESeq2 (v1.26.0) (Love et al., 2014) and Outrider (v1.4.2) (Brechtmann et al., 2018). After a normalization step, expression analysis was performed using the following analysis design: 1 versus all the analysis batch allowing computation of the expression variance on the whole cohort. A Z-score was computed, and filters applied to only retain genes with a z score above 3 or below −3. Aberrant splice events were detected using three parallel methods: rMATS (v4.0.2) (Shen et al., 2014), LeafCutter (v0.2.9) (Jenkinson et al., 2020), and a custom method derived from Cummings and collaborators (Cummings et al., 2017). We computed a Percent-Splice-In (PSI) value, indicating the proportion of the junction implied in a splice event, using rMATS. LeafCutter applies an intron-centered analysis using a clustering method. A Z-score was computed for both methods, applying the same filters as those used for expression. The custom method considered each splice junction as a rare variant and applied a filter based on frequency in the cohort to ensure only rare events were selected.
Ultra-high molecular weight (UHMW) genomic DNA (gDNA) was extracted from 1.5 million white blood cells or fresh cultured fibroblast cells via the SP Blood & Cell Culture DNA Isolation Kit (ref: 80030, Bionano Genomics) according to the Bionano Prep SP frozen human blood DNA isolation protocol or the Bionano Prep SP fresh cells DNA isolation protocol. After quantification, 750 ng of UHMW gDNA was labeled via a DLS DNA Labeling Kit (ref: 80005, Bionano Genomics) according to the Bionano prep DLS protocol. Labeled UHMW gDNA was then analyzed on a Bionano Saphyr instrument with a target output of 500 Gbp. Raw data from Saphyr were directly exported in a BNX molecule-information file format. The de novo assembly was performed by the Bionano Solve pipeline (v3.6.11162020). Short molecules (<120 kbp) or molecules with insufficient labels (<9) were filtered out. Molecules were then sorted using a numeric molecular identifier. Molecule-noise parameters (including missing or extra labels, sizing intervals, and stretch factors) were estimated during the auto-noise stage. The resulting corrected BNX file contained rescaled molecules, which were aligned with each other using a pairwise algorithm producing the basis of an overlap graph. A first assembly was created from the pairwise alignments by analyzing potential paths on the chart, resulting in a consensus map (CMAP file). The longest paths were selected by successive clean-up steps. RefAligner software was used to further refine the draft consensus map in several stages by adjusting the labels and splitting map regions, where necessary, due to poor molecule coverage. The resulting refined consensus maps were then extended and merged iteratively to produce more contiguous maps. Maps were generated during a final refinement stage in which molecules were realigned to the maps. These maps were then modified when other molecules, typically those with alternative alleles, offered better results. The final consensus map was used to detect structural variants by comparing the map to a given reference, aligning the consensus map to the reference map. Results were produced in SMAP files convertible into VCF files. In a final stage, data was uploaded to the Bionano Access (v1.6.1) web server, to display the maps and detected variants.
Fifteen molecularly unsolved individuals (12 males and 3 females) clinically diagnosed with OMIM-morbid syndromes previously tested using standard diagnostic methods were identified via the French AnDDI-rares network. Their clinical diagnosis had been established based on the presence of cardinal symptoms characteristic of a particular rare disease or by a reverse-phenotyping approach after identifying a single pathogenic variant in a gene linked to a recessive disease, compatible with the clinical presentation of the patient. In the eight individuals clinically diagnosed with an autosomal recessive disorder, first-line genetic investigations identified only one causative variant but failed to pick up a second hit. In the other individuals, negative results were obtained for first-line genetic in six individuals and identified a variant of unknown significance in one individual.
Diseases investigated included persistent Mullerian duct syndrome, type II (MIM 261550, autosomal recessive); developmental and epileptic encephalopathy 28 (MIM 616211, autosomal recessive); Cohen syndrome (MIM 216550, autosomal recessive); muscular dystrophy, limb-girdle, autosomal recessive 5 (MIM 253700, autosomal recessive); Alopecia-intellectual disability syndrome 4 (MIM 618840, autosomal recessive); muscular dystrophy, limb-girdle, autosomal recessive 23 (MIM 618138, autosomal recessive); Spermatogenic failure 5 (MIM 243060, autosomal recessive); primary ciliary dyskinesia-7 (MIM 611884, autosomal recessive); Simpson-Golabi-Behmel syndrome, type 1 (MIM 312870, X-linked recessive); Marfan syndrome (MIM 154700, autosomal dominant); Cowden syndrome (MIM 158350, autosomal dominant); tuberous sclerosis complex (MIM 613254, autosomal dominant); familial adenomatous polyposis 1 (MIM 175100, autosomal dominant); and KBG syndrome (MIM 148050, autosomal dominant) (Table 1, supplementary data).
TABLE 1. Cohort of 15 individuals with the different omic tests performed and their outcome. AD, autosomal dominant; AR, autosomal recessive; XLR, X-linked recessive; NP, not performed; sr-GS, short read genome sequencing; mRNA-seq, m RNA sequencing; OM, optical mapping; lr-GS, long read genome sequencing.
Individual 1 was a 30-year-old male, the third child of non-consanguineous French parents, presenting with a typical Cowden syndrome (Supplementary Figures S1A–C). Immunofluorescence confirmed an absence of PTEN expression in thyroid tissue. Genetic investigations consisting of array-CGH and ES with good coverage and depth on PTEN were negative. GS revealed a non-sense variant p.(Arg303*) in exon 6 of PTEN (Supplementary Figure S1D). Reanalysis of previous ES data revealed the presence of the variant on the Integrative Genomics Viewer but also showed that it had been filtered out by the bioinformatic pipeline of the service provider.
Individual 2 was a 34-year-old male from a family with seven children (six boys) with no noteworthy family medical history, presenting with typical Spermatogenic Failure 5. Genetic investigations consisting of AURKC Sanger sequencing identified a single frameshift variant p.(Leu49Trpfs*23) in exon 3 of AURKC. The variant was in gnomAD (v2.1.1) and classified as pathogenic in ClinVar. GS identified a second ClinVar pathogenic hit, i.e., a non-sense variant p.(Tyr248*) in exon 6 of AURKC (Supplementary Figures S1E–F). Revaluation of AURKC Sanger sequencing data revealed the presence of a barely detectable peak, corresponding to the NM_01015878.1:c.744C>G variant identified by srGS. This false negative result was due to partially degraded sequencing primers on a single analysis batch.
Individual 3 was a 2-year-old girl, the first child of unaffected, non-consanguineous African parents. Tuberous sclerosis complex (TSC) was diagnosed prenatally. Prenatal and postnatal genetic investigations including screening for TSC in amniotic fluid and then blood and saliva samples using a specific panel were negative. GS analysis identified a frameshift variant p.(Gly654Alafs*44) in exon 19 of TSC2 (Supplementary Figure S1G). Reanalysis of TSC2 panel data revealed a bioinformatic pipeline anomaly that had filtered out this variant. In fact, it was artifactually detected in all patients from all batches but at an allelic frequency ranging between 0.7%–25% and associated with a sequencing strand bias.
Individual 4 was a 6-year-old boy, the oldest child of unaffected, non-consanguineous French parents, with a clinical diagnostic of Simpson-Golabi-Behmel syndrome and a familial history compatible with X-linked inheritance. GPC3 Sanger sequencing was negative. GS revealed a hemizygous frameshift variant p.(Gln94Serfs*10) in exon 2 of GPC3 (Supplementary Figures S1H, I). This variant was absent in gnomAD (v2.1.1). Familial segregation analysis revealed maternal inheritance. The variant was also identified in a hemizygous state in his affected brother. Revaluation of GPC3 Sanger sequencing data revealed the presence of the variant that had been missed due to poor data quality.
Individual 5 was a 37-year-old male, the third child of unaffected, non-consanguineous French parents, having been diagnosed with Persistent Müllerian duct syndrome (PMDS), and a normal karyotype (46, XY). Targeted mutational AMHR2-gene screening identified only one pathogenic variant, a maternally inherited recurrent 27-bp deletion in the kinase domain. Five AMHR2 promoter sequencing and AMHR2 southern blot performed to discover the second pathogenic allele produced negative results. Sanger sequencing of AMH (Anti-Mullerian hormone) was also negative. GS identified the second allele, which consisted of a missense variant p.(Thr447Ile) in exon 10 of AMHR2 inherited from the father in the kinase domain within the interval of the first deletion (Supplementary Figures S1J, K). This variant was absent in gnomAD (v2.1.1). In silico prediction scores were in favor of its pathogenic effect.
Individual 6 was a 23-year-old male, the third child of unaffected, non-consanguineous French parents, with a typical clinical diagnosis of Marfan syndrome with ectopia lentis (Supplementary Figures S2A, B). The presence of ectopia lentis was highly suggestive of the implication of the FBN1 gene since this clinical feature is not one of the other Marfan syndrome genes. Previous genetic investigations, consisting of Sanger sequencing and MLPA techniques of FBN1, panel genes of Marfan syndrome and related diseases, array-CGH, and ES solo were negative. GS revealed a de novo balanced translocation which disrupted exon 40 of FBN1 (Supplementary Figure S2C). The translocation was confirmed by conventional karyotyping (Supplementary Figure S2D).
Individual 7 was a 33-year-old male, the third child of unaffected non-consanguineous French parents, with a clinical diagnosis of limb-girdle muscular dystrophy. Immunofluorescence on muscle biopsy showed a pattern consistent with a sarcoglycanopathy. Singleton ES identified only one heterozygous missense variant p.(Glu263Lys) in exon 8 of SGCG (Supplementary Figure S3A), previously described in the literature (DiCapua and Patwa, 2014; Al-Zaidy et al., 2015). ClinVar and HGMD databases have reported this variant as pathogenic. No additional single nucleotide, indels, or copy number variants were detected from ES data. GS confirmed the presence of the previous variant and identified a heterozygous intragenic deletion of about 6.5 kb encompassing exon 7 (124 bp) of SGCG and expected to produce an out-of-frame variant p.(Arg193Serfs*46) (Supplementary Figure S3B). This deletion was confirmed by qPCR, and the segregation analysis revealed that deletion was inherited from the mother (Supplementary Figure S3C – Supplemental material and methods).
Individual 8 was the first child of unaffected, non-consanguineous French parents. Gestational diabetes occurred during pregnancy requiring only health and dietary guidance. At 23 weeks of gestation (WG) + 2 days, ultrasound scans revealed complex cardiac malformation. After counseling, the couple opted for termination of pregnancy at 24 W G + 5. The fetal autopsy found situs abnormalities suggesting Ivemark syndrome type 1. Prenatal array-CGH was negative. Trio ES showed a heterozygous frameshift variant p.(Phe2214Trpfs*35) in DNAH11 (Supplementary Figure S3D), inherited from the father. No additional single nucleotide, indels, or copy number variants were detected from ES data. GS identified a heterozygous intragenic deletion of about 4.2 kb encompassing exons 21 and 22 of DNAH11 (Supplementary Figure S3E).
Individual 9 was a 14-month-old boy, the first child of unaffected, non-consanguineous French parents, presenting with neonatal hypotonia, and joint contractures compatible with congenital muscular dystrophy. Previous genetic investigations consisting of array-CGH had shown paternal inherited deletion of exons 3 and 4 of LAMA2. SrGS confirmed the presence of the 82 kb heterozygous intragenic deletion encompassing exons 3 and 4 of LAMA2. It also identified a heterozygous complex structural variant not resolvable by srGS alone, containing a 120 kb intragenic duplication encompassing exons 19 to 39 of LAMA2 followed by a 92 kb duplication including the first exon of TMEM244 fused into a single segment lacking the intergenic region, indicating they were from a single duplication/deletion event (Figures 1A, B). GS revealed the maternal inheritance of this complex structural variant (Figure 1A).
FIGURE 1. Individual 9—Muscular dystrophy, limb-girdle, autosomal recessive 23, MIM 618138. (A) Ideogram showing chromosome 6 and LAMA2 localization. UCSC genome browser snapshot with visualization of LAMA2 sequencing depth with an intragenic deletion encompassing exons 3 and 4 inherited from heterozygous father and intragenic duplications affecting exons 19 through 39 of LAMA2 and the first exon of TMEM244, inherited from heterozygous mother. (B) Short-read sequencing and optical genomic mapping (OM) results demonstrated that LAMA2 and TMEM244 duplications belonged to the same complex event, including a partial deletion of the intergenic region within the duplicated fragment (i.e. copy neutral region). The insertion breakpoint is indicated by the green arrow on the left.
We used long-read genome sequencing (Oxford Nanopore) and optical genome mapping (Saphyr—Bionano Genomics) to resolve this complex structural variant. They confirmed the results obtained by srGS. However, the results were discordant regarding the position of the duplicated region, the latter indicating LAMA2 while the former downstream TMEM244 (Figure 1B and Supplementary Figure S4A). These conflicting results were due to the length of genomic fragments. As optical genome mapping analyzes assembled genomic fragments up to the megabase scale, it outperforms long-read sequencing. Thus revealing that the breakpoint was located near label position NC_000006.11:g.129611978 (Figure 1B). The insertion contained a chimeric fragment composed of partial duplication of LAMA2 (NC_000006.11:g.129611978_129819640), a breakpoint between label positions NC_000006.11:g.129720651 and NC_000006.11:g.129733425, and a partial duplication of TMEM244 and its downstream region (NC_000006.11:g.130181530_130263816) (Figure 1B). A reevaluation of GS data defined breakpoint at position NC_000006.11:g.129604517 in intron 18. The inserted fragment interrupted LAMA2 at intron 39, resulting in a loss-of-function allele (Figure 1B).
Individual 10 was a 5-year-old boy, the fourth child of unaffected, non-consanguineous Tunisian parents, diagnosed with Simpson-Golabi-Behmel syndrome with a family history compatible with X-linked inheritance (Figures 2A, B). Genetic investigations consisting of array-CGH and overgrowth syndrome panel showed a duplication of 140 kb in Xq24, encompassing SLC25A43 and the 5′ terminal of CXorf56, considered as likely benign as duplications of these regions are found in the control population. However, in view of the clinical indication, a targeted inspection of the GPC3 locus revealed the presence of a single deviated probe suggesting a small deletion in intron 2. ES analysis confirmed that the exonic regions were not affected by the deletion. GS showed that both structural variants were part of the same event involving the insertion of the Xq24 region within the deleted region in the second intron of GPC3, mediated by SINE elements located at both ends of the two regions (Figure 2C). These results were corroborated by PCR analysis, using two couples of primers designed to selectively amplify the insertion-deletion event within the GPC3 locus and encompassing the two genomic breakpoints (Figure 2D). Segregation analysis revealed that the insertion-deletion event was present in the elder affected brother and the mildly affected mother but was absent in the healthy maternal grandmother and aunt. The healthy maternal grandfather was not available for DNA testing (Figure 2D).
FIGURE 2. Individual 10—Simpson-Golabi-Behmel syndrome, type 1, MIM 312870. (A) Pedigree of individual 10. The affected status is indicated by filled symbols; the carrier is indicated by a dot symbol. (B) Dysmorphic features include telecanthus, palpebral edema, wide mouth, macroglossia, thin upper lip vermilion, smooth philtrum, supernumerary nipple, and umbilical hernia. (C) X chromosome ideogram and GPC3 and Xq24 loci. Read depth tracks showing a deletion of 30 Kb in intron 2 of GPC3 and an Xq24 duplication encompassing SLC25A43, SLC25A5, and the first exon of CXorf56. Split-read analysis indicated that these copy number variants belonged to the same event involving the insertion of the Xq24 region within the deleted intronic region of GPC3, visualized in the circos plot. (D) Schematic representation of the Xq24 insertion in the GPC3 locus and the control region NBPF1. The insertion-deletion event was validated by PCR using two couples of primers as indicated in the color scheme. Gel lanes are labeled according to the primer scheme. L: ladder, I10: index case, M: mother, F: father, B: brother, A: aunt. (E) RNA-sequencing differential expression analysis in fibroblast cell line showing loss of GPC3 expression (red dot. and arrow) in individual 10 as compared to a cell line obtained from a healthy (unaffected) male. (F) qPCR results at 5′ and 3′. Ends of GPC3 cDNA. The results were normalized according to GAPDH expression and represented as relative expression, according to a healthy (unaffected) male (XY).
RNA-sequencing in a fibroblast cell line derived from the affected individual compared to control male lines showed a loss of GPC3 expression (Figure 2E), also corroborated by qPCR on cDNA (Figure 2F). RNA-sequencing also indicated that no alternative or fusion transcript originated from this locus.
Individual 11 was a 7-year-old girl, the second child of unaffected, non-consanguineous French parents, presenting with epileptic encephalopathy with limb spasticity, ataxia, dysmetria, movement disorders, and stereotypy. Previous genetic investigations consisting of array-CGH, screening for Angelman syndrome (DNA methylation analysis and UBE3A sequence analysis), and mitochondrial DNA sequencing were negative. Trio ES showed a missense variant inherited from the mother p.(Thr12Arg) in exon 1 of WWOX (Supplementary Figures S5A, B), which was absent in gnomAD with in silico prediction scores in favor of its pathogenicity. GS identified the second event, which consisted of a complex rearrangement with two intronic deletions (introns 4 and 5) associated with an inversion of exon 5 (Supplementary Figures S5C, D). Sanger sequencing analysis of PCR-generated amplicons encompassing the breakpoints revealed a 19 bp insertion in the chimeric fragment involving the 3′-end junction (i.e., fragment 2 Supplementary Figure S5D – Supplemental material and methods). An PCR analysis of WWOX transcript, using primers encompassing exons 4 through 6, obtained from blood, revealed the presence of a shorter amplicon isoform (186 bp) in addition to the expected 293 bp band in individual 11 and her father, but not in the mother (Supplementary Figure S5E). Amplicon-sequencing analysis of the 186 bp gel-purified PCR product demonstrated that the structural variant caused exon 5 skipping in individual 11 and also in her father (Supplementary Figures S5E, F – Supplemental material and methods). Western blotting revealed WWOX loss in a fibroblast cell line obtained from a skin biopsy of Individual 11 compared with a sample from a healthy individual, confirming the pathogenic effect of the two identified variants (Supplementary Figure S5G – Supplemental material and methods).
Individual 12 was a 30-year-old male, the first child of unaffected, non-consanguineous French parents whose autosomal recessive Cohen syndrome has been diagnosed due to the association of retinitis pigmentosa, ID, and specific facial features (Figure 3A). Targeted mutational screening of VPS13B had identified a maternally inherited pathogenic heterozygous frameshift variant p.(Tyr2711*). GS analysis was negative for structural variants accounting for the phenotype of the patient. RNA-seq analysis performed in patient-derived fibroblasts revealed the presence of a cryptic 422 bp exon located between exons 6 and 7. Reevaluation of GS data showed a deep intronic heterozygous variant (NC_000008.10:g.100125810G>T, LRG_3514t1:c.763-2118G>T) in intron 6 of VPS13B, inherited from the father and predicted to create an acceptor splice site (SpliceAI acceptor gain 0.28 at 3 base pairs downstream to it). A cryptic splice donor site was predicted at position NC_000008.10:g.100126234 (Figure 3B). The variant was absent in gnomAD (v2.1.1). The cryptic exon altered the open reading frame by creating a premature stop codon p.(Ile255Thrfs*7).
FIGURE 3. Individual 12—Cohen syndrome, MIM 216550. (A) Dysmorphic features include down-slanting palpebral fissures, midface retrusion, short philtrum, open mouth appearance, high palate, micrognathia, truncal obesity, narrow hand with tapered fingers, and joint hyperextensibility. (B) Ideogram showing chromosome 8 and VPS13B localization. UCSC genome browser snapshot with visualization of VPS13B single nucleotide polymorphisms (SNP), VPS13B sequencing depth, VPS13B RNA-sequencing depth and splicing analysis (sashimi plot) in individual 12 and a healthy individual (control). Left panel, visualization of the deep intronic heterozygous variant (NC_000008.10:g.100125810G > T) in intron 6, indicated by a light blue line and corresponding to the beginning of the cryptic exon. Red lines indicate spice events. This variant resulted in the creation of an acceptor splice site visualized on the splice analysis tract. The right panel shows the variant inherited from the mother indicated by the light blue line.
Individual 13 was a 4-year-old boy, the second child of non-consanguineous healthy parents, presenting with a clinical diagnosis of Alopecia-intellectual disability syndrome 4 (Figure 4A). His case was published by Besnard et al. (2019). Sanger sequencing identified only one maternally inherited LSS missense variant (c.1955C>T; p.Thr652Ile) in exon 20 and absent in gnomAD (v2.1.1). Linkage analysis using single nucleotide polymorphisms of LSS showed that individual 13 and his unaffected sister had inherited the same paternal allele but a different maternal allele. The authors also observed an unbalanced expression in favor of the maternal allele. These results were confirmed by RT-PCR in fibroblast from individual 13 and his father. The cause of this allelic imbalance was unexplained. We performed GS in individual 13, and RNA-sequencing in individual 13 and his father (Figure 4B). GS did not reveal any apparent structural variant (Figure 4C). Transcriptome analysis identified the second hit, i.e., an aberrant splice event involving exon 1 and promoter region of LSS (Figure 4D). RNA-seq data also confirmed an altered allelic expression of the maternal allele in individual 13 and a monoallelic expression of LSS in the father (Figure 4C). Reevaluation of GS at the promoter region indicated an unevenly distributed sequencing depth and the potential presence of an ill-defined SV. The predicted SV was too small to be characterized by optical mapping (i.e., below 500 bp). Oxford Nanopore long-read sequencing was therefore used to resolve this region. A long-read data analysis confirmed the presence of a small deletion of 339 bp encompassing exon 1 and the intergenic region between LSS and MCM3AP (Figure 4E).
FIGURE 4. Individual 13—Alopecia-intellectual disability syndrome 4, MIM 618840. (A) Dysmorphic features showing alopecia universalis. (B) Pedigrees of individual 13. The known maternally inherited LSS pathogenic variant was Sanger sequenced. LSS pathogenic variants are highlighted in red. (C) Ideogram showing chromosome 21 and LSS localization. UCSC genome browser snapshot with visualization of LSS SNPs, sequencing depth, and genome and RNA-sequencing allelic balance from IGV (integrative genomics viewer) of three variants, one private maternal variant and two common paternal variants in individual 13, his father and two healthy controls, confirming monoallelic expression of the maternal allele in individual 13. (D) Visualization of the promoter region of LSS. Red arrows show aberrant splicing within the intron 1 of LSS and the intergenic region between LSS and MCM3AP in individual 13 and his father. (E) IGV visualization of long-read Oxford Nanopore genome sequencing showing the sequencing depth and a heterozygous deletion affecting the promoter region of LSS.
Individual 14 was a 34-year-old female, the third child of unaffected, non-consanguineous French parents. A colonoscopy performed at the age of 28 due to abdominal pain revealed multiple colonic and rectal polyposis and adenocarcinoma of the transverse colon. The presence of polyposis at this early age suggested a need for genetic testing for a predisposition to polyposis. Targeted mutational screening of the APC and MYH was performed on blood samples and digestive tract biopsies; gene panels for familial adenomatous polyposis and ES were negative. Replication error (RER) phenotyping of the tumor revealed a microsatellite stable (MSS) phenotype and, in terms of immunohistochemistry, persistent expression of hMLH1, hMSH2, hMSH6, and PMS2 proteins was observed. GS and RNA-sequencing in fibroblasts detected no apparent anomalies, particularly no candidate variant in APC.
Individual 15 was a 22-year-old male, the first child of a single French mother, presenting with a clinical diagnosis of KBG syndrome. Previous genetic investigations, including array-CGH, intellectual disability panel including ANKRD11, and solo ES, produced normal results. GS and RNA-sequencing detected no obvious anomalies. However, the srGS reanalysis identified a variant of unknown clinical significance in OPHN1 (MIM 300486) (NM_002547.2:c.701A>C, p.Asn234Thr) predicted to alter RNA splicing (SPiP 85.91%). OPHN1 expression was not detectable in blood by RNA-seq, and we were unable to investigate the functional impact of this variant on the messenger RNA.
Our study pinpoints the importance of phenotype-driven genomic investigations for identifying causative variants in rare diseases. From a clinical perspective, it is seminal to describe clinical terms with a standardized vocabulary (Human Phenotype Ontology—HPO terms), family history, and mode of inheritance. The goal of using common phenotypic semantics is to cover all phenotypic abnormalities commonly encountered in human monogenic diseases (Köhler et al., 2019). Deep phenotyping allows the characterization of robust diagnostic hypotheses. Different diagnostic approaches should be used if the phenotype is convincing, despite negative first-line genetic testing, or in the presence of only a single heterozygous pathogenic variant in the case of an autosomal recessive mode of inheritance (Frésard and Montgomery, 2018). Here, we described a combined omics strategy to solve 15 unrelated individuals diagnosed with rare Mendelian diseases for which first-line genetic investigations failed to establish a molecular diagnosis. We applied in a stepwise manner GS and mRNA-seq on patient-derived samples, optical genome mapping and long-read sequencing. Overall, these approaches resulted in a molecular diagnosis in 87% of the cohort (13/15 individuals). GS alone identified five causal SNVs/indels, four of which had been missed by first-line targeted tests and four structural variants. The mRNA-seq analysis confirmed the deleterious outcome of two complex rearrangements detected by GS and discovered one structural variant and one deep intronic SNV not previously identified by GS alone.
The failure to identify causal variants in known disease-causing genes by first-line genetic investigations, such as targeted sequencing, gene panel or exome sequencing, can be explained in numerous ways. It can be missed as interpreting the data can be challenging, hence re-analyzing the available data is key before carrying out further investigations. Technical limitations include biases in sequencing data (e.g., reduced coverage/sequencing depth, locus-specific features such as GC-extreme regions, homopolymeric repeats), or bioinformatics issues (Ross et al., 2013). For example, the diagnosis of individuals 1 and 3 allowed a correction of the exome and the panel bioinformatic pipelines of the respective centers. The causal variant can be considered of unknown significance, as the mechanism responsible for the disease may not be obvious (Richards et al., 2015). Functional assays and integration of genomics with other omics data (e.g., transcriptomics, proteomics, epigenomics, or metabolomics where appropriate) will thus enhance genotype-phenotype relationships for variant classification. In our study, a result was obtained for individual 11 using this integrated strategy. Structural variants cannot be adequately picked up, as seen in individuals 7, 8, 9, and 11. Detecting somatic mosaicism is also challenging as the distribution of causative variants is non-random and often absent or in a low level in blood samples. In addition, rare variants located in non-coding regions, such as deep intronic or regulatory variants, may need additional lines of evidence to demonstrate their implication in human disease, as shown in individuals 12 and 13 (Short et al., 2018; Bodle et al., 2021; Bryen et al., 2021).
In our experience, genomics and integrating analyses, such as pan-genomic or targeted transcriptomics approaches, should be used as a first resort to increase the diagnostic yield in negative patients analyzed by first-line genetic tests. GS can identify genomic variants in the coding and non-coding regions, such as indels, CNVs, and chromosomal rearrangements (Gilissen et al., 2014; Belkadi et al., 2015; Boycott et al., 2019). Structural variants are probably more frequently involved in Mendelian inheritance than expected, as observed in our cohort and reported elsewhere (Sanchis-Juan et al., 2018). It remains a bioinformatic challenge to detect and characterize such variants (Mahmoud et al., 2019; Kobren et al., 2021). Re-analyzing the available data using the latest available reference human genome combined with various tools for detecting CNVs may facilitate the identification of variants missed during previous analyses. Long-read sequencing technology and optical mapping approaches may now improve their detection (Chan et al., 2018; Chaisson et al., 2019; Logsdon et al., 2020).
Interpreting coding variants of unknown significance or non-coding variants is also challenging, advocating the need for the integration of transcriptomic data for variant reclassification. Transcriptomics can identify skewed allelic RNA expression, gene expression outliers, splicing aberrations, and gene fusions. So far, several computational approaches have been developed, for transcript abundance or differential splicing analyses (Cummings et al., 2020; Mehmood et al., 2020; Shahjaman et al., 2020). Recent studies have estimated that the diagnostic yield associated with RNA-seq, in combination with ES or GS, ranges from 7.5%–35% (Kremer et al., 2018; 2017; Cummings et al., 2017; Frésard et al., 2019; Gonorazky et al., 2019; Hamanaka et al., 2019; Lee et al., 2020; Stenton and Prokisch, 2020). Murdock et al. (2021) showed that a transcriptome-directed approach resulted in a diagnostic rate of 12% across their entire cohort, or 17%, after excluding cases solved on ES/GS alone. The diagnostic rate associated with RNA-seq is higher in cohorts with well-defined diseases, with an appropriate clinically accessible sample suitable for modeling candidate gene expression and splicing. In fact, tissue and isoform expression are essential factors to consider for RNA-seq analysis. In their cohort of 115 undiagnosed patients with diverse phenotypes, Murdock et al. (2021) showed that RNA-seq derived from fibroblast cell lines exhibited higher and less variable gene expression in clinically-relevant genes. Clinically accessible tissues such as blood samples, skin and muscle biopsies, as well as cell cultures (e.g., Epstein–Barr-transformed lymphocytes, fibroblast cell lines) are sometimes inadequate to model genes prevalently expressed in the central nervous system (Aicher et al., 2020). Reprogrammed neurons or astrocytes obtained from induced pluripotent cell lines should be considered when modeling neural-specific genes (Bronstein et al., 2020).
Overall, we showed that typical clinical diagnosis of rare Mendelian diseases with negative or inconclusive first-line investigations could be largely resolved by the sequential use of srGS and mRNA-seq. With optical mapping and long-read genome sequencing we resolved one complex structural variant and one small deletion, identified either by srGS alone or in combination with RNA-seq, respectively. Overall, this approach allowed us to identify a molecular diagnosis in 13 out of 15 patients (87%). Our findings suggest that srGS should be used to guide the next approach in case of negative or inconclusive results in a multi-step analysis. RNA-seq should be used as a first-line analysis after negative srGS results or to reclassify variants of unknown significance, with a suspected splicing effect, in suitable clinically accessible samples. Structural variants not resolvable by srGS alone may benefit from optical mapping and/or long-read genome sequencing, selected according to the length, composition, and complexity of the structural variant (Figure 5). Longer term, national genomic medicine programs may benefit from implementing this type of analytical approach to reduce diagnostic deadlocks, even in heterogeneous neurodevelopmental disorders not associated with clinically recognizable syndromes. By integrating short-read genome sequencing, transcriptomics, and epigenomics (DNA methylation) analyses, we resolved about 33% of the patients with heterogeneous rare neuro-developmental disorders with previous negative exome sequencing results. We also identified 13% additional candidate variants (Colin et al., 2022). This approach is effective in identifying causative variants when exhaustive clinical information is available for genotype-phenotype correlation analysis. However, additional techniques may be required to reclassify missense variants of unknown significance (VUS) or to link the presence of non-coding regulatory variants to abnormal gene expression. Blood-derived DNA methylation episignatures are highly sensitive and specific DNA methylation biomarkers allowing VUS in genes with an established episignature to be assessed or reclassified (Aref-Eshghi et al., 2020; Sadikovic et al., 2021; Levy et al., 2022), along with additional omics technologies such as proteomics analyses performed in relevant clinically accessible samples/cell lines (Alston et al., 2021), are promising complementary approaches to be used. Recently, global chromosome conformation capture techniques (Hi-C) have been also used to reclassify structural variants of unknown significance in unsolved rare-disease patients (Melo et al., 2020) allowing researchers to identify, in some cases non-coding regulatory elements triggering the ectopic expression of candidate genes (de Bruijn et al., 2020). The implementation of these approaches in diagnostics will require further investigation and validation from independent laboratories.
FIGURE 5. Suggested decision tree indicating the omic strategy implemented after inconclusive or negative results. Green, blue, and yellow backgrounds indicate short-read genome technologies, and transcriptome and long-read optical mapping technologies, respectively. Technics are shown in grey boxes.
The datasets presented in this study can be found in online repositories. The names of the repository/repositories and accession number(s) can be found below: NCBI ClinVar, accession numbers: SUB12126248, SUB12113566, SUB12114050.
The studies involving human participants were reviewed and approved by the appropriate institutional review board of Dijon University Hospital (DC2011-1332). Written informed consent to participate in this study was provided by the participants legal guardian/next of kin. Written informed consent was obtained from the individual(s), and minor(s) legal guardian/next of kin, for the publication of any potentially identifiable images or data included in this article.
AV, YD, CT-R, and LF conceived and designed the study. AV, LF, and CT-R provided funding. EC, BI, JD, AG, MF, F-GD, AS, SM, MG, DB, SC, MD-F, and LF clinically evaluated the patients. EC, LF, CT-R, and AV collected the data. AB, RO, and J-FD performed genome sequencing and RNA sequencing, YD, SV, and ET performed bioinformatic analyses. FM-T, A-SD-P, HS, PC, CP, CT-R, and AV performed variant interpretation. MC and JP performed wet-lab work. TB, BC, CD, KU, NC, and DS contributed to genetic analyses. EC, YD, and AV organized tables and Figures. EC and AV drafted the paper with contributions from all authors who read and approved the submitted version.
This work was supported by grants from Dijon University Hospital, the ISITE-BFC (PIA ANR), the European Union through the FEDER programs (PERSONALISE), and the Burgundy-Franche Comté regional council (INTEGRA). The sequencing performed at the CNRGH was supported by the France Génomique National infrastructure, funded as part of the “Investissements d’Avenir” program managed by the Agence Nationale pour la Recherche (contract ANR-10-INBS-09).
We are grateful to the families for their participation in this study. We thank the “Centre de Ressources Biologiques Ferdinand Cabanne” (CHU Dijon) for sample biobanking. We thank the University of Burgundy Computing Cluster (CCuB). The study was performed within the framework of the GAD (“Génétique des Anomalies du Développement”) collection and approved by the appropriate institutional review board of Dijon University Hospital (DC 2011–1332). We also thank Dr Agnès Guichet and Dr Marine Tessarech for their cytogenetic advice, and Alexandre Plagos for technical assistance.
The authors declare that the research was conducted in the absence of any commercial or financial relationships that could be construed as a potential conflict of interest.
All claims expressed in this article are solely those of the authors and do not necessarily represent those of their affiliated organizations, or those of the publisher, the editors and the reviewers. Any product that may be evaluated in this article, or claim that may be made by its manufacturer, is not guaranteed or endorsed by the publisher.
The Supplementary Material for this article can be found online at: https://www.frontiersin.org/articles/10.3389/fcell.2023.1021920/full#supplementary-material
Aicher, J. K., Jewell, P., Vaquero-Garcia, J., Barash, Y., and Bhoj, E. J. (2020). Mapping RNA splicing variations in clinically accessible and nonaccessible tissues to facilitate Mendelian disease diagnosis using RNA-seq. Genet. Med. Off. J. Am. Coll. Med. Genet. 22, 1181–1190. doi:10.1038/s41436-020-0780-y
Alston, C. L., Stenton, S. L., Hudson, G., Prokisch, H., and Taylor, R. W. (2021). The genetics of mitochondrial disease: Dissecting mitochondrial pathology using multi-omic pipelines. J. Pathol. 254, 430–442. doi:10.1002/path.5641
Al-Zaidy, S. A., Malik, V., Kneile, K., Rosales, X. Q., Gomez, A. M., Lewis, S., et al. (2015). A slowly progressive form of limb-girdle muscular dystrophy type 2C associated with founder mutation in the SGCG gene in Puerto Rican Hispanics. Mol. Genet. Genomic Med. 3, 92–98. doi:10.1002/mgg3.125
Amarasinghe, S. L., Su, S., Dong, X., Zappia, L., Ritchie, M. E., and Gouil, Q. (2020). Opportunities and challenges in long-read sequencing data analysis. Genome Biol. 21, 30. doi:10.1186/s13059-020-1935-5
Aref-Eshghi, E., Kerkhof, J., Pedro, V. P., Barat-Houari, M., Ruiz-Pallares, N., et al. DI France Groupe (2020). Evaluation of DNA methylation episignatures for diagnosis and phenotype correlations in 42 mendelian neurodevelopmental disorders. Am. J. Hum. Genet. 106, 356–370. doi:10.1016/j.ajhg.2020.01.019
Belkadi, A., Bolze, A., Itan, Y., Cobat, A., Vincent, Q. B., Antipenko, A., et al. (2015). Whole-genome sequencing is more powerful than whole-exome sequencing for detecting exome variants. Proc. Natl. Acad. Sci. U. S. A. 112, 5473–5478. doi:10.1073/pnas.1418631112
Besnard, T., Sloboda, N., Goldenberg, A., Küry, S., Cogné, B., Breheret, F., et al. (2019). Biallelic pathogenic variants in the lanosterol synthase gene LSS involved in the cholesterol biosynthesis cause alopecia with intellectual disability, a rare recessive neuroectodermal syndrome. Genet. Med. Off. J. Am. Coll. Med. Genet. 21, 2025–2035. doi:10.1038/s41436-019-0445-x
Bodle, E. E., Zhu, W., Velez-Bartolomei, F., Tesi-Rocha, A., Liu, P., and Bernstein, J. A. (2021). Combined genome sequencing and RNA analysis reveals and characterizes a deep intronic variant in IGHMBP2 in a patient with spinal muscular atrophy with respiratory distress type 1. Pediatr. Neurol. 114, 16–20. doi:10.1016/j.pediatrneurol.2020.09.011
Boeva, V., Popova, T., Bleakley, K., Chiche, P., Cappo, J., Schleiermacher, G., et al. (2012). Control-FREEC: A tool for assessing copy number and allelic content using next-generation sequencing data. Bioinforma. Oxf. Engl. 28, 423–425. doi:10.1093/bioinformatics/btr670
Bose, P., Hermetz, K. E., Conneely, K. N., and Rudd, M. K. (2014). Tandem repeats and G-rich sequences are enriched at human CNV breakpoints. PLoS ONE 9, e101607. doi:10.1371/journal.pone.0101607
Boycott, K. M., Hartley, T., Biesecker, L. G., Gibbs, R. A., Innes, A. M., Riess, O., et al. (2019). A diagnosis for all rare genetic diseases: The horizon and the next Frontiers. Cell 177, 32–37. doi:10.1016/j.cell.2019.02.040
Brechtmann, F., Mertes, C., Matusevičiūtė, A., Yépez, V. A., Avsec, Ž., Herzog, M., et al. (2018). Outrider: A statistical method for detecting aberrantly expressed genes in RNA sequencing data. Am. J. Hum. Genet. 103, 907–917. doi:10.1016/j.ajhg.2018.10.025
Bronstein, R., Capowski, E. E., Mehrotra, S., Jansen, A. D., Navarro-Gomez, D., Maher, M., et al. (2020). A combined RNA-seq and whole genome sequencing approach for identification of non-coding pathogenic variants in single families. Hum. Mol. Genet. 29, 967–979. doi:10.1093/hmg/ddaa016
Bruel, A.-L., Vitobello, A., Tran Mau-Them, F., Nambot, S., Sorlin, A., Denommé-Pichon, A.-S., et al. (2020). Next-generation sequencing approaches and challenges in the diagnosis of developmental anomalies and intellectual disability. Clin. Genet. 98, 433–444. doi:10.1111/cge.13764
Bryen, S. J., Oates, E. C., Evesson, F. J., Lu, J. K., Waddell, L. B., Joshi, H., et al. (2021). Pathogenic deep intronic MTM1 variant activates a pseudo-exon encoding a nonsense codon resulting in severe X-linked myotubular myopathy. Eur. J. Hum. Genet. EJHG 29, 61–66. doi:10.1038/s41431-020-00715-7
Caspar, S. M., Dubacher, N., Kopps, A. M., Meienberg, J., Henggeler, C., and Matyas, G. (2018). Clinical sequencing: From raw data to diagnosis with lifetime value. Clin. Genet. 93, 508–519. doi:10.1111/cge.13190
Chaisson, M. J. P., Sanders, A. D., Zhao, X., Malhotra, A., Porubsky, D., Rausch, T., et al. (2019). Multi-platform discovery of haplotype-resolved structural variation in human genomes. Nat. Commun. 10, 1784. doi:10.1038/s41467-018-08148-z
Chan, S., Lam, E., Saghbini, M., Bocklandt, S., Hastie, A., Cao, H., et al. (2018). Structural variation detection and analysis using Bionano optical mapping. Methods Mol. Biol. Clifton N. J. 1833, 193–203. doi:10.1007/978-1-4939-8666-8_16
Cingolani, P., Platts, A., Wang, L. L., Coon, M., Nguyen, T., Wang, L., et al. (2012). A program for annotating and predicting the effects of single nucleotide polymorphisms, SnpEff: SNPs in the genome of Drosophila melanogaster strain w1118; iso-2; iso-3. Fly. (Austin) 6, 80–92. doi:10.4161/fly.19695
Clark, M. M., Stark, Z., Farnaes, L., Tan, T. Y., White, S. M., Dimmock, D., et al. (2018). Meta-analysis of the diagnostic and clinical utility of genome and exome sequencing and chromosomal microarray in children with suspected genetic diseases. NPJ Genomic Med. 3, 16. doi:10.1038/s41525-018-0053-8
Colin, E., Duffourd, Y., Tisserant, E., Relator, R., Bruel, A-L., Tran Mau-Them, F., et al. (2022). Omixcare: OMICS technologies solved about 33% of the patients with heterogeneous rare neuro developmental disorders and negative exome sequencing results and identified 13% additional candidate variants. Front. Cell Dev. Biol. 10, 1021785. doi:10.3389/fcell.2022.1021785
Cummings, B. B., Karczewski, K. J., Kosmicki, J. A., Seaby, E. G., Watts, N. A., Singer-Berk, M., et al. (2020). Transcript expression-aware annotation improves rare variant interpretation. Nature. 581, 452–458. doi:10.1038/s41586-020-2329-2
Cummings, B. B., Marshall, J. L., Tukiainen, T., Lek, M., Donkervoort, S., Foley, A. R., et al. (2017). Improving genetic diagnosis in Mendelian disease with transcriptome sequencing. Sci. Transl. Med. 9, eaal5209. doi:10.1126/scitranslmed.aal5209
de Bruijn, S. E., Fiorentino, A., Ottaviani, D., Fanucchi, S., Melo, U. S., Corral-Serrano, J. C., et al. (2020). Structural variants create New topological-associated domains and ectopic retinal enhancer-gene contact in dominant retinitis pigmentosa. Am. J. Hum. Genet. 107, 802–814. doi:10.1016/j.ajhg.2020.09.002
DePristo, M. A., Banks, E., Poplin, R., Garimella, K. V., Maguire, J. R., Hartl, C., et al. (2011). A framework for variation discovery and genotyping using next-generation DNA sequencing data. Nat. Genet. 43, 491–498. doi:10.1038/ng.806
DiCapua, D., and Patwa, H. (2014). Puerto Rican founder mutation G787A in the SGCG gene: A case report of 2 siblings with LGMD 2C. J. Clin. Neuromuscul. Dis. 15, 105–107. doi:10.1097/CND.0000000000000018
Dobin, A., Davis, C. A., Schlesinger, F., Drenkow, J., Zaleski, C., Jha, S., et al. (2013). Star: Ultrafast universal RNA-seq aligner. Bioinforma. Oxf. Engl. 29, 15–21. doi:10.1093/bioinformatics/bts635
Frésard, L., and Montgomery, S. B. (2018). Diagnosing rare diseases after the exome. Cold Spring Harb. Mol. Case Stud. 4, a003392. doi:10.1101/mcs.a003392
Frésard, L., Smail, C., Ferraro, N. M., Teran, N. A., Li, X., Smith, K. S., et al. (2019). Identification of rare-disease genes using blood transcriptome sequencing and large control cohorts. Nat. Med. 25, 911–919. doi:10.1038/s41591-019-0457-8
Frith, M. C., Hamada, M., and Horton, P. (2010). Parameters for accurate genome alignment. BMC Bioinforma. 11, 80. doi:10.1186/1471-2105-11-80
Frith, M. C., Mitsuhashi, S., and Katoh, K. (2021). lamassemble: Multiple alignment and consensus sequence of long reads. Methods Mol. Biol. Clifton N. J. 2231, 135–145. doi:10.1007/978-1-0716-1036-7_9
Gilissen, C., Hehir-Kwa, J. Y., Thung, D. T., van de Vorst, M., van Bon, B. W. M., Willemsen, M. H., et al. (2014). Genome sequencing identifies major causes of severe intellectual disability. Nature 511, 344–347. doi:10.1038/nature13394
Gonorazky, H. D., Naumenko, S., Ramani, A. K., Nelakuditi, V., Mashouri, P., Wang, P., et al. (2019). Expanding the boundaries of RNA sequencing as a diagnostic tool for rare mendelian disease. Am. J. Hum. Genet. 104, 466–483. doi:10.1016/j.ajhg.2019.01.012
Hamanaka, K., Miyatake, S., Koshimizu, E., Tsurusaki, Y., Mitsuhashi, S., Iwama, K., et al. (2019). RNA sequencing solved the most common but unrecognized NEB pathogenic variant in Japanese nemaline myopathy. Genet. Med. Off. J. Am. Coll. Med. Genet. 21, 1629–1638. doi:10.1038/s41436-018-0360-6
Hartley, T., Balcı, T. B., Rojas, S. K., Eaton, A., Canada, C. R., and Dyment, D. A., (2018). The unsolved rare genetic disease atlas? An analysis of the unexplained phenotypic descriptions in OMIM®. Am. J. Med. Genet. C Semin. Med. Genet. 178, 458–463. doi:10.1002/ajmg.c.31662
Hartley, T., Lemire, G., Kernohan, K. D., Howley, H. E., Adams, D. R., and Boycott, K. M. (2020). New diagnostic approaches for undiagnosed rare genetic diseases. Annu. Rev. Genomics Hum. Genet. 21, 351–372. doi:10.1146/annurev-genom-083118-015345
Jenkinson, G., Li, Y. I., Basu, S., Cousin, M. A., Oliver, G. R., and Klee, E. W. (2020). LeafCutterMD: An algorithm for outlier splicing detection in rare diseases. Bioinformatics 36, 4609–4615. doi:10.1093/bioinformatics/btaa259
Kobren, S. N., Baldridge, D., Velinder, M., Krier, J. B., LeBlanc, K., Esteves, C., et al. (2021). Commonalities across computational workflows for uncovering explanatory variants in undiagnosed cases. Genet. Med. Off. J. Am. Coll. Med. Genet. 23, 1075–1085. doi:10.1038/s41436-020-01084-8
Köhler, S., Carmody, L., Vasilevsky, N., Jacobsen, J. O. B., Danis, D., Gourdine, J.-P., et al. (2019). Expansion of the human phenotype Ontology (HPO) knowledge base and resources. Nucleic Acids Res. 47, D1018–D1027. doi:10.1093/nar/gky1105
Kremer, L. S., Bader, D. M., Mertes, C., Kopajtich, R., Pichler, G., Iuso, A., et al. (2017). Genetic diagnosis of Mendelian disorders via RNA sequencing. Nat. Commun. 8, 15824. doi:10.1038/ncomms15824
Kremer, L. S., Wortmann, S. B., and Prokisch, H. (2018). Transcriptomics”: Molecular diagnosis of inborn errors of metabolism via RNA-sequencing. J. Inherit. Metab. Dis. 41, 525–532. doi:10.1007/s10545-017-0133-4
Layer, R. M., Chiang, C., Quinlan, A. R., and Hall, I. M. (2014). Lumpy: A probabilistic framework for structural variant discovery. Genome Biol. 15, R84. doi:10.1186/gb-2014-15-6-r84
Lee, H., Huang, A. Y., Wang, L.-K., Yoon, A. J., Renteria, G., Eskin, A., et al. (2020). Diagnostic utility of transcriptome sequencing for rare Mendelian diseases. Genet. Med. Off. J. Am. Coll. Med. Genet. 22, 490–499. doi:10.1038/s41436-019-0672-1
Lelieveld, S. H., Spielmann, M., Mundlos, S., Veltman, J. A., and Gilissen, C. (2015). Comparison of exome and genome sequencing technologies for the complete capture of protein-coding regions. Hum. Mutat. 36, 815–822. doi:10.1002/humu.22813
Levy, M. A., McConkey, H., Kerkhof, J., Barat-Houari, M., Bargiacchi, S., Biamino, E., et al. (2022). Novel diagnostic DNA methylation episignatures expand and refine the epigenetic landscapes of Mendelian disorders. HGG Adv. 3, 100075. doi:10.1016/j.xhgg.2021.100075
Li, H., and Durbin, R. (2009). Fast and accurate short read alignment with Burrows-Wheeler transform. Bioinforma. Oxf. Engl. 25, 1754–1760. doi:10.1093/bioinformatics/btp324
Li, H., Handsaker, B., Wysoker, A., Fennell, T., Ruan, J., Homer, N., et al. (2009). 1000 the sequence alignment/map format and SAMtools. Bioinforma. Oxf. Engl. 25, 2078–2079. doi:10.1093/bioinformatics/btp352
Logsdon, G. A., Vollger, M. R., and Eichler, E. E. (2020). Long-read human genome sequencing and its applications. Nat. Rev. Genet. 21, 597–614. doi:10.1038/s41576-020-0236-x
Lonsdale, J., Thomas, J., Salvatore, M., Phillips, R., Lo, E., Shad, S., et al. (2013). The genotype-tissue expression (GTEx) project. Nat. Genet. 45, 580–585. doi:10.1038/ng.2653
Love, M. I., Huber, W., and Anders, S. (2014). Moderated estimation of fold change and dispersion for RNA-seq data with DESeq2. Genome Biol. 15, 550. doi:10.1186/s13059-014-0550-8
Mahmoud, M., Gobet, N., Cruz-Dávalos, D. I., Mounier, N., Dessimoz, C., and Sedlazeck, F. J. (2019). Structural variant calling: The long and the short of it. Genome Biol. 20, 246. doi:10.1186/s13059-019-1828-7
Marshall, C. R., Bick, D., Belmont, J. W., Taylor, S. L., Ashley, E., Dimmock, D., et al. (2020). The medical genome initiative: Moving whole-genome sequencing for rare disease diagnosis to the clinic. Genome Med. 12, 48. doi:10.1186/s13073-020-00748-z
Mehmood, A., Laiho, A., Venäläinen, M. S., McGlinchey, A. J., Wang, N., and Elo, L. L. (2020). Systematic evaluation of differential splicing tools for RNA-seq studies. Brief. Bioinform. 21, 2052–2065. doi:10.1093/bib/bbz126
Meienberg, J., Bruggmann, R., Oexle, K., and Matyas, G. (2016). Clinical sequencing: Is WGS the better WES? Hum. Genet. 135, 359–362. doi:10.1007/s00439-015-1631-9
Melo, U. S., Schöpflin, R., Acuna-Hidalgo, R., Mensah, M. A., Fischer-Zirnsak, B., Holtgrewe, M., et al. (2020). Hi-C identifies complex genomic rearrangements and TAD-shuffling in developmental diseases. Am. J. Hum. Genet. 106, 872–884. doi:10.1016/j.ajhg.2020.04.016
Mitsuhashi, S., and Matsumoto, N. (2020). Long-read sequencing for rare human genetic diseases. J. Hum. Genet. 65, 11–19. doi:10.1038/s10038-019-0671-8
Mitsuhashi, S., Ohori, S., Katoh, K., Frith, M. C., and Matsumoto, N. (2020). A pipeline for complete characterization of complex germline rearrangements from long DNA reads. Genome Med. 12, 67. doi:10.1186/s13073-020-00762-1
Murdock, D. R., Dai, H., Burrage, L. C., Rosenfeld, J. A., Ketkar, S., Müller, M. F., et al. (2021). Transcriptome-directed analysis for Mendelian disease diagnosis overcomes limitations of conventional genomic testing. J. Clin. Invest. 131, 141500. doi:10.1172/JCI141500
Peymani, F., Farzeen, A., and Prokisch, H. (2022). RNA sequencing role and application in clinical diagnostic. Pediatr. Investig. 6, 29–35. doi:10.1002/ped4.12314
Rentas, S., Rathi, K. S., Kaur, M., Raman, P., Krantz, I. D., Sarmady, M., et al. (2020). Diagnosing Cornelia de Lange syndrome and related neurodevelopmental disorders using RNA sequencing. Genet. Med. Off. J. Am. Coll. Med. Genet. 22, 927–936. doi:10.1038/s41436-019-0741-5
Richards, S., Aziz, N., Bale, S., Bick, D., Das, S., Gastier-Foster, J., et al. (2015). ACMG laboratory quality assurance CommitteeStandards and guidelines for the interpretation of sequence variants: A joint consensus recommendation of the American college of medical genetics and genomics and the association for molecular pathology. Genet. Med. Off. J. Am. Coll. Med. Genet. 17, 405–424. doi:10.1038/gim.2015.30
Ross, M. G., Russ, C., Costello, M., Hollinger, A., Lennon, N. J., Hegarty, R., et al. (2013). Characterizing and measuring bias in sequence data. Genome Biol. 14, R51. doi:10.1186/gb-2013-14-5-r51
Sadikovic, B., Levy, M. A., Kerkhof, J., Aref-Eshghi, E., Schenkel, L., Stuart, A., et al. (2021). Clinical epigenomics: Genome-wide DNA methylation analysis for the diagnosis of mendelian disorders. Genet. Med. Off. J. Am. Coll. Med. Genet. 23, 1065–1074. doi:10.1038/s41436-020-01096-4
Sanchis-Juan, A., Stephens, J., French, C. E., Gleadall, N., Mégy, K., Penkett, C., et al. (2018). Complex structural variants in mendelian disorders: Identification and breakpoint resolution using short- and long-read genome sequencing. Genome Med. 10, 95. doi:10.1186/s13073-018-0606-6
Sanlaville, D., Vidaud, M., Thauvin-Robinet, C., Nowak, F., and Lethimonnier, F. (2021). French genomic medicine plan 2025 (PFMG2025): France enters the era of genomic medicine. Rev. Prat. 71, 1061–1064.
Sawyer, S. L., Hartley, T., Dyment, D. A., Beaulieu, C. L., Schwartzentruber, J., Smith, A., et al. (2016). Utility of whole-exome sequencing for those near the end of the diagnostic odyssey: Time to address gaps in care. Clin. Genet. 89, 275–284. doi:10.1111/cge.12654
Sedlazeck, F. J., Lee, H., Darby, C. A., and Schatz, M. C. (2018). Piercing the dark matter: Bioinformatics of long-range sequencing and mapping. Nat. Rev. Genet. 19, 329–346. doi:10.1038/s41576-018-0003-4
Shahjaman, M., Manir Hossain Mollah, M., Rezanur Rahman, M., Islam, S. M. S., and Nurul Haque Mollah, M. (2020). Robust identification of differentially expressed genes from RNA-seq data. Genomics 112, 2000–2010. doi:10.1016/j.ygeno.2019.11.012
Shen, S., Park, J. W., Lu, Z., Lin, L., Henry, M. D., Wu, Y. N., et al. (2014). rMATS: Robust and flexible detection of differential alternative splicing from replicate RNA-Seq data. Proc. Natl. Acad. Sci. 111, E5593–E5601. doi:10.1073/pnas.1419161111
Short, P. J., McRae, J. F., Gallone, G., Sifrim, A., Won, H., Geschwind, D. H., et al. (2018). De novo mutations in regulatory elements in neurodevelopmental disorders. Nature 555, 611–616. doi:10.1038/nature25983
Smedley, D., Smith, K. R., Martin, A., Thomas, E. A., McDonagh, E. M., Cipriani, V., et al. 100,000 Genomes Project Pilot Investigators (2021). 100,000 genomes pilot on rare-disease diagnosis in health care - preliminary report. N. Engl. J. Med. 385, 1868–1880. doi:10.1056/NEJMoa2035790
Stenton, S. L., and Prokisch, H. (2020). The clinical application of RNA sequencing in genetic diagnosis of mendelian disorders. Clin. Lab. Med. 40, 121–133. doi:10.1016/j.cll.2020.02.004
Yépez, V. A., Gusic, M., Kopajtich, R., Mertes, C., Smith, N. H., Alston, C. L., et al. (2022). Clinical implementation of RNA sequencing for Mendelian disease diagnostics. Genome Med. 14, 38. doi:10.1186/s13073-022-01019-9
Keywords: genome sequencing, RNA-seq, optical genome mapping, long-read sequencing, clinical diagnoses
Citation: Colin E, Duffourd Y, Chevarin M, Tisserant E, Verdez S, Paccaud J, Bruel A-L, Tran Mau-Them F, Denommé-Pichon A-S, Thevenon J, Safraou H, Besnard T, Goldenberg A, Cogné B, Isidor B, Delanne J, Sorlin A, Moutton S, Fradin M, Dubourg C, Gorce M, Bonneau D, El Chehadeh S, Debray F-G, Doco-Fenzy M, Uguen K, Chatron N, Aral B, Marle N, Kuentz P, Boland A, Olaso R, Deleuze J-F, Sanlaville D, Callier P, Philippe C, Thauvin-Robinet C, Faivre L and Vitobello A (2023) Stepwise use of genomics and transcriptomics technologies increases diagnostic yield in Mendelian disorders. Front. Cell Dev. Biol. 11:1021920. doi: 10.3389/fcell.2023.1021920
Received: 17 August 2022; Accepted: 30 January 2023;
Published: 28 February 2023.
Edited by:
Angela Peron, Santi Paolo e Carlo Hospital, ItalyReviewed by:
Naiara Akizu, Children’s Hospital of Philadelphia, United StatesCopyright © 2023 Colin, Duffourd, Chevarin, Tisserant, Verdez, Paccaud, Bruel, Tran Mau-Them, Denommé-Pichon, Thevenon, Safraou, Besnard, Goldenberg, Cogné, Isidor, Delanne, Sorlin, Moutton, Fradin, Dubourg, Gorce, Bonneau, El Chehadeh, Debray, Doco-Fenzy, Uguen, Chatron, Aral, Marle, Kuentz, Boland, Olaso, Deleuze, Sanlaville, Callier, Philippe, Thauvin-Robinet, Faivre and Vitobello. This is an open-access article distributed under the terms of the Creative Commons Attribution License (CC BY). The use, distribution or reproduction in other forums is permitted, provided the original author(s) and the copyright owner(s) are credited and that the original publication in this journal is cited, in accordance with accepted academic practice. No use, distribution or reproduction is permitted which does not comply with these terms.
*Correspondence: Estelle Colin, ZXNjb2xpbkBjaHUtYW5nZXJzLmZy; Antonio Vitobello, YW50b25pby52aXRvYmVsbG9AdS1ib3VyZ29nbmUuZnI=
†These authors have contributed equally to this work
Disclaimer: All claims expressed in this article are solely those of the authors and do not necessarily represent those of their affiliated organizations, or those of the publisher, the editors and the reviewers. Any product that may be evaluated in this article or claim that may be made by its manufacturer is not guaranteed or endorsed by the publisher.
Research integrity at Frontiers
Learn more about the work of our research integrity team to safeguard the quality of each article we publish.