- Laboratory of Physical Chemistry and Soft Matter, Wageningen University and Research, Wageningen, Netherlands
Cells residing in living tissues apply forces to their immediate surroundings to promote the restructuration of the extracellular matrix fibres and to transmit mechanical signals to other cells. Here we use a minimalist model to study how these forces, applied locally by cell contraction, propagate through the fibrous network in the extracellular matrix. In particular, we characterize how the transmission of forces is influenced by the connectivity of the network and by the bending rigidity of the fibers. For highly connected fiber networks the stresses spread out isotropically around the cell over a distance that first increases with increasing contraction of the cell and then saturates at a characteristic length. For lower connectivity, however, the stress pattern is highly asymmetric and is characterised by force chains that can transmit stresses over very long distances. We hope that our analysis of force transmission in fibrous networks can provide a new avenue for future studies on how the mechanical feedback between the cell and the ECM is coupled with the microscopic environment around the cells.
1 Introduction
Living tissues are constituted by the extracellular matrix (ECM), a complex network of proteins and polysaccharides that gives structural support to surrounding cells. In animal tissues, the main component of the ECM is collagen, which forms a crosslinked network of stiff fibres that provides the ECM with its elasticity and mechanical strength (Mouw et al., 2014; Burla et al., 2019a). Cells are embedded within this network and are linked to the matrix by focal adhesion complexes (FAs), which act as physical anchors via which cells can mechanically interact with their environment (Totsukawa et al., 2004; Lecuit et al., 2011). Indeed, many cellular processes are regulated by mechanical feedback between cells and the ECM. Cells actively exert forces on the surrounding matrix, leading to structural reorganisations in the surrounding network, like fibre alignment, plastic rearrangements, and densification around the cell (Vader et al., 2009; Kim et al., 2017; Sopher et al., 2018; Goren et al., 2020). Cells also sense the mechanical properties of the surrounding medium. For example, cancer cells exhibit a preferential migration to regions with higher stiffness (durotaxis) (Lo et al., 2000; DuChez et al., 2019; Rens and Merks, 2020), and can adapt their shape as a function of the matrix stiffness (Koch et al., 2012). Likewise, wound healing requires contractile forces applied by myofibroblasts around the injured zone (Li and Wang, 2011). Cells also use mechanical signals to communicate with other cells; they actively exert forces on the surrounding matrix, transmitted through the ECM to distant cells (Reinhart-King et al., 2008; Winer et al., 2009; Han et al., 2018). This mechanical signalling is believed to play an essential role in tissue development, as well as in the development of cancer and other diseases (Bates et al., 2007; Hinz et al., 2012). Therefore, understanding how forces propagate in the extracellular matrix is relevant for obtaining fundamental knowledge about biological processes in both healthy and pathological tissue. Likewise, this insight can be revealing in tissue engineering, where tailoring the mechanical properties and cell-matrix interactions are crucial for the successful development of artificial tissues and organs (Chen et al., 2004; Causa et al., 2007; Wegst et al., 2015).
The challenge in describing mechanical signal propagation through the ECM is that the ECM is a very heterogeneous fibre network, with a typical mesh size that is comparable to the size of the cell. This means that continuum theories cannot be used (Notbohm et al., 2015; Ronceray et al., 2016; Han et al., 2018). The heterogeneity of the fibre network is regulated by the network connectivity z and the bending rigidity of the fibres, which thereby influence the mechanical response of the ECM. It is well-known that networks with only central-force interactions become mechanically stable only when the connectivity exceeds a critical threshold known as the isostatic point, which has been shown by Maxwell to be equal to zc = 2d, with d the spatial dimensionality (Maxwell, 1864). However, the extracellular fibre networks surrounding cells have a lower connectivity, ranging from z = 3 for branched networks to z = 4 for cross-linked fibres. In particular, collagen networks exhibit an average connectivity
To understand mechanical signalling between cells in the ECM, it is thus necessary to develop a model for force propagation that incorporates the disordered network structure and its mechanical nonlinearity. To do this, we employ a minimalist model based on two-dimensional triangular athermal networks, where the disorder is induced by controlling the connectivity. Such network models have been shown to give a very accurate description of the mechanics of collagen networks (Licup et al., 2015; Sharma et al., 2016a; Burla et al., 2020). To model an embedded cell, we incorporate a rigid circular body, which shrinks in area, generating local compression. We then examine how forces propagate from the contracting cell through the network, using concepts from network theory. Our findings reveal that the propagation in the case of high connectivity is isotropic and limited when the surrounding network around the cell is highly stressed. By contrast, asymmetry emerges at low connectivity, and the transmission achieves larger distances. The bending rigidity in this regime has a more pronounced role in controlling the force transmission.
2 Modeling
We perform numerical simulations on 2D diluted triangular networks of N × N nodes, with N = 100, and with spacing l0. Periodic boundary conditions are applied in all directions. We dilute the lattice by randomly removing bonds with probability 1 − p, and remove all dangling ends. This leads to an average network connectivity of
We model fibrous biopolymers such as collagen by considering stretching and bending rigidity. Thus, we consider every bond in the diluted network as a Hookean spring with stretching modulus μ, while sequences of contiguous colinear bonds have an associated bending rigidity κ. The Hamiltonian
where, in the first term, the sum runs over the bonded pairs
According to Maxwell’s rigidity criterion, the isostatic point (i.e., the connectivity below which the rigidity of the network vanishes) for these networks in the absence of bending stiffness (so for
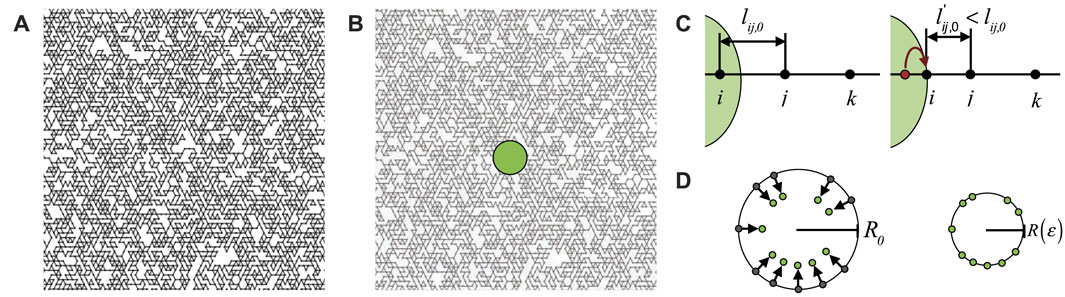
FIGURE 1. (A) Diluted triangular network with p = 0.55. (B) A circular model cell with radius R0 = 3l0 is placed in the center of the network (C) All bonds inside the cell are removed, while bonds that cross the cell surface are adjusted by moving the connected nodes at the cell interior to the cell surface, and adjusting the corresponding bond length lij,0. (D) Schematic showing the affine compression of the cell, by moving the nodes on the cell surface inward.
We then introduce a circular rigid body with radius R0 = 3l0 that mimics an embedded cell in the center of the network, as we show in Figure 1B, and we place nodes on the intersection points between the network and the surface of the cell, while adjusting the corresponding equilibrium bond lengths, see Figure 1C. Nodes at the interior of the cell are removed.
Finally, we induce an isotropic contraction of the cell body by applying affine deformation on nodes on the cell surface towards the cell center, as we schematically represent in Figure 1D. This local deformation is quantified by the strain
3 Results
3.1 Local Deformation in the Network
Cell contraction leads to mechanical stresses in the surrounding network. To investigate how these stresses propagate for different contractile strains, we identify the nodes in the network that have at least one stretched or compressed bond. Here we define a bond to be stretched or compressed when the corresponding force fij is equal to or greater than a threshold
where the brackets
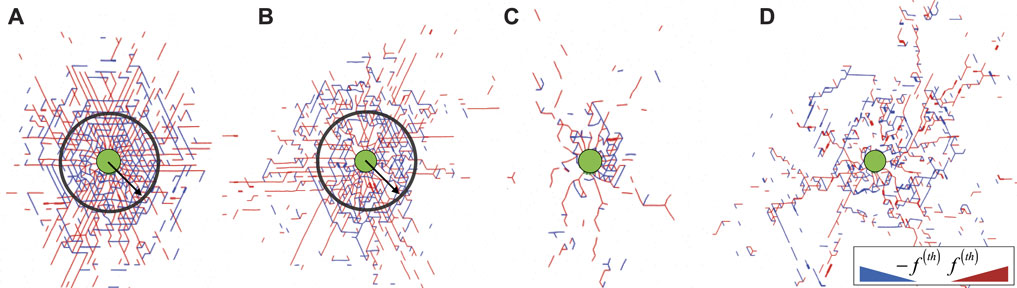
FIGURE 2. Snapshots for networks with (A) p = 0.85, (B) p = 0.75, (C) p = 0.65 and (D) p = 0.55, showing all compressed (
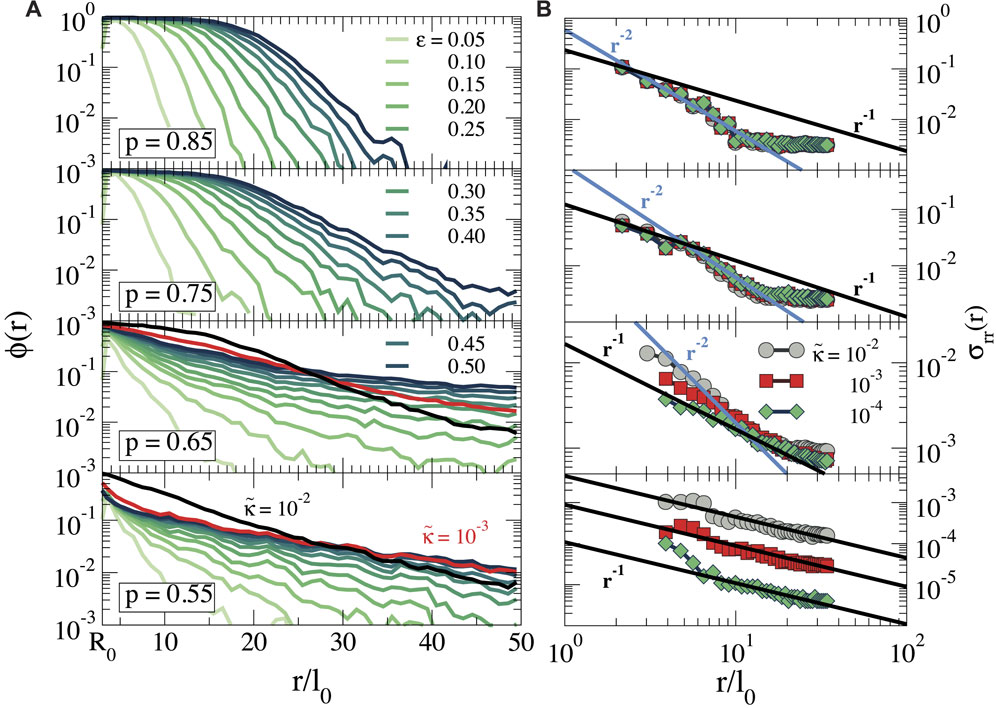
FIGURE 3. (A) Fraction of nodes at a distance r, that is, stretched or compressed,
We further characterize the propagation of forces generated by the cell by computing the local stress tensor at each bond connecting nodes i and j (Ronceray et al., 2016), defined as
where
3.2 Pattern of Force Transmission
Next, we study the pattern of the forces in more detail. We treat compressed and stretched bonds separately, as shown in Supplementary Figures S1A,B. Likewise, we only consider nodes connected to the cell surface via other deformed bonds. For each resulting cluster of deformed bonds, we compute the gyration tensor as
where ri,α is the α − coordinate of particle i and where the sum runs over all NG nodes that are part of the deformed cluster. We diagonalize the tensor obtaining the principal moments, λ1 and λ2 and from this we compute the radius of gyration
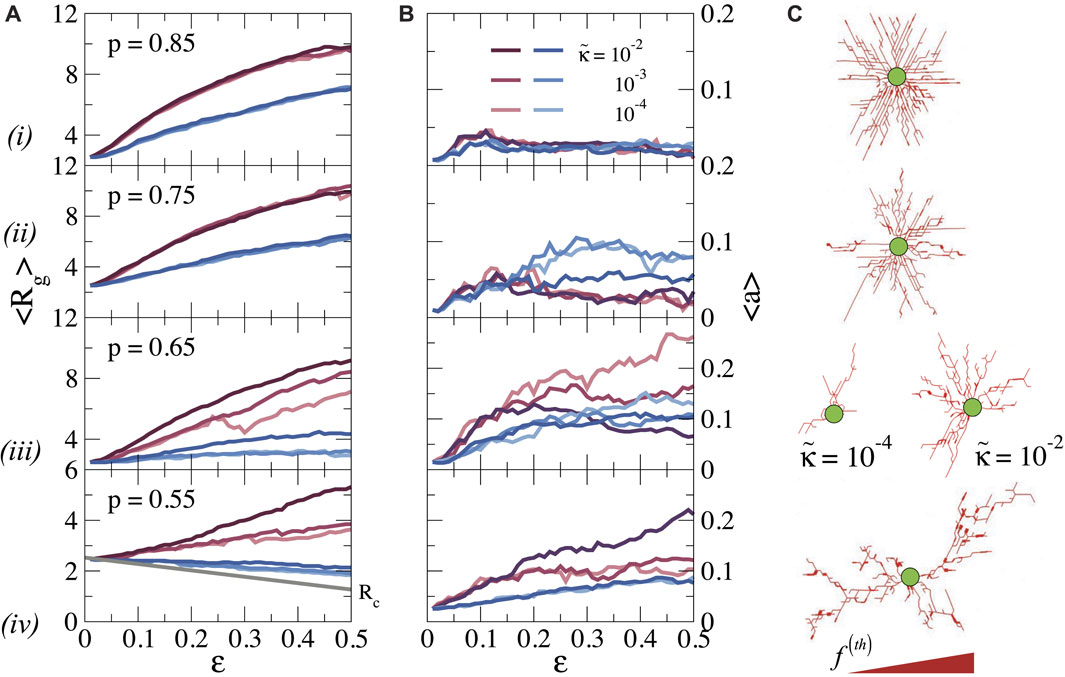
FIGURE 4. (A) Average radius of gyration Rg, and (B) asphericity a as a function of ϵ and bending rigidity
We first discuss the pattern of stretched bonds. For p ≥ 0.75 we find that Rg increases continuously with ϵ, preserving the spherical symmetry, as indicated by the low value of a. The growth of Rg with ϵ obviously is related to the growth of the stressed region seen in Figure 3A, and indicates how the stresses propagate further out as the cell contracts more. Again, the bending rigidity is unimportant in this stretching-dominated regime. When the connectivity is reduced to p = 0.65, we observe that Rg becomes dependent on
Repeating this analysis for the compressed bonds, we see that for all p values Rg is significantly smaller than for the stretched bonds; for p = 0.55, Rg even decreases with increasing strain, as the cell pulls the nodes inwards. Hence, compression forces do not propagate far away from the cell surface, especially for low p and the transmission of forces over long distances is dominated by stretched bonds.
We note, finally, that our previous observation that forces can propagate over longer distances in more sparsely connected networks (Figure 3) does not lead to a larger radius of gyration of the force patterns. This is because the stress propagation in the more distant regions for low connectivity is governed by a small number of force chains, which contribute less to Rg than the dense zone of stressed bonds at higher connectivity.
3.3 Force Chains in the Network
As is clear from the snapshots in Figures 2, 4C, the pattern of the local forces differs greatly between networks of high and low connectivity. While the forces radiate outwards more or less isotropically at high p, the pattern at lower p is characterized by so-called force chains, sequences of stretched bonds that can transmit forces over long distances in certain directions (Grill et al., 2021). To analyze the pattern of these force chains in more detail, we follow previous work that explored force transmission in granular systems using concepts of graph theory (Bassett et al., 2015; Newman, 2018). It is well-established that a granular material (i.e., a material consisting of jammed granular particles) can be mapped on an athermal network, with contact forces between neighbouring particles represented as bonds between nodes. The mechanical properties of such granular materials are characterized by discrete force chains that are very similar to the force chains observed in our simulations. Mechanical forces are transmitted along these force chains, while regions between the force chains are shielded from mechanical stresses (Tordesillas, 2007; Somfai et al., 2005; Owens and Daniels, 2011).
To analyze the network of force chains, we start from the cluster of stretched bonds, as shown in Figure 4C, and we first identify the nodes at the end of each force chain, i.e., the nodes at the periphery of the cluster after which the force propagates no further (see also Supplementary Figure S1C). We then construct all simple paths, i.e., all non-repeating sequences of nodes (Newman, 2018), that connect each of the nodes at the periphery to the cell surface, obtaining thus a distribution of all force chains. In Figure 5 we show the distribution of the topological lengths of these force chains, for different strains and different connectivities. The top row shows only the shortest paths between each periphery node and the cell surface, as a measure for the typical distance over which the force propagates, while the bottom row shows the distribution of all simple paths. A difference between the distribution of shortest paths and all paths indicates the presence of many secondary paths due to cross-connections between force chains. Such cross-connections make the propagation of forces more robust, since the mechanical transmission does not rely on one single path, but multiple paths can transmit the force.
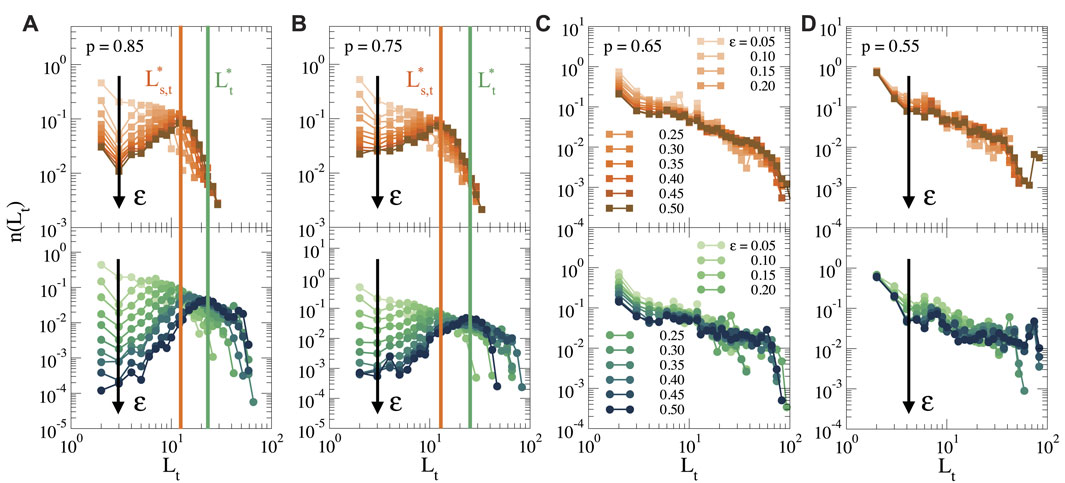
FIGURE 5. Topological length distribution of force chains that connect the periphery of the cluster to the cell surface for different strains (as indicated by the black arrow), and for (A) p = 0.85, (B) p = 0.75, (C) p = 0.65 and (D) p = 0.55. Top: Length distribution of the shortest paths, and bottom: length distribution of all simple paths. The vertical orange line indicates the characteristic shortest path
For p = 0.85 (Figure 5A) we see that at low strains, the distribution of path lengths decays monotonically, indicating that most of the force chains are short. However, when ϵ increases, the distribution acquires a clear optimum. This reveals that there is a characteristic length
To analyze the morphology of the force chain network in more detail, we plot the topological length of each force chain in the network Lt as a function of the Euclidean distance LE between the end of the force chain and the cell surface, see Figure 6 for p = 0.85 and 0.55, and Supplementary Figure S4 for p = 0.75 and 0.65. Here, Lt = LE corresponds to a straight force chain, while Lt > LE corresponds to a curved or irregular force chain Ref. (Bassett et al., 2015) (note that Lt cannot be smaller than LE, so that the grey area in Figure 6 is unphysical). For small strains (ϵ ≤ 0.10), we observe that Lt ≃ LE for all p. However, as ϵ increases, we find significant deviations from straight force chains for p = 0.85 and 0.75 (see Figure 6A and Supplementary Figure S3B, respectively), especially in the dense inner region, due to alternative paths that link the periphery of the force network to the cell surface. By contrast, for p = 0.65 and 0.55 and for larger
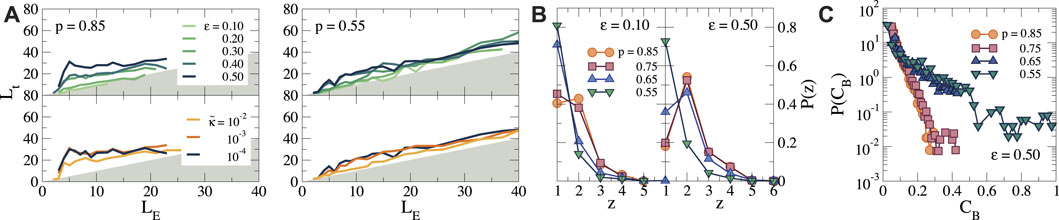
FIGURE 6. (A) The topological length Lt of the force chains as a function of the Euclidean distance that the force chain reaches LE, for different strains ϵ and bending rigidity
The complex structure of all simple force paths that emerges can be further analyzed by computing the distribution degree
Another way to characterize the force transmission in the networks is by analyzing the node betweenness centrality CB for each node in the network. This is a measure often used in graph theory to denote the importance of a certain node for transmission within a network, and is defined as (Brandes, 2001):
where ni,k is the number of shortest paths between nodes i and k, and where
4 Concluding Remarks
Mechanical communication between cells relies on force transmission over large distances through the extracellular matrix (Janmey and Miller, 2011). The disordered structure of the matrix, its large mesh size and its mechanical non-linearity make this a highly non-trivial process. Our results highlight how the connectivity of the fibre network and the bending rigidity of the collagen fibrils influence the local force transmission. On the one hand, for highly connected (and therefore relatively stiff) networks, the forces propagate isotropically in all directions over a characteristic distance that can be controlled by the contraction of the cell. On the other hand, in dilute (and soft) networks, forces propagate along a few force chains that can transmit forces over very long distances, but only in a few directions. This communication is less reliable and robust than for more rigid networks, which may be one of the reasons for the large variability and heterogeneity in cell behaviour in such matrices. In particular, networks close to the rigidity threshold (p = 0.65 in our case) are very sensitive to bending rigidity. These findings may be relevant for cell and tissue morphology and collective cell migration in environments of different rigidity. Indeed, our results are consistent with various experimental studies, which have reported that the distance over which cells can communicate mechanically appears to depend on the stiffness of the matrix (Guo et al., 2006; Reinhart-King et al., 2008; Winer et al., 2009; Janmey and Miller, 2011; Koorman et al., 2022). Furthermore, we speculate that the appearance of a characteristic transmission length that emerges around the cell when local stiffness increases may be related to the observation that cells in a colony organise at a typical distance from each other (Reinhardt-King et al., 2008).
We hope that this paper will provide an incentive for future research on force transmission in disordered networks, as many questions remain open. For example, we have considered only uniform cell contraction, but previous work has suggested that cells often contract anisotropically to influence the direction of stress propagation (Baker and Chen, 2012; Koch et al., 2012; Ahmadzadeh et al., 2017). Furthermore, we have here used a rigid contractile body to model the cell. It would be interesting to study how mechanical feedback between the matrix and the cell emerges when the cell is itself modelled as a soft deformable object, for example, by treating the perimeter of the cell as a ring of springs that can stretch and bend (Gandikota et al., 2020). In such cases the anisotropic force chains may lead to spontaneous polarization of the cell. Cells can also actively restructure the matrix around them by inducing plastic deformations and thus influencing the propagation of mechanical signals (Vader et al., 2009; Kim et al., 2017). In addition, mechanical signalling may be affected by the hydrodynamic coupling between the collagen fibrils and the embedding fluid (Yucht et al., 2013; Head and Storm, 2019), as well as by the complex network composed of polysaccharides and glycosylated proteins in which collagen fibrils are embedded (Mouw et al., 2014; Burla et al., 2019b). Finally, we emphasise that the networks that we have studied here are 2D. While such networks have been shown previously to be excellently suited for characterising the mechanical properties of experimental collagen networks experimentally (Sharma et al., 2016a; Sharma et al., 2016b), it would be interesting to observe how force transmission occurs in 3D networks, introducing thus an additional degree of freedom to relax the local deformation in the network.
Finally, we suggest the possibility of using graph theory to characterize the mechanical propagation and the local distortion generated by cells on the ECM. In addition to the characteristics used here, many additional descriptors can be used to characterize the network’s topology, also experimentally. These parameters may be used to train neural networks, for example, to develop a machine learning-based approach to identify cell-matrix and cell-cell interactions. This could eventually be used as a diagnostic tool or help to design synthetic matrices with optimal mechanical characteristics for mechanical feedback.
Data Availability Statement
The original contributions presented in the study are included in the article/Supplementary Material, further inquiries can be directed to the corresponding authors.
Author Contributions
All authors listed have made a substantial, direct, and intellectual contribution to the work and approved it for publication.
Funding
The authors would like to acknowledge the financial support by the European Research Council (Softbreak, grant agreement 682782).
Conflict of Interest
The authors declare that the research was conducted in the absence of any commercial or financial relationships that could be construed as a potential conflict of interest.
Publisher’s Note
All claims expressed in this article are solely those of the authors and do not necessarily represent those of their affiliated organizations, or those of the publisher, the editors and the reviewers. Any product that may be evaluated in this article, or claim that may be made by its manufacturer, is not guaranteed or endorsed by the publisher.
Supplementary Material
The Supplementary Material for this article can be found online at: https://www.frontiersin.org/articles/10.3389/fcell.2022.931776/full#supplementary-material
References
Ahmadzadeh, H., Webster, M. R., Behera, R., Jimenez Valencia, A. M., Wirtz, D., Weeraratna, A. T., et al. (2017). Modeling the Two-Way Feedback Between Contractility and Matrix Realignment Reveals a Nonlinear Mode of Cancer Cell Invasion. Proc. Natl. Acad. Sci. U. S. A. 114, E1617–E1626. doi:10.1073/pnas.1617037114
Arzash, S., Shivers, J. L., and MacKintosh, F. C. (2020). Finite Size Effects in Critical Fiber Networks. Soft Matter 16, 6784–6793. doi:10.1039/d0sm00764a
Baker, B. M., and Chen, C. S. (2012). Deconstructing the Third Dimension: How 3D Culture Microenvironments Alter Cellular Cues. J. Cell Sci. 125, 3015–3024. doi:10.1242/jcs.079509
Bassett, D. S., Owens, E. T., Porter, M. A., Manning, M. L., and Daniels, K. E. (2015). Extraction of Force-Chain Network Architecture in Granular Materials Using Community Detection. Soft Matter 11, 2731–2744. doi:10.1039/c4sm01821d
Bates, J. H. T., Davis, G. S., Majumdar, A., Butnor, K. J., and Suki, B. (2007). Linking Parenchymal Disease Progression to Changes in Lung Mechanical Function by Percolation. Am. J. Respir. Crit. Care Med. 176, 617–623. doi:10.1164/rccm.200611-1739oc
Bitzek, E., Koskinen, P., Gähler, F., Moseler, M., and Gumbsch, P. (2006). Structural Relaxation Made Simple. Phys. Rev. Lett. 97, 170201. doi:10.1103/physrevlett.97.170201
Brandes, U. (2001). A Faster Algorithm for Betweenness Centrality*. J. Math. Sociol. 25, 163–177. doi:10.1080/0022250x.2001.9990249
Broedersz, C. P., and MacKintosh, F. C. (2014). Modeling Semiflexible Polymer Networks. Rev. Mod. Phys. 86, 995–1036. doi:10.1103/revmodphys.86.995
Broedersz, C. P., Mao, X., Lubensky, T. C., and MacKintosh, F. C. (2011). Criticality and Isostaticity in Fibre Networks. Nat. Phys. 7, 983–988. doi:10.1038/nphys2127
Burla, F., Dussi, S., Martinez-Torres, C., Tauber, J., van der Gucht, J., and Koenderink, G. H. (2020). Connectivity and Plasticity Determine Collagen Network Fracture. Proc. Natl. Acad. Sci. U.S.A. 117, 8326–8334. doi:10.1073/pnas.1920062117
Burla, F., Mulla, Y., Vos, B. E., Aufderhorst-Roberts, A., and Koenderink, G. H. (2019a). From Mechanical Resilience to Active Material Properties in Biopolymer Networks. Nat. Rev. Phys. 1, 249–263. doi:10.1038/s42254-019-0036-4
Burla, F., Tauber, J., Dussi, S., van Der Gucht, J., and Koenderink, G. H. (2019b). Stress Management in Composite Biopolymer Networks. Nat. Phys. 15, 549–553. doi:10.1038/s41567-019-0443-6
Causa, F., Netti, P. A., and Ambrosio, L. (2007). A Multi-Functional Scaffold for Tissue Regeneration: the Need to Engineer a Tissue Analogue. Biomaterials 28, 5093–5099. doi:10.1016/j.biomaterials.2007.07.030
Chen, C. S., Tan, J., and Tien, J. (2004). Mechanotransduction at Cell-Matrix and Cell-Cell Contacts. Annu. Rev. Biomed. Eng. 6, 275–302. doi:10.1146/annurev.bioeng.6.040803.140040
DuChez, B. J., Doyle, A. D., Dimitriadis, E. K., and Yamada, K. M. (2019). Durotaxis by Human Cancer Cells. Biophysical J. 116, 670–683. doi:10.1016/j.bpj.2019.01.009
Gandikota, M. C., Pogoda, K., Van Oosten, A., Engstrom, T. A., Patteson, A. E., Janmey, P. A., et al. (2020). Loops versus Lines and the Compression Stiffening of Cells. Soft matter 16, 4389–4406. doi:10.1039/c9sm01627a
Goren, S., Koren, Y., Xu, X., and Lesman, A. (2020). Elastic Anisotropy Governs the Range of Cell-Induced Displacements. Biophysical J. 118, 1152–1164. doi:10.1016/j.bpj.2019.12.033
Grill, M. J., Kernes, J., Slepukhin, V. M., Wall, W. A., and Levine, A. J. (2021). Directed Force Propagation in Semiflexible Networks. Soft Matter 17, 10223–10241. doi:10.1039/d0sm01177k
Guo, W.-h., Frey, M. T., Burnham, N. A., and Wang, Y.-l. (2006). Substrate Rigidity Regulates the Formation and Maintenance of Tissues. Biophysical J. 90, 2213–2220. doi:10.1529/biophysj.105.070144
Han, Y. L., Ronceray, P., Xu, G., Malandrino, A., Kamm, R. D., Lenz, M., et al. (2018). Cell Contraction Induces Long-Ranged Stress Stiffening in the Extracellular Matrix. Proc. Natl. Acad. Sci. U.S.A. 115, 4075–4080. doi:10.1073/pnas.1722619115
Head, D., and Storm, C. (2019). Nonaffinity and Fluid-Coupled Viscoelastic Plateau for Immersed Fiber Networks. Phys. Rev. Lett. 123, 238005. doi:10.1103/physrevlett.123.238005
Hinz, B., Phan, S. H., Thannickal, V. J., Prunotto, M., Desmoulière, A., Varga, J., et al. (2012). Recent Developments in Myofibroblast Biology: Paradigms for Connective Tissue Remodeling. Am. J. pathology 180, 1340–1355. doi:10.1016/j.ajpath.2012.02.004
Janmey, P. A., and Miller, R. T. (2011). Mechanisms of Mechanical Signaling in Development and Disease. J. cell Sci. 124, 9–18. doi:10.1242/jcs.071001
Jansen, K. A., Licup, A. J., Sharma, A., Rens, R., MacKintosh, F. C., and Koenderink, G. H. (2018). The Role of Network Architecture in Collagen Mechanics. Biophysical J. 114, 2665–2678. doi:10.1016/j.bpj.2018.04.043
Jones, C. A., Cibula, M., Feng, J., Krnacik, E. A., McIntyre, D. H., Levine, H., et al. (2015). Micromechanics of Cellularized Biopolymer Networks. Proc. Natl. Acad. Sci. U. S. A. 112, E5117–E5122. doi:10.1073/pnas.1509663112
Kim, J., Feng, J., Jones, C. A. R., Mao, X., Sander, L. M., Levine, H., et al. (2017). Stress-induced Plasticity of Dynamic Collagen Networks. Nat. Commun. 8, 842–847. doi:10.1038/s41467-017-01011-7
Koch, T. M., Münster, S., Bonakdar, N., Butler, J. P., and Fabry, B. (2012). 3d Traction Forces in Cancer Cell Invasion. Plos one 7, e33476. doi:10.1371/journal.pone.0033476
Koorman, T., Jansen, K. A., Khalil, A., Haughton, P. D., Visser, D., Rätze, M. A., et al. (2022). Spatial Collagen Stiffening Promotes Collective Breast Cancer Cell Invasion by Reinforcing Extracellular Matrix Alignment. Oncogene 43, 1–12. doi:10.1038/s41388-022-02258-1
Lecuit, T., Lenne, P.-F., and Munro, E. (2011). Force Generation, Transmission, and Integration during Cell and Tissue Morphogenesis. Annu. Rev. Cell Dev. Biol. 27, 157–184. doi:10.1146/annurev-cellbio-100109-104027
Li, B., and Wang, J. H.-C. (2011). Fibroblasts and Myofibroblasts in Wound Healing: Force Generation and Measurement. J. tissue viability 20, 108–120. doi:10.1016/j.jtv.2009.11.004
Licup, A. J., Münster, S., Sharma, A., Sheinman, M., Jawerth, L. M., Fabry, B., et al. (2015). Stress Controls the Mechanics of Collagen Networks. Proc. Natl. Acad. Sci. U.S.A. 112, 9573–9578. doi:10.1073/pnas.1504258112
Lo, C.-M., Wang, H.-B., Dembo, M., and Wang, Y.-l. (2000). Cell Movement Is Guided by the Rigidity of the Substrate. Biophysical J. 79, 144–152. doi:10.1016/s0006-3495(00)76279-5
Maxwell, J. C. (1864). L. On the Calculation of the Equilibrium and Stiffness of Frames. Lond. Edinb. Dublin Philosophical Mag. J. Sci. 27, 294–299. doi:10.1080/14786446408643668
Mouw, J. K., Ou, G., and Weaver, V. M. (2014). Extracellular Matrix Assembly: a Multiscale Deconstruction. Nat. Rev. Mol. Cell Biol. 15, 771–785. doi:10.1038/nrm3902
Narmoneva, D. A., Wang, J. Y., and Setton, L. A. (1999). Nonuniform Swelling-Induced Residual Strains in Articular Cartilage. J. Biomechanics 32, 401–408. doi:10.1016/s0021-9290(98)00184-5
Notbohm, J., Lesman, A., Rosakis, P., Tirrell, D. A., and Ravichandran, G. (2015). Microbuckling of Fibrin Provides a Mechanism for Cell Mechanosensing. J. R. Soc. Interface. 12, 20150320. doi:10.1098/rsif.2015.0320
Owens, E. T., and Daniels, K. E. (2011). Sound Propagation and Force Chains in Granular Materials. Epl Europhys. Lett. 94, 54005. doi:10.1209/0295-5075/94/54005
Reinhart-King, C. A., Dembo, M., and Hammer, D. A. (2008). Cell-cell Mechanical Communication through Compliant Substrates. Biophysical J. 95, 6044–6051. doi:10.1529/biophysj.107.127662
Rens, E. G., and Merks, R. M. H. (2020). Cell Shape and Durotaxis Explained from Cell-Extracellular Matrix Forces and Focal Adhesion Dynamics. Iscience 23, 101488. doi:10.1016/j.isci.2020.101488
Rens, R., Vahabi, M., Licup, A. J., MacKintosh, F. C., and Sharma, A. (2016). Nonlinear Mechanics of Athermal Branched Biopolymer Networks. J. Phys. Chem. B 120, 5831–5841. doi:10.1021/acs.jpcb.6b00259
Ronceray, P., Broedersz, C. P., and Lenz, M. (2016). Fiber Networks Amplify Active Stress. Proc. Natl. Acad. Sci. U.S.A. 113, 2827–2832. doi:10.1073/pnas.1514208113
Sharma, A., Licup, A. J., Jansen, K. A., Rens, R., Sheinman, M., Koenderink, G. H., et al. (2016a). Strain-controlled Criticality Governs the Nonlinear Mechanics of Fibre Networks. Nat. Phys. 12, 584–587. doi:10.1038/nphys3628
Sharma, A., Licup, A. J., Rens, R., Vahabi, M., Jansen, K. A., Koenderink, G. H., et al. (2016b). Strain-driven Criticality Underlies Nonlinear Mechanics of Fibrous Networks. Phys. Rev. E 94, 042407. doi:10.1103/PhysRevE.94.042407
Somfai, E., Roux, J. N., Snoeijer, J. H., Van Hecke, M., and Van Saarloos, W. (2005). Elastic Wave Propagation in Confined Granular Systems. Phys. Rev. E Stat. Nonlin Soft Matter Phys. 72, 021301. doi:10.1103/PhysRevE.72.021301
Sopher, R. S., Tokash, H., Natan, S., Sharabi, M., Shelah, O., Tchaicheeyan, O., et al. (2018). Nonlinear Elasticity of the Ecm Fibers Facilitates Efficient Intercellular Communication. Biophysical J. 115, 1357–1370. doi:10.1016/j.bpj.2018.07.036
Tauber, J., Van Der Gucht, J., and Dussi, S. (2022). Stretchy and Disordered: Toward Understanding Fracture in Soft Network Materials via Mesoscopic Computer Simulations. J. Chem. Phys. 156, 160901. doi:10.1063/5.0081316
Tordesillas, A. (2007). Force Chain Buckling, Unjamming Transitions and Shear Banding in Dense Granular Assemblies. Philos. Mag. 87, 4987–5016. doi:10.1080/14786430701594848
Totsukawa, G., Wu, Y., Sasaki, Y., Hartshorne, D. J., Yamakita, Y., Yamashiro, S., et al. (2004). Distinct Roles of Mlck and Rock in the Regulation of Membrane Protrusions and Focal Adhesion Dynamics during Cell Migration of Fibroblasts. J. cell Biol. 164, 427–439. doi:10.1083/jcb.200306172
Vader, D., Kabla, A., Weitz, D., and Mahadevan, L. (2009). Strain-induced Alignment in Collagen Gels. Plos One 4, e5902. doi:10.1371/journal.pone.0005902
Wegst, U. G. K., Bai, H., Saiz, E., Tomsia, A. P., and Ritchie, R. O. (2015). Bioinspired Structural Materials. Nat. Mater 14, 23–36. doi:10.1038/nmat4089
Winer, J. P., Oake, S., and Janmey, P. A. (2009). Non-linear Elasticity of Extracellular Matrices Enables Contractile Cells to Communicate Local Position and Orientation. Plos One 4, e6382. doi:10.1371/journal.pone.0006382
Keywords: fiber networks, local stresses, force transmission, connectivity, bending rigidity, graph theory
Citation: Ruiz-Franco J and van Der Gucht J (2022) Force Transmission in Disordered Fibre Networks. Front. Cell Dev. Biol. 10:931776. doi: 10.3389/fcell.2022.931776
Received: 29 April 2022; Accepted: 06 June 2022;
Published: 30 June 2022.
Edited by:
Claudia Tanja Mierke, Leipzig University, GermanyCopyright © 2022 Ruiz-Franco and van Der Gucht. This is an open-access article distributed under the terms of the Creative Commons Attribution License (CC BY). The use, distribution or reproduction in other forums is permitted, provided the original author(s) and the copyright owner(s) are credited and that the original publication in this journal is cited, in accordance with accepted academic practice. No use, distribution or reproduction is permitted which does not comply with these terms.
*Correspondence: José Ruiz-Franco, jose.ruizfranco@wur.nl; Jasper van Der Gucht, jasper.vandergucht@wur.nl