- 1Division of General Surgery, Department of Surgery, Tri-Service General Hospital, National Defense Medical Center, Taipei, Taiwan
- 2PhD Program for Cancer Molecular Biology and Drug Discovery, College of Medical Science and Technology, Taipei Medical University and Academia Sinica, Taipei, Taiwan
- 3Graduate Institute for Cancer Biology and Drug Discovery, College of Medical Science and Technology, Taipei Medical University, Taipei, Taiwan
- 4Department of Otolaryngology-Head and Neck Surgery, Tri-Service General Hospital, National Defense Medical Center, Taipei, Taiwan
- 5Department of Biochemistry, Bingham University, Karu, Nigeria
- 6Medical Genetics Department, Faculty of Medicine, Umm al-Qura Univeristy, Mecca, Saudi Arabia
- 7Division of Hematology and Oncology Medicine, Department of Internal Medicine, Tri-Service General Hospital, National Defense Medical Center, Taipei, Taiwan
- 8Faculty of Medicine and Pharmacy, University of Oradea, Oradea, Romania
- 9Department of Pharmacology and Therapeutics, Faculty of Veterinary Medicine, Damanhour University, Damanhour, Egypt
- 10Department of Biotechnology, College of Science, Taif University, Taif, Saudi Arabia
- 11The PhD Program of Translational Medicine, College of Medical Science and Technology, Taipei Medical University, Taipei, Taiwan
- 12Clinical Research Center, Taipei Medical University Hospital, Taipei Medical University, Taipei, Taiwan
- 13TMU Research Center of Cancer Translational Medicine, Taipei Medical University, Taipei, Taiwan
- 14Graduate Institute of Medical Sciences, National Defense Medical Center, Taipei, Taiwan
Papillary thyroid carcinoma (PTC) is the most prevalent endocrine malignancy with a steadily increasing global incidence in recent decades. The pathogenesis of PTC is poorly understood, and the present diagnostic protocols are deficient. Thus, identifying novel prognostic biomarkers to improve our understanding of the mechanisms of pathogenesis, diagnosis, and designing therapeutic strategies for PTC is crucial. In this study, we integrated 27 PTC transcriptomic datasets and identified overlapping differentially expressed genes (DEGs) and differentially expressed microRNAs, collectively known as thyroid tumor-enriched proteins (TTEPs), and TTEmiRs, respectively. Our integrated bioinformatics analysis revealed that TTEPs were associated with tumor stages, poor surgical outcomes, distant metastasis, and worse prognoses in PTC cohorts. In addition, TTEPs were found to be associated with tumor immune infiltrating cells and immunosuppressive phenotypes of PTC. Enrichment analysis suggested the association of TTEPs with epithelial-to-mesenchymal transition (EMT), cell-matrix remodeling, and transcriptional dysregulation, while the TTEmiRs (miR-146b-5p and miR-21-5p) were associated with the modulation of the immune response, EMT, migration, cellular proliferation, and stemness. Molecular docking simulations were performed to evaluate binding affinities between TTEPs and antrocinnamomin, antcin, and antrocin, the bioactive compounds from one of the most reputable Taiwan indigenous medicinal plants (Antrodia camphorata). Our results revealed that antcin exhibited higher binding efficacies toward FN1, ETV5, and NRCAM, whereas antrocin demonstrated the least. Among the targets, fibronectin (FN1) demonstrated high ligandability potential for the compounds whereas NRCAM demonstrated the least. Collectively, our results hinted at the potential of antcin for targeting TTEPs. In conclusion, this comprehensive bioinformatics analysis strongly suggested that TTEPs and TTEmiRs could be used as potential diagnostic biomarker signatures and be exploited as potential targets for therapeutics development.
Introduction
Thyroid carcinoma (THCA) is a common endocrine malignancy. In recent decades, the global incidence of THCA has been on a steady increase, and for some countries, THCA is the fastest-growing malignant tumor (Chen et al., 2018). In 2020, 586,202 new cases and 43,646 deaths from THCA were reported globally, with a higher incidence in females (Sung et al., 2021). Histologically, THCA is categorized into anaplastic thyroid cancer (ATC), follicular thyroid carcinoma (FTC), and papillary thyroid carcinoma (PTC) (Luo et al., 2021). PTC and FTC are well-differentiated, accounting for more than 75% of all cases of thyroid cancers with an optimal prognosis of about 10-year disease-specific survival (Fagin and Wells, 2016). However, ATC is poorly differentiated with proliferative stem cell-like properties, resistance to present therapies (Knauf et al., 2005), and is responsible for most thyroid cancer-related deaths (Molinaro et al., 2017a). The etiology of thyroid cancer is not well understood. However, a rapid increase in PTC incidence has been primarily attributed to exposure to ionizing radiation via ultrasonography and diagnostic imaging modalities (Brito et al., 2013; Udelsman and Zhang, 2014). Also, accumulating evidence suggests that other factors, including hormonal exposures, obesity, metabolic syndromes, and environmental pollutants, contribute to the development of PTC-THCA (Kitahara et al., 2018).
The clinical management of PTC comprises multiple approaches, including surgery and chemo-radiotherapies (Molinaro et al., 2017b). However, accurate diagnosis before the initiation of treatment is fundamental to achieving a better prognosis for the patients. The present diagnostic protocols are suboptimal, resulting in over-diagnosing and over-treating patients with lower-risk diseases or benign thyroid nodules (Barrea, Gallo, Ruggeri, Giacinto, Sesti, Prinzi, Adinolfi, Barucca, Renzelli, Muscogiuri, et al.). At the same time, it is also essential to identify patients with high-risk and advanced stages of the disease for taking a more aggressive therapeutic approach (Durante et al., 2015). Based on these unmet clinical needs, identifying novel prognostic biomarkers represents a critical endeavor to improve our understanding of the mechanism of PTC pathogenesis, aid diagnosis, and develop therapeutic strategies.
MicroRNAs (miRNAs) are small non-coding RNAs of about 19–25 nucleotides, which regulate the translation of target mRNAs (Tanase et al., 2012). A single microRNA molecule can regulate the translation of several hundreds of genes to modulate several biological pathways (John et al., 2004). MiRNAs regulate the gene expression at the posttranscriptional level by base-pairing with complementary sequences, thereby degrading and inhibiting the translation of target mRNAs (Tran et al., 2017). Increasing evidence indicates that miRs are involved in the tumorigenesis and metastatic process of several human cancers (Farazi et al., 2013; Peng and Croce, 2016). Therefore, determining differentially expressed miRNAs between tumor and non-tumor samples has become one of the most studied areas in oncology to discover novel biomarkers for the diagnosis, prognosis, and prediction of therapeutic responses (Nikitina et al., 2012). However, because of the large number of targets that a single miRNA can regulate, large-scale transcriptomic and more comprehensive approaches are required.
The tumor microenvironment (TME), a complex system that contains cancer, immune and stroma cells, chemokines, and cytokines, has been shown to be an important player in the development of various tumors (Wu et al., 2021). The interactions of these components play an essential role in the initiation and progression of various cancers (Chen et al., 2021a). The tumor mass is infiltrated with immunosuppressive cells that vary with the host immune status and have latent prognostic value in various cancers. For instance, cancer-associated fibroblasts (CAFs), regulatory T cells (Treg), tumor-associated macrophages (TAMs), and myeloid-derived suppressor cells (MSDCs) are immunosuppressive cells that play a crucial role in suppressing the host’s anti-cancer immunological responses (Lawal et al., 2022). The aforementioned immunosuppressive cells can inhibit cytotoxic lymphocytes’ function and abundance, leading to T-cell exclusion and invasive phenotype of the cancer cells (Chen et al., 2021a). In another context, tumors are infiltrated with TAMs, and the TAMs’ immune-suppressive secretome promotes T-cell anergy and dysfunction, resulting in suboptimal T-cell differentiation, maturation, and loss of effector function (Lawal et al., 2021a). Increasing evidence indicates that genetic alterations in oncogenic genes in part can determine the infiltration of immune cells. Thus, identifying and targeting these altered oncogenes could lead to a more precise model of immunotherapy and better clinical outcomes for the patients.
With the recent advancement in sequencing technologies, many microarrays and sequencing data are deposited and available for exploring theranostic biomarkers for PTC (He et al., 2005; Giordano et al., 2006; Salvat ore et al., 2007; Vasko et al., 2007; Yu et al., 2008; Hinsch et al., 2009; Pita et al., 2009; Tomás et al., 2012; Pita et al., 2014; Reeb and Reigh-Yi, 2015; Rusinek et al., 2015; Tarabichi et al., 2015; von Roemeling et al., 2015; Handkiewicz-Junak et al., 2016; Lassalle et al., 2016; Minna et al., 2016; Romeo et al., 2018; Lee et al., 2019; Barro s-Filho et al., 2020; Colombo et al., 2020; Minna et al., 2020). However, the low sample sizes in individual studies create a considerable inter-study variability of hallmark genes with limited statistical accuracy (Chen et al., 2021b). Therefore, integrating differentially expressed genes (DEGs) from large-scale cancer transcriptomic datasets to identify intersected DEGs would provide more reliable biomarkers with unified pathogenic and prognostic relevance in PTC. This study integrated 27 transcriptomic datasets from PTC and normal samples. We identified graded levels of biomarkers associated with the immunological pathogenesis of PTC suggestive of T-cell exclusion, EMT, and cell-matrix adhesion mechanisms. We identified a synthetic compound (clopidogrel) and a peptide (ocriplasmin) as the candidate ligand inhibitors of TTEPs. Furthermore, we used molecular docking simulation of receptor-ligand interactions to explore the therapeutic potential of antrocinnamomin, antcin, and antrocin, the bioactive compounds from one of the most reputable Taiwan indigenous medicinal plants (Antrodia camphorata) (Geethangili and Tzeng, 2011). Our results served as a foundation for subsequent experimental validation and precision medicine approaches in managing PTC.
Materials and methods
Large-scale acquisition of genes and microRNA sequencing data of thyroid cancers
We queried the NCBI Gene Expression Omnibus (GEO) microarray datasets (http://www.ncbi.nlm.nih.gov/geo/) for transcriptomic data of RNA and microRNA (miRNA) profiles from PTC in comparison with normal tissue. We identified a total of 27 datasets (He et al., 2005; Giordano et al., 2006; Salvat ore et al., 2007; Vasko et al., 2007; Yu et al., 2008; Hinsch et al., 2009; Pita et al., 2009; Tomás et al., 2012; Pita et al., 2014; Reeb and Reigh-Yi, 2015; Rusinek et al., 2015; Tarabichi et al., 2015; von Roemeling et al., 2015; Handkiewicz-Junak et al., 2016; Lassalle et al., 2016; Minna et al., 2016; Romeo et al., 2018; Lee et al., 2019; Barro s-Filho et al., 2020) (Colombo et al., 2020; Minna et al., 2020) comprising 22 RNA and five miRNA sequencing datasets (Table 1). The dataset containing primary or metastatic cancer tissues (tumor samples) and normal human samples (normal counterparts) was included. Controls were normal healthy cohorts with no cancer. No ethics committee approval or patient consent was required for the present study. The datasets were analyzed for differentially expressed genes (DEGs) and differentially expressed microRNAs (DEmiRNAs) between the cancer and normal tissues using the LIMMA package of R based on the selection cutoff points of logFC: >1.5 and p-value adjusted Benjamini–Hochberg correction and false discovery rate (FDR) < 0.05. The DEGs that met these criteria were sorted to identify the intersected DEGs from all datasets using the Excel lookup and data sorting. The online tool Multiple List Comparator (https://www.molbiotools.com/listcompare.html) was used to visualize the intersected DEGs and generate a Venn diagram for the visualization of the overlapping DEmiRNAs. Furthermore, the DEGs clustering from each dataset were generated using the ImageGP visualization tools (http://www.ehbio.com/ImageGP/). In addition, we also explored The Cancer Genome Atlas (TCGA) dataset of THCA, which comprises 507 cohorts with papillary thyroid carcinoma (PTC).
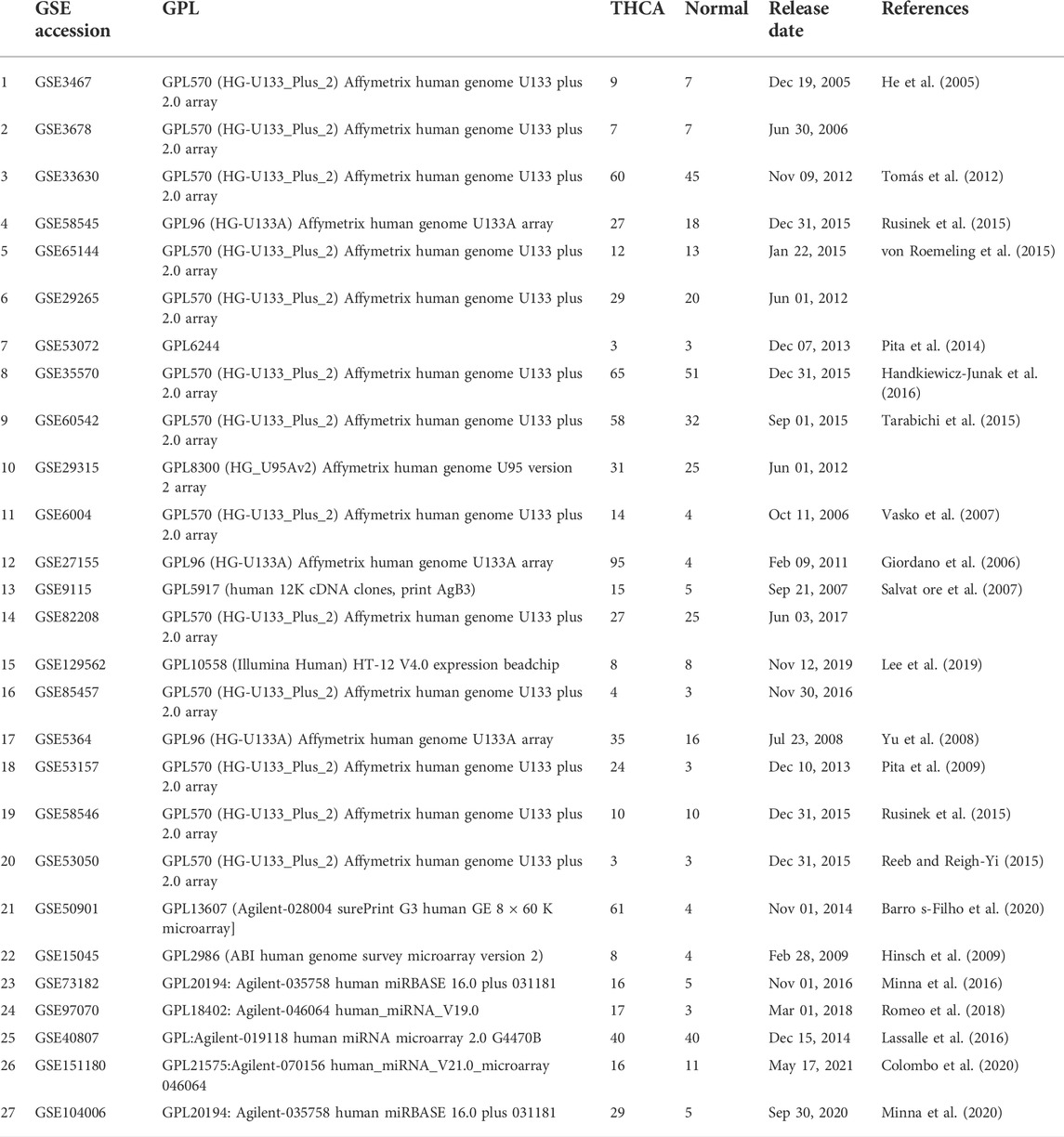
TABLE 1. Characteristics of the datasets used for the large-scale acquisition of differentially expressed genes (DEGs) andmicroRNAs (miRs) in thyroid cancers.
Differential expressions of thyroid tumor-enriched proteins in The Cancer Genome Atlas-thyroid carcinoma cohorts
We analyzed differential expression levels of the TTEPs between tumor and adjacent normal tissues of The Cancer Genome Atlas (TCGA) cohorts of the PTC dataset using the Tumor Immune Estimation Resource (TIMER, version 2.0) (Li et al., 2017). Furthermore, the TTEP sets were analyzed for gene set variation scores between TCGA thyroid tumor and adjacent normal tissue, and also between four types of thyroid tumor stages, namely, the well-differentiated (low grade, stage I), moderately differentiated (intermediate grade, stage II), poorly differentiated (high grade, stage III), and undifferentiated (high grade, stage IV). Differential expression was considered statistically significant at p < 0.05, <0.01, and <0.001.
Differential methylation of thyroid tumor-enriched proteins in The Cancer Genome Atlas-thyroid carcinoma cohorts
We explored the methylation module of the GSCALite server (Liu et al., 2018) to analyze the TTEPs’ methylation differences between the thyroid tumor and paired normal tissues in TCGA database. The differential methylation between tumor and normal samples was compared using Student’s t-test with p-value adjusted by FDR ≤ 0.05. In addition, we analyzed the effect of methylation on the expression levels of TTEPs by assessing the mRNA expression correlation with methylation levels using Pearson’s correlation coefficient and the t-distribution with an FDR-adjusted p-value.
Analysis of thyroid tumor-enriched proteins’ genetic alterations, and prognostic and clinical attributes in thyroid carcinoma
We used the cBioPortal tool (http://www.cbioportal.org/) (Cerami et al., 2012) to analyze the genomic alterations, survival analysis, and clinical attributes, and perform group comparisons of TTEPs in 508 patients (516 samples) of TCGA-PTC cohorts. Based on the genetic alteration profile of the cohorts, we split the cohorts into two groups, the TTEP altered and non-altered cohorts, and used the Kaplan–Meier survival plot to assess the overall survival (OS) and disease-free survival (DFS). We also compared these groups for several clinical attributes, including metastasis, response to surgery, and new neoplasm event after initial therapy using the chi-squared statistical test at p-value < 0.05. The analyzed results were considered significant when p < 0.05.
Analysis of thyroid tumor-enriched proteins’ regulation of the abundance of immune and immuno-suppressive cell infiltrations in thyroid carcinoma
The regulation of the abundance of immune cell infiltration of PTC by the TTEPs was evaluated by analyzing the correlation between the mRNA expression levels of the TTEPs and infiltration of immunosuppressive cells that are known to promote T-cell exclusion; vis myeloid-derived suppressor cell (MDSCs), cancer-associated fibroblast (CAF), tumor-associated macrophages (M2-TAM), and regulatory T cells (Treg) using the Tumor IMmune Estimation Resource (TIMER2.0) (http://timer.cistrome.org/) server (Li et al., 2020). Furthermore, the GSCALite server (Liu et al., 2018) was used to analyze the correlation between the expression levels of the TTEPs and the tumor infiltrations of cytotoxic lymphocytes (B cells, CD4 naïve, CD4 T, CD8 naïve, CD8 T, MAIT cells, NK cells, and γδ T cells), T helper cells (Th1, Th2, Th17, and Tfh), neutrophils, dendritic cells, macrophages, and monocytes. The correlation analysis was conducted using the purity-corrected partial Spearman’s Rho value and statistical significance (p < 0.05) while the correlation data were visualized using the heatmap constructed with GraphPad Prism software (version 8.0.0 for Windows).
Interaction and functional enrichment network analysis of the thyroid tumor-enriched genes
We used the IntAct molecular interaction database (https://www.ebi.ac.uk/intact/home) to analyze the direct protein–protein interaction of the TTEPs while the protein interaction network analysis (PINA) platform was used to analyze the thyroid cancer-specific protein–protein interaction network (https://omics.bjcancer.org/pina/home.action). The thyroid-specific protein interaction network was constructed based on the cutoff point of the tumor type-specific score of two and spearman correlation of 0.5. In addition, the GENEMANIA (https://genemania.org/), an online gene interaction platform (Mostafavi et al., 2008), was used to analyze the gene–gene interaction network of the TTEPs. The Enrichr, an online gene set enrichment analysis (GSEA) server (Chen et al., 2013; Kuleshov et al., 2016) and the search tool for retrieval of interacting genes/proteins (STRING) server (http://string-db.org/, v10.5) were used to analyze functional enrichment profiles of the TTEPs. The official gene symbols of the DEGs were directly uploaded into the Enrichr server and analyzed for the Kyoto Encyclopedia of Genes and Genomes (KEGG) and Gene Ontology (GO) terms under the default enrichment cutoff value of p < 0.05. The Entrez gene symbols of the DEGs were uploaded to the multiple protein modules of the STRING server and analyzed for known and predicted PPI interactions in Homo sapiens under the high confidence (0.70) search and at a significant level of p < 0.05. The significantly (p < 0.05) enriched terms of KEGG and GO)biological processes and molecular functions from the two servers were integrated and visualized using the ImageGP visualization tools.
Thyroid tumor-enriched protein-mediated drug sensitivity analysis
Gene expression-induced drug sensitivity against different cancer cell lines was evaluated using the GSCALite (http://bioinfo.life.hust.edu.cn/web/GSCALite/) server (Liu et al., 2018). The Spearman correlation was used to correlate the messenger (m) RNA expression levels of the TTEPs and the 50% inhibitory concentration (IC50) of the small molecules against various cells in the Cancer Therapeutics Response Portal (CTRP) and Genomics of Drug Sensitivity (GDSC) databases.
Interactions, functional, and disease enrichment network analysis of thyroid tumor-enriched microRNAs
We used the miR-TV database (http://mirtv.ibms.sinica.edu.tw/index.php) to evaluate the differential expression levels of mature miRNA between TCGA thyroid cancer and normal tissue from TCGA version 18.0. We also explored the miRTarBase (https://mirtarbase.cuhk.edu.cn/), an experimentally validated microRNA-target interaction database to identify the gene targets of the thyroid tumor-enriched microRNAs (TTEMiRs). While the disease and functional enrichment of the TTEMiRs were analyzed using the miRNA Enrichment Analysis and Annotation (miEAA) tool (https://ccb-compute2.cs.uni-saarland.de/mieaa2/), analysis was conducted using FDR (Benjamini–Hochberg), a p-value adjustment of 0.05 and a minimum required hit of two miRNAs. The gene ontology (GO) enrichment of the miRNAs was analyzed using the miRDB (http://mirdb.org/mirdb/index.html), an online database for miRNA target prediction and functional annotations (Chen and Wang, 2019). Enrichment analysis was conducted at FDR p < 0.05.
Molecular docking studies of receptor-ligand interactions
The predocking and docking analysis was conducted via the AutoDock Vina (version 0.8, Scripps Research Institute, La Jolla, CA, United States). The three-dimensional (3D) structure of the proteins FN1 (PDB:3MQL), ETV5 (PDB:5ILV), and NRCAM (PDB:1UEN) was downloaded from the protein data bank (PDB). The mol2 files of the 3D structure of compounds were obtained using the Avogadro molecular builder and visualization tool version 1. XX (Hanwell et al., 2012) and converted to PDB format using the PyMOL Molecular Graphics System, version 1.2r3pre. The PDB files of the crystal structures of the targets were transformed to pdbqt format using AutoDock Vina (version 0.8, Scripps Research Institute, La Jolla, CA, United States) (Trott and Olson, 2010). Ligand and receptor preparations for docking were conducted as described previously (Lawal et al., 2021b; Lawal et al., 2021c; Alorabi et al., 2022; Onikanni et al., 2022). In contrast, molecular docking was performed using AutoDock Vina (version 0.8) (Trott and Olson, 2010) with all parameters set to default values, and all bonds in the ligand rotated freely while considering the receptor to be rigid. A spacing of 1.0 Å and a grid box of 40 Å × 40 Å × 40 Å in the X, Y, and Z dimensions were used (Wu et al., 2022). All docking was performed at energy range = 4 and exhaustiveness of 8. The docked complexes were generated in PDF format using the pyMOL software. The complexes were analyzed for interaction affinities between the ligand and the receptors and were expressed as binding energy values in Kcal/mol. The interaction distance of the conventional hydrogen bonding between the amino acid residues of the receptors and the ligand atoms was also analyzed and expressed in Angstrom (Å). The 2D conformation of the receptor-ligand complex was visualized using the Discovery studio visualizer version 19.1.0.18287 (BIOVIA, San Diego, CA, United States) (Visualizer, 2020).
Results
Large-scale transcriptomic data analysis identified graded levels of biomarker signatures of thyroid cancer pathogenesis
The flowchart of the study is shown in Figure 1. We integrated DEGs from large-scale datasets of thyroid cancer to identify graded levels of biomarker signatures of thyroid cancer pathogenesis (Figure 2A). Based on the number of datasets at which each DEG was identified and the prognostic role of the DEGs, we categorized the DEGs as grade 1 (ETV5, FN1, and NRCAM), grade 2 (ABCC3 and ETV4), grade 3 (ALDH1A3, CKS2, GALE, GDF15, and GGCT), and grade 4 (MAMLD1, MAP4K4, PSD3, PTP4A3, TNFRSF10B, TNIK, TUSC3, and ZMAT3) levels of biomarker signatures of thyroid cancer pathogenesis. Collectively, these DEGs are termed tumor thyroid-enriched genes (TTEPs) and were used for the subsequent analysis of the mechanisms of thyroid carcinogenesis. Furthermore, the integration of differentially expressed microRNAs (DEmiRs) from four other GEO datasets (GSE97070, GSE151180, GSE73128, and GSE104006) of miRNAs in thyroid cancers (Figure 2B) identified only two intersected miRNAs, miR-21-5p and miR-146b-5p, collectively termed thyroid tumor-enriched miRs (TTEmiRs). Altogether, our large-scale transcriptomic data analysis identified graded levels of biomarker signatures of thyroid cancer pathogenesis, and our further analysis (Figures 3–7) suggested that these signatures modulate specified genetic signaling and are thyroid cancer-specific, and hence, could be useful in the clinical diagnosis of thyroid cancer.
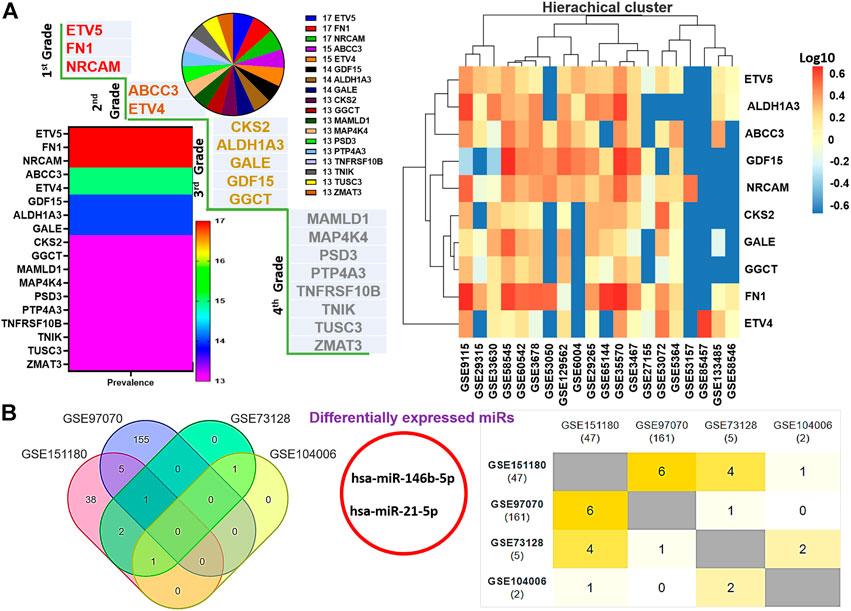
FIGURE 2. Large-scale transcriptomic data analysis identified graded levels of biomarker signatures of thyroid cancer pathogenesis. (A) Multiplot and hierarchical clustering of differentially expressed genes (DEGs) identified from the large-scale transcriptomic datasets. (B) Venn diagram and Jaccard plot of the differentially expressed microRNAs identified from the integrations of DEmiRs in THCA datasets.
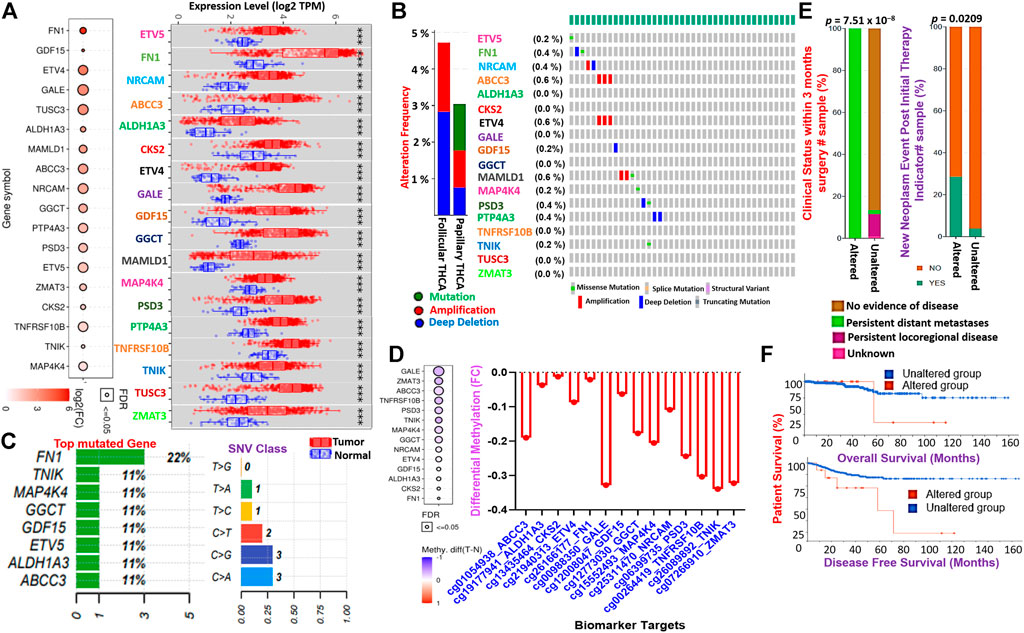
FIGURE 3. TTEPs are associated with poor surgical outcome, distant metastasis, and poor prognosis in THCA cohorts. (A) Bubble and boxplots of the mRNA expression levels of the TTEPs between TCGA thyroid tumor and adjacent normal tissues. (B) Bar and waterfall of the genetic alteration profile and (C) mutation plots of the TTEPs in TCGA pan-cancer of THCA cohorts. (D) Bubble and bar plots of the methylation status of the TTEPs in TCGA-THCA cohorts. (E) Bars plot of the clinical attribute of TCGA-THCA cohorts with genetically altered TTEPs. (F) Kaplan–Meier plots of the overall and disease-free survival differences between TCGA-THCA cohorts with genetically altered and wild-type TTEPs.
Thyroid tumor-enriched proteins are associated with tumor stages, poor surgical outcome, distance metastasis, and worse prognosis
To validate our findings from the large-scale transcriptomic data analysis, we used TCGA cohorts to evaluate the differential expression levels of the TTEPs between the cohorts’ tumor tissue and adjacent normal tissue. Notably, we found that all members of the TTEPs were significantly (p < 0.001) overexpressed in the thyroid tumor tissue compared with the adjacent normal tissues (Figure 3A). In addition, the TTEPs exhibited various types of genetic alterations (mutation, amplification, and deep deletion) in thyroid cancer (Figures 3B,C), with the grade 1 signature having the highest occurrence of genetic mutations (Figure 3C). Consistently, the TTEPs were found to be significantly hypomethylated in THCA tumors compared with the normal tissues (Figure 3D). Interestingly, we found that thyroid cancer patients with genetic alterations of TTEPs exhibited persistent distant metastases within 3 months of surgery (Figure 3E). In addition, this group of thyroid cancer patients demonstrated significantly (p < 0.0209) higher levels of new neoplasm events after initial therapy (Figure 3E) and worse prognosis as measured by the overall survival and disease-free survival (Figure 3F). TTEPs exhibited high enrichment and GSVA (gene set variation analysis) scores in PTC compared with adjacent non-tumor tissues (Figure 4A). In addition, the expression levels of the TTEPs are significantly associated with tumor stages (Figure 4B), high risk, and shorter survival duration of the cohorts (Figures 4C,D). The GSVA of the TTEPs revealed a strict activation of EMT among 10 other pathways (Supplementary Figure S1) and immunosuppressive cells of invasive tumor phenotypes (Supplementary Figure S2) in PTC. Collectively, our results demonstrated that the TTEPs’ expression increased with disease stages (except for stage 2), and is associated with a poor surgical outcome, increased incidence of distance metastases, and poor prognosis in clinical PTC cohorts.
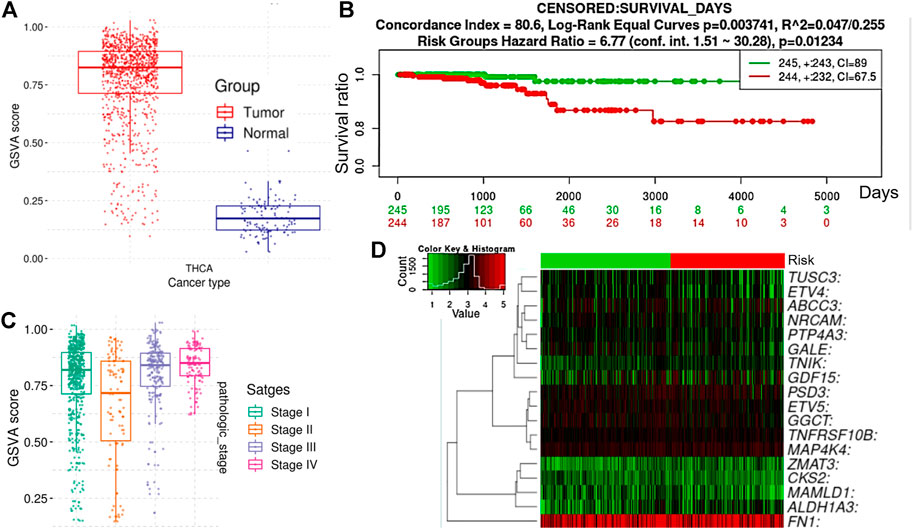
FIGURE 4. Gene enrichment analyses of the TTEPs from TCGA-PTC cohorts. (A) Bar plot of the gene set variation analysis (GSVA) score of TTEPs between TCGA-PTC cohorts and adjacent normal tissue. The GSVA is a GSE method that estimates the variation of pathway activity over a sample population in an unsupervised manner. Compared to the normal tissue, the TTEPs achieved a very high GSVA score, which shows a negative GSVA score in PTC. (B) Bar plots of the differential GSVA score between tumor stages. (C) Kaplan–Meier plot, and (D) risk plot of TCGA-PTC cohorts with high and low-expression profiles of TTEPs. The PTC cohorts with high expression levels of TTEPs exhibited high hazard risk and shorter survival duration when compared with cohorts with low expression levels of the TTEPs.
Thyroid tumor-enriched proteins demonstrated a unified association with the T-cell exclusion mechanism of immune-invasive phenotypes of thyroid carcinoma
Our analysis revealed that all members of TTEPs demonstrated a cooperative association with the infiltration levels of different categories of immune and immunosuppressive cells in THCA (Figures 5A–D). We found that the mRNA expression levels of the TTEP showed no significant (p > 0.05) positive association with the infiltration levels of cytotoxic lymphocyte (Figures 5A,B) cells, CD4 naïve, CD4 T, CD8 naïve, CD8 T, MAIT cells, NK cells, and γδ T cells, and significant negative association (p < 0.05) with the infiltration of T-helper cells (Figure 5B) and neutrophils (Figure 5D). However, the mRNA expression levels of the TTEPs show a significant and positive (p < 0.05) association with the infiltration levels of immunosuppressive cells (CAF, MDSC, TAM, nTreg, iTreg, and Tr1) (Figure 5C), dendritic cells, macrophages, and monocytes (Figure 5D). These immunosuppressive phenotypes are markers of T-cell exclusion. Hence, our results strongly suggested that TTEPs exhibited a unified association with the T-cell exclusion mechanism of immune-invasive phenotypes in PTC.
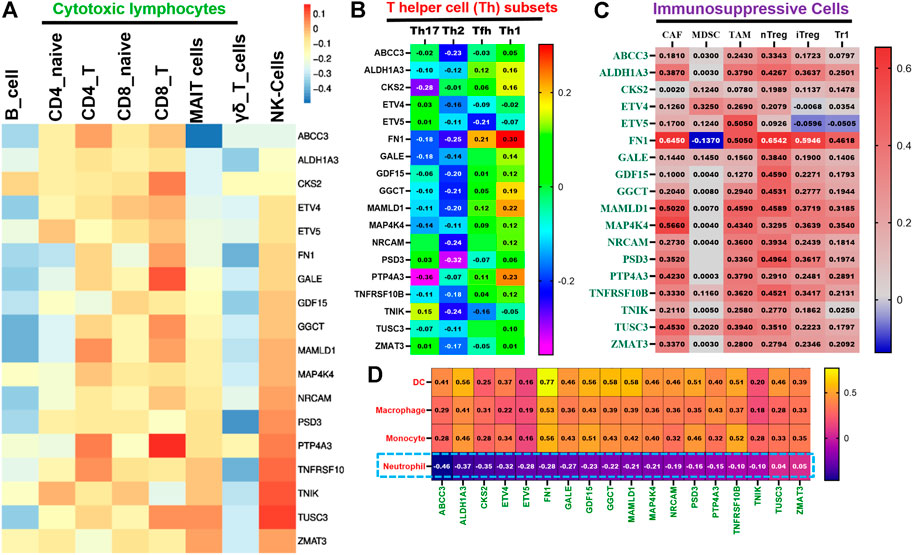
FIGURE 5. TTEPs demonstrated a significant association with the T-cell exclusion mechanism of immune-invasive phenotypes in THCA. Heatmap plots of the mRNA association with tumor in infiltrations of cytotoxic lymphocytes (A), T-helper cells (B), immunosuppressive cells (C), myeloid cells, and neutrophils (D) in TCGA-THCA cohorts.
Thyroid tumor-enriched proteins are significantly associated with cell-matrix adhesion mechanisms and transcriptional dysregulation in thyroid carcinoma
We conducted a series of functional and interaction analyses to gain further insight into the potential pathological mechanisms of the TTEP. Notably, the direct protein–protein interaction analysis revealed a total of 74 interactors and 181 direct interactions with the targets, of which FN1 occupied a total of 126 interactions. In addition, FN1 serves as the major mutated protein of the network, affecting the biological roles of LOX, ACE2, DCN, ITGAV, and FNbB (Figure 6A). Thyroid cancer-specific PPI of the targets identified 121 unique disease-related interactions with the targets (Supplementary Figure S3). Specifically, we found that the interactions of the DEGs with SPTBN1, TUBB2A, ANK2, CEP350, PCLO, CDK12, MRPS16, TUBB2B, and IL20RA, were significantly associated with poor prognosis of the PTC cohorts (Supplementary Figure S3). Furthermore, we identified 38 gene–gene interacting networks significantly involved in membrane/cell ruffling, leading-edge membrane, cell division site, and lyse activity (Supplementary Figure S3). Similarly, our gene set enrichment analysis (GSEA) demonstrated that thyroid tumor-enriched proteins were significantly enriched in KEGG pathways, including the ABC transporters, transcriptional misregulation in cancer, extracellular matrix (ECM) receptor interaction, proteoglycans in cancer, cell adhesion molecules, and focal adhesion and regulation of the actin cytoskeleton. The enriched gene ontologies of the TTEP included cell migration, extracellular matrix organization, integrin-mediated signaling pathway, cell–cell adhesion mediated by integrin substrate adhesion-dependent cell spreading, regulation of fibroblast migration, and galactose metabolism (Figure 6B). Collectively, our results suggested that the thyroid tumor-enriched proteins (TTEP) induced significant pathological interactions associated with tumor progression and worse prognosis via the cell-matrix adhesion mechanism and transcriptional dysregulation.
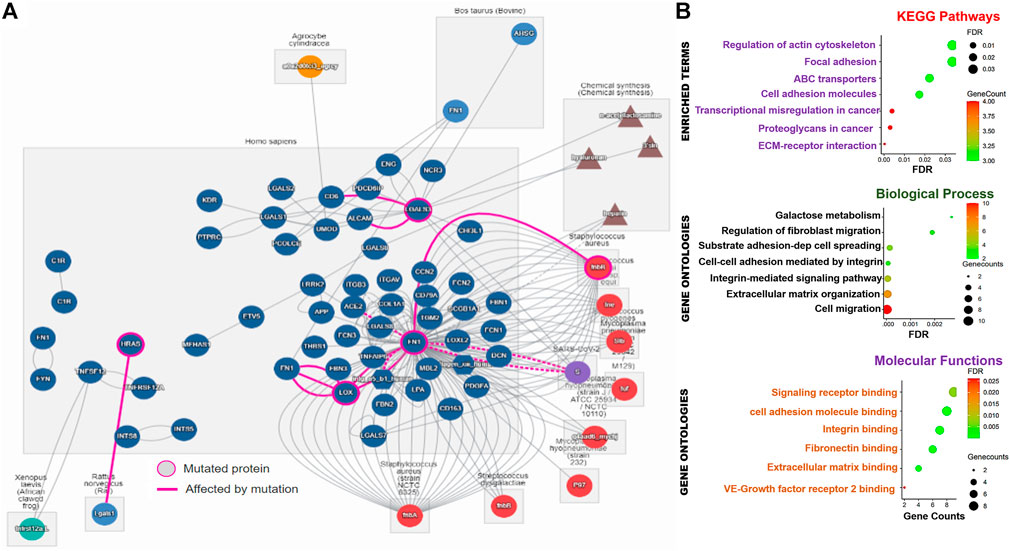
FIGURE 6. Thyroid tumor-enriched proteins (TTEPs) induced significant pathological interactions via cell-matrix adhesion and transcriptional dysregulation. (A) Network plots of direct protein–protein interactions of TTEPs. The direct protein–protein interaction was queried based on the human interaction of the proteins under the influence of mutation or chemical treatment. (B) Enrichment network plots of KEGG pathways, GO molecular functions, and biological processes of the TTEPs.
Thyroid tumor-enriched proteins mediate antitumor drug resistance and unique association with cancer cell response to MEK-inhibitors
Scientific evidence has indicated that the expression of several oncogenic genes is associated with drug resistance in various cancers (Gillet et al., 2011). On this premise, we analyzed the relationship between the levels of TTEP expression and the response of cancer cell lines to several chemotherapies. The data obtained revealed that with the exclusion of PTP4A3 and GGCT, and the levels of TTEP expressions were significantly (p < 0.05) associated with the resistance of cancer cell lines to several classes of small molecules of anti-cancer functions. On the contrary, we observed a distinct association with MEK inhibitors; these gene signatures induced increased sensitivity of the cancer cell lines to numerous inhibitors of MEK, including trametinib, selumetinib, 17-AAG, RDEA119, PD-0325901, (5Z)-7-oxozeaenol, and a mutant-BRAF kinase inhibitor, dabrafenib (Figure 7).
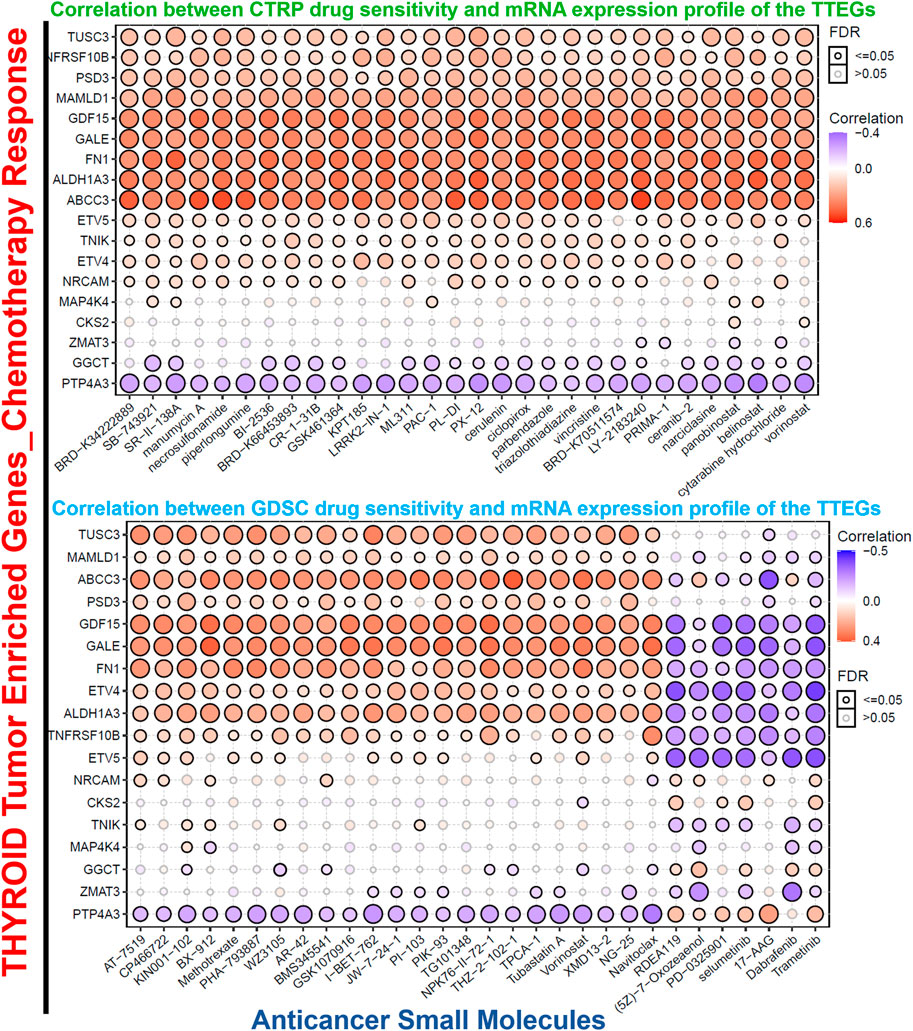
FIGURE 7. Bubble plots of TTEPs’ association with the sensitivity of various GDSC and CTRP anti-cancer drugs.
Identification of miR-146b-5p and miR-21-5p as thyroid carcinoma-specific oncogenic microRNA
We evaluated and compared the hierarchal clustering and differential expression profiles of miR-146b-5p and miR-21-5p between TCGA cohorts of THCA and healthy tissues. We found that, of the 33 TCGA cancer cohorts, miR-146b-5p and miR-21-5p formed three clusters (Figure 8A). Notably, both miRs were found significantly (p = 2.2 × 10–16) overexpressed in THCA compared to the adjacent normal tissues (Figure 8B). However, our dataset analysis of differential miRNA expression between tumor histology revealed no significant differential expression of these miRs among the different THCA histology. The subsequent enrichment analysis revealed that miR-146b-5p and miR-21-5p were specifically associated with the regulation of immune response, EMT, cell–cell adhesion, cell growth, stem cell population maintenance, and the progression of several thyroid-associated diseases (Figure 8C). More importantly, through exploration of experimental evidence from Western blot, reporter assay, qPCR, NGS, and microarray, RASGRP1, CDC25A, BCL2, TIMP3, PTEN, RECK, TGFBR2, TRAF6, IRAK1, PDGFRA, and IL6 were identified as the major target genes of miR-146b-5p and miR-21-5p in THCA (Figure 8D). Consistently, we found that the aforementioned genes were significantly downregulated in THCA compared with adjacent normal tissues. Our analyses strongly suggested that miR-146b-5p and miR-21-5p (which we termed TTEmiRs) are THCA-specific oncogenic miRs through immune modulation and EMT induction to promote cellular adhesion/growth, and stem cell population maintenance in THCA.
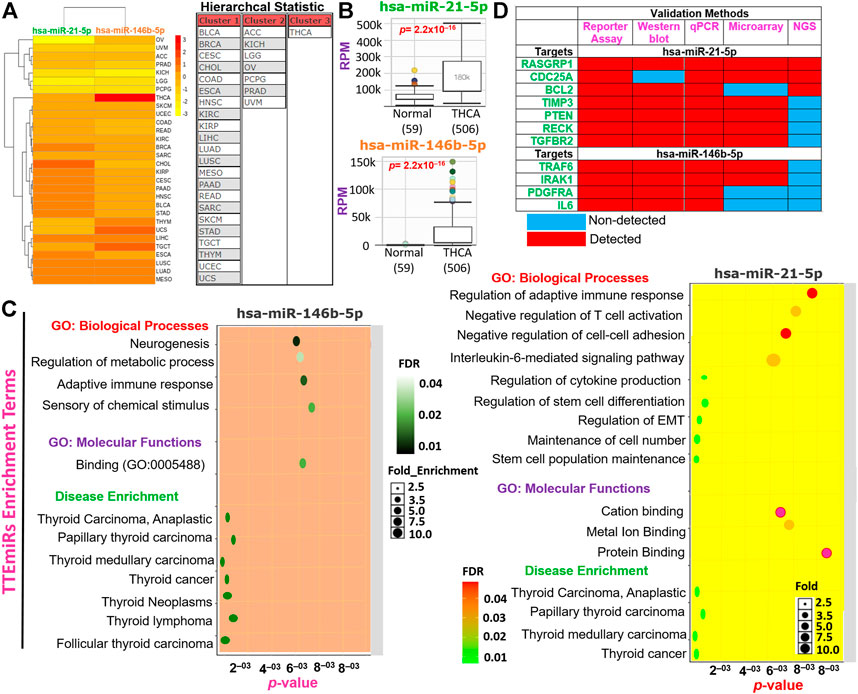
FIGURE 8. Thyroid tumor-enriched microRNA (TTEmiRs) are THCA-specific oncogenic microRNA (miRs) that modulate the immune response, EMT, cell–cell adhesion to promote cell growth, and stem cell population. (A) Heatmap plot and hierarchal clustering of the TTEmiRs in TCGA cancer cohorts. (B) Bar plots showing the differential expression levels of the TTEmiRs between TCGA-THCA tumor and adjacent normal tissue and (C) enrichment plots of the TTEmiRs. (D) Plots of the TTEmiRs target genes based on experimentally validated protocols (D).
Potential drug target exploration and molecular docking of target-ligand simulation
We queried FN1, ETV5, and NRCAM for potential drug targets based on the structural activity relationship (SAR) and identified a synthetic compound (clopidogrel) and a peptide (ocriplasmin) with high connectivity scores and probable ligand inhibitors of the three targets (Figure 9A). Furthermore, we explored the therapeutic potential of bioactive compounds from one of the most reputable Taiwan indigenous medicinal plant (Antrodia camphorata). Of about 80 characterized compounds from this plant (Geethangili and Tzeng, 2011), we identified antrocinnamomin, antcin, and antrocin as the three most potent compounds primarily implicated in this plant’s bioactivity (Figure 9B). In addition, these compounds have been previously reported for anti-cancer, anti-inflammatory, and immunomodulatory activities in our laboratory and elsewhere (Chen et al., 2007; Male et al., 2008; Yeh et al., 2009; Rao et al., 2011; Yeh et al., 2013; Chiu et al., 2016; Chen et al., 2019a; Chen et al., 2019b). Our molecular docking study revealed that the synthetic compound, clopidogrel, docked well by hydrogen bonding, alkyl interaction, and several van der Waals forces to the binding cavity of FN1, ETV5, and NRCAM with binding affinities of –6.70, –5.90, and –5.50 Kcal/mol, respectively (Figures 9C–E; Table 2). On the other hand, the protein-peptide docking revealed a robust binding of ocriplasmin to ETV5, FN1, and NRCAM with affinities of ‒200.316 Pbe, ‒197.054 Pbe, and ‒164.942 Pbe, respectively (Figure 9F). Interestingly, the three phytoactive compounds (antrocinnamomin, antcin, and antrocin) demonstrated higher binding affinities (–5.50 ∼ –7.50 Kcal/mol) to FN1, ETV5, and NRCAM (Figure 10) than the affinities for ligands demonstrated by the synthetic compound and peptide (Table 2). The compounds are bonded to the active site of the ligands by hydrogen, alkyl, and pi interactions, and several van der Waals forces formed around the ligand’s backbones with several amino acid residues of the receptor’s binding sites (Figures 10A–C). Among the phytochemicals, we found that antcin exhibited higher affinities to the binding sites of all the receptors, followed by antrocinnamomin, while antrocin demonstrated the least. In addition, FN1 demonstrated high ligandability potential for the compounds, whereas NRCAM demonstrated the least (Table 2). Although antrocinnamomin docked well to NRCAM, our analysis revealed that the interactions are not favorable for ligandability (Figure 10B).
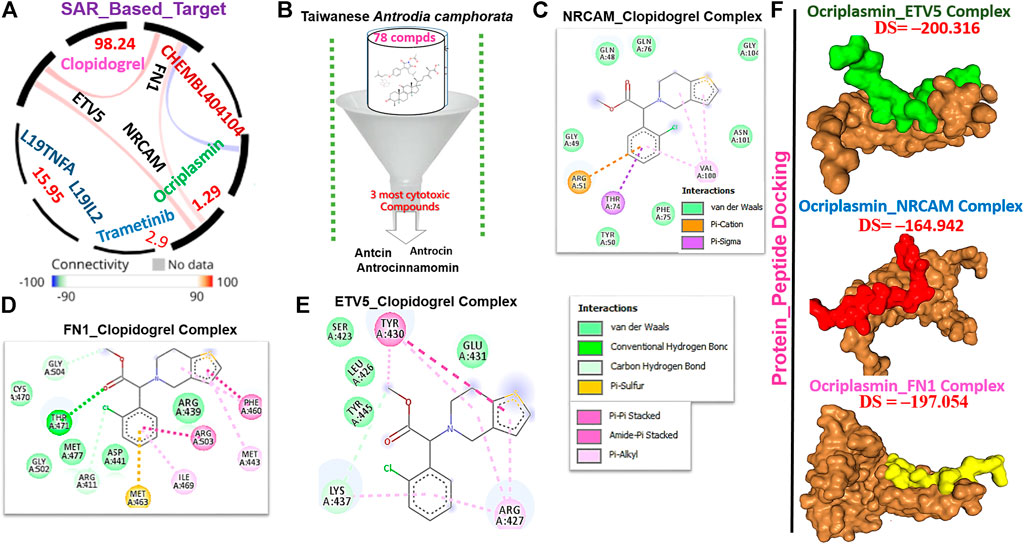
FIGURE 9. Drug target identification and molecular docking study of clopidogrel (synthetic compound) or ocriplasmin with the thyroid tumor-enriched protein (TTEP). (A) Target connectivity mapping of FN1/ETV5/NRCAM. (B) Sorting and identification of potent phytocompounds from Antrodia camphorata. Molecular docking profile of clopidogrel with (C) FN1, (D) NRCAM, and (E) ETV5. (F) Protein–peptide docking of ocriplasmin with FN1/ETV5/NRCAM.
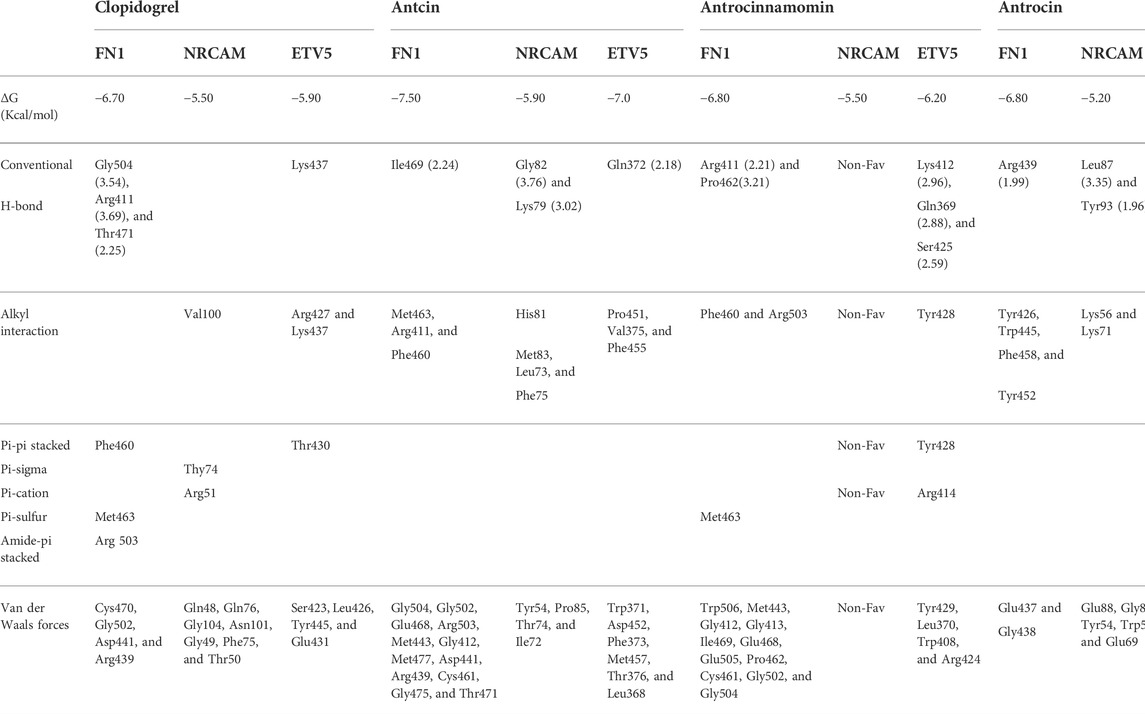
TABLE 2. Docking profile of TTEPs with antrocinnamomin, antcin, and antrocin isolated from Taiwan Antrodia camphorata.
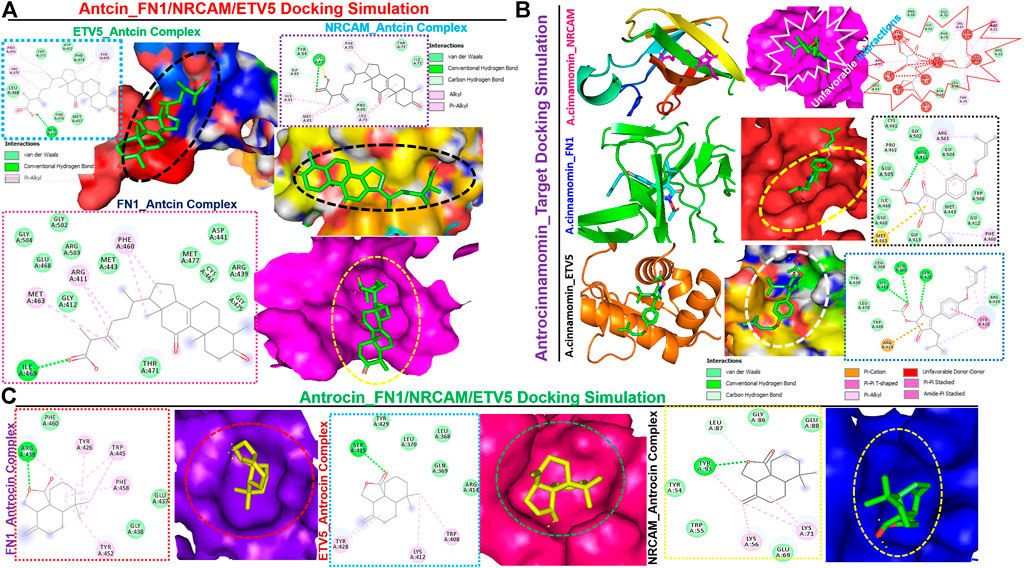
FIGURE 10. Molecular docking profile of FN1/ETV5/NRCAM with the phytocompound isolated from Taiwan Antrodia camphorata. Docking profile of (A) antcin, (B) antrocinnamomin, and (C) antrocin with FN1/ETV5/NRCAM.
Thyroid tumor-downregulated genes are mainly involved in the maintenance of ion and membrane structures, and the inhibition of the cell cycle at the G1/S phase transition
We also integrated differentially downregulated DEGs from the datasets and identified six downregulated DEGs, namely, MPPED2, TNFRSF11B, FHL1, CRABP1, MATN2, and TFF3, collectively known as thyroid tumor-downregulated genes (TTDGs) (Supplementary Figure S4). The expression fold change (log2) of the TTDG is shown in Table 3. Enrichment analysis revealed that the downregulated DEGs (TTDGs) are mainly involved in the maintenance of the epithelial structure, membrane depolarization, ion membrane structure-activity, and negative regulation of cell cycle G1/S phase transition (Table 4). Altogether, our analysis revealed that the TTDGs are involved in the maintenance of ion and membrane structures and inhibition of the cell cycle at the G1/S phase transition.
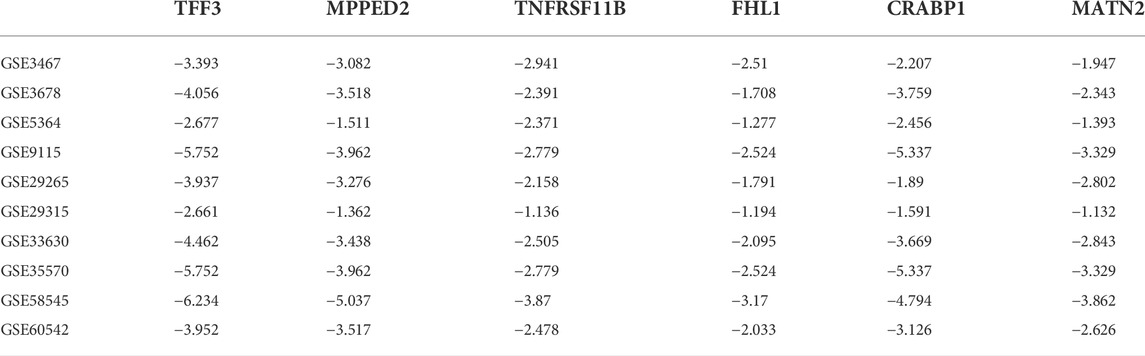
TABLE 3. Expression fold change (log2) of differentially downregulated genes (DDG) in papillary thyroid carcinoma.
Discussion
The present study represents the largest ever transcriptomic analysis of THCA datasets. Our comprehensive, integrated analysis illuminates the potential molecular characteristics and enhances our understanding of the pathological processes of thyroid carcinoma. We integrated all the available transcriptomic datasets of the gene expression profile from THCA and non-THCA tumors and identified graded levels: grade 1 (ETV5, FN1, and NRCAM), grade 2 (ABCC3 and ETV4), grade 3 (ALDH1A3, CKS2, GALE, GDF15, and GGCT), and grade 4 (MAMLD1, MAP4K4, PSD3, PTP4A3, TNFRSF10B, TNIK, TUSC3, and ZMAT3) levels of biomarker signatures for THCA (TTEPs), and two microRNAs collectively known as thyroid tumor-enriched miRs (TTEmiRs). We found that TTEPs are associated with tumor stages, poor surgical outcome, distance metastasis, and worse prognosis in the cohorts. In addition, TTEPs demonstrated a cooperative association with the T-cell exclusion mechanism of immune-invasive phenotypes of THCA and induced significant pathological interactions via EMT, cell-matrix adhesion mechanism, and transcriptional dysregulation in PTC.
Our differential gene expression analysis based on TCGA cohorts of THCA revealed that all members of the TTEPs are highly significantly (p < 0.001) overexpressed in the thyroid tumor tissue compared to the adjacent normal tissue. The highly significant levels of overexpression of these genes strongly validated our large-scale transcriptomic data analysis and suggested that these signatures may be a useful genetic biomarker for diagnosing PTC. DNA methylation is a crucial epigenetic modulation in mammalian genomes and plays a crucial role in regulating gene expression and may serve as a noninvasive biomarker for cancer diagnosis and prognosis (Robertson, 2005; Lawal et al., 2021a). Compared with the normal tissue, the significant hypomethylation of the TTEPs in the PTC tumor tissue is in sharp contrast with the expression levels of these genes in the corresponding tumor sample. These negative associations between the mRNA level and methylation status of the TTEPs suggest the epigenetic regulation of the TTEPs, which could be attributed to the recruitment of the methyl moieties containing gene repressor proteins or by inhibiting the binding of transcription factors to DNA (Robertson, 2005; Shinawi et al., 2013).
Cancer cell interactions with the TME play an important cancer etiologic role in various tumors via distinct mechanisms involving a phenotype of dysfunctional T-cells and a phenotype of T-cell exclusion (Chen et al., 2021a; Wu et al., 2021). Our analysis revealed that all members of TTEPs demonstrated a cooperative relationship with the infiltrating levels of different categories of the cells of immunosuppressive and immune response in THCA. Infiltrations of these cells within the tumor vary with the immune status of the host and have pertinent prognostic roles. CAF, Tregs, TAM, and MSDC are immunosuppressive cells that inhibit the activities of CTL causing the exclusion of T cells and the induction of invasive phenotypes (Chen et al., 2021a). However, some tumors have abundant infiltration of immune cells; these cells are dysfunctional and unable to activate the immune response, a condition known as T-cell allergy (Lawal et al., 2021a; Lawal et al., 2021d). We found that the mRNA expression levels of the TTEP show no significant (p > 0.05) association with the infiltration levels of cytotoxic lymphocytes (B cells, CD4 naïve, CD4 T, CD8 naïve, CD8 T, MAIT cells, NK cells, and γδ T cells), and negative association (p < 0.05) with infiltration of T-helper cells and neutrophils. However, the mRNA expression levels of the TTEP show a significant and positive (p < 0.05) association with the infiltration levels of immunosuppressive cells (CAF, MDSC, TAM, nTreg, iTreg, and Tr1), dendritic cells, macrophages, and monocytes. These immunosuppressive phenotypes are markers of T-cell exclusion. Hence, our results strongly suggested that TTEPs exhibited a cooperative association with the T-cell exclusion mechanism of immune-invasive phenotypes in PTC.
During innate and adaptive immune responses, the TME secretes growth factors that induce EMT, one of the hallmarks of cancer (Kalluri and Weinberg, 2009; Mittal, 2018). EMT mediated the interruption of cellular polarity and cell–cell adhesion, cell-matrix adhesion, and cytoskeleton remodeling (Roche, 2018). In addition, EMT induced marked resistance to therapy and apoptotic stimuli (Mittal, 2018). However, more knowledge about the deregulatory mechanism of EMT in THCA, its control, and EMT-THCA-specific molecular markers is necessary. Indeed, all our enrichment and functional analyses revealed that the TTEPs are strictly involved in transcriptional regulation of EMT-associated processes, including the ABC transporters, interactions of ECM-receptors, proteoglycans in malignance, focal adhesion, actin cytoskeleton regulation, and cell adhesion molecules.
Consistently, our analyses revealed that patients with altered TTEP profiles showed little response to surgical intervention, increased incidence of distant metastases, tumor recurrence, and significantly lower survival. These findings all suggest that the TTEPs represent a specific biomarker set for assessing thyroid tumor staging, metastasis, prognosis, and therapeutic response.
An important challenge in cancer treatment is the development of resistance to chemotherapeutic drugs (Choi et al., 2020). While some cancer patients may achieve an initial remission with present therapeutic regimens, tumor recurrence is observed in most patients even with aggressive treatment (Steinbach et al., 2002). Our analysis revealed that with the exclusion of PTP4A3 and GGCT, the expression levels of the TTEPS were significantly (p < 0.05) associated with the resistance against several classes of small-molecule drugs. On the contrary, we observed that these gene signatures induced increased sensitivity of cancer cell lines to several MEK inhibitors, including trametinib, selumetinib, 17-AAG, RDEA119, PD-0325901, (5Z)-7-oxozeaenol, and, a mutant-BRAF kinase inhibitor, dabrafenib (Figure 7), suggesting that this class of anti-cancer drugs may still be useful in the treatment of thyroid cancer.
A previous study reported that hypomethylation induced the overexpression of miR-146b and miR-21 in THCA with consequent conferment of proliferative and invasive tumor phenotypes (Ortiz et al., 2018), in agreement with our findings. He et al. (2005) also identified the upregulation of miRNA 146b in 20 patients with PTC (He et al., 2005). Similarly, Geraldo et al. (2012) reported that miR-146b-5p is highly expressed in PTC and is considered an important diagnostic biomarker for this cancer type. It has been reported that miR-146b-5p plays an oncogenic role by putatively binding to the 3′ UTR of the targets to regulate the TGF-β signaling pathway in favor of thyroid cancer tumorigenesis (Geraldo et al., 2012). Similarly, our validation of the miRs using TCGA cohorts’ dataset revealed that miR-21-5p and miR-146b-5p are more than 100-fold overexpressed in THCA compared to the non-THCA cohorts. Therefore, the expression levels of these miRs can be used as reliable non-coding biomarkers of thyroid cancer. However, our dataset analysis revealed no significant differential expression of these miRs among the different THCA histology, suggesting that miR-21-5p and miR-146b-5p are generally thyroid tumor-specific rather than histologically-specific. Indeed, several experimental studies have identified these two miRs as important regulators of different thyroid tumor histology including ATC, PTC, and FTC (Zhang et al., 2013; Mancikova et al., 2015; Hu et al., 2016). However, miR-146b-5p expression has been identified to increase significantly throughout stages I to III of PTC (Zhang et al., 2013), suggesting its involvement in thyroid tumor progression. Our functional analyses of miR-146b-5p and miR-21-5p revealed their involvement in various cellular functions, including immune response, EMT, cell–cell adhesion, cell growth, and stemness. These findings are supported by various pieces of experimental evidence implicating these miRs in migration, proliferation, invasion, colony-forming ability, cell cycle, and chemotherapy resistance (Gonda et al., 2010; Chou et al., 2013; Deng et al., 2015; Ortiz et al., 2018). Furthermore, hsa-miR-146b-5p expression has been regarded as an independent risk factor for poor prognosis in PTC together with lymph node metastasis and advanced tumor stage (Chou et al., 2013).
Interestingly, we identified a synthetic compound (clopidogrel) and a peptide (ocriplasmin) as the most probable ligand inhibitors of the TTEPs. Natural products serve as an important reservoir of bioactive metabolites for the treatment of several diseases (Lawal et al., 2015; Lawal et al., 2016; Onikanni et al., 2021). Our molecular docking study revealed that antrocinnamomin, antcin, and antrocin, the bioactive compounds from one of the most reputable Taiwan indigenous medicinal plant (Antrodia camphorata), demonstrated higher binding affinities (–5.50 ∼ –7.50 Kcal/mol) to FN1, ETV5, and NRCAM (Figure 10) than the affinities for ligands demonstrated by the synthetic compound and peptide (Table 2). The phytocompounds are bound to the active site of the ligands by hydrogen, alkyl, and pi interactions and several van der Waals forces formed around the backbones of the ligands with numerous AAs residues of the receptor’s binding sites (Figures 10A–C). Among the phytocompounds, we found that antcin exhibited higher affinities to the binding sites of all the receptors, followed by antrocinnamomin, while antrocin demonstrated the least. In addition, FN1 demonstrated high ligandability potential for the compounds whereas NRCAM demonstrated the least (Table 2). Although antrocinnamomin docked well to NRCAM, our analysis revealed that the interactions are not favorable for ligandability (Figure 10A).
Collectively, by the integration of various GEO datasets, we not only identified various significant coding and non-coding theranostic biomarkers that underlay PTC progression but also found graded levels of the hub genes, which may be considered novel and potential biomarkers of thyroid cancer. Cohorts from TCGA database were finally explored to affirm the precision of our findings and we found that the identified biomarkers were associated with tumor stages, poor surgical outcome, distance metastasis, immune-invasive phenotypes, and worse prognosis of the cohorts. Therefore, our results provide novel and reliable biomarkers for elucidating the pathogenesis of thyroid cancer, which will be helpful in future clinical applications in prognosis, diagnosis, target therapy, and monitoring treatment outcome in thyroid cancer.
Furthermore, we identified MPPED2/TNFRSF11B/FHL1/CRABP1/MATN2/TFF3 as important downregulated DEGs in PTC. MPPED2 has been reported to act as a tumor suppressor that inhibits PTC via activation of PTEN and suppression of the AKT pathway (Li et al., 2021). Qu et al. (2016) also reported the downregulation of TNFRSF11B in PTC. Our study, therefore, serves as a validation for the previous studies where MPPED2, TNFRSF11B, FHL1, CRABP1, or MATN2/TFF3 were individually or jointly found to be downregulated in thyroid cancer (Barros-Filho et al., 2015; Gomez-Rueda et al., 2016; Qu et al., 2016; Sepe et al., 2018; Li et al., 2021). Interestingly, we found that these gene signatures are mainly involved in the maintenance of ion and membrane structures and the inhibition of the cell cycle at the G1/S phase transition. This stresses the pathological importance of ion and membrane structure homeostasis and cell cycle at the G1/S phase transition in the development of papillary thyroid carcinoma.
However, the limitation of our study must be acknowledged. The absence of patient cohorts to corroborate our findings marked a critical area that required further studies. Moreover, functional studies, including potential target validation and the therapeutic potential of antcin in cells and tumor-bearing mice are necessary for the clinical applicability of our findings.
Conclusion
Collectively, our analysis of large-scale transcriptomic datasets of THCA identified graded levels of gene and microRNA biomarkers, implicating the underlying mechanisms involving T-cell exclusion, EMT, and cell-matrix remodeling. Further investigation is warranted for future clinical applications.
Data availability statement
The original contributions presented in the study are included in the article/Supplementary Material; further inquiries can be directed to the corresponding author.
Author contributions
BL and M-LS, performed the study and wrote the manuscript. S-YC, JOO, AOB, C-LH, SC, GE-SB, SA and SSA, participated in data collection and analyses; J-CL and ATHW designed and oversaw the study.
Funding
ATHW is funded by the Ministry of Science and Technology, Taiwan (111-2314-B-038-142 and 111-2314-B-038-098). J-CL is supported by the TSGH research grant (TSGH-D-111060); C-LH is supported by a TSGH grant (TSGH-C02-111024).
Acknowledgments
The authors would like to acknowledge the Taif University Researchers Supporting Project (number TURSP-2020/202), Taif University, Taif, Saudi Arabia.
Conflict of interest
The authors declare that the research was conducted in the absence of any commercial or financial relationships that could be construed as a potential conflict of interest.
Publisher’s note
All claims expressed in this article are solely those of the authors and do not necessarily represent those of their affiliated organizations, or those of the publisher, the editors, and the reviewers. Any product that may be evaluated in this article, or claim that may be made by its manufacturer, is not guaranteed or endorsed by the publisher.
Supplementary material
The Supplementary Material for this article can be found online at: https://www.frontiersin.org/articles/10.3389/fcell.2022.923503/full#supplementary-material
References
Alorabi, M., Mohammed, D. S., Mostafa-Hedeab, G., El-Sherbeni, S. A., Negm, W. A., Mohammed, A. I. A., et al. (2022). Combination treatment of omega-3 fatty acids and vitamin C exhibited promising therapeutic effect against oxidative impairment of the liver in methotrexate-intoxicated mice. Biomed. Res. Int. 2022, 4122166. doi:10.1155/2022/4122166
Barrea, L., Gallo, M., Ruggeri, R. M., Giacinto, P. D., Sesti, F., Prinzi, N., et al. (2021). Nutritional status and follicular-derived thyroid cancer: An update. Crit. Rev. Food Sci. Nutr. 61, 25–59. doi:10.1080/10408398.2020.1714542
Barros-Filho, M. C., de Mello, J. B. H., Marchi, F. A., Pinto, C. A. L., da Silva, I. C., Damasceno, P. K. F., et al. (2020). GADD45B transcript is a prognostic marker in papillary thyroid carcinoma patients treated with total thyroidectomy and radioiodine therapy. Front. Endocrinol. 11, 269. doi:10.3389/fendo.2020.00269
Barros-Filho, M. C., Marchi, F. A., Pinto, C. A., Rogatto, S. R., and Kowalski, L. P. (2015). High diagnostic accuracy based on CLDN10, HMGA2, and LAMB3 transcripts in papillary thyroid carcinoma. J. Clin. Endocrinol. Metab. 100, E890–E899. doi:10.1210/jc.2014-4053
Brito, J. P., Morris, J. C., and Montori, V. M. (2013). Thyroid cancer: Zealous imaging has increased detection and treatment of low risk tumours. Bmj 347, f4706. doi:10.1136/bmj.f4706
Cerami, E., Gao, J., Dogrusoz, U., Gross, B. E., Sumer, S. O., Aksoy, B. A., et al. (2012). The cBio cancer genomics portal: An open platform for exploring multidimensional cancer genomics data. Cancer Discov. 2, 401–404. doi:10.1158/2159-8290.cd-12-0095
Chen, E. Y., Tan, C. M., Kou, Y., Duan, Q., Wang, Z., Meirelles, G. V., et al. (2013). Enrichr: Interactive and collaborative HTML5 gene list enrichment analysis tool. BMC Bioinforma. 14, 128. doi:10.1186/1471-2105-14-128
Chen, J.-H., Wu, A. T. H., Lawal, B., Tzeng, D. T. W., Lee, J.-C., Ho, C.-L., et al. (2021). Identification of cancer hub gene signatures associated with immune-suppressive tumor microenvironment and ovatodiolide as a potential cancer immunotherapeutic agent. Cancers 13, 3847. doi:10.3390/cancers13153847
Chen, J.-H., Wu, T., Tzeng, T. W., Huang, C.-C., Tzeng, Y.-M., and Chao, T.-Y. (2019). Antrocin, a bioactive component from Antrodia cinnamomea, suppresses breast carcinogenesis and stemness via downregulation of β-catenin/Notch1/Akt signaling. Phytomedicine 52, 70–78. doi:10.1016/j.phymed.2018.09.213
Chen, J.-J., Lin, W.-J., Liao, C.-H., and Shieh, P.-C. (2007). Anti-inflammatory benzenoids from Antrodia camphorata. J. Nat. Prod. 70, 989–992. doi:10.1021/np070045e
Chen, W., Sun, K., Zheng, R., Zeng, H., Zhang, S., Xia, C., et al. (2018). Cancer incidence and mortality in China, 2014. Chin. J. Cancer Res. 30, 1–12. doi:10.21147/j.issn.1000-9604.2018.01.01
Chen, X., Ma, R., Li, H., Tang, Y., and Yin, D. (2021). Bioinformatic analysis and identification of potential hallmarks in poorly differentiated thyroid cancer. doi:10.21203/rs.3.rs-153940/v1
Chen, Y.-A., Tzeng, D. T. W., Huang, Y.-P., Lin, C.-J., Lo, U.-G., Wu, C.-L., et al. (2019). Antrocin sensitizes prostate cancer cells to radiotherapy through inhibiting PI3K/AKT and MAPK signaling pathways. Cancers 11, 34. doi:10.3390/cancers11010034
Chen, Y., and Wang, X. (2019). miRDB: an online database for prediction of functional microRNA targets. Nucleic Acids Res. 48, D127–D131. doi:10.1093/nar/gkz757
Chiu, K.-Y., Wu, C.-C., Chia, C.-H., Hsu, S.-L., and Tzeng, Y.-M. (2016). Inhibition of growth, migration and invasion of human bladder cancer cells by antrocin, a sesquiterpene lactone isolated from Antrodia cinnamomea, and its molecular mechanisms. Cancer Lett. 373, 174–184. doi:10.1016/j.canlet.2015.11.046
Choi, J., Park, S., and Ahn, J. (2020). Refdnn: A reference drug based neural network for more accurate prediction of anticancer drug resistance. Sci. Rep. 10, 1861. doi:10.1038/s41598-020-58821-x
Chou, C. K., Yang, K. D., Chou, F. F., Huang, C. C., Lan, Y. W., Lee, Y. F., et al. (2013). Prognostic implications of miR-146b expression and its functional role in papillary thyroid carcinoma. J. Clin. Endocrinol. Metab. 98, E196–E205. doi:10.1210/jc.2012-2666
Colombo, C., Minna, E., Gargiuli, C., Muzza, M., Dugo, M., De Cecco, L., et al. (2020). The molecular and gene/miRNA expression profiles of radioiodine resistant papillary thyroid cancer. J. Exp. Clin. Cancer Res. 39, 245. doi:10.1186/s13046-020-01757-x
Deng, X., Wu, B., Xiao, K., Kang, J., Xie, J., Zhang, X., et al. (2015). MiR-146b-5p promotes metastasis and induces epithelial-mesenchymal transition in thyroid cancer by targeting ZNRF3. Cell. Physiol. biochem. 35, 71–82. doi:10.1159/000369676
Durante, C., Costante, G., Lucisano, G., Bruno, R., Meringolo, D., Paciaroni, A., et al. (2015). The natural history of benign thyroid nodules. Jama 313, 926–935. doi:10.1001/jama.2015.0956
Fagin, J. A., and Wells, S. A. (2016). Biologic and clinical perspectives on thyroid cancer. N. Engl. J. Med. 375, 1054–1067. doi:10.1056/NEJMra1501993
Farazi, T. A., Hoell, J. I., Morozov, P., and Tuschl, T. (2013). MicroRNAs in human cancer. Adv. Exp. Med. Biol. 774, 1–20. doi:10.1007/978-94-007-5590-1_1
Geethangili, M., and Tzeng, Y.-M. (2011). Review of pharmacological effects of Antrodia camphorata and its bioactive compounds. Evid. Based. Complement. Altern. Med. 2011, 212641. doi:10.1093/ecam/nep108
Geraldo, M. V., Yamashita, A. S., and Kimura, E. T. (2012). MicroRNA miR-146b-5p regulates signal transduction of TGF-β by repressing SMAD4 in thyroid cancer. Oncogene 31, 1910–1922. doi:10.1038/onc.2011.381
Gillet, J.-P., Calcagno, A. M., Varma, S., Marino, M., Green, L. J., Vora, M. I., et al. (2011). Redefining the relevance of established cancer cell lines to the study of mechanisms of clinical anti-cancer drug resistance. Proc. Natl. Acad. Sci. U. S. A. 108, 18708–18713. doi:10.1073/pnas.1111840108
Giordano, T. J., Au, A. Y., Kuick, R., Thomas, D. G., Rhodes, D. R., Wilhelm, K. G., et al. (2006). Delineation, functional validation, and bioinformatic evaluation of gene expression in thyroid follicular carcinomas with the PAX8-PPARG translocation. Clin. Cancer Res. 12, 1983–1993. doi:10.1158/1078-0432.ccr-05-2039
Gomez-Rueda, H., Palacios-Corona, R., Gutiérrez-Hermosillo, H., and Trevino, V. (2016). A robust biomarker of differential correlations improves the diagnosis of cytologically indeterminate thyroid cancers. Int. J. Mol. Med. 37, 1355–1362. doi:10.3892/ijmm.2016.2534
Gonda, T. A., Varro, A., Wang, T. C., and Tycko, B. (2010). Molecular biology of cancer-associated fibroblasts: Can these cells be targeted in anti-cancer therapy? Semin. Cell Dev. Biol. 21, 2–10. doi:10.1016/j.semcdb.2009.10.001
Handkiewicz-Junak, D., Swierniak, M., Rusinek, D., Oczko-Wojciechowska, M., Dom, G., Maenhaut, C., et al. (2016). Gene signature of the post-Chernobyl papillary thyroid cancer. Eur. J. Nucl. Med. Mol. Imaging 43, 1267–1277. doi:10.1007/s00259-015-3303-3
Hanwell, M. D., Curtis, D. E., Lonie, D. C., Vandermeersch, T., Zurek, E., and Hutchison, G. R. (2012). Avogadro: An advanced semantic chemical editor, visualization, and analysis platform. J. Cheminform. 4, 17. doi:10.1186/1758-2946-4-17
He, H., Jazdzewski, K., Li, W., Liyanarachchi, S., Nagy, R., Volinia, S., et al. (2005). The role of microRNA genes in papillary thyroid carcinoma. Proc. Natl. Acad. Sci. U. S. A. 102, 19075–19080. doi:10.1073/pnas.0509603102
Hinsch, N., Frank, M., Döring, C., Vorländer, C., and Hansmann, M. L. (2009). Qprt: A potential marker for follicular thyroid carcinoma including minimal invasive variant; a gene expression, RNA and immunohistochemical study. BMC Cancer 9, 93. doi:10.1186/1471-2407-9-93
Hu, Y., Wang, H., Chen, E., Xu, Z., Chen, B., Lu, G., et al. (2016). Candidate microRNAs as biomarkers of thyroid carcinoma: A systematic review, meta-analysis, and experimental validation. Cancer Med. 5, 2602–2614. doi:10.1002/cam4.811
John, B., Enright, A. J., Aravin, A., Tuschl, T., Sander, C., Marks, D. S., et al. (2004). Human microRNA targets. PLoS Biol. 2, e363. doi:10.1371/journal.pbio.0020363
Kalluri, R., and Weinberg, R. A. (2009). The basics of epithelial-mesenchymal transition. J. Clin. Invest. 119, 1420–1428. doi:10.1172/JCI39104
Kitahara, C. M., Schneider, A., and Brenner, A. V. (2018). “Thyroid cancer,” in Cancer epidemiology and prevention. 4th Edn, Editors M. J. Thun, M. S. Linet, J. R. Cerhan, C. A. Haiman, and D. Schottefeld (New York, NY: Oxford University Press), 839–860.
Knauf, J. A., Ma, X., Smith, E. P., Zhang, L., Mitsutake, N., Liao, X.-H., et al. (2005). Targeted expression of BRAFV600E in thyroid cells of transgenic mice results in papillary thyroid cancers that undergo dedifferentiation. Cancer Res. 65, 4238–4245. doi:10.1158/0008-5472.CAN-05-0047
Kuleshov, M. V., Jones, M. R., Rouillard, A. D., Fernandez, N. F., Duan, Q., Wang, Z., et al. (2016). Enrichr: A comprehensive gene set enrichment analysis web server 2016 update. Nucleic Acids Res. 44, W90–W97. doi:10.1093/nar/gkw377
Lassalle, S., Zangari, J., Popa, A., Ilie, M., Hofman, V., Long, E., et al. (2016). MicroRNA-375/SEC23A as biomarkers of the in vitro efficacy of vandetanib. Oncotarget 7, 30461–30478. doi:10.18632/oncotarget.8458
Lawal, B., Wang, Y.-C., Wu, A. T. H., and Huang, H.-S. (2021). Pro-oncogenic c-Met/EGFR, biomarker signatures of the tumor microenvironment are clinical and therapy response prognosticators in colorectal cancer, and therapeutic targets of 3-phenyl-2H-benzo[e][1,3]-oxazine-2,4(3H)-dione derivatives. Front. Pharmacol. 12, 691234. doi:10.3389/fphar.2021.691234
Lawal, B., Lee, C.-Y., Mokgautsi, N., Sumitra, M. R., Khedkar, H., Wu, A. T. H., et al. (2021). mTOR/EGFR/iNOS/MAP2K1/FGFR/TGFB1 are druggable candidates for N-(2,4-difluorophenyl)-2',4'-difluoro-4-hydroxybiphenyl-3-carboxamide (NSC765598), with consequent anticancer implications./MAP2K1/FGFR/TGFB1 are druggable candidates for N-(2, 4-difluorophenyl)-2′, 4′-difluoro-4-hydroxybiphenyl-carboxamide (NSC765598), with consequent anticancer implications. Front. Oncol. 11, 656738. doi:10.3389/fonc.2021.656738
Lawal, B., Lin, L.-C., Lee, J.-C., Chen, J.-H., Bekaii-Saab, T. S., Wu, A. T. H., et al. (2021). Multi-omics data analysis of gene expressions and alterations, cancer-associated fibroblast and immune infiltrations, reveals the onco-immune prognostic relevance of STAT3/CDK2/4/6 in human malignancies. Cancers 13, 954. doi:10.3390/cancers13050954
Lawal, B., Liu, Y.-L., Mokgautsi, N., Khedkar, H., Sumitra, M. R., Wu, A. T., et al. (2021). Pharmacoinformatics and preclinical studies of nsc765690 and nsc765599, potential stat3/cdk2/4/6 inhibitors with antitumor activities against nci60 human tumor cell lines. Biomedicines 9, 92. doi:10.3390/biomedicines9010092
Lawal, B., Shittu, O. K., Kabiru, A. Y., Jigam, A. A., Umar, M. B., Berinyuy, E. B., et al. (2015). Potential antimalarials from african natural products: A reviw. J. Intercult. Ethnopharmacol. 4, 318–343. doi:10.5455/jice.20150928102856
Lawal, B., Shittu, O. K., Oibiokpa, F. I., Berinyuy, E. B., and Mohammed, H. (2016). African natural products with potential antioxidants and hepatoprotectives properties: A review. Clin. Phytosci. 2, 23. doi:10.1186/s40816-016-0037-0
Lawal, B., Wu, A. T. H., and Huang, H.-S. (2022). Leveraging bulk and single-cell RNA sequencing data of nsclc tumor microenvironment and therapeutic potential of NLOC-15a, A novel multi-target small molecule. Front. Immunol. 13, 872470. doi:10.3389/fimmu.2022.872470
Lee, S., Bae, J. S., Jung, C. K., and Chung, W. Y. (2019). Extensive lymphatic spread of papillary thyroid microcarcinoma is associated with an increase in expression of genes involved in epithelial-mesenchymal transition and cancer stem cell-like properties. Cancer Med. 8, 6528–6537. doi:10.1002/cam4.2544
Li, S., Zhang, Y., Dong, J., Li, R., Yu, B., Zhao, W., et al. (2021). LINC00893 inhibits papillary thyroid cancer by suppressing AKT pathway via stabilizing PTEN. Cancer Biomark. 30, 277–286. doi:10.3233/CBM-190543
Li, T., Fan, J., Wang, B., Traugh, N., Chen, Q., Liu, J. S., et al. (2017). Timer: A web server for comprehensive analysis of tumor-infiltrating immune cells. Cancer Res. 77, e108–e110. doi:10.1158/0008-5472.can-17-0307
Li, T., Fu, J., Zeng, Z., Cohen, D., Li, J., Chen, Q., et al. (2020). TIMER2.0 for analysis of tumor-infiltrating immune cells. Nucleic Acids Res. 48, W509–W514. doi:10.1093/nar/gkaa407
Liu, C.-J., Hu, F.-F., Xia, M.-X., Han, L., Zhang, Q., Guo, A.-Y., et al. (2018). GSCALite: A web server for gene set cancer analysis. Bioinformatics 34, 3771–3772. doi:10.1093/bioinformatics/bty411
Luo, H., Xia, X., Kim, G. D., Liu, Y., Xue, Z., Zhang, L., et al. (2021). Characterizing dedifferentiation of thyroid cancer by integrated analysis. Sci. Adv. 7, eabf3657. doi:10.1126/sciadv.abf3657
Male, K. B., Rao, Y. K., Tzeng, Y.-M., Montes, J., Kamen, A., Luong, J. H., et al. (2008). Probing inhibitory effects of Antrodia camphorata isolates using insect cell-based impedance spectroscopy: Inhibition vs chemical structure. Chem. Res. Toxicol. 21, 2127–2133. doi:10.1021/tx800202a
Mancikova, V., Castelblanco, E., Pineiro-Yanez, E., Perales-Paton, J., de Cubas, A. A., Inglada-Perez, L., et al. (2015). MicroRNA deep-sequencing reveals master regulators of follicular and papillary thyroid tumors. Mod. Pathol. 28, 748–757. doi:10.1038/modpathol.2015.44
Minna, E., Brich, S., Todoerti, K., Pilotti, S., Collini, P., Bonaldi, E., et al. (2020). Cancer associated fibroblasts and senescent thyroid cells in the invasive front of thyroid carcinoma. Cancers (Basel) 12, E112. doi:10.3390/cancers12010112
Minna, E., Romeo, P., Dugo, M., De Cecco, L., Todoerti, K., Pilotti, S., et al. (2016). miR-451a is underexpressed and targets AKT/mTOR pathway in papillary thyroid carcinoma. Oncotarget 7, 12731–12747. doi:10.18632/oncotarget.7262
Mittal, V. (2018). Epithelial mesenchymal transition in tumor metastasis. Annu. Rev. Pathol. 13, 395–412. doi:10.1146/annurev-pathol-020117-043854
Molinaro, E., Romei, C., Biagini, A., Sabini, E., Agate, L., Mazzeo, S., et al. (2017). Anaplastic thyroid carcinoma: From clinicopathology to genetics and advanced therapies. Nat. Rev. Endocrinol. 13, 644–660. doi:10.1038/nrendo.2017.76
Molinaro, E., Romei, C., Biagini, A., Sabini, E., Agate, L., Mazzeo, S., et al. (2017). Anaplastic thyroid carcinoma: From clinicopathology to genetics and advanced therapies. Nat. Rev. Endocrinol. 13, 644–660. doi:10.1038/nrendo.2017.76
Mostafavi, S., Ray, D., Warde-Farley, D., Grouios, C., and Morris, Q. (2008). GeneMANIA: A real-time multiple association network integration algorithm for predicting gene function. Genome Biol. 9, S4. doi:10.1186/gb-2008-9-s1-s4
Onikanni, A. S., Lawal, B., Olusola, A. O., Olugbodi, J. O., Sani, S., Ajiboye, B. O., et al. (2021). Sterculia tragacantha lindl leaf extract ameliorates STZ-induced diabetes, oxidative stress, inflammation and neuronal impairment. J. Inflamm. Res. 14, 6749–6764. doi:10.2147/jir.s319673
Onikanni, A. S., Lawal, B., Oyinloye, B. E., Mostafa-Hedeab, G., Alorabi, M., Cavalu, S., et al. (2022). Therapeutic efficacy of Clompanus pubescens leaves fractions via downregulation of neuronal cholinesterases/Na+-K+ATPase/IL-1 β, and improving the neurocognitive and antioxidants status of streptozotocin-induced diabetic rats. Biomed. Pharmacother. 148, 112730. doi:10.1016/j.biopha.2022.112730
Ortiz, I. M. D. P., Barros-Filho, M. C., dos Reis, M. B., Beltrami, C. M., Marchi, F. A., Kuasne, H., et al. (2018). Loss of DNA methylation is related to increased expression of miR-21 and miR-146b in papillary thyroid carcinoma. Clin. Epigenetics 10, 144. doi:10.1186/s13148-018-0579-8
Peng, Y., and Croce, C. M. (2016). The role of MicroRNAs in human cancer. Signal Transduct. Target. Ther. 1, 15004. doi:10.1038/sigtrans.2015.4
Pita, J. M., Banito, A., Cavaco, B. M., and Leite, V. (2009). Gene expression profiling associated with the progression to poorly differentiated thyroid carcinomas. Br. J. Cancer 101, 1782–1791. doi:10.1038/sj.bjc.6605340
Pita, J. M., Figueiredo, I. F., Moura, M. M., Leite, V., and Cavaco, B. M. (2014). Cell cycle deregulation and TP53 and RAS mutations are major events in poorly differentiated and undifferentiated thyroid carcinomas. J. Clin. Endocrinol. Metab. 99, E497–E507. doi:10.1210/jc.2013-1512
Qu, T., Li, Y. P., Li, X. H., and Chen, Y. (2016). Identification of potential biomarkers and drugs for papillary thyroid cancer based on gene expression profile analysis. Mol. Med. Rep. 14, 5041–5048. doi:10.3892/mmr.2016.5855
Rao, Y. K., Wu, A. T., Geethangili, M., Huang, M.-T., Chao, W.-J., Wu, C.-H., et al. (2011). Identification of antrocin from Antrodia camphorata as a selective and novel class of small molecule inhibitor of Akt/mTOR signaling in metastatic breast cancer MDA-MB-231 cells. Chem. Res. Toxicol. 24, 238–245. doi:10.1021/tx100318m
Reeb, N., and Reigh-Yi, L. (2015). Microarray analysis identifies a unique molecular signature of human thyroid cancer stem cells. J. Thyroid. Disord. Ther. 4, 2. doi:10.4172/2167-7948.1000e179
Robertson, K. D. (2005). DNA methylation and human disease. Nat. Rev. Genet. 6, 597–610. doi:10.1038/nrg1655
Roche, J. (2018). The epithelial-to-mesenchymal transition in cancer. Cancers 10, 52. doi:10.3390/cancers10020052
Romeo, P., Colombo, C., Granata, R., Calareso, G., Gualeni, A. V., Dugo, M., et al. (2018). Circulating miR-375 as a novel prognostic marker for metastatic medullary thyroid cancer patients. Endocr. Relat. Cancer 25, 217–231. doi:10.1530/erc-17-0389
Rusinek, D., Swierniak, M., Chmielik, E., Kowal, M., Kowalska, M., Cyplinska, R., et al. (2015). BRAFV600E-Associated gene expression profile: Early changes in the transcriptome, based on a transgenic mouse model of papillary thyroid carcinoma. PloS one 10, e0143688. doi:10.1371/journal.pone.0143688
Salvatore, G., Nappi, T. C., Salerno, P., Jiang, Y., Garbi, C., Ugolini, C., et al. (2007). A cell proliferation and chromosomal instability signature in anaplastic thyroid carcinoma. Cancer Res. 67, 10148–10158. doi:10.1158/0008-5472.can-07-1887
Sepe, R., Pellecchia, S., Serra, P., D’Angelo, D., Federico, A., Raia, M., et al. (2018). The long non-coding RNA RP5-1024C24.1 and its associated-gene MPPED2 are down-regulated in human thyroid neoplasias and act as tumour suppressors. Cancers 10, 146. doi:10.3390/cancers10050146
Shinawi, T., Hill, V. K., Krex, D., Schackert, G., Gentle, D., Morris, M. R., et al. (2013). DNA methylation profiles of long- and short-term glioblastoma survivors. Epigenetics 8, 149–156. doi:10.4161/epi.23398
Steinbach, D., Sell, W., Voigt, A., Hermann, J., Zintl, F., and Sauerbrey, A. (2002). BCRP gene expression is associated with a poor response to remission induction therapy in childhood acute myeloid leukemia. Leukemia 16, 1443–1447. doi:10.1038/sj.leu.2402541
Sung, H., Ferlay, J., Siegel, R. L., Laversanne, M., Soerjomataram, I., Jemal, A., et al. (2021). Global cancer statistics 2020: GLOBOCAN estimates of incidence and mortality worldwide for 36 cancers in 185 countries. Ca. Cancer J. Clin. 71, 209–249. doi:10.3322/caac.21660
Tanase, C. P., Ogrezeanu, I., and Badiu, C. (2012). “8 - MicroRNAs,” in Molecular Pathology of pituitary adenomas. Editors C. P. Tanase, I. Ogrezeanu, and C. Badiu (London: Elsevier). 91–96. doi:10.1016/B978-0-12-415830-6.00008-1pp
Tarabichi, M., Saiselet, M., Trésallet, C., Hoang, C., Larsimont, D., Andry, G., et al. (2015). Revisiting the transcriptional analysis of primary tumours and associated nodal metastases with enhanced biological and statistical controls: Application to thyroid cancer. Br. J. Cancer 112, 1665–1674. doi:10.1038/bjc.2014.665
Tomás, G., Tarabichi, M., Gacquer, D., Hébrant, A., Dom, G., Dumont, J. E., et al. (2012). A general method to derive robust organ-specific gene expression-based differentiation indices: Application to thyroid cancer diagnostic. Oncogene 31, 4490–4498. doi:10.1038/onc.2011.626
Tran, T. H., and Montano, M. A. (2017). “Chapter 1 - MicroRNAs: Mirrors of health and disease,” in Translating MicroRNAs to the clinic. Editor J. Laurence (Boston: Academic Press). 1–15. doi:10.1016/B978-0-12-800553-8.00001-9pp
Trott, O., and Olson, A. J. (2010). AutoDock Vina: Improving the speed and accuracy of docking with a new scoring function, efficient optimization, and multithreading. J. Comput. Chem. 31, 455–461. doi:10.1002/jcc.21334
Udelsman, R., and Zhang, Y. (2014). The epidemic of thyroid cancer in the United States: The role of endocrinologists and ultrasounds. Thyroid 24, 472–479. doi:10.1089/thy.2013.0257
Vasko, V., Espinosa, A. V., Scouten, W., He, H., Auer, H., Liyanarachchi, S., et al. (2007). Gene expression and functional evidence of epithelial-to-mesenchymal transition in papillary thyroid carcinoma invasion. Proc. Natl. Acad. Sci. U. S. A. 104, 2803–2808. doi:10.1073/pnas.0610733104
Visualizer, D. S. (2020). San Diego: Dassault Systèmes.BIOVIA, dassault systèmes, BIOVIA workbook, release 2020
von Roemeling, C. A., Marlow, L. A., Pinkerton, A. B., Crist, A., Miller, J., Tun, H. W., et al. (2015). Aberrant lipid metabolism in anaplastic thyroid carcinoma reveals stearoyl CoA desaturase 1 as a novel therapeutic target. J. Clin. Endocrinol. Metab. 100, E697–E709. doi:10.1210/jc.2014-2764
Wu, A. T. H., Lawal, B., Tzeng, Y.-M., Shih, C.-C., and Shih, C.-M. (2022). Identification of a novel theranostic signature of metabolic and immune-inflammatory dysregulation in myocardial infarction, and the potential therapeutic properties of ovatodiolide, a diterpenoid derivative. Int. J. Mol. Sci. 23, 1281. doi:10.3390/ijms23031281
Wu, S.-Y., Lin, K.-C., Lawal, B., Wu, A. T. H., and Wu, C.-Z. (2021). MXD3 as an onco-immunological biomarker encompassing the tumor microenvironment, disease staging, prognoses, and therapeutic responses in multiple cancer types. Comput. Struct. Biotechnol. J. 19, 4970–4983. doi:10.1016/j.csbj.2021.08.047
Yeh, C.-T., Huang, W.-C., Rao, Y. K., Ye, M., Lee, W.-H., Wang, L.-S., et al. (2013). A sesquiterpene lactone antrocin from Antrodia camphorata negatively modulates JAK2/STAT3 signaling via microRNA let-7c and induces apoptosis in lung cancer cells. Carcinogenesis 34, 2918–2928. doi:10.1093/carcin/bgt255
Yeh, C.-T., Rao, Y. K., Yao, C.-J., Yeh, C.-F., Li, C.-H., Chuang, S.-E., et al. (2009). Cytotoxic triterpenes from Antrodia camphorata and their mode of action in HT-29 human colon cancer cells. Cancer Lett. 285, 73–79. doi:10.1016/j.canlet.2009.05.002
Yu, K., Ganesan, K., Tan, L. K., Laban, M., Wu, J., Zhao, X. D., et al. (2008). A precisely regulated gene expression cassette potently modulates metastasis and survival in multiple solid cancers. PLoS Genet. 4, e1000129. doi:10.1371/journal.pgen.1000129
Keywords: thyroid carcinoma (THCA), theranostic biomarkers, immune infiltration, epithelial-to-mesenchymal transition, t-cell exclusion
Citation: Shih M-L, Lawal B, Cheng S-Y, Olugbodi JO, Babalghith AO, Ho C-L, Cavalu S, Batiha GE-S, Albogami S, Alotaibi SS, Lee J-C and Wu ATH (2022) Large-scale transcriptomic analysis of coding and non-coding pathological biomarkers, associated with the tumor immune microenvironment of thyroid cancer and potential target therapy exploration. Front. Cell Dev. Biol. 10:923503. doi: 10.3389/fcell.2022.923503
Received: 19 April 2022; Accepted: 30 June 2022;
Published: 03 August 2022.
Edited by:
Paula Díez, Leiden University Medical Center, NetherlandsReviewed by:
Afeez Ishola, Johns Hopkins All Children’s Hospital, United StatesEusbong Berinyuy, University of Yaounde I, Cameroon
Copyright © 2022 Shih, Lawal, Cheng, Olugbodi, Babalghith, Ho, Cavalu, Batiha, Albogami, Alotaibi, Lee and Wu. This is an open-access article distributed under the terms of the Creative Commons Attribution License (CC BY). The use, distribution or reproduction in other forums is permitted, provided the original author(s) and the copyright owner(s) are credited and that the original publication in this journal is cited, in accordance with accepted academic practice. No use, distribution or reproduction is permitted which does not comply with these terms.
*Correspondence: Jih-Chin Lee, ZG9jMzA0NTBAZ21haWwuY29t; Alexander T. H. Wu, Y2hhdzEyMTFAdG11LmVkdS50dw==
†These authors have contributed equally to this work