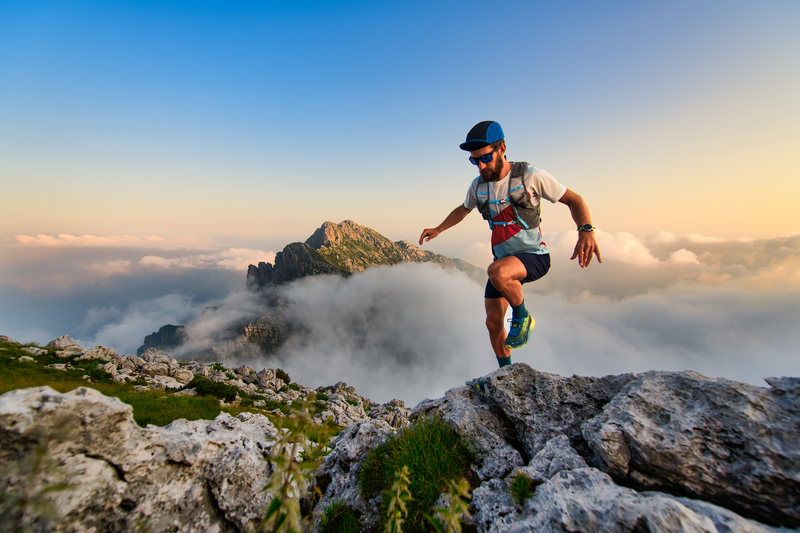
94% of researchers rate our articles as excellent or good
Learn more about the work of our research integrity team to safeguard the quality of each article we publish.
Find out more
REVIEW article
Front. Cell Dev. Biol. , 06 June 2022
Sec. Molecular and Cellular Pathology
Volume 10 - 2022 | https://doi.org/10.3389/fcell.2022.883861
This article is part of the Research Topic Single-Cell Analysis in Cardiovascular System Development and Diseases View all 5 articles
With the development of ever more powerful and versatile high-throughput sequencing techniques and innovative ways to capture single cells, mapping the multicellular tissues at the single-cell level is becoming routine practice. However, it is still challenging to depict the epigenetic landscape of a single cell, especially the genome-wide chromatin accessibility, histone modifications, and DNA methylation. We summarize the most recent methodologies to profile these epigenetic marks at the single-cell level. We also discuss the development and advancement of several multi-omics sequencing technologies from individual cells. Advantages and limitations of various methods to compare and integrate datasets obtained from different sources are also included with specific practical notes. Understanding the heart tissue at single-cell resolution and multi-modal levels will help to elucidate the cell types and states involved in physiological and pathological events during heart development and disease. The rich information produced from single-cell multi-omics studies will also promote the research of heart regeneration and precision medicine on heart diseases.
With the development of massively parallel DNA sequencing technologies, reading the whole genome and transcripts in cells has become a promising approach to studying cellular states. Transcriptomes can be amplified to sufficient quantity for high-throughput sequencing. Compared to conventional bulk sequencing methods, single-cell analysis resolves cell heterogeneity otherwise masked by bulk analysis. It enables new cell types and intermediate states to be discovered based on transcriptional and epigenetic signatures of individual cells. These cells are often missed in conventional studies using pre-defined markers or lineage tracing studies due to the lack of known markers or precursors. This is particularly important for studying human primary tissue samples, where knowledge from model organisms may not always be applicable. Recently, single-cell analysis has been used to study cell fate changes during the reprogramming of cardiomyocytes (CMs) (Liu et al., 2017), in the heart from gene knock-out animals (Nkx2.5 KO, Hand1 KO, etc.) (DeLaughter et al., 2016; de Soysa et al., 2019) or mouse model of myocardial infarction (MI), heart failure (HF), etc. (Martini et al., 2019; Wang Z. et al., 2020). Researchers could detect early changes in the transcriptome before the phenotype and cell fate changes in all cardiac cells, including CMs, fibroblast cells, immune cells, etc. The single-cell multi-omics atlas offers multi-modal and panoramic views of diseased hearts, allowing scientists to analyze from many different directions. Moreover, cell trajectory analysis based on single-cell data could reveal the continuum of cell fate transition, adding the temporal information missed from samples collected at particular timepoints.
The first single-cell whole-transcriptome profiling was reported by Tang et al., in 2009. Single oocytes were picked by mouth pipette, followed by reverse transcription, polyA tailing, and second-strand synthesis (Tang et al., 2009). Later, various single-cell capture, barcoding, and pooling strategies were developed to handle single cells more efficiently and automatically, which greatly facilitated the development of single-cell multi-omics sequencing.
The cell-type-specific epigenetic landscape is shaped by unique distributions of DNA methylation, chromatin accessibility, key histone marks, and transcription factors (TFs) binding across the genome. Genome-wide profiling of these epigenetic features facilitated the mechanistic study of the developmental process and gene function. The epigenomic profiling technologies include Assay for Transposase Accessible Chromatin with high-throughput sequencing (ATAC-seq) (Buenrostro et al., 2013), Chromatin immunoprecipitation assays with sequencing (ChIP-seq) (Park 2009) and DNA methylome sequencing (DNAme-seq), etc. (Meissner et al., 2005; Laird 2010). They usually require many cells because each cell only has one set of genomic DNA, and thus the number of the template is much less than the transcriptome. However, with the development of more powerful tool enzymes and a better streamlining of the procedure, chromatin analysis at the single-cell level has become a reality. It helps to reveal the heterogeneity in the epigenome and multi-modal regulation of gene expression.
To capture multi-modal information of single cells, samples can be split into multiple parts for multi-omics sequencing separately. However, multi-omics sequence information from the same cells can genuinely reveal the correlation between different layers of epigenetic modification and gene expression (Ma A. et al., 2020). With the explosion of sequencing data from multiple modalities, many algorithms were developed to analyze multi-modal information, compare data generated from different platforms, and remove batch effects for single-cell datasets. Here we will also briefly summarize the bioinformatic methods to merge or compare single-cell datasets and cross-reference epigenomic data derived from single cells with Genome-wide association studies (GWAS).
This review will summarize the platforms for single-cell capture and multi-omics sequencing, the recently emerged technologies and analysis tools, and their potential application in cardiovascular research.
Several effective methods were developed to amplify the transcriptome of a single cell, including tagging transcripts with T7 promoter and in vitro transcription by T7 RNA polymerase (Hashimshony et al., 2012) or template switching by M-MLV reverse transcriptase and template switching oligo (SMART-seq2 (Picelli et al., 2014)). These methods improved the sensitivity and streamlined the procedure of cDNA library generation, paving the way for higher throughput single-cell RNA-seq.
Various platforms are engineered to capture single cells to suit different needs. Based on where the cell barcoding takes place, the single-cell sorting strategy can be classified into three types, cell-per-well (CPW) strategy (Tang et al., 2009), droplet-based strategy (Klein et al., 2015; Macosko et al., 2015; Zheng et al., 2017; Zilionis et al., 2017; Briggs et al., 2018; Farrell et al., 2018; Wagner et al., 2018; Zhang X. et al., 2019), and single-cell combinatorial indexing (SCI) strategy (Cao et al., 2017; Rosenberg et al., 2018).
CPW strategies [e.g., manual picking, integrated fluidic circuit (IFC) system, ICELL8 platform, and Microwell-Seq] directly put one cell into one well or tube, followed by library construction separately. Droplet-based strategies [e.g., inDrops, Drop-seq, and 10✕ Genomics Chromium et al. (10✕)] use a “co-flow” device for cell encapsulation with barcoded microparticles to capture mRNA. SCI strategies use the intact cell or nucleus as an indexing unit, and each cell will, with high probability, have a unique barcode combination after multiple rounds of split-pool indexing (Figure 1). Here we will briefly summarize the advantages and features of the three strategies.
FIGURE 1. Graphical illustration of single-cell sorting strategy and multi-omics sequencing. The left side illustrates the principles of different cell sorting strategies, CPW, droplet-based, and SCI. Four types of commonly adopted CPW methods include manual picking of single cells; FC and ICELL8, which are both dependent on Hydrodynamic focusing for single-cell flow generation; microwell platform, which uses gravity and size selection for cell capture; IFC system, which integrates multi-steps of library construction. Droplet-based strategies use the co-flow system to generate the emulsion drops containing one cell and one uniquely barcoded bead to barcode the cell by capturing nucleic acid. SCI strategy barcode tens of cells simultaneously and repeat the barcoding after pooling and splitting. Multiple rounds of pooling and splitting followed by barcoding result in a high proportion of uniquely barcoded single cells. The ultimate goal of different cell capture and labeling strategies is to give each cell or nuclei a unique barcode. Then the multi-omics library construction can be performed.
The earliest CPW strategy relied on labor-intensive manually picking single cells into each tube (Tang et al., 2009; Islam et al., 2011; Wen and Tang 2016). Later, high-throughput platforms for CPW strategy are developed. IFC system [e.g., Fluidigm C1 (Wu et al., 2014)] and ICELL8 could perform cell isolation, lysis, and reverse transcription in dedicated chambers for each cell in an automated workflow, allowing profiling of several hundred cells per run. Sampling bias should be considered if input cells have different sizes. Technically, the IFC system is more suited for cells less than 25 µm in diameter, while ICELL8 and flow cytometry-based sorting have different nozzle sizes for bigger cells to pass with minimal damage.
Microwell-based methods (agarose-based microwell or BD Rhapsody™ microwell cartridge) offer another solution to improve the throughput and partially overcome the constraints in cell size. (Fan et al., 2015; Han et al., 2018). These methods cast plates containing over 105 microwells. Each microwell can seed one cell and one barcoded bead. Cells are seeded into microwells by gravity, followed by beads settling. After cell lysis in the plate, RNA transcripts are released into the microwell and captured by beads conjugated with barcoded primers; afterward, the beads are collected, and subsequent reverse transcription is performed in bulk. Compared to microfluidic-based workflows, microwell-based workflows offer higher cell throughput, higher tolerance for cell size variation, and gentle treatment by eliminating shear stress from micro-fluidic channels. Microwell-based methods can interrogate a high volume of samples in a single assay, albeit producing lower sequencing depth. It is an excellent choice for large-scale single-cell profiling. For example, Han et al. depicted the cell atlas of mice (more than 400 thousand cells) and human cells (more than 700 thousand cells) using the microwell platform (Han et al., 2018; Han et al., 2020).
Droplet-based platforms pack single cells and barcoded beads into nanoliter droplets in oil emulsions and utilize these tiny droplets as the reaction chambers. This approach eliminates the need for microfluidic chambers or individual tubes and significantly improves the throughput.
There are three state-of-art droplet-based scRNA-seq strategies, inDrops (Zilionis et al., 2017), Drop-seq (Macosko et al., 2015), and 10✕ genomics. These three systems employ different types of beads (deformable hydrogel beads for inDrop, dissolvable gel beads for 10✕, and hard, brittle resin beads for Drop-seq). All three systems perform cell lysis and mRNA capture in a droplet. However, the reverse transcription reaction is inside droplet for inDrops, out of droplet for 10✕ and Drop-seq. 10✕ and Drop-seq pipelines can be completed within one day. According to a benchmark comparison among the three droplet-based systems, 10✕ shows the highest sensitivity and proportion of effective reads and relatively fewer mismatches in cell barcodes, indicating good quality control in bead fabrication and well-optimized chemistry in DNA synthesis (Zhang X. et al., 2019). However, Drop-seq has considerable advantages in input and cost (∼$0.1 per cell for Drop-seq, ∼$0.5 for 10✕) with a slight compromise in sensitivity. Overall, droplet-based platforms yield very high cell throughput (103∼104 cells per run) with sufficient gene coverage (average 10–50 thousand unique reads for each cell, 3000–6000 detected genes), but they all require specific instruments for droplet generation and have a limitation on cell size (less than 30 µm in diameter for most pipelines).
The single-cell combinatorial indexing (SCI) method is a new high-throughput single-cell sequencing paradigm. It takes advantage of the intactness of the cell nucleus during reverse transcription and Tn5 tagmentation. The nuclear membrane is a physical barrier where the indexing reaction occurs inside. Cells are indexed in dozens of wells simultaneously, and multiple rounds of indexing are carried out through pooling and splitting cells into other dozens of wells. After multiple rounds of split-pool indexing, each nucleus will, with high probability, have a unique barcode combination. The SCI method quickly gained popularity because it requires no special instruments other than conventional flow cytometry equipment, and the cost per cell is vastly reduced.
In 2017, Junyue Cao et al. developed sci-RNA-seq that involved two rounds of indexing: the first round during the reverse transcription and the second round during the PCR amplification (Cao et al., 2017). They reported over 20,000–50,000 reads per cell using human and mouse cell lines and profiled over 50,000 C. elegans cells with a median of 575 Unique Molecular Identifiers (UMIs). Other methods employed more complex indexing steps to increase the input or sample multiplexing. For example, in 2018, Alexander B. Rosenberg et al. developed SPLiT-seq that introduced four rounds of barcoding (one round during reverse transcription, two rounds by ligation, and the final round through PCR amplification), which yielded over 21 million barcode combinations, vastly decreased the probability of barcode collisions (Rosenberg et al., 2018). In 2020, Sanjay R. Srivatsan et al. first used indexed polyadenylated single-strand DNA to tag each sample, then pooled all samples for downstream three rounds of combinatorial indexing, which allowed them to profile >650,000 cells from more than 5000 independent samples, demonstrating the power of SCI methods in High Throughput Screen studies (Srivatsan et al., 2020). SCI strategy has also been employed to profile chromatin accessibility (Cusanovich et al., 2015), genome sequence (Vitak et al., 2017), 3D genome conformation (Ramani et al., 2017), and DNA methylation (Mulqueen et al., 2018) in an ultra-high throughput manner.
Cell fate determination is a highly regulated process accompanied by changes in the epigenetic landscape, including DNA methylation, open chromatin, and histone modifications. Bulk sequencing of these epigenomic modalities has been well-developed and recently adapted to single-cell studies. Here we will briefly summarize single-cell Assay for Transposase Accessible Chromatin with high-throughput sequencing (scATAC-seq), single-cell Chromatin immunoprecipitation assays with sequencing (scChIP-seq), and single-cell DNA methylome sequencing (scDNAme-seq).
Open chromatin regions often harbor more transcription factor (TF) binding sites and regulatory regions, which are of great interest. Several enzymes preferably cut the open regions of genome DNA [e.g. deoxyribonuclease I (DNase I), Micrococcal nuclease (MNase), Tn5n transposase] and thus have been used to interrogate chromatin accessibility (Jin et al., 2015) (Schones et al., 2008; Valouev et al., 2011). DNase-based probing combined with either restriction enzyme cleavage (Boyle et al., 2008) or size selection (Hesselberth et al., 2009) produced the first accessible chromatin dataset (Crawford et al., 2006; Sabo et al., 2006; Thurman et al., 2012). Later, Buenrostro et al. used Tn5 transposase to bind, fragment, and tag the accessible genome region with sequencing adaptors and developed ATAC-seq (Buenrostro et al., 2013). ATAC-seq significantly streamlined the processes and increased the sensitivity compared to DNase-seq.
In 2015, scATAC-seq was developed. Researchers isolated 254 GM12878 lymphoblastoid cells and used Tn5 tagmentation to profile the accessible chromatin on the CPW platform. The authors showed that the open chromatin profile from single cells strongly correlated with those obtained by DNase-seq and bulk ATAC-seq generated from tens of thousands or millions of cells. An average of 7.3 × 104 fragments were generated from each cell and mapped to the human genome (Buenrostro et al., 2015). Other CPW-based scATAC-seq were also developed later to analyze cells captured by flow cytometry (Chen et al., 2018a; Chen et al., 2018b), Takara ICELL8 (Mezger et al., 2018), or microwell (Chen et al., 2021).
In 2015, Cusanovich et al. developed sci-ATAC-seq based on the SCI strategy (Cusanovich et al., 2015). The initial version of sci-ATAC-seq analyzed hundreds of cells, and the median reads per cell were only 2.5 × 103. However, the theoretical barcode combination of sci-ATAC-seq is sufficient for tens of thousands of single nuclei. In 2018, Shendure and his colleagues performed sci-ATAC-seq on thousands of cells in one experiment and obtained an average of 13,000 de-duplicated unique reads per cell (Cusanovich et al., 2018a; Cusanovich et al., 2018b). Later in 2020, sci-ATAC-seq was upgraded to sci-ATAC-seq3 by performing three rounds of indexing through ligation instead of one-round Tn5 indexing. After five years of optimization, researchers reduced the collision rate from 11% in original sci-ATAC-seq to lower than 4% in sci-ATAC-seq3 and increased the throughput from thousands of cells to millions of cells. With sci-ATAC-seq3, the authors profiled 1.6 million cells from 59 fetal samples and generated a human fetal cell atlas of chromatin accessibility (Domcke et al., 2020).
Meanwhile, Buenrostro and his colleague developed dsc-ATAC-seq by employing droplet-based single-cell capturing (Lareau et al., 2019). The super-load beads strategy processed 46,000 adult mouse brain cells from 12 experiments and generated 34,000 median unique reads per cell. 10✕ Chromium also launched a standardized commercialized solution for scATAC-seq with a lower multiplet rate (<1%) and high coverage (27.8 × 10^3 mean unique reads per cell) (Satpathy et al., 2019). In addition, dsci-ATAC-seq combined droplet-based dsc-ATAC-seq and SCI strategy, adding another round of indexing by split and pool, which increased the throughput by roughly two orders of magnitude. Caleb et al. had adopted dsci-ATAC-seq to profile more than 60,000 human bone marrow cells with around 3,800 unique reads per cell (Lareau et al., 2019).
Modification of histone tails regulates the structure of chromatin and significantly influences gene expression. Several well-known histone modifications are associated with active transcription, including H3K4me1 [active and primed enhancers (Local et al., 2018)], H3K4me2 [active and primed promoter (Pekowska et al., 2010)], H3K4me3 [active promoter, mainly at regions flanking transcription starting sites (Liu et al., 2016)], H3K27ac [active enhancers and promoters (Creyghton et al., 2010)], H3K36me3 [actively transcribed genes (Musselman et al., 2013)]. Histone modifications associated with transcription repression are also of great importance, especially H3K27me3 [repressed loci (Liu et al., 2016)] and H3K9me3 (marking heterochromatin) (Consortium, Encode Project, 2012; Nicetto and Zaret 2019).
ChIP-seq has been widely adopted to profile different types of histone modification and define various regulatory elements in cardiovascular development and disease studies (Creyghton et al., 2010; Shen et al., 2012; Akerberg et al., 2019; Nakato et al., 2019; Reyes-Palomares et al., 2020). Henikoff and his colleagues developed Cleavage Under Targets & Release Using Nuclease (CUT&RUN), where antibodies of specific histone modifications were bound by protein A which was covalently linked to MNase. The MNase cleavages DNA on either side of the antibody binding site. CUT&RUN combined the endonuclease activity of MNase and the specificity of the antibody. It also bypassed the immunoprecipitation step and significantly simplified the ChIP-seq process. Henikoff group showed that CUT&RUN generated a dataset with a higher signal-to-noise ratio. Rotem et al. adopted CUT&RUN to the droplet-based single-cell capturing platform to profile H3K4me3 and H3K4me2 of mouse embryonic cells, demonstrating that the sensitivity of CUT&RUN is sufficient for single-cell profiling (Rotem et al., 2015). Henikoff group further developed Cleavage Under Targets and Tagmentation (CUT&Tag), where MNase was replaced by Tn5 transposase, which offers the advantage of direct tagmentation of the antibody binding site. CUT&Tag was widely used to profile the genome-wide association of TFs and histone modifications. Although CUT&RUN and CUT&Tag avoided the immunoprecipitation procedure, some researchers still use the term ChIP-seq to nominate these two methods. CUT&Tag has been combined with different single-cell capture strategies to profile histone modification at the single-cell level (e.g., CPW: ChIL-seq (Harada et al., 2019), SCI: itChIP-seq (Ai et al., 2019) and droplet-based strategy: scChIP-seq, scCUT&Tag (Grosselin et al., 2019; Kaya-Okur et al., 2019; Bartosovic et al., 2021)).
DNA methylation is a fundamental epigenetic modality closely linked to cell identity and transcriptional regulation. Methylation at the promoter region often marks silenced genes, while methylation in the gene body is a feature of actively transcribed genes (Jones 2012). Bisulfite conversion leads to the deamination of unmethylated cytosines into uracil. Whole-genome bisulfite sequencing (WGBS) can profile methylated CpG sites across the genome at the single-nucleotide resolution but require a large amount of input DNA and deep sequencing. In comparison, reduced representative bisulfite sequencing (RRBS) used the MspI restriction enzyme to enrich CpG sites, thus reducing the sequencing cost at the expense of relatively lower genome coverage.
Based on bulk WGBS and RRBS methods, scRRBS (Guo et al., 2013; Guo et al., 2015) and scBS-seq (Smallwood et al., 2014; Clark et al., 2017) are the first two scDNAme-seq methods. Importantly, scBS-seq is the first method to use the post bisulfite adaptor tagging (PBAT) strategy to minimize the impact of DNA degradation during the bisulfite conversion step. A scDNAme-seq database of more than 8,000 cells across 29 cell types was obtained and provided a valuable database for DNA methylome study (Zong et al., 2022).
Several methods have been developed to profile DNA methylome, transcriptome, and other epigenetic information simultaneously. Most methods physically separate the nucleus and the cytoplasm for bisulfite sequencing and RNA-seq, such as scMT-seq (Hu et al., 2016) and scTrio-seq (Hou et al., 2016). Other methods [scNOMe-seq (Pott 2017) and scCOOL-seq (Li et al., 2018)] utilized GpC methylase, M.CviPI, which specifically methylate GpC sites in the open chromatin regions. This approach enabled profiling DNA methylation and chromatin accessibility simultaneously.
In recent years, it has become feasible to simultaneously profile chromatin accessibility, histone modification, TF binding, DNA methylation, and transcriptome from the same cell, namely single-cell multi-omics sequencing (scMulti-omics-seq). ScMulti-omics-seq avoids the need to match datasets from parallel samples, reducing the technical variations. ScMulti-omics-seq can potentially be used to distinguish fluctuation in gene expression and different cell states due to a typical larger feature set than scRNA-seq, which is limited by the number of genes expressed and sequenced (Corces et al., 2016; Chen H. et al., 2019).
The first single-cell RNA-ATAC co-assay method is sciCAR-seq, published in 2018 by Jay Shendure and his colleagues, who had adopted the concept of SCI to scMulti-omics-seq by performing reverse transcription and tagmentation consecutively in the same nuclei before the second round of indexing (Cao et al., 2018). This research provided the proof-of-concept for sciMulti-omic-seq; however, low sensitivity led to a relatively imperfect correlation between gene expression and chromatin accessibility. Paired-seq (Zhu et al., 2019) and SHARE-seq (Ma S. et al., 2020) significantly upgraded the throughput by multiple rounds of indexing. In addition, tagmentation of the genome before reverse transcription prevented cDNA contamination in ATAC libraries due to the potential tagmentation of RNA/DNA hybrids (Di et al., 2020; Lu et al., 2020). Another strategy for transcriptomic chromatin accessibility co-assay is to use splint oligo to bridge tagmented DNA and oligo (dT) bearing barcoded beads so that they can be barcoded with captured RNA and sequenced together (Chen S. et al., 2019). Other studies try to segregate cytosol and nucleus from the same cell and perform scRNA-seq together with scATAC-seq (Liu L. et al., 2019) or scDNAme-seq based on either scBS-seq (Angermueller et al., 2016) or RRBS method (Hu et al., 2016).
Recently published Paired-tag (Zhu et al., 2021) and CoTECH (Xiong et al., 2021) have accomplished the co-assay of single histone modification and transcriptome by performing CUT&Tag and reverse transcription sequentially utilizing the SCI strategy. CoTECH adopted one round of split-pool, leading to relatively lower throughput (theoretical barcode complexity is about 104). Paired-tag adopted three rounds of barcoding through ligation, reaching a theoretically thousand folds higher complexity. Unique reads generated by Paired-tag varied among different histone markers but generally fell within 1-10k, sufficient for analyzing differentially modified regulatory elements. CoTECH generates a dataset with a slightly lower number of unique reads. Zhu et al. profiled ∼70,000 mouse brain cells on H3K4me1, H3K4me3, H3K27ac, H3K27me3, and H3K9me3 genome-wide association and the transcriptome. They successfully partitioned cells into different neuron types using H3K4me1 and H3K27ac modals alone, but H3K4me3, H3K27me3, and H3K9me3 modals failed to resolve the cellular heterogeneity and only clustered cells into broad brain cell types, indicating that it may be unfeasible to link different histone modification modalities directly if matched RNA information is not profiled. Using the transcriptome to bridge with other modalities, they assembled five histone modification datasets to form a comprehensive epigenetic landscape of mouse neurons.
Paired-tag and CoTECH can only profile transcriptome and one single histone modification at a time, so it is not possible to study the relationship between different histone modifications around the same genomic region. To map multiple histone modifications simultaneously in the same cell, multi-CUT&Tag (Gopalan et al., 2021) and MulTI-Tag (Meers et al., 2021) are developed. Both used indexed pA-Tn5 to connect with antibodies of different histone modifications. Multi-CUT&Tag used pre-assembled indexed pA-Tn5 and antibodies for different histone modifications, while MulTI-Tag adopted a covalently conjugated Tn5-antibody complex. According to the preliminary result from Gopalan et al., covalent conjugation significantly removed the cross-contamination between different histone modification maps. Interestingly, MulTI-Tag reported that about 10% of reads would have a different index in each end, which indicated that this region is co-occupied by two histone modifications in the same cell. However, the mix-indexed ratio reported by multi-CUT&Tag is higher (18–20%), possibly due to cross-contamination of histone modalities. Generally, Multi-CUT&TAG and MulTI-Tag are promising tools to investigate the relationship between different histone modifications and their influence on the transcriptome.
New bioinformatics methods are needed to analyze the vast amount of data generated from scMulti-omics sequencing experiments. Comparative analysis of the same modality, e.g., scATAC-seq, from different experiments turns out to be challenging, as researchers often need to remove batch effects due to different cell capturing strategies, library construction methods, or sequencing platforms. The choice of batch-removal strategy can be significantly determined by the research topic. According to a benchmark study (Luecken et al., 2022), if cell identities are known, that is, one is not expecting novel cell types, then it should be beneficial to integrate scRNA-seq batches via scANVI (Xu et al., 2021) or scGen(Lotfollahi et al., 2019). However, Scanorama (Hie et al., 2019) and scVI (Lopez et al., 2018) are recommended for the large unlabeled dataset, while Harmony (Korsunsky et al., 2019) seems more beneficial for the unlabeled smaller dataset with distinct biological signal (Tran et al., 2020).
Multi-omics data analysis needs to consider distinct modalities’ different sizes and unique nature. Chromatin accessibility and DNA methylome datasets contained information across the whole genome, and histone modifications also mark a significant fraction of the genome. These datasets are significantly larger than the transcriptome (usually no more than 5% of the genome) and TF binding regions. Dozens of computational strategies have been developed to perform integrative analysis of multi-modal datasets, including matched (multi-omics data from the same cell) or unmatched datasets (different modalities profiled from different cells) (Miao et al., 2021). For matched datasets, scAI (Jin et al., 2020), and MOFA+ (Argelaguet et al., 2020) could integrate scRNA and scATAC data. There are more algorithms developed to integrate unmatched scATAC-seq and scRNA-seq. MAESTRO (Wang C. et al., 2020) performs well on a dataset after manually annotating cell types and then analyzing the clustered cell-type level. LIGER (Welch et al., 2019) and Seurat 3.0 (Stuart et al., 2019) integrate scATAC-seq and scRNA-seq data by first predicting putative gene expression by nearest accessibility peak and integrating predicted and observed expression data. SCOT (Demetci et al., 2022) constructed similarity matrixes for each modality separately and integrated them through an optimal transport algorithm. LIGER also performs well for integrative analysis of transcriptome and DNA methylome data but needs a converted feature set, while MMD-MA (Liu J. et al., 2019), UnionCom (Cao et al., 2020) do not require feature matching.
GWAS has identified hundreds of thousands of genetic variants associated with the broad spectrum of human traits and diseases, most of which are noncoding (Claussnitzer et al., 2020). It is challenging but informative to link the noncoding GWAS variants to specific biological processes, pathways, or putative target genes. VAMPIRE has tried to cross-reference datasets of different modalities (e.g., epigenome, transcriptome, and 3D genome conformation) to annotate GWAS loci (Sun et al., 2022). scATAC-seq and scChIP-seq can also bridge noncoding GWAS variants with specific cell types, which provides valuable insights into the etiology and pathology of diseases (Ord et al., 2021). In addition, Cicero could predict cis-regulatory DNA interactions and construct chromatin co-accessibility networks, then correlated GWAS loci to specific genes within the network (Pliner et al., 2018).
In recent years, increasing numbers of studies employed scRNA-seq to depict the cell atlas of embryonic, adult, and diseased heart and vasculatures, such as dilated cardiomyopathy (See et al., 2017; Nomura et al., 2018; Rao et al., 2021), congenital heart defect (Suryawanshi et al., 2020), hypoplastic left heart syndrome (Miao et al., 2020), aortic stenosis (Nicin et al., 2020; Nicin et al., 2022), heart failure (Nicin et al., 2020), calcific aortic valve disease (Xu et al., 2020), Ascending thoracic aortic aneurysm (Li et al., 2020), and ischemic cardiomyopathy (Rao et al., 2021) (Table 1).
Sampling on cardiovascular tissues, especially from the heart, requires special cautions. The size of CM (100–150 μm by 20–35 μm) poses a significant challenge for single-cell analysis. Cui et al. adopted modified STRT-seq (Li et al., 2017; Cui et al., 2019) to construct single-cell transcriptome libraries, where mouth pipetting was adopted to capture single cells. ICELL8 platform offers an automated solution to harvest giant cells such as CMs. Another widely adopted solution is to construct libraries on single-nucleus and single-cell simultaneously for heart profiling, and the transcriptome data of CM are primarily derived from snRNA-seq (Litvinukova et al., 2020; Tucker et al., 2020). Several other features of the human heart (i.e., compactness, compartmentalization, and heterogeneity) make it even harder to isolate all types of cells unbiasedly with minimal damage. Li Wang et al. adopted both CM-enriched digestion (Guo et al., 2018) and conventional enzyme digestion of the left ventricle and left auricle/left atrial appendage to harvest large quantities of CMs and non-cardiomyocytes (NCMs) and performed single-cell RNA-seq through ICELL8 platform.
Some studies have adopted scRNA-seq to profile human pluripotent stem cell (hPSC) derived CMs. Data mining in the scRNA-seq helped optimize hPSC differentiation protocols (Zhang H. et al., 2019). Yang et al. employed RNA velocity and SLICER-based trajectory reconstruction to identify the critical fate decision process during cardiac reprogramming. They also developed a cell fate index (CFI) algorithm to assess reprogramming progression and provide valuable insights into how to optimize the differentiation methods (Zhou et al., 2019). Based on hPSC-derived CMs, scRNA-seq was also used to measure the gene regulatory network in drug screening (Ballan et al., 2020), cellular processes under physiological (Cyganek et al., 2018; Gambardella et al., 2019; Schmid et al., 2021; Esfandyari et al., 2022) and pathological conditions (Guo et al., 2019) (Kathiriya et al., 2021) and developmental progression (Hulin et al., 2019; Ruan et al., 2019; Sahara et al., 2019).
ScATAC-seq, combined with scRNA-seq, provides valuable insights into characteristics underlying developmental plasticity. Information from single-cell chromatin accessibility during the cardiovascular system development can predict the cell-type-specific regulatory TFs through motif enrichment analysis. Furthermore, researchers are more convinced by the more stable epigenetic landscape of the cell for cell-type identification, while RNA expression can be variable and change rapidly (Depuydt et al., 2020; Alexanian et al., 2021; Hocker et al., 2021).
Only a few published research used scATAC-seq to profile embryonic or adult human hearts. Most of them focus on constructing the regulome atlas (Domcke et al., 2020; Zhang et al., 2021). Hocker et al. performed sci-ATAC-seq of around 80,000 cells from adult human hearts without known cardiovascular disease (CVD) and constructed a human cardiac cis-regulatory element (CRE) atlas of different cell types. Apart from CMs, four types of NCMs (cardiac fibroblasts, endothelial cells, smooth muscle cells, and macrophages) were profiled with specific cardiac chamber annotated. Thus, this research depicted a chromatin accessibility map at single-cell resolution with spatial information. scRNA-seq were performed simultaneously, aiding cell type and subtype annotation. In addition, transcriptome profiling also helped to verify and refine the conclusions derived from scATAC-seq. Enrichment of binding motif for the macrophage TF SPI1/PU.1 combined with high SPI1 expression in macrophage helps to define SP1 as the specific TF in cardiac macrophage. The binding motifs of GATA-family TF were enriched in open chromatin regions of EC, CM, and cardiac fibroblast. GATA6 is highly expressed in cardiac fibroblast, while GATA4 and GATA6 in CM and GATA2 in EC) (Hocker et al., 2021). Such combined analysis helps identify active TFs in the development of different lineages.
The human cardiac CRE atlas derived from the scATAC-seq can be a valuable reference to advance our understanding of gene regulatory mechanisms. For example, for cardiac fibroblasts, there is more differential accessible CREs between right and left ventricles than between atriums and ventricles. Researchers have detected several fibroblast-specific CREs at FN1 gene and adjacent to MMP2 and FBLN2 genes, which all showed higher accessibility in the left atrium. These findings may indicate a more activated fibroblast state associated with higher ECM production in the left atrium (Hocker et al., 2021).
The technology to acquire and analyze multi-omics information from a single cell is rapidly evolving. Single-cell analysis has already provided an unprecedented amount of information about the cellular composition of the heart during development, homeostasis, and diseased conditions. However, there are many pending questions. How does the epigenetic landscape change in different parts of the heart and cell types during embryo development? How do different cardiac cells interact with immune cells during myocardial infarction and heart failure? Are there intermediate cell states during heart regeneration? How do drugs affect different types of cells in the heart? Answering these complex questions requires full resolution of cardiac cell heterogeneity at multiple layers. The multi-modal information at the single-cell level will significantly improve our effort to understand the molecular mechanism regulating cell fate and states in healthy and diseased conditions and find better biomarkers and drug targets to treat cardiovascular diseases.
XZ and HQ conceived the review, FZ and SD contributed to various parts of the review. The authors declare no conflict of interest.
This work was supported by the National Key R&D Program of China Grant (2019YFA0110001 and 2017YFA0102802), the National Natural Science Foundation of China (NSFC) Grant (31970819), and the Tsinghua University Spring Breeze Fund (2021Z99CFY033). The Beijing College Students’ innovation and entrepreneurship training program S202110003055 to XZ.
The authors declare that the research was conducted in the absence of any commercial or financial relationships that could be construed as a potential conflict of interest.
All claims expressed in this article are solely those of the authors and do not necessarily represent those of their affiliated organizations, or those of the publisher, the editors and the reviewers. Any product that may be evaluated in this article, or claim that may be made by its manufacturer, is not guaranteed or endorsed by the publisher.
Ai, S., Xiong, H., Li, C. C., Luo, Y., Shi, Q., Liu, Y., et al. (2019). Profiling Chromatin States Using Single-Cell itChIP-Seq. Nat. Cell. Biol. 21 (9), 1164–1172. doi:10.1038/s41556-019-0383-5
Akerberg, B. N., Gu, F., VanDusen, N. J., Zhang, X., Dong, R., Li, K., et al. (2019). A Reference Map of Murine Cardiac Transcription Factor Chromatin Occupancy Identifies Dynamic and Conserved Enhancers. Nat. Commun. 10 (1), 4907. doi:10.1038/s41467-019-12812-3
Alexanian, M., Przytycki, P. F., Micheletti, R., Padmanabhan, A., Ye, L., Travers, J. G., et al. (2021). A Transcriptional Switch Governs Fibroblast Activation in Heart Disease. Nature 595 (7867), 438–443. doi:10.1038/s41586-021-03674-1
Angermueller, C., Clark, S. J., Lee, H. J., Macaulay, I. C., Teng, M. J., Hu, T. X., et al. (2016). Parallel Single-Cell Sequencing Links Transcriptional and Epigenetic Heterogeneity. Nat. Methods 13 (3), 229–232. doi:10.1038/nmeth.3728
Argelaguet, R., Arnol, D., Bredikhin, D., Deloro, Y., Velten, B., Marioni, J. C., et al. (2020). MOFA+: a Statistical Framework for Comprehensive Integration of Multi-Modal Single-Cell Data. Genome Biol. 21 (1), 111. doi:10.1186/s13059-020-02015-1
Ballan, N., Shaheen, N., Keller, G. M., and Gepstein, L. (2020). Single-Cell Mechanical Analysis of Human Pluripotent Stem Cell-Derived Cardiomyocytes for Drug Testing and Pathophysiological Studies. Stem Cell. Rep. 15 (3), 587–596. doi:10.1016/j.stemcr.2020.07.006
Bartosovic, M., Kabbe, M., and Castelo-Branco, G. (2021). Single-cell CUT&Tag Profiles Histone Modifications and Transcription Factors in Complex Tissues. Nat. Biotechnol. 39 (7), 825–835. doi:10.1038/s41587-021-00869-9
Boyle, A. P., Davis, S., Shulha, H. P., Meltzer, P., Margulies, E. H., Weng, Z., et al. (2008). High-resolution Mapping and Characterization of Open Chromatin across the Genome. Cell. 132 (2), 311–322. doi:10.1016/j.cell.2007.12.014
Briggs, J. A., Weinreb, C., Wagner, D. E., Megason, S., Peshkin, L., Kirschner, M. W., et al. (2018). The Dynamics of Gene Expression in Vertebrate Embryogenesis at Single-Cell Resolution. Science 360 (6392), eaar5780. doi:10.1126/science.aar5780
Buenrostro, J. D., Giresi, P. G., Zaba, L. C., Chang, H. Y., and Greenleaf, W. J. (2013). Transposition of Native Chromatin for Fast and Sensitive Epigenomic Profiling of Open Chromatin, DNA-Binding Proteins and Nucleosome Position. Nat. Methods 10 (12), 1213–1218. doi:10.1038/nmeth.2688
Buenrostro, J. D., Wu, B., Litzenburger, U. M., Ruff, D., Gonzales, M. L., Snyder, M. P., et al. (2015). Single-cell Chromatin Accessibility Reveals Principles of Regulatory Variation. Nature 523 (7561), 486–490. doi:10.1038/nature14590
Cao, J., Cusanovich, D. A., Ramani, V., Aghamirzaie, D., Pliner, H. A., Hill, A. J., et al. (2018). Joint Profiling of Chromatin Accessibility and Gene Expression in Thousands of Single Cells. Science 361 (6409), 1380–1385. doi:10.1126/science.aau0730
Cao, J., Packer, J. S., Ramani, V., Cusanovich, D. A., Huynh, C., Daza, R., et al. (2017). Comprehensive Single-Cell Transcriptional Profiling of a Multicellular Organism. Science 357 (6352), 661–667. doi:10.1126/science.aam8940
Cao, K., Bai, X., Hong, Y., and Wan, L. (2020). Unsupervised Topological Alignment for Single-Cell Multi-Omics Integration. Bioinformatics 36 (Suppl. l_1), i48–i56. doi:10.1093/bioinformatics/btaa443
Chen, H., Lareau, C., Andreani, T., Vinyard, M. E., Garcia, S. P., Clement, K., et al. (2019a). Assessment of Computational Methods for the Analysis of Single-Cell ATAC-Seq Data. Genome Biol. 20 (1), 241. doi:10.1186/s13059-019-1854-5
Chen, H., Liao, Y., Zhang, G., Sun, Z., Yang, L., Fang, X., et al. (2021). High-throughput Microwell-Seq 2.0 Profiles Massively Multiplexed Chemical Perturbation. Cell. Discov. 7 (1), 107. doi:10.1038/s41421-021-00333-7
Chen, S., Lake, B. B., and Zhang, K. (2019b). High-throughput Sequencing of the Transcriptome and Chromatin Accessibility in the Same Cell. Nat. Biotechnol. 37 (12), 1452–1457. doi:10.1038/s41587-019-0290-0
Chen, X., Litzenburger, U. M., Wei, Y., Schep, A. N., LaGory, E. L., Choudhry, H., et al. (2018a). Joint Single-Cell DNA Accessibility and Protein Epitope Profiling Reveals Environmental Regulation of Epigenomic Heterogeneity. Nat. Commun. 9 (1), 4590. doi:10.1038/s41467-018-07115-y
Chen, X., Miragaia, R. J., Natarajan, K. N., and Teichmann, S. A. (2018b). A Rapid and Robust Method for Single Cell Chromatin Accessibility Profiling. Nat. Commun. 9 (1), 5345. doi:10.1038/s41467-018-07771-0
Clark, S. J., Smallwood, S. A., Lee, H. J., Krueger, F., Reik, W., and Kelsey, G. (2017). Genome-wide Base-Resolution Mapping of DNA Methylation in Single Cells Using Single-Cell Bisulfite Sequencing (scBS-Seq). Nat. Protoc. 12 (3), 534–547. doi:10.1038/nprot.2016.187
Claussnitzer, M., Cho, J. H., Collins, R., Cox, N. J., Dermitzakis, E. T., Hurles, M. E., et al. (2020). A Brief History of Human Disease Genetics. Nature 577 (7789), 179–189. doi:10.1038/s41586-019-1879-7
Consortium, Encode Project (2012). An Integrated Encyclopedia of DNA Elements in the Human Genome. Nature 489 (7414), 57–74. doi:10.1038/nature11247
Corces, M. R., Buenrostro, J. D., Wu, B., Greenside, P. G., Chan, S. M., Koenig, J. L., et al. (2016). Lineage-specific and Single-Cell Chromatin Accessibility Charts Human Hematopoiesis and Leukemia Evolution. Nat. Genet. 48 (10), 1193–1203. doi:10.1038/ng.3646
Crawford, G. E., Davis, S., Gabriel, R., Scacheri, P. C., Halawi, M. R. E., Roland, G., et al. (2006). DNase-Chip: a High-Resolution Method to Identify DNase I Hypersensitive Sites Using Tiled Microarrays. Nat. Methods 3 (7), 503–509. doi:10.1038/nmeth888
Creyghton, M. P., Cheng, A. W., Welstead, G. G., Kooistra, T., Carey, B. W., Steine, E. J., et al. (2010). Histone H3K27ac Separates Active from Poised Enhancers and Predicts Developmental State. Proc. Natl. Acad. Sci. U.S.A. 107 (50), 21931–21936. doi:10.1073/pnas.1016071107
Cui, Y., Zheng, Y., Liu, X., Yan, L., Fan, X., Yong, J., et al. (2019). Single-Cell Transcriptome Analysis Maps the Developmental Track of the Human Heart. Cell. Rep. 26 (7), 1934–1950.e5. doi:10.1016/j.celrep.2019.01.079
Cusanovich, D. A., Daza, R., Adey, A., Pliner, H. A., Christiansen, L., Gunderson, K. L., et al. (2015). Multiplex Single-Cell Profiling of Chromatin Accessibility by Combinatorial Cellular Indexing. Science 348 (6237), 910–914. doi:10.1126/science.aab1601
Cusanovich, D. A., Hill, A. J., Aghamirzaie, D., Daza, R. M., Pliner, H. A., Berletch, J. B., et al. (2018a). A Single-Cell Atlas of In Vivo Mammalian Chromatin Accessibility. Cell. 174 (5), 1309–1324 e18. doi:10.1016/j.cell.2018.06.052
Cusanovich, D. A., Reddington, J. P., Garfield, D. A., Daza, R. M., Aghamirzaie, D., Marco-Ferreres, R., et al. (2018b). The Cis-Regulatory Dynamics of Embryonic Development at Single-Cell Resolution. Nature 555 (7697), 538–542. doi:10.1038/nature25981
Cyganek, L., Tiburcy, M., Sekeres, K., Gerstenberg, K., Bohnenberger, H., Lenz, C., et al. (2018). Deep Phenotyping of Human Induced Pluripotent Stem Cell-Derived Atrial and Ventricular Cardiomyocytes. JCI Insight 3 (12), 99941. doi:10.1172/jci.insight.99941
de Soysa, T. Y., Ranade, S. S., Okawa, S., Ravichandran, S., Huang, Y., Salunga, H. T., et al. (2019). Single-cell Analysis of Cardiogenesis Reveals Basis for Organ-Level Developmental Defects. Nature 572 (7767), 120–124. doi:10.1038/s41586-019-1414-x
DeLaughter, D. M., Bick, A. G., Wakimoto, H., McKean, D., Gorham, J. M., Kathiriya, I. S., et al. (2016). Single-Cell Resolution of Temporal Gene Expression during Heart Development. Dev. Cell. 39 (4), 480–490. doi:10.1016/j.devcel.2016.10.001
Demetci, P., Santorella, R., Sandstede, B., Noble, W. S., and Singh, R. (2022). SCOT: Single-Cell Multi-Omics Alignment with Optimal Transport. J. Comput. Biol. 29 (1), 3–18. doi:10.1089/cmb.2021.0446
Depuydt, M. A. C., Prange, K. H. M., Slenders, L., Örd, T., Elbersen, D., Boltjes, A., et al. (2020). Microanatomy of the Human Atherosclerotic Plaque by Single-Cell Transcriptomics. Circ. Res. 127 (11), 1437–1455. doi:10.1161/CIRCRESAHA.120.316770
Di, L., Fu, Y., Sun, Y., Li, J., Liu, L., Yao, J., et al. (2020). RNA Sequencing by Direct Tagmentation of RNA/DNA Hybrids. Proc. Natl. Acad. Sci. U.S.A. 117 (6), 2886–2893. doi:10.1073/pnas.1919800117
Domcke, S., Hill, A. J., Daza, R. M., Cao, J., O’Day, D. R., Pliner, H. A., et al. (2020). A Human Cell Atlas of Fetal Chromatin Accessibility. Science 370 (6518). doi:10.1126/science.aba7612
Esfandyari, D., Idrissou, B. M. G., Hennis, K., Avramopoulos, P., Dueck, A., El-Battrawy, I., et al. (2022). MicroRNA-365 Regulates Human Cardiac Action Potential Duration. Nat. Commun. 13 (1), 220. doi:10.1038/s41467-021-27856-7
Fan, H. C., Fu, G. K., and Fodor, S. P. A. 2015. "Combinatorial Labeling of Single Cells for Gene Expression Cytometry." Science 347 (6222):1258367. doi:10.1126/science.1258367
Farrell, J. A., Wang, Y., Riesenfeld, S. J., Shekhar, K., Regev, A., and Schier, A. F. 2018. "Single-cell Reconstruction of Developmental Trajectories during Zebrafish Embryogenesis." Science 360 (6392):eaar3131. doi:10.1126/science.aar3131
Gambardella, L., McManus, S. A., Moignard, V., Sebukhan, D., Delaune, A., Andrews, S., et al. (2019). BNC1 Regulates Cell Heterogeneity in Human Pluripotent Stem Cell Derived-Epicardium. Development 146 (24). doi:10.1242/dev.174441
Gopalan, S., Wang, Y., Harper, N. W., Garber, M., and Fazzio, T. G. (2021). Simultaneous Profiling of Multiple Chromatin Proteins in the Same Cells. Mol. Cell. 81 (22), 4736–4746 e5. doi:10.1016/j.molcel.2021.09.019
Grosselin, K., Durand, A., Marsolier, J., Poitou, A., Marangoni, E., Nemati, F., et al. (2019). High-throughput Single-Cell ChIP-Seq Identifies Heterogeneity of Chromatin States in Breast Cancer. Nat. Genet. 51 (6), 1060–1066. doi:10.1038/s41588-019-0424-9
Guo, G.-r., Chen, L., Rao, M., Chen, K., Song, J.-p., and Hu, S.-s. (2018). A Modified Method for Isolation of Human Cardiomyocytes to Model Cardiac Diseases. J. Transl. Med. 16 (1), 288. doi:10.1186/s12967-018-1649-6
Guo, H., Tian, L., Zhang, J. Z., Kitani, T., Paik, D. T., Lee, W. H., et al. (2019). Single-Cell RNA Sequencing of Human Embryonic Stem Cell Differentiation Delineates Adverse Effects of Nicotine on Embryonic Development. Stem Cell. Rep. 12 (4), 772–786. doi:10.1016/j.stemcr.2019.01.022
Guo, H., Zhu, P., Guo, F., Li, X., Wu, X., Fan, X., et al. (2015). Profiling DNA Methylome Landscapes of Mammalian Cells with Single-Cell Reduced-Representation Bisulfite Sequencing. Nat. Protoc. 10 (5), 645–659. doi:10.1038/nprot.2015.039
Guo, H., Zhu, P., Wu, X., Li, X., Wen, L., and Tang, F. (2013). Single-cell Methylome Landscapes of Mouse Embryonic Stem Cells and Early Embryos Analyzed Using Reduced Representation Bisulfite Sequencing. Genome Res. 23 (12), 2126–2135. doi:10.1101/gr.161679.113
Han, X., Wang, R., Zhou, Y., Fei, L., Sun, H., Lai, S., et al. (2018). Mapping the Mouse Cell Atlas by Microwell-Seq. Cell. 172 (5), 1091–1107 e17. doi:10.1016/j.cell.2018.02.001
Han, X., Zhou, Z., Fei, L., Sun, H., Wang, R., Chen, Y., et al. (2020). Construction of a Human Cell Landscape at Single-Cell Level. Nature 581 (7808), 303–309. doi:10.1038/s41586-020-2157-4
Harada, A., Maehara, K., Handa, T., Arimura, Y., Nogami, J., Hayashi-Takanaka, Y., et al. (2019). A Chromatin Integration Labelling Method Enables Epigenomic Profiling with Lower Input. Nat. Cell. Biol. 21 (2), 287–296. doi:10.1038/s41556-018-0248-3
Hashimshony, T., Wagner, F., Sher, N., and Yanai, I. (2012). CEL-seq: Single-Cell RNA-Seq by Multiplexed Linear Amplification. Cell. Rep. 2 (3), 666–673. doi:10.1016/j.celrep.2012.08.003
Hesselberth, J. R., Chen, X., Zhang, Z., Sabo, P. J., Sandstrom, R., Reynolds, A. P., et al. (2009). Global Mapping of Protein-DNA Interactions In Vivo by Digital Genomic Footprinting. Nat. Methods 6 (4), 283–289. doi:10.1038/nmeth.1313
Hie, B., Bryson, B., and Berger, B. (2019). Efficient Integration of Heterogeneous Single-Cell Transcriptomes Using Scanorama. Nat. Biotechnol. 37 (6), 685–691. doi:10.1038/s41587-019-0113-3
Hocker, J. D., Poirion, O. B., Zhu, F., Buchanan, J., Zhang, K., Chiou, J., et al. (2021). Cardiac Cell Type-specific Gene Regulatory Programs and Disease Risk Association. Sci. Adv. 7 (20). doi:10.1126/sciadv.abf1444
Hou, Y., Guo, H., Cao, C., Li, X., Hu, B., Zhu, P., et al. (2016). Single-cell Triple Omics Sequencing Reveals Genetic, Epigenetic, and Transcriptomic Heterogeneity in Hepatocellular Carcinomas. Cell. Res. 26 (3), 304–319. doi:10.1038/cr.2016.23
Hu, Y., Huang, K., An, Q., Du, G., Hu, G., Xue, J., et al. (2016). Simultaneous Profiling of Transcriptome and DNA Methylome from a Single Cell. Genome Biol. 17, 88. doi:10.1186/s13059-016-0950-z
Hulin, A., Hortells, L., Gomez-Stallons, M. V., O'Donnell, A., Chetal, K., Adam, M., et al. (2019). Maturation of Heart Valve Cell Populations during Postnatal Remodeling. Development 146 (12). doi:10.1242/dev.173047
Islam, S., Kjällquist, U., Moliner, A., Zajac, P., Fan, J.-B., Lönnerberg, P., et al. (2011). Characterization of the Single-Cell Transcriptional Landscape by Highly Multiplex RNA-Seq. Genome Res. 21 (7), 1160–1167. doi:10.1101/gr.110882.110
Jin, S., Zhang, L., and Nie, Q. (2020). scAI: an Unsupervised Approach for the Integrative Analysis of Parallel Single-Cell Transcriptomic and Epigenomic Profiles. Genome Biol. 21 (1), 25. doi:10.1186/s13059-020-1932-8
Jin, W., Tang, Q., Wan, M., Cui, K., Zhang, Y., Ren, G., et al. (2015). Genome-wide Detection of DNase I Hypersensitive Sites in Single Cells and FFPE Tissue Samples. Nature 528 (7580), 142–146. doi:10.1038/nature15740
Jones, P. A. (2012). Functions of DNA Methylation: Islands, Start Sites, Gene Bodies and beyond. Nat. Rev. Genet. 13 (7), 484–492. doi:10.1038/nrg3230
Kathiriya, I. S., Rao, K. S., Iacono, G., Devine, W. P., Blair, A. P., Hota, S. K., et al. (2021). Modeling Human TBX5 Haploinsufficiency Predicts Regulatory Networks for Congenital Heart Disease. Dev. Cell. 56 (3), 292–309 e9. doi:10.1016/j.devcel.2020.11.020
Kaya-Okur, H. S., Wu, S. J., Codomo, C. A., Pledger, E. S., Bryson, T. D., Henikoff, J. G., et al. (2019). CUT&Tag for Efficient Epigenomic Profiling of Small Samples and Single Cells. Nat. Commun. 10 (1), 1930. doi:10.1038/s41467-019-09982-5
Klein, A. M., Mazutis, L., Akartuna, I., Tallapragada, N., Veres, A., Li, V., et al. (2015). Droplet Barcoding for Single-Cell Transcriptomics Applied to Embryonic Stem Cells. Cell. 161 (5), 1187–1201. doi:10.1016/j.cell.2015.04.044
Korsunsky, I., Millard, N., Fan, J., Slowikowski, K., Zhang, F., Wei, K., et al. (2019). Fast, Sensitive and Accurate Integration of Single-Cell Data with Harmony. Nat. Methods 16 (12), 1289–1296. doi:10.1038/s41592-019-0619-0
Laird, P. W. (2010). Principles and Challenges of Genome-wide DNA Methylation Analysis. Nat. Rev. Genet. 11 (3), 191–203. doi:10.1038/nrg2732
Lareau, C. A., Duarte, F. M., Chew, J. G., Kartha, V. K., Burkett, Z. D., Kohlway, A. S., et al. (2019). Droplet-based Combinatorial Indexing for Massive-Scale Single-Cell Chromatin Accessibility. Nat. Biotechnol. 37 (8), 916–924. doi:10.1038/s41587-019-0147-6
Li, L., Dong, J., Yan, L., Yong, J., Liu, X., Hu, Y., et al. (2017). Single-Cell RNA-Seq Analysis Maps Development of Human Germline Cells and Gonadal Niche Interactions. Cell. Stem Cell. 20 (6), 858–873 e4. doi:10.1016/j.stem.2017.03.007
Li, L., Guo, F., Gao, Y., Ren, Y., Yuan, P., Yan, L., et al. (2018). Single-cell Multi-Omics Sequencing of Human Early Embryos. Nat. Cell. Biol. 20 (7), 847–858. doi:10.1038/s41556-018-0123-2
Li, Y., Ren, P., Dawson, A., Vasquez, H. G., Ageedi, W., Zhang, C., et al. (2020). Single-Cell Transcriptome Analysis Reveals Dynamic Cell Populations and Differential Gene Expression Patterns in Control and Aneurysmal Human Aortic Tissue. Circulation 142 (14), 1374–1388. doi:10.1161/CIRCULATIONAHA.120.046528
Litviňuková, M., Talavera-López, C., Maatz, H., Reichart, D., Worth, C. L., Lindberg, E. L., et al. (2020). Cells of the Adult Human Heart. Nature 588 (7838), 466–472. doi:10.1038/s41586-020-2797-4
Liu, J., Huang, Y., Singh, R., Vert, J. P., and Noble, W. S. (2019). Jointly Embedding Multiple Single-Cell Omics Measurements. Algorithms Bioinform 143. doi:10.4230/LIPIcs.WABI.2019.10
Liu, L., Liu, C., Quintero, A., Wu, L., Yuan, Y., Wang, M., et al. (2019). Deconvolution of Single-Cell Multi-Omics Layers Reveals Regulatory Heterogeneity. Nat. Commun. 10 (1), 470. doi:10.1038/s41467-018-08205-7
Liu, X., Wang, C., Liu, W., Li, J., Li, C., Kou, X., et al. (2016). Distinct Features of H3K4me3 and H3K27me3 Chromatin Domains in Pre-implantation Embryos. Nature 537 (7621), 558–562. doi:10.1038/nature19362
Liu, Z., Wang, L., Welch, J. D., Ma, H., Zhou, Y., Vaseghi, H. R., et al. (2017). Single-cell Transcriptomics Reconstructs Fate Conversion from Fibroblast to Cardiomyocyte. Nature 551 (7678), 100–104. doi:10.1038/nature24454
Local, A., Huang, H., Albuquerque, C. P., Singh, N., Lee, A. Y., Wang, W., et al. (2018). Identification of H3K4me1-Associated Proteins at Mammalian Enhancers. Nat. Genet. 50 (1), 73–82. doi:10.1038/s41588-017-0015-6
Lopez, R., Regier, J., Cole, M. B., Jordan, M. I., and Yosef, N. (2018). Deep Generative Modeling for Single-Cell Transcriptomics. Nat. Methods 15 (12), 1053–1058. doi:10.1038/s41592-018-0229-2
Lotfollahi, M., Wolf, F. A., and Theis, F. J. (2019). scGen Predicts Single-Cell Perturbation Responses. Nat. Methods 16 (8), 715–721. doi:10.1038/s41592-019-0494-8
Lu, B., Dong, L., Yi, D., Zhang, M., Zhu, C., Li, X., et al. (2020). Transposase-assisted Tagmentation of RNA/DNA Hybrid Duplexes. Elife 9. doi:10.7554/eLife.54919
Luecken, M. D., Büttner, M., Chaichoompu, K., Danese, A., Interlandi, M., Mueller, M. F., et al. (2022). Benchmarking Atlas-Level Data Integration in Single-Cell Genomics. Nat. Methods 19 (1), 41–50. doi:10.1038/s41592-021-01336-8
Ma, A., McDermaid, A., Xu, J., Chang, Y., and Ma, Q. (2020). Integrative Methods and Practical Challenges for Single-Cell Multi-Omics. Trends Biotechnol. 38 (9), 1007–1022. doi:10.1016/j.tibtech.2020.02.013
Ma, S., Zhang, B., LaFave, L. M., Earl, A. S., Chiang, Z., Hu, Y., et al. (2020). Chromatin Potential Identified by Shared Single-Cell Profiling of RNA and Chromatin. Cell. 183 (4), 1103–1116 e20. doi:10.1016/j.cell.2020.09.056
Macosko, E. Z., Basu, A., Satija, R., Nemesh, J., Shekhar, K., Goldman, M., et al. (2015). Highly Parallel Genome-wide Expression Profiling of Individual Cells Using Nanoliter Droplets. Cell. 161 (5), 1202–1214. doi:10.1016/j.cell.2015.05.002
Martini, E., Kunderfranco, P., Peano, C., Carullo, P., Cremonesi, M., Schorn, T., et al. (2019). Single-Cell Sequencing of Mouse Heart Immune Infiltrate in Pressure Overload-Driven Heart Failure Reveals Extent of Immune Activation. Circulation 140 (25), 2089–2107. doi:10.1161/CIRCULATIONAHA.119.041694
Meers, Michael. P., Janssens, Derek. H., and Henikoff, Steven. (20212021). Multifactorial Chromatin Regulatory Landscapes at Single Cell Resolution. bioRxiv, 451691. 07.08. doi:10.1101/2021.07.08.451691
Meissner, A., Gnirke, A., Bell, G. W., Ramsahoye, B., Lander, E. S., and Jaenisch, R. (2005). Reduced Representation Bisulfite Sequencing for Comparative High-Resolution DNA Methylation Analysis. Nucleic Acids Res. 33 (18), 5868–5877. doi:10.1093/nar/gki901
Mezger, A., Klemm, S., Mann, I., Brower, K., Mir, A., Bostick, M., et al. (2018). High-throughput Chromatin Accessibility Profiling at Single-Cell Resolution. Nat. Commun. 9 (1), 3647. doi:10.1038/s41467-018-05887-x
Miao, Y., Tian, L., Martin, M., Paige, S. L., Galdos, F. X., Li, J., et al. (2020). Intrinsic Endocardial Defects Contribute to Hypoplastic Left Heart Syndrome. Cell. Stem Cell. 27 (4), 574–589 e8. doi:10.1016/j.stem.2020.07.015
Miao, Z., Humphreys, B. D., McMahon, A. P., and Kim, J. (2021). Multi-omics Integration in the Age of Million Single-Cell Data. Nat. Rev. Nephrol. 17, 710–724. doi:10.1038/s41581-021-00463-x
Mulqueen, R. M., Pokholok, D., Norberg, S. J., Torkenczy, K. A., Fields, A. J., Sun, D., et al. (2018). Highly Scalable Generation of DNA Methylation Profiles in Single Cells. Nat. Biotechnol. 36 (5), 428–431. doi:10.1038/nbt.4112
Musselman, C. A., Gibson, M. D., Hartwick, E. W., North, J. A., Gatchalian, J., Poirier, M. G., et al. (2013). Binding of PHF1 Tudor to H3K36me3 Enhances Nucleosome Accessibility. Nat. Commun. 4, 2969. doi:10.1038/ncomms3969
Nakato, R., Wada, Y., Nakaki, R., Nagae, G., Katou, Y., Tsutsumi, S., et al. (2019). Comprehensive Epigenome Characterization Reveals Diverse Transcriptional Regulation across Human Vascular Endothelial Cells. Epigenetics Chromatin 12 (1), 77. doi:10.1186/s13072-019-0319-0
Nicetto, D., and Zaret, K. S. (2019). Role of H3K9me3 Heterochromatin in Cell Identity Establishment and Maintenance. Curr. Opin. Genet. Dev. 55, 1–10. doi:10.1016/j.gde.2019.04.013
Nicin, L., Abplanalp, W. T., Mellentin, H., Kattih, B., Tombor, L., John, D., et al. (2020). Cell Type-specific Expression of the Putative SARS-CoV-2 Receptor ACE2 in Human Hearts. Eur. Heart J. 41 (19), 1804–1806. doi:10.1093/eurheartj/ehaa311
Nicin, L., Schroeter, S. M., Glaser, S. F., Schulze-Brüning, R., Pham, M.-D., Hille, S. S., et al. Minh-Duc Pham (2022). Hille, Michail Yekelchyk, Badder Kattih, Wesley Tyler Abplanalp, Lukas Tombor, Oliver J. Müller, Thomas Braun, Benjamin Meder, Christoph Reich, Mani Arsalan, Tomas Holubec, Thomas Walther, Fabian Emrich, Jaya Krishnan, Andreas M. Zeiher, David John, and Stefanie DimmelerA Human Cell Atlas of the Pressure-Induced Hypertrophic Heart. Nat. Cardiovasc Res. 1 (2), 174–185. doi:10.1038/s44161-022-00019-7
Nomura, S., Satoh, M., Fujita, T., Higo, T., Sumida, T., Ko, T., et al. (2018). Cardiomyocyte Gene Programs Encoding Morphological and Functional Signatures in Cardiac Hypertrophy and Failure. Nat. Commun. 9 (1), 4435. doi:10.1038/s41467-018-06639-7
Örd, T., Õunap, K., Stolze, L. K., Aherrahrou, R., Nurminen, V., Toropainen, A., et al. (2021). Single-Cell Epigenomics and Functional Fine-Mapping of Atherosclerosis GWAS Loci. Circ. Res. 129 (2), 240–258. doi:10.1161/CIRCRESAHA.121.318971
Park, P. J. (2009). ChIP-seq: Advantages and Challenges of a Maturing Technology. Nat. Rev. Genet. 10 (10), 669–680. doi:10.1038/nrg2641
Pekowska, A., Benoukraf, T., Ferrier, P., and Spicuglia, S. (2010). A Unique H3K4me2 Profile Marks Tissue-specific Gene Regulation. Genome Res. 20 (11), 1493–1502. doi:10.1101/gr.109389.110
Picelli, S., Faridani, O. R., Björklund, Å. K., Winberg, G., Sagasser, S., and Sandberg, R. (2014). Full-length RNA-Seq from Single Cells Using Smart-Seq2. Nat. Protoc. 9 (1), 171–181. doi:10.1038/nprot.2014.006
Pliner, H. A., Packer, J. S., McFaline-Figueroa, J. L., Cusanovich, D. A., Daza, R. M., Aghamirzaie, D., et al. (2018). Cicero Predicts Cis-Regulatory DNA Interactions from Single-Cell Chromatin Accessibility Data. Mol. Cell. 71 (5), 858–871 e8. doi:10.1016/j.molcel.2018.06.044
Pott, S. (2017). Simultaneous Measurement of Chromatin Accessibility, DNA Methylation, and Nucleosome Phasing in Single Cells. Elife 6. doi:10.7554/eLife.23203
Ramani, V., Deng, X., Qiu, R., Gunderson, K. L., Steemers, F. J., Disteche, C. M., et al. (2017). Massively Multiplex Single-Cell Hi-C. Nat. Methods 14 (3), 263–266. doi:10.1038/nmeth.4155
Rao, M., Wang, X., Guo, G., Wang, L., Chen, S., Yin, P., et al. (2021). Resolving the Intertwining of Inflammation and Fibrosis in Human Heart Failure at Single-Cell Level. Basic Res. Cardiol. 116 (1), 55. doi:10.1007/s00395-021-00897-1
Reyes-Palomares, A., Gu, M., Grubert, F., Berest, I., Sa, S., Kasowski, M., et al. (2020). Remodeling of Active Endothelial Enhancers Is Associated with Aberrant Gene-Regulatory Networks in Pulmonary Arterial Hypertension. Nat. Commun. 11 (1), 1673. doi:10.1038/s41467-020-15463-x
Rosenberg, A. B., Roco, C. M., Muscat, R. A., Kuchina, A., Sample, P., Yao, Z., et al. 2018. "Single-cell Profiling of the Developing Mouse Brain and Spinal Cord with Split-Pool Barcoding." Science 360 (6385):176–182. doi:10.1126/science.aam8999
Rotem, A., Ram, O., Shoresh, N., Sperling, R. A., Goren, A., Weitz, D. A., et al. (2015). Single-cell ChIP-Seq Reveals Cell Subpopulations Defined by Chromatin State. Nat. Biotechnol. 33 (11), 1165–1172. doi:10.1038/nbt.3383
Ruan, H., Liao, Y., Ren, Z., Mao, L., Yao, F., Yu, P., et al. (2019). Single-cell Reconstruction of Differentiation Trajectory Reveals a Critical Role of ETS1 in Human Cardiac Lineage Commitment. BMC Biol. 17 (1), 89. doi:10.1186/s12915-019-0709-6
Sabo, P. J., Kuehn, M. S., Thurman, R., Johnson, B. E., Johnson, E. M., Johnson, E. M., et al. (2006). Genome-scale Mapping of DNase I Sensitivity In Vivo Using Tiling DNA Microarrays. Nat. Methods 3 (7), 511–518. doi:10.1038/nmeth890
Sahara, M., Santoro, F., Sohlmér, J., Zhou, C., Witman, N., Leung, C. Y., et al. (2019). Population and Single-Cell Analysis of Human Cardiogenesis Reveals Unique LGR5 Ventricular Progenitors in Embryonic Outflow Tract. Dev. Cell. 48 (4), 475–490. e7. doi:10.1016/j.devcel.2019.01.005
Satpathy, A. T., Granja, J. M., Yost, K. E., Qi, Y., Meschi, F., McDermott, G. P., et al. (2019). Massively Parallel Single-Cell Chromatin Landscapes of Human Immune Cell Development and Intratumoral T Cell Exhaustion. Nat. Biotechnol. 37 (8), 925–936. doi:10.1038/s41587-019-0206-z
Schmid, C., Abi-Gerges, N., Leitner, M., Zellner, D., and Rast, G. (2021). Ion Channel Expression and Electrophysiology of Singular Human (Primary and Induced Pluripotent Stem Cell-Derived) Cardiomyocytes. Cells 10 (12), 3370. doi:10.3390/cells10123370
Schones, D. E., Cui, K., Cuddapah, S., Roh, T.-Y., Barski, A., Wang, Z., et al. (2008). Dynamic Regulation of Nucleosome Positioning in the Human Genome. Cell. 132 (5), 887–898. doi:10.1016/j.cell.2008.02.022
See, K., See, W. L. W., Lim, E. H., Tiang, Z., Lee, L. T., Li, P. Y. Q., et al. (2017). Single Cardiomyocyte Nuclear Transcriptomes Reveal a lincRNA-Regulated De-differentiation and Cell Cycle Stress-Response In Vivo. Nat. Commun. 8 (1), 225. doi:10.1038/s41467-017-00319-8
Shen, Y., Yue, F., McCleary, D. F., Ye, Z., Edsall, L., Kuan, S., et al. (2012). A Map of the Cis-Regulatory Sequences in the Mouse Genome. Nature 488 (7409), 116–120. doi:10.1038/nature11243
Smallwood, S. A., Lee, H. J., Angermueller, C., Krueger, F., Saadeh, H., Peat, J., et al. (2014). Single-cell Genome-wide Bisulfite Sequencing for Assessing Epigenetic Heterogeneity. Nat. Methods 11 (8), 817–820. doi:10.1038/nmeth.3035
Srivatsan, S. R., McFaline-Figueroa, J. L., Ramani, V., Saunders, L., Cao, J., Packer, J., et al. 2020. Massively Multiplex Chemical Transcriptomics at Single-Cell Resolution. Science 367 (6473):45–51. doi:10.1126/science.aax6234
Stuart, T., Butler, A., Hoffman, P., Hafemeister, C., Papalexi, E., Mauck, W. M., et al. (2019). Comprehensive Integration of Single-Cell Data. Cell. 177 (7), 1888–1902 e21. doi:10.1016/j.cell.2019.05.031
Sun, Q., Crowley, C. A., Huang, L., Wen, J., Chen, J., Bao, E. L., et al. (2022). From GWAS Variant to Function: A Study of ∼148,000 Variants for Blood Cell Traits. Hum. Genet. Genomics Adv. 3 (1), 100063. doi:10.1016/j.xhgg.2021.100063
Suryawanshi, H., Clancy, R., Morozov, P., Halushka, M. K., Buyon, J. P., and Tuschl, T. (2020). Cell Atlas of the Foetal Human Heart and Implications for Autoimmune-Mediated Congenital Heart Block. Cardiovasc Res. 116 (8), 1446–1457. doi:10.1093/cvr/cvz257
Tang, F., Barbacioru, C., Wang, Y., Nordman, E., Lee, C., Xu, N., et al. (2009). mRNA-Seq Whole-Transcriptome Analysis of a Single Cell. Nat. Methods 6 (5), 377–382. doi:10.1038/nmeth.1315
Thurman, R. E., Rynes, E., Humbert, R., Vierstra, J., Maurano, M. T., Haugen, E., et al. (2012). The Accessible Chromatin Landscape of the Human Genome. Nature 489 (7414), 75–82. doi:10.1038/nature11232
Tran, H. T. N., Ang, K. S., Chevrier, M., Zhang, X., Lee, N. Y. S., Goh, M., et al. (2020). A Benchmark of Batch-Effect Correction Methods for Single-Cell RNA Sequencing Data. Genome Biol. 21 (1), 12. doi:10.1186/s13059-019-1850-9
Tucker, N. R., Chaffin, M., Fleming, S. J., Hall, A. W., Parsons, V. A., Bedi, K. C., et al. (2020). Transcriptional and Cellular Diversity of the Human Heart. Circulation 142 (5), 466–482. doi:10.1161/CIRCULATIONAHA.119.045401
Valouev, A., Johnson, S. M., Boyd, S. D., Smith, C. L., Fire, A. Z., and Sidow, A. (2011). Determinants of Nucleosome Organization in Primary Human Cells. Nature 474 (7352), 516–520. doi:10.1038/nature10002
Vitak, S. A., Torkenczy, K. A., Rosenkrantz, J. L., Fields, A. J., Christiansen, L., Wong, M. H., et al. (2017). Sequencing Thousands of Single-Cell Genomes with Combinatorial Indexing. Nat. Methods 14 (3), 302–308. doi:10.1038/nmeth.4154
Wagner, D. E., Weinreb, C., Briggs, J. A., Megason, Sean. G., Collins, Z. M., Briggs, J. A., et al. (2018). Single-cell Mapping of Gene Expression Landscapes and Lineage in the Zebrafish Embryo. Science 360 (6392), 981–987. doi:10.1126/science.aar4362
Wang, C., Sun, D., Huang, X., Wan, C., Li, Z., Han, Y., et al. (2020). Integrative Analyses of Single-Cell Transcriptome and Regulome Using MAESTRO. Genome Biol. 21 (1), 198. doi:10.1186/s13059-020-02116-x
Wang, Z., Cui, M., Shah, A. M., Tan, W., Liu, N., Bassel-Duby, R., et al. (2020). Cell-Type-Specific Gene Regulatory Networks Underlying Murine Neonatal Heart Regeneration at Single-Cell Resolution. Cell. Rep. 33 (10), 108472. doi:10.1016/j.celrep.2020.108472
Welch, J. D., Kozareva, V., Ferreira, A., Vanderburg, C., Martin, C., and Macosko, E. Z. (2019). Single-Cell Multi-Omic Integration Compares and Contrasts Features of Brain Cell Identity. Cell. 177 (7), 1873–1887. doi:10.1016/j.cell.2019.05.006
Wen, L., and Tang, F. (2016). Single-cell Sequencing in Stem Cell Biology. Genome Biol. 17, 71. doi:10.1186/s13059-016-0941-0
Wu, A. R., Neff, N. F., Kalisky, T., Dalerba, P., Treutlein, B., Rothenberg, M. E., et al. (2014). Quantitative Assessment of Single-Cell RNA-Sequencing Methods. Nat. Methods 11 (1), 41–46. doi:10.1038/nmeth.2694
Xiong, H., Luo, Y., Wang, Q., Yu, X., and He, A. (2021). Single-cell Joint Detection of Chromatin Occupancy and Transcriptome Enables Higher-Dimensional Epigenomic Reconstructions. Nat. Methods 18 (6), 652–660. doi:10.1038/s41592-021-01129-z
Xu, C., Lopez, R., Mehlman, E., Regier, J., Jordan, M. I., and Yosef, N. (2021). Probabilistic Harmonization and Annotation of Single‐cell Transcriptomics Data with Deep Generative Models. Mol. Syst. Biol. 17 (1), e9620. doi:10.15252/msb.20209620
Xu, K., Xie, S., Huang, Y., Zhou, T., Liu, M., Zhu, P., et al. (2020). Cell-Type Transcriptome Atlas of Human Aortic Valves Reveal Cell Heterogeneity and Endothelial to Mesenchymal Transition Involved in Calcific Aortic Valve Disease. Atvb 40 (12), 2910–2921. doi:10.1161/ATVBAHA.120.314789
Zhang, H., Tian, L., Shen, M., Tu, C., Wu, H., Gu, M., et al. (2019). Generation of Quiescent Cardiac Fibroblasts from Human Induced Pluripotent Stem Cells for In Vitro Modeling of Cardiac Fibrosis. Circ. Res. 125 (5), 552–566. doi:10.1161/CIRCRESAHA.119.315491
Zhang, K., Hocker, J. D., Miller, M., Hou, X., Chiou, J., Poirion, O. B., et al. (2021). A Single-Cell Atlas of Chromatin Accessibility in the Human Genome. Cell. 184 (24), 5985–6001 e19. doi:10.1016/j.cell.2021.10.024
Zhang, X., Li, T., Liu, F., Chen, Y., Yao, J., Li, Z., et al. (2019). Comparative Analysis of Droplet-Based Ultra-high-throughput Single-Cell RNA-Seq Systems. Mol. Cell. 73 (1), 130–142 e5. doi:10.1016/j.molcel.2018.10.020
Zheng, G. X. Y., Terry, J. M., Belgrader, P., Ryvkin, P., Bent, Z. W., Wilson, R., et al. (2017). Massively Parallel Digital Transcriptional Profiling of Single Cells. Nat. Commun. 8, 14049. doi:10.1038/ncomms14049
Zhou, Y., Liu, Z., Welch, J. D., Gao, X., Wang, L., Garbutt, T., et al. (2019). Single-Cell Transcriptomic Analyses of Cell Fate Transitions during Human Cardiac Reprogramming. Cell. Stem Cell. 25 (1), 149–164 e9. doi:10.1016/j.stem.2019.05.020
Zhu, C., Yu, M., Huang, H., Juric, I., Abnousi, A., Hu, R., et al. (2019). An Ultra High-Throughput Method for Single-Cell Joint Analysis of Open Chromatin and Transcriptome. Nat. Struct. Mol. Biol. 26 (11), 1063–1070. doi:10.1038/s41594-019-0323-x
Zhu, C., Zhang, Y., Li, Y. E., Lucero, J., Behrens, M. M., and Ren, B. (2021). Joint Profiling of Histone Modifications and Transcriptome in Single Cells from Mouse Brain. Nat. Methods 18 (3), 283–292. doi:10.1038/s41592-021-01060-3
Zilionis, R., Nainys, J., Veres, A., Savova, V., Zemmour, D., Klein, A. M., et al. (2017). Single-cell Barcoding and Sequencing Using Droplet Microfluidics. Nat. Protoc. 12 (1), 44–73. doi:10.1038/nprot.2016.154
Keywords: single-cell technology, multi-omics, epigenomic, integrative analysis, cardiovascular system
Citation: Zhang X, Qiu H, Zhang F and Ding S (2022) Advances in Single-Cell Multi-Omics and Application in Cardiovascular Research. Front. Cell Dev. Biol. 10:883861. doi: 10.3389/fcell.2022.883861
Received: 25 February 2022; Accepted: 23 May 2022;
Published: 06 June 2022.
Edited by:
Li Wang, Chinese Academy of Medical Sciences and Peking Union Medical College, ChinaCopyright © 2022 Zhang, Qiu, Zhang and Ding. This is an open-access article distributed under the terms of the Creative Commons Attribution License (CC BY). The use, distribution or reproduction in other forums is permitted, provided the original author(s) and the copyright owner(s) are credited and that the original publication in this journal is cited, in accordance with accepted academic practice. No use, distribution or reproduction is permitted which does not comply with these terms.
*Correspondence: Xingwu Zhang, enh3MTdAbWFpbHMudHNpbmdodWEuZWR1LmNu
†These authors have contributed equally to this work and share first authorship
Disclaimer: All claims expressed in this article are solely those of the authors and do not necessarily represent those of their affiliated organizations, or those of the publisher, the editors and the reviewers. Any product that may be evaluated in this article or claim that may be made by its manufacturer is not guaranteed or endorsed by the publisher.
Research integrity at Frontiers
Learn more about the work of our research integrity team to safeguard the quality of each article we publish.