- 1The First School of Medicine, School of Information and Engineering, The First Affiliated Hospital of Wenzhou Medical University, Wenzhou, China
- 2Department of Anatomy, School of Basic Medicine and Life Science, Hainan Medical University, Haikou, China
Pancreatic adenocarcinoma (PAAD) is the fourth leading cause of cancer-related deaths worldwide. 5-Hydroxymethylcytosine (5hmC)-mediated epigenetic regulation has been reported to be involved in cancer pathobiology and has emerged to be promising biomarkers for cancer diagnosis and prognosis. However, 5hmC alterations at long non-coding RNA (lncRNA) genes and their clinical significance remained unknown. In this study, we performed the genome-wide investigation of lncRNA-associated plasma cfDNA 5hmC changes in PAAD by plotting 5hmC reads against lncRNA genes, and identified six PAAD-specific lncRNAs with abnormal 5hmC modifications compared with healthy individuals. Then we applied machine-learning and Cox regression approaches to develop predictive diagnostic (5hLRS) and prognostic (5hLPS) models using the 5hmC-modified lncRNAs. The 5hLRS demonstrated excellent performance in discriminating PAAD from healthy controls with an area under the curve (AUC) of 0.833 in the training cohort and 0.719 in the independent testing cohort. The 5hLPS also effectively divides PAAD patients into high-risk and low-risk groups with significantly different clinical outcomes in the training cohort (log-rank test p = 0.04) and independent testing cohort (log-rank test p = 0.0035). Functional analysis based on competitive endogenous RNA (ceRNA) and enrichment analysis suggested that these differentially regulated 5hmC modified lncRNAs were associated with angiogenesis, circulatory system process, leukocyte differentiation and metal ion homeostasis that are known important events in the development and progression of PAAD. In conclusion, our study indicated the potential clinical utility of 5hmC profiles at lncRNA loci as valuable biomarkers for non-invasive diagnosis and prognostication of cancers.
Introduction
Pancreatic adenocarcinoma (PAAD) is the fourth leading cause of cancer-related deaths worldwide (Siegel et al., 2020). The lack of early-stage diagnostics has hindered the development of therapeutics that can slow down or reverse PAAD (Moutinho-Ribeiro et al., 2017; Sohal et al., 2017). Carbohydrate antigen 19–9 (CA19-9) is the biomarker currently used for PAAD diagnosis (Poruk et al., 2013). However, CA19-9 has a pooled sensitivity of 75.4% (95% CI: 73.4–77.4%) and a specificity of 77.6% (95% CI: 75.4–79.7%) for differentiation between malignant and non-malignant forms of cancer (Zhang et al., 2015). Moreover, the specificity of distinction between PAAD and CP often does not exceed 60% (Schultz et al., 2014), which has prompted a search for alternative biomarkers.
Circulating cell-free DNA (cfDNA) originates from cell death in different tissues, which has attracted massive interest as a non-invasive biomarker for cancer detection (Wan et al., 2017). Tumor cells release small nucleic acid fragments into the blood via multiple mechanisms, allowing cancer-associated genetic alterations to be detected (Diaz and Bardelli, 2014; Wan et al., 2017). Non-invasive biomarkers offer substantial advantages over tissue biopsy as their easily accessible characteristics make them ideal candidates for cancer diagnosis and progression monitoring (Xu et al., 2017).
5-methylcytosine (5mC) modifications potentially characterized various health conditions (Lehmann-Werman et al., 2016; Guo et al., 2017). Nonetheless, there has been no investigation to identify and sequence alternative modifications in circulating cfDNA because the DNA samples are low-input. 5-Hydroxymethylcytosine (5hmC) is a novel identified epigenetic mark generated from 5mC by the ten-eleven translocation proteins (Tahiliani et al., 2009). Increasing evidence showed that low levels of 5hmC are observed in many tumors frequently compared to corresponding normal tissues (Jin et al., 2011). 5hmC is a stable intermediate of cytosine demethylation. Active gene modification was associated with the levels of 5hmC accumulation in promoters, gene bodies and gene regulatory elements (Han et al., 2016). 5hmC modifications play a curial role in cell development, differentiation, maturation and self-renewal (Wang et al., 2014). These characteristics suggested 5hmC signaling changes in cfDNA may have potential values in cancer diagnosis and progression monitoring using highly robust and sensitive 5hmC sequencing technologies (Song et al., 2017).
Long non-coding RNA (lncRNA) expression is the most pervasive transcriptional change in cancer, which is demonstrated by the recent genome-wide characterization of the human cancer transcriptome (Marchese et al., 2017; Zhou et al., 2019a; Bao et al., 2020; Zhou et al., 2021b). Multiple studies have shown that lncRNAs play a critical role in the mechanism of occurrence, evolution, invasion and metastasis of pancreatic cancer (Fu et al., 2017; Zhang et al., 2020; Tang et al., 2021). Several studies have indicated the impact of lncRNAs on the prognosis of pancreatic cancer (Huang et al., 2016; Zhou et al., 2016). There is increasing evidence that lncRNA expression could also be regulated by epigenetic DNA modifications, such as DNA methylation, histone modifications and 5hmC modification (Guttman et al., 2009; White et al., 2014; Yan et al., 2015). Epigenetic DNA modifications in lncRNA genes have been revealed to be valuable non-invasive biomarkers for cancer diagnosis, prognosis and surveillance (Hu et al., 2017; Zhou et al., 2021a).
In the present study, we performed machine learning-based integrative analysis of 5-hydroxymethylcytosine and transcriptional profiling to identify plasma-derived lncRNAs with PAAD-specific abnormal 5hmC modifications and conducted a two-phase discovery-validation experiment to explore the clinical potential of 5hmC-modified lncRNAs as non-invasive biomarkers for diagnosis and prognosis of pancreatic cancer.
Materials and Methods
Sample Datasets
A total of 130 publicly available genome-wide nano-hmC-Seal profiles from plasma cfDNA samples were used in this study, including 34 PAAD patients and 96 healthy individuals from Sequence Read Archive (SRA, SRP080977) of the National Center for Biotechnology Information (NCBI) (Li et al., 2017). Clinical and transcriptomic data (RNA-Seq, v.2019-07-20) of 182 PAAD cases, including 176 primary tumors and four solid tissue normal, generated by The Cancer Genome Atlas (TCGA) were downloaded from UCSC Xena (https://xena.ucsc.edu/). In addition, stem-loop expression (miRNA, v.2019.7.20) of 183 PAAD cases, including 1881 miRNAs, was also obtained from UCSC Xena. Detailed information on the study population is shown in Table 1. The workflow diagram of the study design is shown in Figure 1
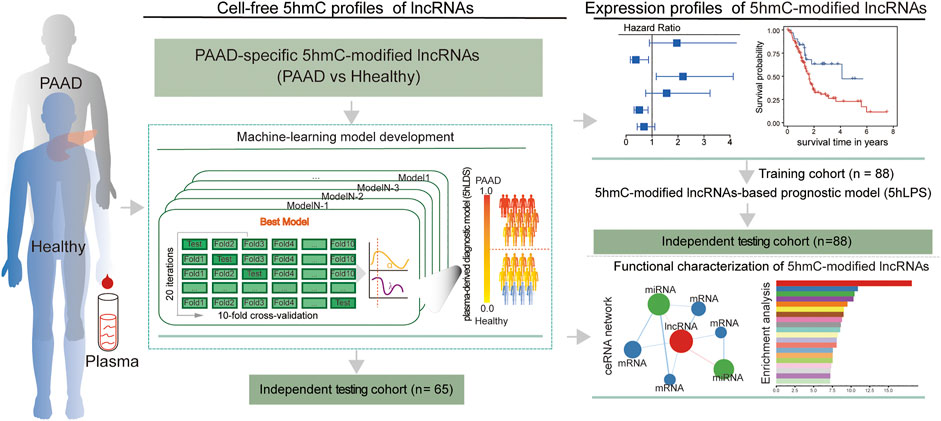
FIGURE 1. Workflow diagram of the study design. A two-phase discovery-validation study was conducted. The 5hmC-modified lncRNA-based predictive and prognostic models for PAAD were developed using the machine-learning methods in the training cohort and validated in the independent testing cohort. PAAD, Pancreatic adenocarcinoma; lncRNA, long non-coding RNAs; 5hmC, 5-Hydroxymethylcytosine.
Data Preprocessing and Mapping of 5hmC-Modified lncRNAs
Read sequences were extracted in FASTQ files using the SRA toolkit (https://trace.ncbi.nlm.nih.gov/Traces/sra/sra.cgi?view=software, version 2.9.2), and then were aligned to the human genome GRCh37 using Bowtie2 (version 2.3.4.2) with default parameters (Langmead and Salzberg, 2012). To convert and sort the alignment SAM files into BAM files, SAMtools (version 1.9) was used to generate the files (Li et al., 2009). The picard-2.18.4 was used to retain unique non-duplicate matches to the genome (http://broadinstitute.github.io/picard/). The released version of the lncRNA reference gene annotation file (GRCh38 version 34) was downloaded from the GENCODE database (https://www.gencodegenes.org/). LiftOver was used to transfer the mapping information from the GRCh38 version of the lncRNA reference gene annotation file to the GRCh37 version. Genes encoding lncRNAs were extracted based on GRCh37 annotation. Read counts of 5hmC-modified lncRNAs were calculated using the fragment counts in each RefSeq lncRNA obtained by BEDtools (version 2.27.1) (Quinlan and Hall, 2010). The read counts were converted into Transcripts Per kilobase of 5hmC in lncRNA per million mapped reads. Finally, 5hmC profiles of 16,827 lncRNAs were obtained for further analysis.
Machining Learning-Based Establishment of a Non-Invasive Diagnostic Model Based on 5hmc Modified lncRNAs
The 5hmC profiles of lncRNAs were compared between PAAD and healthy control samples. The lncRNAs with differential 5hmC modification were identified using the DESeq2 package (version 1.22.2) (Love et al., 2014) with a |log2foldchange|>0.58 and false discovery rate adjusted p < 0.05, and were selected as PAAD-specific 5hmC-modified lncRNAs. Then a 5hmC-modified lncRNA-based risk scoring model (termed 5hLDS) was constructed using the elastic net regularization on a multivariable logistic regression approaches to distinguish between PAAD and healthy individuals. The 5hLDS was trained with 10-fold cross-validation and optimized using a ROC curve for a grid of parameter values for α and λ (α range, 0.05 to 1.00 with a length = 10; λ range: from 10–1 to 5*10–1 with a 0.1 increment), and this selection process was repeated 20 times. Finally, the 5hLDS was established based on PAAD-specific 5hmC-modified lncRNA markers. The 5hLDS range was 0–1.0 and represented a final probability of PAAD for each sample. The 5hLDS was established using the “trainControl” and “train” functions from the Caret (version 6.0–86) R package.
Functional Analysis of PAAD-Specific 5hmC-Modified lncRNA Markers
Pearson correlation coefficient was used to measure the expression relevance among PAAD-specific 5hmC-modified lncRNA markers, miRNAs and mRNAs in the TCGA cohort. Human experimentally validated miRNA-mRNA interactions were downloaded from ENCORI (https://starbase.sysu.edu.cn/index.php), the updated version of the StarBase database providing the most comprehensive network of miRNA-mRNA interactions supported by CLIP-Seq data sets (Li et al., 2014). The collected data contain 9,664 experimentally validated miRNA-mRNA interactions, including 276 miRNAs and 14,837 mRNAs. Finally, PAAD-specific 5hmC-modified lncRNA marker-related competitive endogenous RNA (ceRNA) networks were constructed based on ceRNA mechanism as follows: 1) there was a significantly high negative co-expression relationship (p < 0.05 and r < -0.2) between miRNA and mRNA, and between miRNA and lncRNAs; 2) there was significantly high positive co-expression relationship (p < 0.05 and r > 0.4) between mRNA and lncRNAs; 3) there were experimentally validated miRNA-mRNA interactions; This ceRNA network was visualized using the Cytoscape software (version 3.8.2). Functional enrichment analysis of Gene Ontology (GO) and Kyoto Encyclopedia of Genes and Genomes (KEGG) for mRNAs in the ceRNA network was performed with Metascape (Zhou et al., 2019b).
Statistical Analysis
A diagnostic model was developed using the machining learning approach, and a prognostic model was developed using the Cox regression model. The diagnostic model was evaluated with cross-validation and ROC methods. The conventional univariate Cox proportional hazards regression model for overall survival data was implemented to identify variables associated with overall survival. Significant factors in univariate analysis were further subjected to a multivariate Cox regression analysis. Hazard ratios (HR) and corresponding 95% confidence interval (CI) were calculated in the Cox models. The optimal risk cut-off value of the prognostic model was calculated using the R package “survminer”. The PAAD patients were stratified into high- and low-risk groups according to the risk cut-off. Kaplan-Meier curve analysis and Log-rank test were performed to visualize and compare the survival difference between the two risk groups. A Multivariate Cox regression model was used to examine whether the prognostic model remained significant after adjusting clinical variables. Statistical significance was performed for categorical variables using the Wilcoxon signed-rank test for two-group comparisons unless otherwise specified in the figure legend. All statistical analysis was conducted in R software, version 3.6.1.
Results
Identification of Altered Plasma 5hmC Modifications in lncRNAs Genes Involved in PAAD
To examine the 5hmC modification of lncRNAs genes in PAAD, we compared the 5hmC profiles of lncRNAs between PAAD (n = 34) and healthy (n = 96) samples and found a total of six lncRNAs that showed differential 5hmC modification patterns (Figure 2A). Among six lncRNAs, five lncRNAs (RP11-262A16.1, IGF2-AS1, AC108462.1, RP11-714M23.2 and RP3-470B24.5) showed increasing 5hmC modification and one lncRNAs (LINC00486) showed decreasing 5hmC modification in PAAD compared to healthy samples (Figure 2B). These six lncRNAs with abnormal 5hmC modifications were defined as 5hmC-modified lncRNAs. As shown in Figure 2C, the 5hmC levels of six 5hmC-modified lncRNAs were significantly different and revealed clinical potential as diagnostic biomarkers in distinguishing PAAD from healthy controls (Figure 2C).
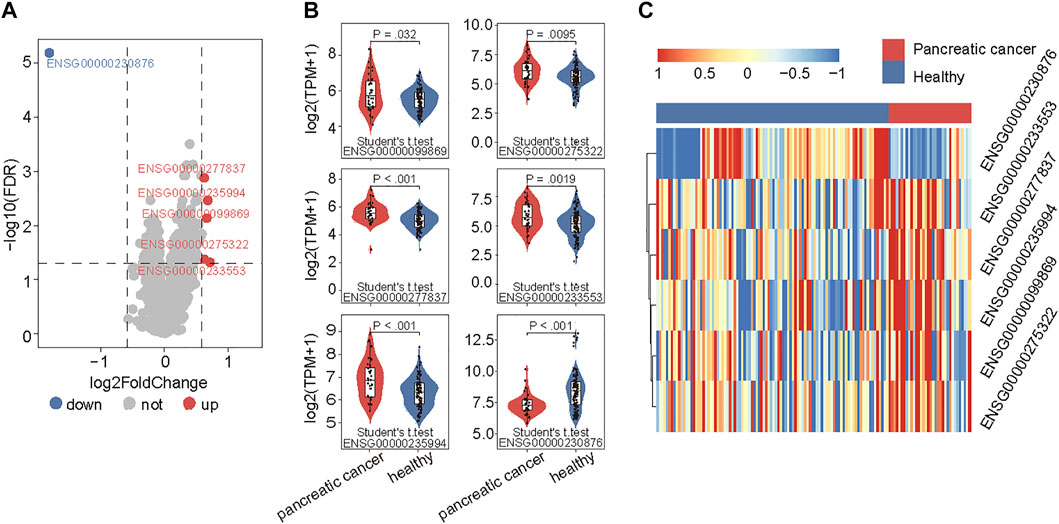
FIGURE 2. Identification of PAAD-specific 5hmC-modified lncRNAs. (A) Volcano plot visualizing the intersections of 5hmC-modified up- and down-enriched lncRNAs in PAAD samples compared with healthy controls. (B) Boxplots showing 5hmC modification levels of lncRNAs between PAAD and healthy samples. Statistical significance was determined using the Student’s t-test. (C) Heatmap of unsupervised hierarchical clustering of PAAD-specific 5hmC modification levels in lncRNAs. PAAD, Pancreatic adenocarcinoma; lncRNA, long non-coding RNAs; 5hmC, 5-Hydroxymethylcytosine.
Development and Validation of a Plasma-Derived Diagnostic Model Based on 5hmC-Modified lncRNAs
To further evaluate the potential of differentially regulated 5hmC modified lncRNAs as diagnostic biomarkers for PAAD, we conducted a discovery-validation study, in which 130 plasma cfDNA samples were split randomly into equally sized training cohort and testing cohort, respectively. We estimated the contribution of each differentially regulated 5hmC modified lncRNAs to disease diagnosis using the 5hmC-modified levels as the contributed score in the training and testing cohorts. As shown in Figures 3A,B, each of the differentially regulated 5hmC modified lncRNAs exhibited a diagnostic performance AUC of 0.62–0.745 in the training cohort and 0.58 to 0.741, respectively.
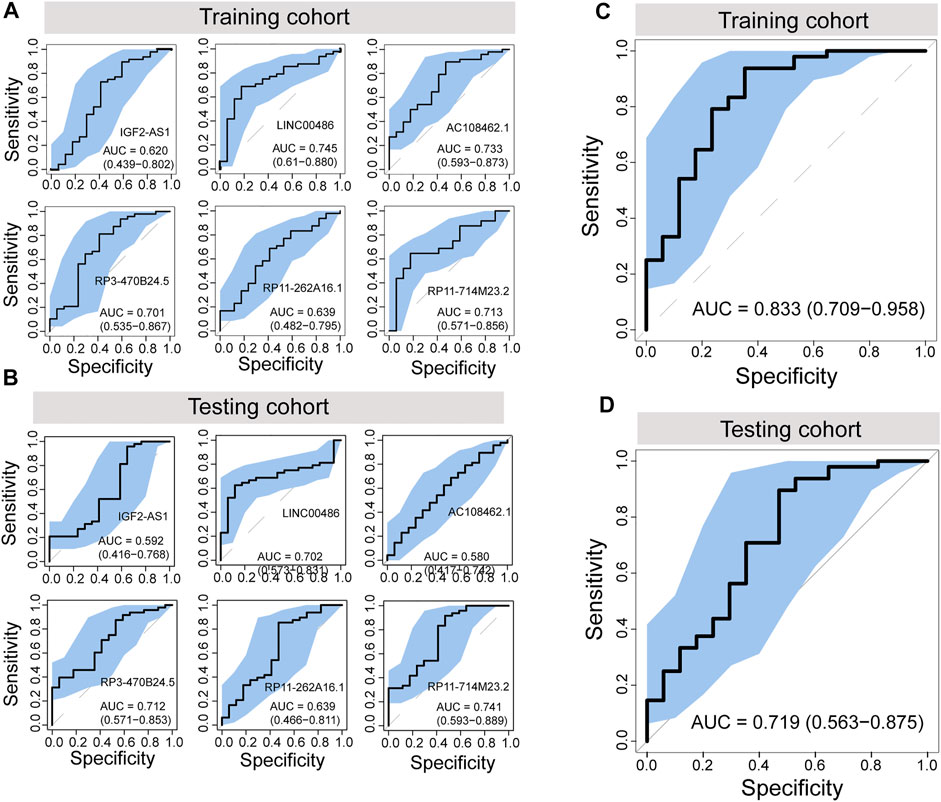
FIGURE 3. Development and independent validation of a plasma-derived diagnostic model (5hLDS) based on 5hmC-modified lncRNAs. ROC curve and AUC value of each PAAD-specific 5hmC-modified lncRNA for cancer diagnosis in the training cohort (A) and testing cohort (B). ROC curve and AUC value of the 5hLDS for cancer diagnosis in the training cohort (C) and testing cohort (D). AUC, Area under the ROC curve; PAAD, Pancreatic adenocarcinoma; p5hmC-score, PAAD 5hmC-LncRNA diagnosis score. PAAD, pancreatic adenocarcinoma.
Then, we developed a machining learning-based non-invasive diagnostic model based on six 5hmC modified lncRNAs using the elastic net regularization on a multivariable logistic regression approach (termed 5hLDS) in the training cohort. Using the ten-cross-validation, the 5hLDS could discriminate PAAD from healthy individuals with an overall AUC of 0.833 (95% CI 0.709-0.958) (Figure 3C). Then the 5hLDS was validated in the independent testing cohort and yielded a classification performance AUC of 0.719 (95% CI 0.563-0.875) for PAAD detection (Figure 3D). At a disease risk cut-off of 0.5, the 5hLDS achieved 81.54% (95% CI 69.97%-90.08%) and 78.46% (95% CI 66.51%-87.69%) of accuracy to distinguish PAAD patients from healthy individuals in the training cohort and testing cohort, respectively. These results demonstrated AUC improvement when incorporated six differentially regulated 5hmC modified lncRNAs into the diagnostic model.
Association Between 5hmC-Modified lncRNAs and Prognosis
We further examined whether expression levels of 5hmC-modified lncRNAs were dysregulated in PAAD compared to controls. Expression profiles of 5hmC-modified lncRNAs were obtained from TCGA and were compared between PAAD primary tumors and normal solid tissues. Two of six 5hmC-modified lncRNAs exhibited differential expression patterns between PAAD primary tumors and normal solid tissues. As shown in Figure 4A, lncRNAs RP3-470B24.5 and RP11-262A16.1 revealed significant or marginally significant downregulated expression levels in PAAD compared to normal solid tissues. Furthermore, three of six 5hmC-modified lncRNAs showed significant association correlation between expression levels and OS, including RP11-262A16.1 (HR = 0.377, 95% CI 0.165–0.865, p = 0.021), RP11-714M23.2 (HR = 2.190, 95% CI 1.163–4.125, p = 0.015) and RP3-470B24.5 (HR = 0.51, 95% CI 0.307–0.848, p = 0.009) (Figure 4B). Kaplan-Meier survival analysis showed that PAAD patients with high expression of RP3-470B24.5 and RP11-262A16.1 have significant improved OS compared to those with low expression (log-rank test p < 0.0082 for RP3-470B24.5 and p < 0.017 for RP11-262A16.1), while PAAD patients with high expression of RP11-714M23.2 tended to have poor OS compared to those with low expression (log-rank test p < 0.013) (Figure 4C). These results demonstrated the potential of three 5hmC-modified lncRNAs as prognostic biomarkers.
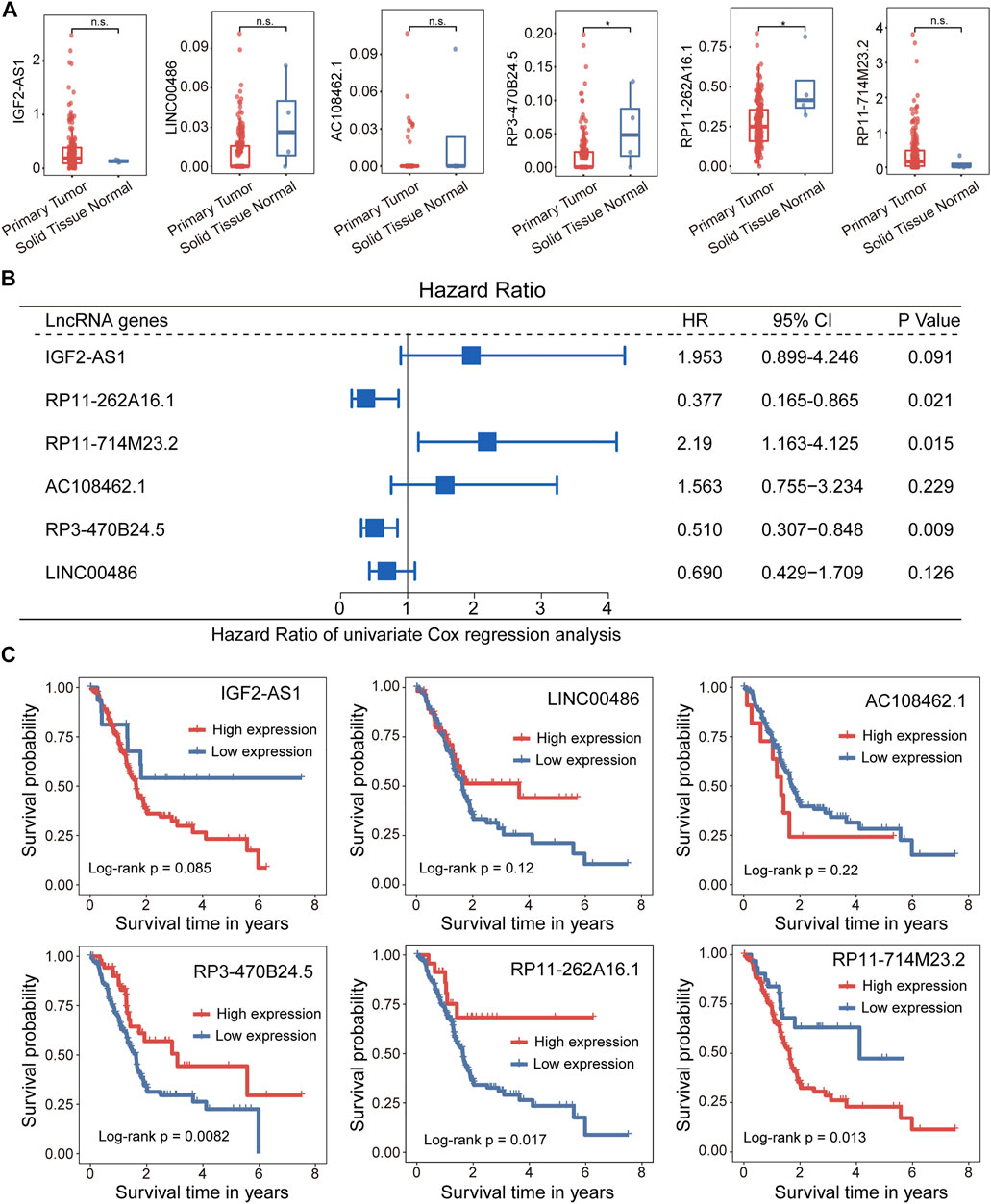
FIGURE 4. Association between the 5hLD-score and disease outcome. (A) Boxplots showing the distribution of expression levels of lncRNAs between PAAD patients and healthy controls. Statistical significance was determined using Wilcoxon signed-rank test. (B) Metaplot of univariate analysis of 5hmC-modified lncRNA between high-expression and low-expression group. (C). Kaplan-Meier survival curves of each PAAD-specific lncRNA between high-expression and low-expression groups. PAAD, pancreatic adenocarcinoma.
5hmC-Modified lncRNAs-Based Prognostic Prediction Model for PAAD
We developed a 5hmC-modified lncRNAs-based prognostic risk score model (5hLPS) for the prognostication of PAAD using the linear combination of the expression of three 5hmC-modified lncRNAs and weighted by relative coefficients in the multivariate Cox regression as follows: 5hLPS = (-0.24378700) × expression value of RP11-262A16.1 + 0.02015193 × expression value of RP11-714M23.2 + (-1.97034856) × expression value of RP3-470B24.5. Then we also conducted a discovery-validation study by splitting PAAD samples from TCGA into equally sized training (n = 88) and testing (n = 88) cohort. The optimal risk cut-off value of the 5hLPS stratified 88 patients of the training cohort into the high-risk group (n = 62) and low-risk group (n = 26) with significantly different OS. As shown in Figure 5A, patients in the high-risk group had significantly shorter OS time than those in the low-risk group (log-rank test p = 0.04). When validated in the testing cohort, the 5hLPS separated the 88 patients of the testing cohort into high-risk (n = 68) and low-risk groups (n = 20). The median survival time in the low-risk group was significantly better than that in the high-risk group (log-rank test p = 0.0035) (Figure 5B). The univariate Cox analysis also showed that the 5hLPS has a significant association with OS both in the training (HR = 2.4, 95% CI: 1.0–5.7, p < 0.05) and testing (HR = 3.6, 95% CI: 1.4–9.2, p < 0.01) cohorts. To further examine whether the 5hLPS was independent of other clinical and pathological factors. We performed multivariable Cox proportional hazards analysis, including individual clinical variables with the 5hLPS in each cohort. As shown in Figure 5C, in the training cohort, the 5hLPS (HR = 2.49, 95% CI 1.05–5.9, p < 0.039) and age (HR = 1.04, 95% CI 1.01–1.1, p = 0.006) were significantly associated with OS in the multivariate analysis. In the testing cohort, only the 5hLPS maintained a significant association with OS (HR = 3.75, 95% CI: 1.48–9.5, p = 0.005) (Figure 5D). These results suggested that 5hLPS is an independent predictive factor for patients with PAAD.
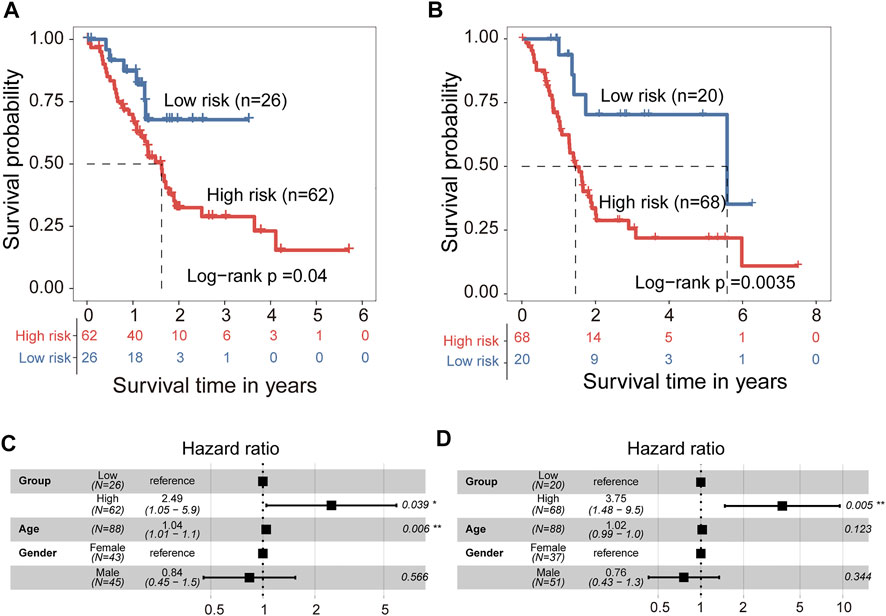
FIGURE 5. Development and independent validation of 5hmC-modified lncRNAs-based prognostic prediction model (5hLPS). Kaplan-Meier survival curves of patients between the high-risk and low-risk groups in training cohort (A) and testing cohort (B). Forest plot of HR deriving from multivariate Cox regression analysis of 5hLPS with other clinical characteristics in training cohort (C) and testing cohort (D). HR, Hazard ratios.
Functional Characterization of 5hmC-Modified lncRNA Markers
To explore the functional roles of 5hmC-modified lncRNAs, we constructed a 5hmC-modified lncRNA marker-associated ceRNA network which included 5,604 interactions among 358 mRNAs, three lncRNAs and 22 miRNAs (Supplementary File S1), as shown in Figure 6A. Then we performed pathway functional enrichment analysis for 358 mRNAs in this ceRNA via Metascape to infer potential functional roles of 5hmC-modified lncRNA markers. As shown in Figure 6B, 5hmC-modified lncRNA marker-associated mRNAs were significantly enriched in angiogenesis, circulatory system process, leukocyte differentiation, and metal ion homeostasis, which are known important events in the development and progression of the development and progression of PAAD.
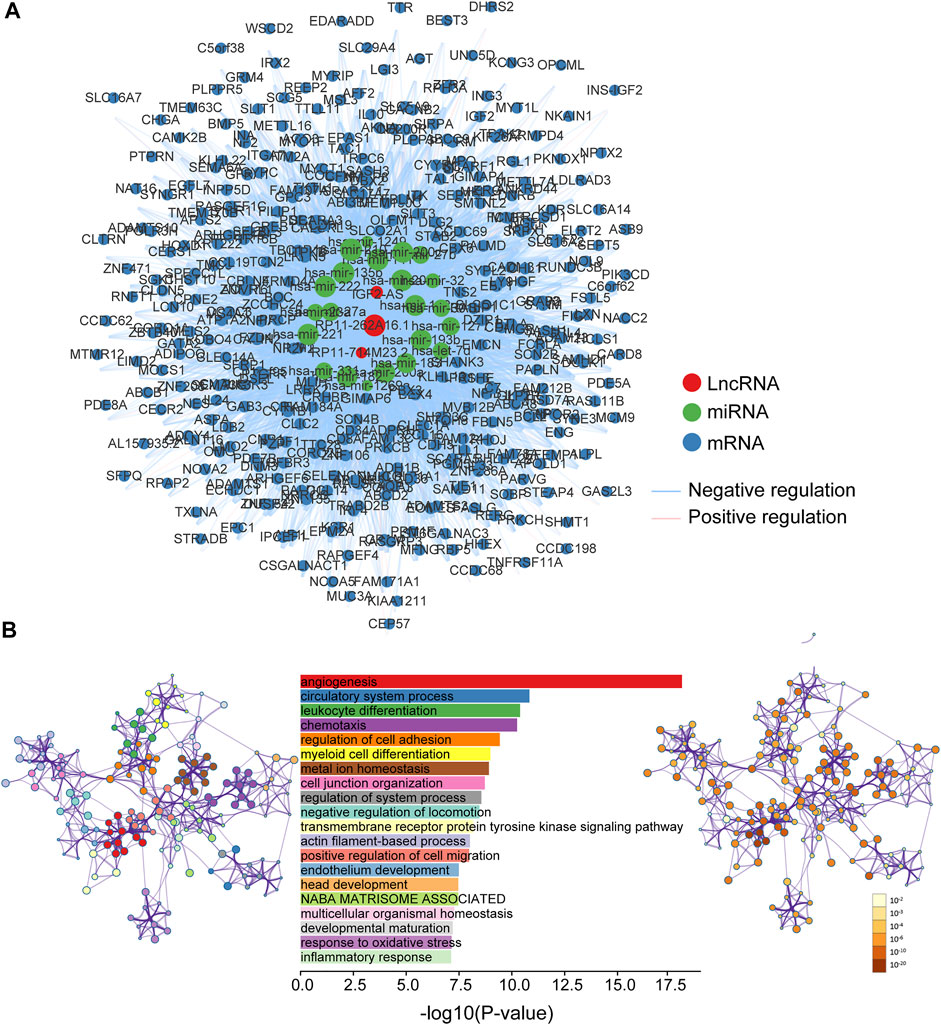
FIGURE 6. Functional characterization of PAAD-specific 5hmC-modified lncRNAs. (A) PAAD-specific 5hmC-modified lncRNA-associated ceRNA network. Blue balls represent mRNAs; red balls represent lncRNA; green balls represent miRNA. Blue edges indicate lncRNA-miRNA-mRNA negative interactions; pink edges indicate lncRNA-miRNA-mRNA positive interactions. The size of the shapes represents the degrees that are involved. The bigger the shape, the higher the degree. (B) Bar graph of enriched terms across mRNA gene lists, length by -log10(P-value). Network graph of the enriched term (left pannel) colored by cluster-ID, where nodes that share the same cluster-ID are typically close to each other; and enriched p-value (right panel) colored by p-value, where terms containing more genes tend to have a more significant p-value. miRNA, microRNA; lncRNA, long non-coding RNA; ceRNA, competitive endogenous RNA; PAAD, pancreatic adenocarcinoma.
Discussion
Pancreatic adenocarcinoma (PAAD) has an inferior prognosis and remains a lethal malignancy (Schizas et al., 2020). Recent studies suggest that aberrant expression of lncRNA drives the initiation and progression of malignancies (Bhan et al., 2017). Liquid biopsies provide a non-invasive approach to detect tumors (Shen et al., 2018) and a novel Nano-hmC-Seal technology to generate the genome-wide profiles of 5hmC in cfDNA from blood plasma for multiple cancer types (Li et al., 2017). Whether plasma-derived 5hmC-modified lncRNA is a conclusive biomarker for distinguishing the type of cancer and diagnosing cancer is unclear. In this study, we explored the potential application of the plasma-derived 5hmC modification level in lncRNA being used as an alternative biomarker for PAAD diagnosis and monitoring.
In this study, by redefining 5hmC sequencing reads to lncRNA genes, 5hmC alterations of lncRNAs were characterized in PAAD. Several altered 5hmC modifications were distributed at lncRNAs in patients with PAAD compared with healthy subjects. Herein, these differentially regulated 5hmC modified lncRNAs were considered as PAAD-specific markers. We trained machine-learning algorithms with 10-fold cross-validation using the differentially regulated 5hmC modified lncRNAs as features (termed 5hLDS), and evaluated the prediction performance in the training and testing cohorts. The 5hLDS achieved superior diagnostic performance in distinguishing PAAD from healthy controls both in the training and testing cohorts.
Further exploring of expression relevance of the 5hmC-modified lncRNAs in PAAD identified two of six 5hmC-modified lncRNAs that were dysregulated expression in PAAD compared to normal tissues, suggesting that these 5hmC modifications changes might lead to dysregulated lncRNAs expression that involved in the development of PAAD. Furthermore, we further investigated the effect of expression of 5hmC-modified lncRNAs on survival and found that 5hmC-modified lncRNAs were also able to make a distinction between each PAAD patient into distinct groups with better or worse survival outcomes. These results also support the idea that 5hmC-modified lncRNAs can serve as potential biomarkers for the prognosis of PAAD patients. Therefore, we trained a regression model with Cox analysis using the differentially regulated 5hmC modified lncRNAs as features (termed 5hLPS), and evaluated the prognostic performance in the training and testing cohorts. The 5hLPS exhibited superior performance in classifying the patients into two groups with significantly different overall survival independent of clinical variables.
Although many lncRNAs have been discovered and recorded in a vast amount of literature, only a few have been well-functionally studied and characterized. It has been reported that lncRNAs can act as ceRNA for miRNA and emerge as important regulators involved in diverse biological and physiopathological contexts. Among six 5hmC-modified lncRNAs identified in this study, lncRNA IGF2-AS1 is expressed in antisense to the insulin-like growth factor 2 (IGF2) gene and is imprinted and paternally expressed (Vu et al., 2003). Many studies suggested that transcripts from IGF2-AS1 are produced in tumors and may suppress cell growth (Unger et al., 2017; Kasprzak and Adamek, 2019; Contractor et al., 2020). Another 5hmC-modified lncRNAs, LINC00486, has been reported to be a hot spot of breakpoints and has a high rearrangement rate in non-small cell lung cancer cells (Wang et al., 2018). A recent study also found that LINC00486 may act as a tumor suppressor gene and its overexpression can inhibit the proliferation and promote the apoptosis of breast cancer tissues by suppressing miR-182–5p expression (Yuan et al., 2020). To further gain a novel functional insight for 5hmC-modified lncRNAs, we construed a 5hmC-modified lncRNAs-associated ceRNA network according to the ceRNA hypothesis and found that mRNA in this ceRNA network were involved in the known crucial event in the development and progression of PAAD, such as angiogenesis, circulatory system process, leukocyte differentiation and metal ion homeostasis.
The present study still had its limitations. First, this study did not obtain significant clinical variables of 5hmC-modified lncRNAs, such as follow-up time. Further independent validation studies should be performed to help address problems such as the potential selection bias for model construction. Secondly, the regulatory mechanism of 5hmC and lncRNA genes remains unclear due to the lack of paired 5hmC profiles and lncRNAs expression profiles. Finally, although the 5hmC-modified lncRNAs have been validated in expression levels from the TCGA cohort, further validation is required in other retrospective or prospective cohorts to demonstrate the generalizability of 5hmC-modified lncRNA for PAAD diagnosis and prognosis.
Conclusion
This study characterized the genome-wide pattern of lncRNA-associated plasma cfDNA 5hmC changes in PAAD, and demonstrated potential roles for 5hmC in the transcriptional regulation of lncRNAs contributing to the development and progression of PAAD. Finally, we identified and validated 5hmC-modified lncRNA panels for the diagnosis and prognosis of PAAD with high and robust performance, which presented potential clinical utility of 5hmC-modified lncRNAs as valuable biomarkers for non-invasive diagnosis and prognostication of cancers.
Data Availability Statement
Publicly available datasets were analyzed in this study. This data can be found here: The datasets of this article are available in the Sequence Read Archive repository (https://trace.ncbi.nlm.nih.gov/Traces/sra/?study=SRP080977) and UCSC Xena (https://xena.ucsc.edu/).
Author Contributions
GC, MW, and MZ designed the study; SL and YW developed the methodology; SL, YW, and CW performed data analysis. SL and GC drafted the manuscript. All authors read and approved the final manuscript.
Funding
This study was supported by the Key Laboratory of Intelligent Medical Imaging of Wenzhou (No. 2021HZSY0057).
Conflict of Interest
The authors declare that the research was conducted in the absence of any commercial or financial relationships that could be construed as a potential conflict of interest.
Publisher’s Note
All claims expressed in this article are solely those of the authors and do not necessarily represent those of their affiliated organizations, or those of the publisher, the editors and the reviewers. Any product that may be evaluated in this article, orclaim that may be made by its manufacturer, is not guaranteed or endorsed by the publisher.
Supplementary Material
The Supplementary Material for this article can be found online at: https://www.frontiersin.org/articles/10.3389/fcell.2022.845641/full#supplementary-material
References
Bao, S., Zhao, H., Yuan, J., Fan, D., Zhang, Z., Su, J., et al. (2020). Computational Identification of Mutator-Derived lncRNA Signatures of Genome Instability for Improving the Clinical Outcome of Cancers: a Case Study in Breast Cancer. Brief Bioinform 21, 1742–1755. doi:10.1093/bib/bbz118
Bhan, A., Soleimani, M., and Mandal, S. S. (2017). Long Noncoding RNA and Cancer: A New Paradigm. Cancer Res. 77, 3965–3981. doi:10.1158/0008-5472.can-16-2634
Contractor, T., Clausen, R., Harris, G. R., Rosenfeld, J. A., Carpizo, D. R., Tang, L., et al. (2020). IGF2 Drives Formation of Ileal Neuroendocrine Tumors in Patients and Mice. Endocr. Relat. Cancer 27, 175–186. doi:10.1530/erc-19-0505
Diaz, L. A., and Bardelli, A. (2014). Liquid Biopsies: Genotyping Circulating Tumor DNA. Jco 32, 579–586. doi:10.1200/jco.2012.45.2011
E. Poruk, K., Z. Gay, D., Brown, K., D. Mulvihill, J., M. Boucher, K., L. Scaife, C., et al. (2013). The Clinical Utility of CA 19-9 in Pancreatic Adenocarcinoma: Diagnostic and Prognostic Updates. Cmm 13, 340–351. doi:10.2174/1566524011313030003
Fu, Z., Chen, C., Zhou, Q., Wang, Y., Zhao, Y., Zhao, X., et al. (2017). LncRNA HOTTIP Modulates Cancer Stem Cell Properties in Human Pancreatic Cancer by Regulating HOXA9. Cancer Lett. 410, 68–81. doi:10.1016/j.canlet.2017.09.019
Guo, S., Diep, D., Plongthongkum, N., Fung, H.-L., Zhang, K., and Zhang, K. (2017). Identification of Methylation Haplotype Blocks Aids in Deconvolution of Heterogeneous Tissue Samples and Tumor Tissue-Of-Origin Mapping from Plasma DNA. Nat. Genet. 49, 635–642. doi:10.1038/ng.3805
Guttman, M., Amit, I., Garber, M., French, C., Lin, M. F., Feldser, D., et al. (2009). Chromatin Signature Reveals over a Thousand Highly Conserved Large Non-coding RNAs in Mammals. Nature 458, 223–227. doi:10.1038/nature07672
Han, D., Lu, X., Shih, A. H., Nie, J., You, Q., Xu, M. M., et al. (2016). A Highly Sensitive and Robust Method for Genome-wide 5hmC Profiling of Rare Cell Populations. Mol. Cel 63, 711–719. doi:10.1016/j.molcel.2016.06.028
Hu, H., Shu, M., He, L., Yu, X., Liu, X., Lu, Y., et al. (2017). Epigenomic Landscape of 5-hydroxymethylcytosine Reveals its Transcriptional Regulation of lncRNAs in Colorectal Cancer. Br. J. Cancer 116, 658–668. doi:10.1038/bjc.2016.457
Huang, X., Zhi, X., Gao, Y., Ta, N., Jiang, H., and Zheng, J. (2016). LncRNAs in Pancreatic Cancer. Oncotarget 7, 57379–57390. doi:10.18632/oncotarget.10545
Jin, S.-G., Jiang, Y., Qiu, R., Rauch, T. A., Wang, Y., Schackert, G., et al. (2011). 5-Hydroxymethylcytosine Is Strongly Depleted in Human Cancers but its Levels Do Not Correlate with IDH1 Mutations. Cancer Res. 71, 7360–7365. doi:10.1158/0008-5472.can-11-2023
Kasprzak, A., and Adamek, A. (2019). Insulin-Like Growth Factor 2 (IGF2) Signaling in Colorectal Cancer-From Basic Research to Potential Clinical Applications. Int. J. Mol. Sci. 20, 4915. doi:10.3390/ijms20194915
Langmead, B., and Salzberg, S. L. (2012). Fast Gapped-Read Alignment with Bowtie 2. Nat. Methods 9, 357–359. doi:10.1038/nmeth.1923
Lehmann-Werman, R., Neiman, D., Zemmour, H., Moss, J., Magenheim, J., Vaknin-Dembinsky, A., et al. (2016). Identification of Tissue-specific Cell Death Using Methylation Patterns of Circulating DNA. Proc. Natl. Acad. Sci. USA 113, E1826–E1834. doi:10.1073/pnas.1519286113
Li, H., Handsaker, B., Wysoker, A., Fennell, T., Ruan, J., Homer, N., et al. (2009). The Sequence Alignment/Map Format and SAMtools. Bioinformatics 25, 2078–2079. doi:10.1093/bioinformatics/btp352
Li, J.-H., Liu, S., Zhou, H., Qu, L.-H., and Yang, J.-H. (2014). starBase v2.0: Decoding miRNA-ceRNA, miRNA-ncRNA and Protein-RNA Interaction Networks from Large-Scale CLIP-Seq Data. Nucl. Acids Res. 42, D92–D97. doi:10.1093/nar/gkt1248
Li, W., Zhang, X., Lu, X., You, L., Song, Y., Luo, Z., et al. (2017). 5-Hydroxymethylcytosine Signatures in Circulating Cell-free DNA as Diagnostic Biomarkers for Human Cancers. Cell Res 27, 1243–1257. doi:10.1038/cr.2017.121
Love, M. I., Huber, W., and Anders, S. (2014). Moderated Estimation of Fold Change and Dispersion for RNA-Seq Data with DESeq2. Genome Biol. 15, 550. doi:10.1186/s13059-014-0550-8
Marchese, F. P., Raimondi, I., and Huarte, M. (2017). The Multidimensional Mechanisms of Long Noncoding RNA Function. Genome Biol. 18, 206. doi:10.1186/s13059-017-1348-2
Moutinho-Ribeiro, P., Coelho, R., Giovannini, M., and Macedo, G. (2017). Pancreatic Cancer Screening: Still a Delusion? Pancreatology 17, 754–765. doi:10.1016/j.pan.2017.07.001
Quinlan, A. R., and Hall, I. M. (2010). BEDTools: a Flexible Suite of Utilities for Comparing Genomic Features. Bioinformatics 26, 841–842. doi:10.1093/bioinformatics/btq033
Schizas, D., Charalampakis, N., Kole, C., Economopoulou, P., Koustas, E., Gkotsis, E., et al. (2020). Immunotherapy for Pancreatic Cancer: A 2020 Update. Cancer Treat. Rev. 86, 102016. doi:10.1016/j.ctrv.2020.102016
Schultz, N. A., Dehlendorff, C., Jensen, B. V., Bjerregaard, J. K., Nielsen, K. R., Bojesen, S. E., et al. (2014). MicroRNA Biomarkers in Whole Blood for Detection of Pancreatic Cancer. JAMA 311, 392–404. doi:10.1001/jama.2013.284664
Shen, S. Y., Singhania, R., Fehringer, G., Chakravarthy, A., Roehrl, M. H. A., Chadwick, D., et al. (2018). Sensitive Tumour Detection and Classification Using Plasma Cell-free DNA Methylomes. Nature 563, 579–583. doi:10.1038/s41586-018-0703-0
Siegel, R. L., Miller, K. D., and Jemal, A. (2020). Cancer Statistics, 2020. CA A. Cancer J. Clin. 70, 7–30. doi:10.3322/caac.21590
Sohal, D. P. S., Willingham, F. F., Falconi, M., Raphael, K. L., and Crippa, S. (2017). Pancreatic Adenocarcinoma: Improving Prevention and Survivorship. Am. Soc. Clin. Oncol. Educ. Book 37, 301–310. doi:10.1200/edbk_175222
Song, C.-X., Yin, S., Ma, L., Wheeler, A., Chen, Y., Zhang, Y., et al. (2017). 5-Hydroxymethylcytosine Signatures in Cell-free DNA Provide Information about Tumor Types and Stages. Cel Res 27, 1231–1242. doi:10.1038/cr.2017.106
Tahiliani, M., Koh, K. P., Shen, Y., Pastor, W. A., Bandukwala, H., Brudno, Y., et al. (2009). Conversion of 5-methylcytosine to 5-hydroxymethylcytosine in Mammalian DNA by MLL Partner TET1. Science 324, 930–935. doi:10.1126/science.1170116
Tang, X., Zhang, M., Sun, L., Xu, F., Peng, X., Zhang, Y., et al. (2021). The Biological Function Delineated across Pan-Cancer Levels through lncRNA-Based Prognostic Risk Assessment Factors for Pancreatic Cancer. Front. Cel Dev. Biol. 9, 694652. doi:10.3389/fcell.2021.694652
Unger, C., Kramer, N., Unterleuthner, D., Scherzer, M., Burian, A., Rudisch, A., et al. (2017). Stromal-derived IGF2 Promotes colon Cancer Progression via Paracrine and Autocrine Mechanisms. Oncogene 36, 5341–5355. doi:10.1038/onc.2017.116
Vu, T. H., Chuyen, N. V., Li, T., and Hoffman, A. R. (2003). Loss of Imprinting of IGF2 Sense and Antisense Transcripts in Wilms' Tumor. Cancer Res. 63, 1900–1905.
Wan, J. C. M., Massie, C., Garcia-Corbacho, J., Mouliere, F., Brenton, J. D., Caldas, C., et al. (2017). Liquid Biopsies Come of Age: towards Implementation of Circulating Tumour DNA. Nat. Rev. Cancer 17, 223–238. doi:10.1038/nrc.2017.7
Wang, C., Yin, R., Dai, J., Gu, Y., Cui, S., Ma, H., et al. (2018). Whole-genome Sequencing Reveals Genomic Signatures Associated with the Inflammatory Microenvironments in Chinese NSCLC Patients. Nat. Commun. 9, 2054. doi:10.1038/s41467-018-04492-2
Wang, J., Tang, J., Lai, M., and Zhang, H. (2014). 5-Hydroxymethylcytosine and Disease. Mutat. Research/Reviews Mutat. Res. 762, 167–175. doi:10.1016/j.mrrev.2014.09.003
White, N. M., Cabanski, C. R., Silva-Fisher, J. M., Dang, H. X., Govindan, R., and Maher, C. A. (2014). Transcriptome Sequencing Reveals Altered Long Intergenic Non-coding RNAs in Lung Cancer. Genome Biol. 15, 429. doi:10.1186/s13059-014-0429-8
Xu, R.-h., Wei, W., Krawczyk, M., Wang, W., Luo, H., Flagg, K., et al. (2017). Circulating Tumour DNA Methylation Markers for Diagnosis and Prognosis of Hepatocellular Carcinoma. Nat. Mater 16, 1155–1161. doi:10.1038/nmat4997
Yan, X., Hu, Z., Feng, Y., Hu, X., Yuan, J., Zhao, S. D., et al. (2015). Comprehensive Genomic Characterization of Long Non-coding RNAs across Human Cancers. Cancer Cell 28, 529–540. doi:10.1016/j.ccell.2015.09.006
Yuan, X., Li, Y., and Li, P. (2020). Regulatory Effects of Linc00486 on Biological Characteristics of Breast Cancer Cells. Revista argentina de clínica psicológica 29, 356–363.
Zhang, H., Zhu, C., He, Z., Chen, S., Li, L., and Sun, C. (2020). LncRNA PSMB8-AS1 Contributes to Pancreatic Cancer Progression via Modulating miR-382-3p/STAT1/PD-L1 axis. J. Exp. Clin. Cancer Res. 39, 179. doi:10.1186/s13046-020-01687-8
Zhang, Y., Yang, J., Li, H., Wu, Y., Zhang, H., and Chen, W. (2015). Tumor Markers CA19-9, CA242 and CEA in the Diagnosis of Pancreatic Cancer: a Meta-Analysis. Int. J. Clin. Exp. Med. 8, 11683–11691.
Zhou, M., Diao, Z., Yue, X., Chen, Y., Zhao, H., Cheng, L., et al. (2016). Construction and Analysis of Dysregulated lncRNA-Associated ceRNA Network Identified Novel lncRNA Biomarkers for Early Diagnosis of Human Pancreatic Cancer. Oncotarget 7, 56383–56394. doi:10.18632/oncotarget.10891
Zhou, M., Hou, P., Yan, C., Chen, L., Li, K., Wang, Y., et al. (2021a). Cell-free DNA 5-hydroxymethylcytosine Profiles of Long Non-coding RNA Genes Enable Early Detection and Progression Monitoring of Human Cancers. Clin. Epigenet 13, 197. doi:10.1186/s13148-021-01183-6
Zhou, M., Zhang, Z., Bao, S., Hou, P., Yan, C., Su, J., et al. (2021b). Computational Recognition of lncRNA Signature of Tumor-Infiltrating B Lymphocytes with Potential Implications in Prognosis and Immunotherapy of Bladder Cancer. Brief Bioinform 22, bbaa047. doi:10.1093/bib/bbaa047
Zhou, M., Zhao, H., Wang, X., Sun, J., and Su, J. (2019a). Analysis of Long Noncoding RNAs Highlights Region-specific Altered Expression Patterns and Diagnostic Roles in Alzheimer's Disease. Brief Bioinform 20, 598–608. doi:10.1093/bib/bby021
Keywords: 5-hydroxymethylcytosine, pancreatic cancer, machining learning, long non-coding RNA, non-invasive biomarker
Citation: Li S, Wang Y, Wen C, Zhu M, Wang M and Cao G (2022) Integrative Analysis of 5-Hydroxymethylcytosine and Transcriptional Profiling Identified 5hmC-Modified lncRNA Panel as Non-Invasive Biomarkers for Diagnosis and Prognosis of Pancreatic Cancer. Front. Cell Dev. Biol. 10:845641. doi: 10.3389/fcell.2022.845641
Received: 30 December 2021; Accepted: 07 February 2022;
Published: 25 March 2022.
Edited by:
Chunjie Jiang, University of Pennsylvania, United StatesReviewed by:
Jie Zhang, Tongji University, ChinaHao Lin, University of Electronic Science and Technology of China, China
Copyright © 2022 Li, Wang, Wen, Zhu, Wang and Cao. This is an open-access article distributed under the terms of the Creative Commons Attribution License (CC BY). The use, distribution or reproduction in other forums is permitted, provided the original author(s) and the copyright owner(s) are credited and that the original publication in this journal is cited, in accordance with accepted academic practice. No use, distribution or reproduction is permitted which does not comply with these terms.
*Correspondence: Mingxi Zhu, aHkwMjA2MTc1QGhhaW5tYy5lZHUuY24=; Meihao Wang, d3p3bWhAd211LmVkdS5jbg==; Guoquan Cao, Y2FvZ3VvcXVhbkB3bXUuZWR1LmNu
†These authors have contributed equally to this work