- 1Department of Thoracic Surgery, Zhongnan Hospital, Wuhan University, Wuhan, China
- 2School of Computer Science and Technology, Harbin Institute of Technology, Harbin, China
- 3Department of Biological Repositories, Zhongnan Hospital, Wuhan University, Wuhan, China
Lung carcinoma is one of the most deadly malignant tumors in mankind. With the rising incidence of lung cancer, searching for the high effective cures become more and more imperative. There has been sufficient research evidence that living habits and situations such as smoking and air pollution are associated with an increased risk of lung cancer. Simultaneously, the influence of individual genetic susceptibility on lung carcinoma morbidity has been confirmed, and a growing body of evidence has been accumulated on the relationship between various risk factors and the risk of different pathological types of lung cancer. Additionally, the analyses from many large-scale cancer registries have shown a degree of familial aggregation of lung cancer. To explore lung cancer-related genetic factors, Genome-Wide Association Studies (GWAS) have been used to identify several lung cancer susceptibility sites and have been widely validated. However, the biological mechanism behind the impact of these site mutations on lung cancer remains unclear. Therefore, this study applied the Summary data-based Mendelian Randomization (SMR) model through the integration of two GWAS datasets and four expression Quantitative Trait Loci (eQTL) datasets to identify susceptibility genes. Using this strategy, we found ten of Single Nucleotide Polymorphisms (SNPs) sites that affect the occurrence and development of lung tumors by regulating the expression of seven genes. Further analysis of the signaling pathway about these genes not only provides important clues to explain the pathogenesis of lung cancer but also has critical significance for the diagnosis and treatment of lung cancer.
Introduction
Pulmonary carcinoma is the leading cause of cancer deaths in the world. According to the Global Cancer Statistics report, there were about 2 million newly detected lung cancer cases worldwide in 2018, accounting for 11.6% of all tumors (Bray et al., 2018). Deaths from lung cancer worldwide were roughly 1.7 million, accounting for 18.4% of tumor-related deaths. Lung cancer is a malignant disease resulting from malignant changes of bronchial and alveolar epithelial cells. Lung cancer is divided into two categories: non-small cell lung cancer (NSCLC) and small cell lung cancer (SCLC). Of all diagnosed cases of lung cancer, NSCLC accounts for about 85% (Oser et al., 2015). Based on the characteristics of lung carcinoma, non-small cell lung cancer is also split into three: pulmonary adenocarcinoma, Lung squamous cell carcinoma, and large cell lung cancer. Although SCLC is a small proportion, it grows and spreads faster than NSCLC and tends to metastasize early in the disease (Zakaria et al., 2015). Bearing these facts in mind, the therapy of lung cancer faces a serious challenge.
It is immediately unclear how pulmonary carcinoma develops. As shown in many kinds of research, the occurrence of lung cancer can be driven by multiple factors including genetic and environmental factors. In particular, SCLC is generally related to lifestyle and environment (Rudina et al., 2021). Besides external environmental factors, genetic factors also play an important role in the occurrence of lung cancer. For example, the incidence of lung adenocarcinoma represents more than 55% of NSCLC, and it is more appropriate to many women and non-smokers (Barr Kumarakulasinghe et al., 2015). These people tend to carry gene mutations. The common mutated genes contain EGFR, ALK, ROS1 gene, and so on (Lamberti et al., 2020). In a word, exploring genetic factors has critical implications for understanding the pathogenic mechanism of pulmonary cancer.
In recent decades, many genetic studies have been utilized to determine the genetic factors of lung cancer. Genome-wide association studies (GWAS) as a hot research tool at present have been applied in lung cancer, and have successfully identified many genetic susceptibility sites (Landi et al., 2009). GWAS perform genotype on several hundreds of thousands of Single Nucleotide Polymorphisms (SNPs) according to the comparison of a large number of lung cancer cases and healthy controls, and then bioinformatics techniques and statistical methods were employed to compare the frequency diversities of each SNP between the two groups (Ko and Urban, 2013). In the results of GWAS analysis, most important SNPs are located in non-coding regions, thus it is difficult to directly explore the regulatory mechanism of these loci (Rojano et al., 2016). Therefore, although we have identified many susceptibility sites associated with lung tumors, it remains unknown about the role of lung cancer pathogenesis-related genes or DNA elements.
Accordingly, this research adopted the method of multi-omics analysis to consolidate various sources of data in an attempt to identify genes related to lung cancer incidence. With the development of high-throughput omics platforms, multi-omics analysis is one of the most important and widely used in biomedical research (Tanaka et al., 2020; Tarwadi et al., 2020; Zhao et al., 2021; Zhao, 2021). As these are kind of hard to describe diseases with complex etiologies by a single omics analysis, the multi-omics research method is developed to provide more evidence for the disease pathogenesis and dig out candidate key factors in depth. Data from different omics sources such as genomics, transcriptomics, and proteomics can be integrated through machine learning or statistical methods for purpose of underlying biological mechanisms (Zhao et al., 2020; Reel, 2021). In this study, Summary data-based Mendelian Randomization (SMR) was used as a statistical method for multi-omics analysis.
SMR was conducted to investigate the pathogenic genes of lung cancer. SMR analysis is an improved model based on Mendelian randomization study that uses genetic variation as a tool to predict the effects of simulated exposure factor on disease using gene-disease causal inference (Zhu et al., 2016). Compared with the general instrumental variable models, the MR model can significantly reduce the estimation bias caused by measuring error. In the MR model, genetic variants as an instrumental variable have to satisfy three core assumptions. The three core assumptions consist of the relevance assumption, the independence assumption, and the exclusion restriction assumption (Hartwig and Davies, 2016). The relevance refers to a robust correlation between genetic variants and exposure factors. The independence assumption represents the independence of genetic variation and confounding factors affecting the expose-outcome relationship (Zhao et al., 2019). The exclusion restriction states that genetic variation can affect outcomes only through exposure factors and not through other pathways. The violation of any of the core assumptions may distort the statistical inference results of MR analysis, leading to incorrect inferences of causality.
However, the existence of linkage disequilibrium (LD) or pleiotropy of instrumental variables can be contrary to the exclusion restriction. Once two genes are not completely independent, they will show some degree of linkage, which is called linkage imbalance. Pleiotropy is the phenomenon that one gene can affect more than one trait. Due to the coordinate positions in the genome between SNPs and the complicated biological effects between genetic variants and traits, LD and pleiotropy are often unavoidable (Wang, 2021). Therefore, to soften the influence of the exclusivity hypothesis, a variety of modified MR methods, such as Mendelian Randomization-Egger regression (MR-Egger), Inverse Variance Weighted (IVW), Mode-Based Estimate (MBE) method, have been proposed in recent years. These methods only consider the analytical error because of pleiotropy. SMR method also takes into account the LD between instrumental variables to decrease the false positive rate. In addition, there are fewer requirements for the summary data of GWAS and eQTL, and the sample size in SMR analysis. As a result, we adopted this approach because of its wide applicability and high accuracy.
In this research, we integrated lung cancer GWAS data and eQTL data to recognize functional genes and regulatory elements in pulmonary carcinoma. Statistical analysis of the relationship between a single SNP and gene expression is referred to as eQTL analysis (Shabalin, 2012). Namely, the expression of a gene is affected by a single SNP, this variable site is considered to be an eQTL locus. Since the subjects of both GWAS and eQTL were SNPs, SMR analysis of overlapping mutants presented in GWAS and eQTL data could reveal lung cancer related-genes. The discovery of these genes could effectively support the design of targeted drugs and precise treatment of lung cancer.
Materials and Methods
Acquisition of GWAS Summary Data
We downloaded two available GWAS summary data of lung cancer from the GWAS Catalog website. One of the GWAS datasets was obtained from the meta-analysis of the United Kingdom Biobank (UKB) and the Kaiser Permanente Genetic Epidemiology Research on Adult Health and Aging (GERA) (Rashkin et al., 2020). The UKB study was composed of 502,611 British people aged 40 to 69 at the time of recruitment. Study participants provided biological samples as well as detailed information on lifestyle and health-related factors. GERA participants were members of the Adult Kaiser Permanente Northern California (KPNC) Health Plan. The subjects of this study included 102,979 participants selected for their genotyping. Cancer cases in the UKB were identified on the basis of information provided by various national cancer registries that gathered data from hospitals, nursing homes, and so on. GERA cancer cases group were classified by using diagnoses from the KPNC cancer registry. The control group for the UKB and GERA studies was limited to individuals in the study who did not have any history of lung cancer in the relevant registries.
The other GWAS summary data was collected from the analysis of the BioBank Japan Project (BBJ) data (Sakaue, 2021). BBJ is a prospective biobank that collaboratively collects the DNA and serum samples of 200,000 Japanese participants from 12 medical institutions in Japan. All study participants were diagnosed with one or more of 47 target diseases by doctors. In this study, 178,726 participants of Japanese ancestry were selected from all participants. The researchers used a sample of the cohort without a given lung cancer diagnosis as a control group.
Collection of eQTL Summary Data
Four eQTL data containing three blood eQTL data and one lung eQTL data were applied in our research. Two of eQTL data were obtained by sequencing whole peripheral blood mRNA of 2,116 healthy adults from four Dutch cohorts (Zhernakova et al., 2017). The difference between the two datasets was that one described 23,060 eQTL effects of gene level, the other included 21,888 eQTL effects of exon level. The remaining two of eQTL data were downloaded from the GTEx website. Since eQTL varies in different tissues or cells, we selected one lung eQTL dataset and one blood eQTL dataset to perform the analysis. Lung and blood eQTL datasets stored eQTL information about 25,245 genes and 20,049 genes, respectively.
SMR Analysis Through Lung Cancer GWAS and eQTL Summary Data
The research adopted the SMR analysis method to perform the conjoint analysis of eQTL and GWAS (Figure 1). Prior to compute SMR, we first need to find overlapped SNPs of GWAS and eQTL and generate a new dataset containing all overlapping SNPs information. Then the GWAS and eQTL data of new datasets were standardized by Z Score. We can calculate the Z value based on the Beta (OR) value and one of the p values or the SE value. The core algorithms of eQTL and GWAS are based on linear regression. Therefore, the Beta/OR value of GWAS and eQTL represents the value of the regression coefficient. SE value refers to the standard error of regression coefficient. The p value means the value of whether the association is significant. We listed two calculation formulas of the Z value below (Eq. 1; Eq. 2).
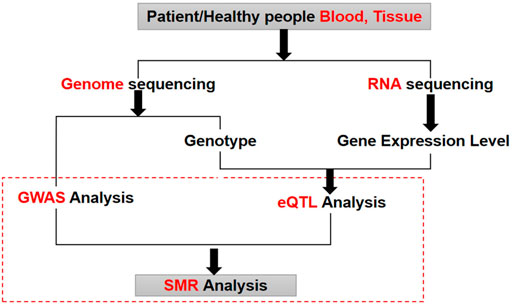
FIGURE 1. The process of GWAS and eQTL conjoint analysis. GWAS analysis and eQTL analysis are two different types of omics analysis. Using SMR method can discover the biological mechanism behind lung cancer by combining these two different omics data.
The Z values of GWAS and eQTL were used to calculate the TSMR values according to Eq. 3 based on the Delta algorithm (Figure 2). The TSMR values were output as PSMR values by chi-square test. As False Discovery Rate (FDR) method can control false positive events by correcting the p values, we used FDR to adjust the PSMR values, resulting in obtaining the Padj values. The Padj value of 0.05 was taken as the threshold of statistical significance, which means that the genes corresponding to Padj less than 0.05 could be regarded as lung cancer-related pathogenic genes found in this study.
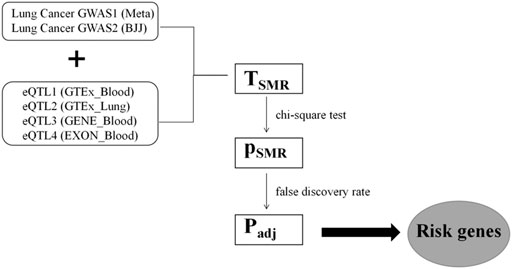
FIGURE 2. The process of GWAS and eQTL conjoint analysis. The two GWAS datasets of lung cancer were cross-analyzed with four groups of eQTL datasets. Significance of gene expression was found after TSMR calculation, chi-square test, and FDR correction.
Results
Discovery of the Overlapping SNPs Between GWAS and eQTL
In this SMR analysis, the first step was to find the same SNPs between one of the GWAS datasets and one of the eQTL datasets and generate a new SMR dataset containing the details of all the overlapping SNPs (Table 1). We performed four SMR analyses by using GWAS summary data from a large meta-analysis to examine the association between lung cancer and gene expression probes from four eQTL data. The number of SNPs found in Meta_Blood_Exon data was higher than the other three data, with nearly a hundred thousand SNPs and more than twenty thousand genes found (Figure 3). The remaining three SMR datasets contained 10,000–20,000 SNPs and gene overlaps. In addition, GWAS data collected from the Japanese populations was also conducted four SMR analyses with different eQTL data. The overlap analysis results show that both BBJ_GTEx_Blood and BBJ_GTEx_Lung contained about 20,000 SNPs and genes. BBJ_GENE_Blood and BBJ_EXON_Blood included over 20,000 genes and thirty thousand SNPs and a hundred thousand SNPs, respectively.
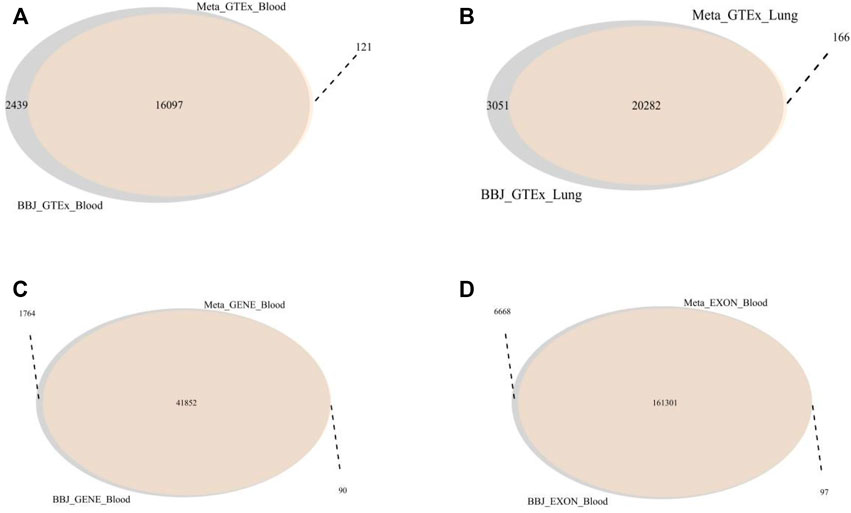
FIGURE 3. The overlapping genes between two different SMR datasets. (A) The overlap genes of Meta_GTEx_Blood and BBJ_GTEx_Blood. 16,097 genes were overlapped. (B) The overlap genes of the Meta_GTEx_Lung and BBJ_GTEx_Lung. There were 20,282 overlapped genes. (C) The overlap genes of Meta_GENE_Blood and BBJ_GENE_Blood. These two datasets have 41,852 identical genes. (D) the overlap genes of Meta_EXON_Blood and BBJ_EXON_Blood. These two datasets have 161,301 identical genes.
Identification of Genes Associated With Lung Carcinoma
The Z values of GWAS and eQTL were calculated by the TSMR formula. After the Chi-squared test, TSMR values were transferred to PSMR (Figure 4). Then we performed FDR on PSMR to obtain Padj values. Finally, we filtered Padj values not less than 5%. As a result, two Padj values corresponding to different SNP susceptibility loci (rs8042849 and rs931794) were screened out (Table 2). These two SNP loci might regulate gene expression and hence cause the occurrence and development of cancer. Both loci were relevant to the expression of PSMA4 gene.
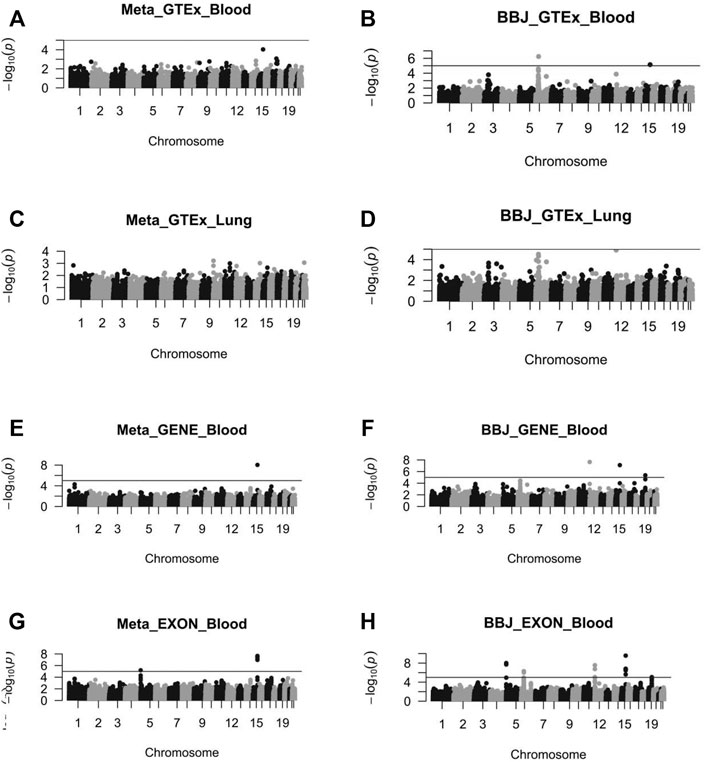
FIGURE 4. The Manhattan diagram of these eight SMR analysis results. (A) the SMR analysis results of Meta_GTEx_Blood. (B) the SMR analysis results of BBJ_GTEx_Blood. (C) the SMR analysis results of Meta_GTEx_Lung. (D) the SMR analysis results of BBJ_GTEx_Lung. (E) the SMR analysis results of Meta_GENE_Blood. (F) the SMR analysis results of BBJ_GENE_Blood. (G) the SMR analysis results of Meta_EXON_Blood. (H) the SMR analysis results of BBJ_EXON_Blood.
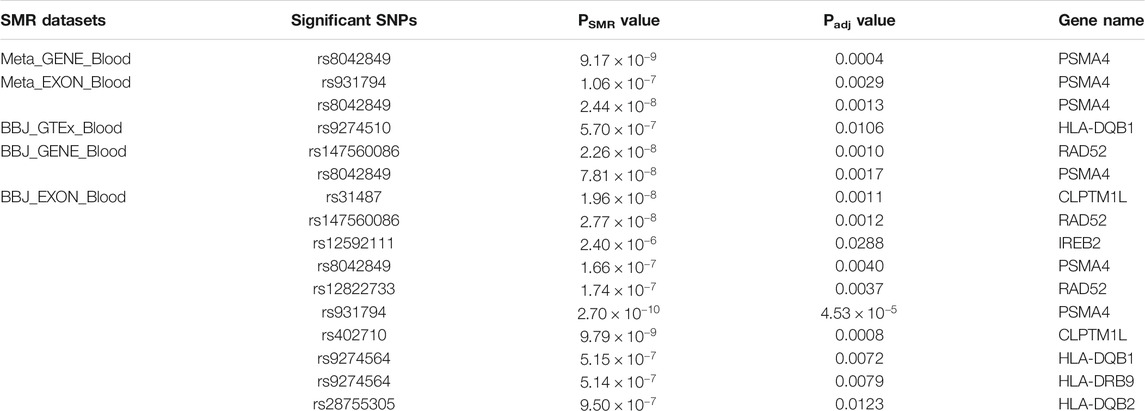
TABLE 2. The list of discovered lung cancer causative genes by the SMR analysis. This list showed the significant SNPs and genes found in different datasets, and the corresponding value of PSMR and Padj calculated.
The analysis of four SMR datasets from Asian populations identified a total of 10 SNP significant sites, most of which were repeatedly identified in these SMR analyses. The expression of seven genes was influenced by these 10 SNPs. The seven genes were comprised of HLA-DQB1, CLPTM1L, RAD52, IREB2, PSMA4, HLA-DRB9, and HLA-DQB2. According to the results, PSMA4 was recognized again. Furthermore, no significant susceptibility sites were found in the association analysis of GWAS data and lung eQTL data.
Gene Function Analysis
To further explain the potential regulatory mechanism of gene expression on lung cancer susceptibility, we conducted the analysis of gene function and involved biological pathways on these seven genes on the Kyoto Encyclopedia of Genes and Genomes (KEGG) database. According to the analysis results, PSMA4 is an essential subunit of the proteasome protein. The proteasome is a large protein complex and is the key to regulating cell function cellular functions, such as cell differentiation, cell cycle (Voutsadakis, 2017). Many studies have shown that the proteasome plays an important role in the development and progression of various tumors, including lung cancer. In addition, three of the seven genes were the members of the Major Histocompatibility Complex (MHC) gene family. Human Leukocyte Antigen (HLA) stands for human MHC, The HLA gene family is divided into three subgroups, which encode three types of molecules: MHC class I molecules, MHC class II molecules, and MHC class III molecules. These three genes were of the MHC class II. When the external invaders are engulfed and processed by lysosome to be fragmented, the MHC class II molecules bind to these fragments and present them on the cell surface for T cells to recognize. The study indicates that HLA class II genes on tumor cells impact tumor immunogenicity (Chen et al., 2019).
Moreover, the CLPTM1L gene can encode cleft lip and palate transmembrane protein 1-Like protein. Recent research shows that the SNPs of CLPTM1L were associated with many human malignancies, especially in some highly aggressive and metastatic cancers (Shete et al., 2020). RAD52 function on the recombination repair of double strand breaks (DSB) is important for maintaining chromosome integrity. Based on the cause of cell death by DSB unrepair, cancer cells have a higher DSB burden, and hence the relationship between DSB and RAD52 can be exploited for cancer treatment (Trenner and Sartori, 2019). IREB2 gene can encode an iron-responsive element-binding protein that is an RNA-binding protein and can bind to iron response elements (IRES) for the regulation of the translation and stability of mRNAs in cells. Variants in the gene have been linked to lung cancer and chronic obstructive pulmonary disease (COPD). These results further proved this inference of our analysis. Accordingly, the analysis of the relationship between these genes and lung cancer can provide an important theoretical basis for revealing the genetic mechanism behind lung cancer.
Discussion
We applied multi-omics analysis to integrate two of the GWAS datasets and four of eQTL datasets based on the SMR method, resulting in the functionally important genes related-lung cancer being found. SMR research can facilitate more accurate and effective identification of the potential causal relationship between exposure and outcome, for disease risk factors had a further understanding. Once risk factors have been successfully discovered, they can be prevented or targeted for cancer treatment. By analyzing the causal genes associated with lung cancer, we recognized seven genes associated with lung cancer, suggesting a possible mechanism for the development of lung cancer by altering the expression of risk genes.
With the analysis of these SMR datasets, the PSMA4 gene was repeatedly identified. It has been reported that down-regulation of proteasome subunit encoding gene PSMA4 reduces proteasome activity (Wang et al., 2007). The proteasome is a large protein complex that can degrade various cellular proteins rapidly and timely. The proteasome is responsible for regulating many cellular processes, including transcription, cell cycle progression, and apoptosis (Cardoso et al., 2004). Proteasome dysfunction leads to many diseases including cancer. Drugs that inhibit proteasome activity directly affect the susceptibility of lung cancer by regulating cell proliferation and apoptosis. Additionally, PSMA4 is involved in cancer cell proliferation, also its polymorphisms have been shown to increase the risk of lung cancer in the Chinese Han population (Wang et al., 2015). Based on the above information, we predicted that PSMA4 may govern cell proliferation and apoptosis, and hence can promote the proliferation of lung cancer cells.
Additionally, HLA-DQB1, HLA-DRB9, and HLA-DQB2 are all homologs of HLA class II molecules, which are essential for immune response. HLA plays a key role in the interaction between tumor cells and the human immune system. HLA II molecules are involved in anti-tumor immunity and exogenous antigen presentation by CD4+ T cells. There is evidence that HLA II on tumor cells affects tumor migration and invasion, cancer progression, immune response and prognosis of a variety of malignancies in vitro and in vivo. For example, some studies have shown that tumor HLA-DQB1 level is associated with the recurrence free survival (RFS) of early Lung Adenocarcinoma, and the mechanism may be related to anti-tumor immunity (Zhang et al., 2019). Currently, there is no definitive study on the relationship between HLA-DRB9, HLA-DQB2 and lung cancer, and further verification will be needed.
CLPTM1L is a mitochondrial protein and is thought to be involved in lung cancer. Some studies have found that the expression of CLPTM1L is significantly increased in lung cancer tissues compared with normal tissues by immunohistochemistry, especially in lung adenocarcinoma (Ni et al., 2012). Also, the researchers found that increased copy number in the CLPTM1L region is the most common genetic event in the early stages of NSCLC (James et al., 2012). Some GWAS data illustrate that the region of CLPTM1L gene is associated with lung cancer. Therefore, the gene expression of CLPTM1L is closely related to lung tumor susceptibility.
Moreover, according to the GWAS results, the SNPs of RAD52 gene were associated with increased risk of lung cancer, particularly the development of lung squamous cell carcinoma (Shi et al., 2012). As the development of lung squamous cell carcinoma is closely associated with smoking, DNA repair is increased in the lung tissue of smokers (Lieberman et al., 2017). RAD52 encoded gene product can bind to the single stranded DNA terminal and can be involved in homologous recombination and DNA repair in mammals. To sum up, the relationship between RAD52 gene expression and the occurrence of lung cancer may be due to its DNA repair function in lung tissue.
The iron-binding protein encoded by IREB2 is involved in maintaining iron homeostasis in human cells. IREB2 is located in the susceptibility locus of lung cancer. A previous study showed that iron levels in the lungs increase with age, with higher concentrations in the lungs of smokers. Therefore, abnormal IREB2 expression or function may lead to iron metabolism disorder. Iron load leads to cell proliferation in cancer cell lines, and cancer cell lines have a poor ability to regulate IREB2 expression (Khiroya et al., 2017). In addition, GWAS results illustrate that IREB2 has been shown to be associated COPD and the expression of its protein product IRP2 is altered in lung cancer patients carrying lung cancer (Fehringer et al., 2012). The role of IRP2 is to control iron levels in cells by regulating the input, output and storage of various iron proteins. Therefore, the misexpression of IREB2 gene may alter intracellular iron levels and hence lead to the occurrence of malignant tumors. If IRP2 gene can be targeted therapeutically, it may provide a new approach for lung cancer treatment.
In summary, our study integrated genomic and transcriptome information and found seven genes associated with lung cancer by the SMR method, revealing possible regulatory mechanisms that alter the expression of these genes and further pathogenicity. This provides a research direction for understanding the precise treatment and targeted drug design of lung cancer. Although the SMR method can avoid the interference of confounding effect and reverse causal association in the study, and can be not limited by the sample size, thus producing more reliable results, this method can only preliminarily judge that the gene expression may affect the development of lung cancer, and cannot show how gene expression regulates the onset and progression of this disease. It takes experiments and other analytical methods to confirm a causal link between genes and disease. In the following study, we will continue to use SMR analysis method to integrate GWAS and other types of QTL data to mine more genes affecting lung cancer.
Data Availability Statement
The original contributions presented in the study are included in the article/Supplementary Material, further inquiries can be directed to the corresponding author.
Author Contributions
NC wrote this manuscript. XC did experiments. CC collected data. CL helped revise this manuscript. JH designed the whole work.
Conflict of Interest
The authors declare that the research was conducted in the absence of any commercial or financial relationships that could be construed as a potential conflict of interest.
Publisher’s Note
All claims expressed in this article are solely those of the authors and do not necessarily represent those of their affiliated organizations, or those of the publisher, the editors and the reviewers. Any product that may be evaluated in this article, or claim that may be made by its manufacturer, is not guaranteed or endorsed by the publisher.
References
Barr Kumarakulasinghe, N., Zanwijk, N. v., and Soo, R. A. (2015). Molecular Targeted Therapy in the Treatment of Advanced Stage Non-small Cell Lung Cancer (NSCLC). Respirology 20 (3), 370–378. doi:10.1111/resp.12490
Bray, F., Ferlay, J., Soerjomataram, I., Siegel, R. L., Torre, L. A., and Jemal, A. (2018). Global Cancer Statistics 2018: GLOBOCAN Estimates of Incidence and Mortality Worldwide for 36 Cancers in 185 Countries. CA: a Cancer J. clinicians 68 (6), 394–424. doi:10.3322/caac.21492
Cardoso, F., Ross, J. S., Piccart, M. J., Sotiriou, C., and Durbecq, V. (2004). Targeting the Ubiquitin-Proteasome Pathway in Breast Cancer. Clin. Breast Cancer 5 (2), 148–157. doi:10.3816/cbc.2004.n.020
Chen, B., Khodadoust, M. S., Olsson, N., Wagar, L. E., Fast, E., Liu, C. L., et al. (2019). Predicting HLA Class II Antigen Presentation through Integrated Deep Learning. Nat. Biotechnol. 37 (11), 1332–1343. doi:10.1038/s41587-019-0280-2
Fehringer, G., Liu, G., Pintilie, M., Sykes, J., Cheng, D., Liu, N., et al. (2012). Association of the 15q25 and 5p15 Lung Cancer Susceptibility Regions with Gene Expression in Lung Tumor Tissue. Cancer Epidemiol. Biomarkers Prev. 21 (7), 1097–1104. doi:10.1158/1055-9965.epi-11-1123-t
Hartwig, F. P., and Davies, N. M. (2016). Why Internal Weights Should Be Avoided (Not Only) in MR-Egger Regression. Int. J. Epidemiol. 45 (5), 1676–1678. doi:10.1093/ije/dyw240
James, M. A., Wen, W., Wang, Y., Byers, L. A., Heymach, J. V., Coombes, K. R., et al. (2012). Functional Characterization of CLPTM1L as a Lung Cancer Risk Candidate Gene in the 5p15.33 Locus. PloS one 7 (6), e36116. doi:10.1371/journal.pone.0036116
Khiroya, H., Moore, J. S., Ahmad, N., Kay, J., Woolnough, K., Langman, G., et al. (2017). IRP2 as a Potential Modulator of Cell Proliferation, Apoptosis and Prognosis in Nonsmall Cell Lung Cancer. Eur. Respir. J. 49 (4). doi:10.1183/13993003.00711-2016
Ko, D. C., and Urban, T. J. (2013). Understanding Human Variation in Infectious Disease Susceptibility through Clinical and Cellular GWAS. Plos Pathog. 9 (8), e1003424. doi:10.1371/journal.ppat.1003424
Lamberti, G., Andrini, E., Sisi, M., Rizzo, A., Parisi, C., Di Federico, A., et al. (2020). Beyond EGFR, ALK and ROS1: Current Evidence and Future Perspectives on Newly Targetable Oncogenic Drivers in Lung Adenocarcinoma. Crit. Rev. Oncology/Hematology 156, 103119. doi:10.1016/j.critrevonc.2020.103119
Landi, M. T., Chatterjee, N., Yu, K., Goldin, L. R., Goldstein, A. M., Rotunno, M., et al. (2009). A Genome-wide Association Study of Lung Cancer Identifies a Region of Chromosome 5p15 Associated with Risk for Adenocarcinoma. Am. J. Hum. Genet. 85 (5), 679–691. doi:10.1016/j.ajhg.2009.09.012
Lieberman, R., Pan, J., Zhang, Q., and You, M. (2017). Rad52 Deficiency Decreases Development of Lung Squamous Cell Carcinomas by Enhancing Immuno-Surveillance. Oncotarget 8 (21), 34032–34044. doi:10.18632/oncotarget.16371
Ni, Z., Tao, K., Chen, G., Chen, Q., Tang, J., Luo, X., et al. (2012). CLPTM1L Is Overexpressed in Lung Cancer and Associated with Apoptosis. PloS one 7 (12), e52598. doi:10.1371/journal.pone.0052598
Oser, M. G., Niederst, M. J., Sequist, L. V., and Engelman, J. A. (2015). Transformation from Non-small-cell Lung Cancer to Small-Cell Lung Cancer: Molecular Drivers and Cells of Origin. Lancet Oncol. 16 (4), e165–e172. doi:10.1016/s1470-2045(14)71180-5
Rashkin, S. R., Graff, R. E., Kachuri, L., Thai, K. K., Alexeeff, S. E., Blatchins, M. A., et al. (2020). Pan-cancer Study Detects Genetic Risk Variants and Shared Genetic Basis in Two Large Cohorts. Nat. Commun. 11 (1), 4423–4514. doi:10.1038/s41467-020-18246-6
Reel, P. S. (2021). Using Machine Learning Approaches for Multi-Omics Data Analysis: A Review. Biotechnol. Adv.. doi:10.1016/j.biotechadv.2021.107739
Rojano, E., Ranea, J. A., and Perkins, J. R. (2016). Characterisation of Non-coding Genetic Variation in Histamine Receptors Using AnNCR-SNP. Amino acids 48 (10), 2433–2442. doi:10.1007/s00726-016-2265-5
Rudin, C. M., Brambilla, E., Faivre-Finn, C., and Sage, J. (2021). Small-cell Lung Cancer. Nat. Rev. Dis. Primers 7 (1), 3–20. doi:10.1038/s41572-020-00235-0
Sakaue, S., Kanai, M., and Tanigawa, Y. (2021). A Global Atlas of Genetic Associations of 220 Deep Phenotypes. medRxiv. doi:10.1101/2020.10.23.20213652
Shabalin, A. A. (2012). Matrix eQTL: Ultra Fast eQTL Analysis via Large Matrix Operations. Bioinformatics 28 (10), 1353–1358. doi:10.1093/bioinformatics/bts163
Shete, S., Liu, H., Wang, J., Yu, R., Sturgis, E. M., Li, G., et al. (2020). A Genome-wide Association Study Identifies Two Novel Susceptible Regions for Squamous Cell Carcinoma of the Head and Neck. Cancer Res. 80 (12), 2451–2460. doi:10.1158/0008-5472.can-19-2360
Shi, J., Chatterjee, N., Rotunno, M., Wang, Y., Pesatori, A. C., Consonni, D., et al. (2012). Inherited Variation at Chromosome 12p13.33, Including RAD52, Influences the Risk of Squamous Cell Lung Carcinoma. Cancer Discov. 2 (2), 131–139. doi:10.1158/2159-8290.cd-11-0246
Tanaka, E., Uchida, D., Shiraha, H., Kato, H., Ohyama, A., Iwamuro, M., et al. (2020). Promising Gene Therapy Using an Adenovirus Vector Carrying REIC/Dkk-3 Gene for the Treatment of Biliary Cancer. Cgt 20 (1), 64–70. doi:10.2174/1566523220666200309125709
Tarwadi., T., Jazayeri, J. A., Pambudi, S., Arbianto, A. D., Rachmawati, H., Kartasasmita, R. E., et al. (2020). In-silico Molecular Interaction of Short Synthetic Lipopeptide/Importin-Alpha and In-Vitro Evaluation of Transgene Expression Mediated by Liposome- Based Gene Carrier. Cgt 20, 383–394. doi:10.2174/1566523220666201005104224(
Trenner, A., and Sartori, A. A. (2019). Harnessing DNA Double-Strand Break Repair for Cancer Treatment. Front. Oncol. 9, 1388. doi:10.3389/fonc.2019.01388
Voutsadakis, I. A. (2017). Proteasome Expression and Activity in Cancer and Cancer Stem Cells. Tumour Biol. 39 (3), 1010428317692248. doi:10.1177/1010428317692248
Wang, T., Chen, T., Thakur, A., Liang, Y., Gao, L., Zhang, S., et al. (2015). Association of PSMA4 Polymorphisms with Lung Cancer Susceptibility and Response to Cisplatin-Based Chemotherapy in a Chinese Han Population. Clin. Transl Oncol. 17 (7), 564–569. doi:10.1007/s12094-015-1279-x
Wang, W., Spitz, M. R., Yang, H., Lu, C., Stewart, D. J., and Wu, X. (2007). Genetic Variants in Cell Cycle Control Pathway Confer Susceptibility to Lung Cancer. Clin. Cancer Res. 13 (19), 5974–5981. doi:10.1158/1078-0432.ccr-07-0113
Wang, Y. (2021). A Novel Method for Mendelian Randomization Analyses with Pleiotropy and Linkage Disequilibrium in Genetic Variants from Individual Data. Front. Genet. 12, 394. doi:10.3389/fgene.2021.634394
Zakaria, N., Yusoff, N. M., Zakaria, Z., Lim, M. N., Baharuddin, P. J., Fakiruddin, K. S., et al. (2015). Human Non-small Cell Lung Cancer Expresses Putative Cancer Stem Cell Markers and Exhibits the Transcriptomic Profile of Multipotent Cells. BMC cancer 15 (1), 84–16. doi:10.1186/s12885-015-1086-3
Zhang, L., Li, M., Deng, B., Dai, N., Feng, Y., Shan, J., et al. (2019). HLA-DQB1 Expression on Tumor Cells Is a Novel Favorable Prognostic Factor for Relapse in Early-Stage Lung Adenocarcinoma. Cmar Vol. 11, 2605–2616. doi:10.2147/cmar.s197855
Zhao, T., Hu, Y., and Cheng, L. (2021). Deep-DRM: a Computational Method for Identifying Disease-Related Metabolites Based on Graph Deep Learning Approaches. Brief Bioinform 22 (4), bbaa212. doi:10.1093/bib/bbaa212
Zhao, T., Hu, Y., Peng, J., and Cheng, L. (2020). DeepLGP: a Novel Deep Learning Method for Prioritizing lncRNA Target Genes. Bioinformatics 36 (16), 4466–4472. doi:10.1093/bioinformatics/btaa428
Zhao, T., Hu, Y., Zang, T., and Wang, Y. (2019). Integrate GWAS, eQTL, and mQTL Data to Identify Alzheimer's Disease-Related Genes. Front. Genet. 10, 1021. doi:10.3389/fgene.2019.01021
Zhernakova, D. V., Deelen, P., Vermaat, M., van Iterson, M., van Galen, M., Arindrarto, W., et al. (2017). Identification of Context-dependent Expression Quantitative Trait Loci in Whole Blood. Nat. Genet. 49 (1), 139–145. doi:10.1038/ng.3737
Keywords: lung cancer, susceptibility genes, GWAS, eQTL, SMR
Citation: Cheng N, Cui X, Chen C, Li C and Huang J (2021) Exploration of Lung Cancer-Related Genetic Factors via Mendelian Randomization Method Based on Genomic and Transcriptomic Summarized Data. Front. Cell Dev. Biol. 9:800756. doi: 10.3389/fcell.2021.800756
Received: 24 October 2021; Accepted: 22 November 2021;
Published: 06 December 2021.
Edited by:
Lei Deng, Central South University, ChinaReviewed by:
Yuansong Zhao, University of Texas Health Science Center at Houston, United StatesWeiyue Ding, Harvard Medical School, United States
Copyright © 2021 Cheng, Cui, Chen, Li and Huang. This is an open-access article distributed under the terms of the Creative Commons Attribution License (CC BY). The use, distribution or reproduction in other forums is permitted, provided the original author(s) and the copyright owner(s) are credited and that the original publication in this journal is cited, in accordance with accepted academic practice. No use, distribution or reproduction is permitted which does not comply with these terms.
*Correspondence: Jingyu Huang, huangjingyu1009@163.com