- 1Department of Dermatology, Central Hospital Affiliated to Shandong First Medical University, Jinan, China
- 2Department of Gastroenterological Surgery, Shandong Provincial Hospital, Cheeloo College of Medicine, Shandong University, Jinan, China
- 3Medical Science and Technology Innovation Center, Shandong First Medical University and Shandong Academy of Medical Sciences, Jinan, China
- 4Department of Gastroenterological Surgery, The First Affiliated Hospital of Shandong First Medical University, Jinan, China
- 5Department of Respiratory and Critical care, Shandong public health clinical center, Jinan, China
- 6Department of Oncology, Shandong Provincial Hospital Affiliated to Shandong First Medical University, Jinan, China
- 7Department of Dermatology, Shandong Provincial Hospital Affiliated to Shandong First Medical University, Jinan, China
- 8Outpatient of Podiatric Rehabilitation, Maternity and Child Health Care of Zaozhuang, Zaozhuang, China
- 9Department of Dermatology, Qilu Hospital, Shandong University, Jinan, China
- 10Department of General Surgery, Xuanwu Hospital, Capital Medical University, Beijing, China
RNA N6-methyladenosine (m6A) modification in tumorigenesis and progression has been highlighted and discovered in recent years. However, the molecular and clinical implications of m6A modification in melanoma tumor microenvironment (TME) and immune infiltration remain largely unknown. Here, we utilized consensus molecular clustering with nonnegative matrix factorization based on the melanoma transcriptomic profiles of 23 m6A regulators to determine the m6A modification clusters and m6A-related gene signature. Three distinct m6A modification patterns (m6A-C1, C2, and C3), which are characterized by specific m6A regulator expression, survival outcomes, and biological pathways, were identified in more than 1,000 melanoma samples. The immune profile analyses showed that these three m6A modification subtypes were highly consistent with the three known immune phenotypes: immune-desert (C1), immune-excluded (C2), and immune-inflamed (C3). Tumor digital cytometry (CIBERSORT, ssGSEA) algorithm revealed an upregulated infiltration of CD8+ T cell and NK cell in m6A-C3 subtype. An m6A scoring scheme calculated by principal component of m6A signatures stratified melanoma patients into high- and low-m6sig score subgroups; a high score was significantly associated with prolonged survival and enhanced immune infiltration. Furthermore, fewer somatic copy number alternations (SCNA) and PD-L1 expression were found in patients with high m6Sig score. In addition, patients with high m6Sig score demonstrated marked immune responses and durable clinical benefits in two independent immunotherapy cohorts. Overall, this study indicated that m6A modification is involved in melanoma tumor microenvironment immune regulation and contributes to formation of tumor immunogenicity. Comprehensive evaluation of the m6A modification pattern of individual tumors will provide more insights into molecular mechanisms of TME characterization and promote more effective personalized biotherapy strategies.
Introduction
Methylation of N6 adenosine (m6A) is a revisable RNA modification process that is widely present in various types of common RNAs, such as mRNAs, lncRNAs, and miRNAs, and essential for a variety of physiological processes and disease progression (Zhao et al., 2017; Zaccara et al., 2019). The m6A modification is manipulated by three regulatory proteins, methyltransferases (“writers”), demethylases (“erasers”), and binding proteins (“reader”), and this modification process is dynamic and reversible (He et al., 2019). Increasing evidence has identified the important roles m6A modifications play in various cellular processes and in cancer progression through regulating RNA stability, mRNA splicing and translation, and microRNA processing (Li et al., 2019; Chen et al., 2020a). Meanwhile, a large number of studies have shown that the process of tumor development and abnormal immune regulation of the body are associated with abnormal expression of m6A-modified regulatory proteins (Chen et al., 2019a; Shulman and Stern-Ginossar, 2020; Wang et al., 2020). Therefore, systematic and comprehensive explanation of tumor heterogeneity brought about by genetic variation and epigenetic regulation will facilitate the development and advancement of new therapeutic technologies based on RNA methylation (Martínez-Riaño et al., 2019).
Malignant melanoma is a highly metastatic cancer caused by abnormal transformation of pigment cells and melanocytes resulting from prolonged exposure to ultraviolet radiation (Mazurkiewicz et al., 2021). Since melanoma is curable in its initial stages, early diagnosis of this disease is crucial (Eddy and Chen, 2020). Global data show that patients with melanoma who develop metastases have a 5-years survival rate of only 25% due to the difficulty of treatment (Eddy and Chen, 2020). Multiple novel targeted therapies targeting melanoma-specific markers have been developed in recent years; however, most patients often show lower effectiveness or shorter duration to these treatments (Mazurkiewicz et al., 2021). Among the multiple factors that influence treatment outcome, the tumor microenvironment might account for a major cause in the melanoma progression. The composition of the microenvironment in melanoma is relatively complex, which includes adventitial cells (keratin-forming cells, cancer-associated fibroblasts CAF, adipocytes and infiltrating immune cells), extracellular matrix components, and tumor-specific physicochemical properties (Mazurkiewicz et al., 2021). With the increased understanding of the tumor microenvironment, the key immune cell subsets in tumorigenesis and metastasis were also gradually recognized. The evaluation of immune infiltration based on the characteristics of TME was supposed as a key technique to infer the pre-existing antitumor immunity and predict patient response to immune checkpoint inhibitor therapy (Binnewies et al., 2018; Galon and Bruni, 2019; Li et al., 2020a). Recently, the new concept of “immune context” on tumor, which classifies the TME characteristics of melanoma into three categories, i.e., hot, excluded, and cold, also implies three different types of effective treatment options (Hegde et al., 2016; Chen and Mellman, 2017). In summary, systematic and comprehensive dissection of the components of the tumor microenvironment of melanoma and thus identification of the corresponding tumor immune phenotype is a feasible and reliable means to guide immunotherapy and predict the effectiveness of immunotherapy (Mariathasan et al., 2018; Pagès et al., 2018).
Recent studies suggest an association between TME immune cell infiltration and m6A modification; however, this does not appear to be fully explained by RNA degradation mechanisms (Zhao et al., 2017; Chen et al., 2019a; He et al., 2019). It has been reported that YTHDF1 can promote lysozyme in dendritic cells to regulate the degradation of tumor neoantigens, and the key to this process is that YTHDF1 can accurately recognize the m6A modification process of tumor neoantigens and enhance their translation level (Han et al., 2019). When YTHDF1 is absent in dendritic cells, this leads to enhanced cross-presentation of antigens and enhanced cross-stimulation of CD8+ T cells. FTO has been reported to be associated with cytotoxic effects in colon cells by inhibiting YTHDF2-mediated RNA decay, which in turn promotes PD-1, CXCR4, and SOX10, and suppresses interferon-gamma (IFN-γ) expression (Yang et al., 2019). This result was confirmed in an in vitro experiment. When FTO is knocked down exogenously, IFN-γ is substantially upregulated, which in turn makes colon cancer mice sensitive to anti-PD-1 drug treatment. METTL3, which also regulates mRNA m6A modifications, regulates the dynamic balance of CD40, CD80, and Snail (Lewinska et al., 2017; Wang et al., 2019). Unfortunately, due to the unsophisticated nature of the current technology, the studies mentioned are all on one or two m6A regulatory molecules, and the antitumor effects produced by these regulatory molecules are not the contribution of one or several molecules, but rather they work together to regulate the m6A modification process in the body and thus affect cancer development and metastasis. Fortunately, the explosive growth on transcriptomics and genomics sequencing database provides a rich resource for a comprehensive and integrated analysis of the role of m6A-related molecules in cancer and immune regulation (Finotello and Trajanoski, 2018; Chen et al., 2020b). Thus, deepening our understanding of cancer immunity and developing new targets for cancer immunotherapy requires a systematic and comprehensive dissection of the TME immune cell infiltration profile regulated by m6A-related molecules.
In this study, we integrated the transcriptome and genome sequencing data from 1,020 melanoma samples across TCGA and GEO databases, and systematically analyzed and discovered the direct and specific association between m6A modification patterns and TME immune cell infiltration features in melanoma. Using non-negative matrix factorization (NMF) clustering analysis, we identified three novel m6A modification patterns with TME features highly consistent with three previously reported immune phenotypes: immune inflammatory, immune rejection, and immune desert phenotypes (Chen and Mellman, 2017). Not only that, we quantified the m6A modification clusters of individualized tumors in the form of scores, which can be used to predict the effectiveness of patients to ICI therapy. Our findings suggest that m6A modifications play a crucial role in tumor immune microenvironment signature formation and melanoma treatment planning.
Materials and Methods
Collect and Preprocess of Publicly Attainable Expression Datasets
Gene expression data and clinical information for melanoma patient samples were obtained from the GEO database (https://www.ncbi.nlm.nih.gov/geo/) and TCGA database (https://portal.gdc.cancer.gov/), which are publicly and freely available. We screened the melanoma dataset and eventually included a total of 1,020 patients in the study for subsequent analysis, including the GSE19234, GSE22154, GSE50509, GSE59455, GSE65904, GSE22153, GSE54437, and TCGA-SCKM datasets. For data pre-processing, we downloaded the “CEL” files from the GEO database, relying on the “affy” and “simpleaffy” R packages for background correction and normalization, while the RNA sequencing data from the TCGA database is downloaded in normalized FPKM format and then converted to transcripts per kilobase million (TPM) format. With reference to previous experience, the “ComBat” method of the “sva” R package was used to reduce the batch effect between different datasets, which was mainly a problem for datasets from the GEO database (Dai et al., 2018). Both somatic mutation data and copy number variation data of TCGA-SCKM were curated from the UCSC Xena database and Davoli et al. (Davoli et al., 2017). The copy number variation of 23 m6A regulators in human chromosomes was mapped by the “RCircos” R package. For non-synonymous mutations such as frameshift mutations, inflammatory mutations, missense mutations, nonsense mutations, and splice site mutations, numbers represent the tumor mutational load (TML). Supplementary Table S1 presents the clinical information of the samples from the meta-GEO and TCGA-SKCM databases.
Nonnegative Matrix Factorization Clustering Analysis of 23 m6A Regulators
A literature review of m6A methylation modifications revealed that there are now 23 recognized m6A regulators, which constitute the modification pattern of m6A methylation (Zhao et al., 2017; Chen et al., 2019a; He et al., 2019; Zaccara et al., 2019). Specifically, eight writers include CBLL1, KIAA1429, METTL14, METTL3, RBM15, RBM15B, WTAP, and ZC3H13; two erasers include ALKBH5 and FTO; 13 readers include ELAVL1, FMR1, HNRNPA2B1, HNRNPC, IGF2BP1, IGF2BP2, IGF2BP3, LRPPRC, YTHDC1, YTHDC2, YTHDF1, YTHDF2, and YTHDF3; and 23 of them shared the key task of m6A methylation modification. Using non-negative matrix decomposition (NMF), we performed a clustering analysis of the 23 m6A regulators based on their expression, which could identify different types of m6A modification patterns. The expression matrix A of the 23 m6A regulators was first split into non-negative matrices W and H, as A ≈ WH, and then the matrix A was subjected to repeated factorization, and finally the output data was summarized, which gave the clustering results of the melanoma samples. It is crucial to consider factors such as covariance, dispersion, and silhouette coefficient to determine the optimal number of clustering groups. The “NMF” R package to perform the clustering analysis used the “brunet” and “200 nruns” algorithms.
Functional Analysis and Annotation
The Hallmarker gene set (Subramanian et al., 2005) and Mariathasan et al. (Mariathasan et al., 2018) constructed gene set were used as well-defined biometric backgrounds for gene set variation analysis (GSVA) with “GSVA” R package (Hänzelmann et al., 2013), which was designed to explore the variation in biological processes across different m6A modification patterns. In the gene ontology (GO) analysis, we annotated the functions of 23 m6A regulators under three entries of cellular component (CC), molecular function (MF), and biological process (BP), which was done using the “clusterProfiler” R package. For GSVA and GO analysis, the cut-off value was set to a false discovery rate (FDR) < 0.01.
Estimation of Immune Cell Infiltration
In quantifying the relative abundance of 28 immune cell types curated by Charoentong et al. in the tumor microenvironment, we refer to recent studies using the single sample gene enrichment analysis (ssGSEA) method, which well marks the specific functional gene panels of each immune cell type (Charoentong et al., 2017; Jia et al., 2018). As in the previous study (Chen et al., 2020a), we expressed the relative abundance of various immune cell types in the form of enrichment scores, and they were normalized to a uniform distribution from 0 to 1. In terms of biosimilarity, infiltrating immune cells were evaluated and acted upon using multidimensional scaling (MDS) and Gaussian fitting models, and moreover, the deconvolution approach CIBERSORT (Newman et al., 2019) (http://cibersort.stanford.edu/) was then used to estimate the abundance of 22 different subpopulations of leukocytes, which have melanoma gene expression profiles.
Quantification of Immune Response Predictor
T cell-inflamed gene expression profile (GEP) is a superior predictor of response to anti-PD-1 regimens, which contained IFN-γ-responsive genes related to antigen presentation, cytotoxic activity, and adaptive immune resistance (Ayers et al., 2017). The T cell-inflamed scores were calculated and weighted by averaging of the included genes for the IFN-γ (6-gene) and expanded immune (18-gene) signatures. In modeling different types of tumor immune evasion mechanisms, we drew on the Tumor Immune Dysfunction and Exclusion (TIDE) algorithm proposed by Jiang et al. (Jiang et al., 2018). This algorithm integrates the dysfunction of tumor-infiltrating toxic T lymphocytes (CTLs) and rejection of CTLs by immunosuppressive factors. The higher TIDE score implies greater chance of immune escape of tumor cells and represents a possible poor outcome of treatment with ICIs. The method of Estimation of Stromal and Immune cells in MAlignant Tumor tissues using Expression data (ESTIMATE) (Yoshihara et al., 2013) was adopted to calculate the immune score of tumors, and this algorithm can be better based on transcriptional profiles to estimate the cellularity of the tumor and the purity of the tumor. The level of infiltrating immune and stromal cells is the basis for tumor purity, which is predicted by the immune score of the tumor. In detail, a high immune score of a tumor is an indication of a high infiltration of immune cells in the tumor tissue, or a low tumor purity.
Capture of Significantly Mutated Genes and Tumor Mutation Features
The MutSigCV algorithm was used to identify significantly mutated genes (SMGs) (Lawrence et al., 2013; Chen et al., 2019b), which takes into account the specific background mutation rate in the mutation context before evaluating the significant enrichment of non-resting somatic mutations in a gene. We considered q < 0.1 as statistically significant, and these genes needed to be certified in the Cancer Cell Line Encyclopedia of Humans (CCLE) (Ghandi et al., 2019) to be defined as SMGs (Chen et al., 2020c) (Supplementary Table S2). The “maftools” R package (Mayakonda et al., 2018) was used to characterize genes in the TCGA-SKCM cohort that underwent m6A modification, the mutation details of SMGs, and the capture of mutational features in the genomic data. The ExtractSignatures function based on Bayesian variation non-negative matrix decomposition was used for model construction; specifically, using this function, we split the mutation portrait matrix into two non-negative matrices and noted as “signature” and “contribution,” where “signature” represents the mutation process and “contribution” represents the corresponding mutation activities (Chong et al., 2021a). Better still, the SignatureEnrichment function allows determining the optimal number of extracted mutation features and assigning them appropriately to each sample. For comparison and annotation, using the Catalogue of Somatic Mutations in Cancer (COSMIC) (Kandoth et al., 2013) as a reference, we performed a cosine similarity analysis on the extracted melanoma mutation portraits.
Identify Differentially Expressed Genes Between Different m6A Modification Phenotypes
Patients were classified into three clusters of m6A modification patterns using a consensus clustering algorithm, and then the “limma” R package (Ritchie et al., 2015) was used to find differentially expressed genes between groups. Voom normalized data were then subjected to “lmFit” and “eBayes” function algorithms, which in turn allowed the calculation of specific data for differential expression. In this process, we set adjusted p-values <0.001 as statistically significant differences.
Construct the m6Sig Score System
Based on principal component analysis (PCA), we constructed an m6A score system to quantify the level of m6A modifications in specific patients. According to DEGs, they are the intersecting parts of different m6A clusters, and we analyzed the prognostic impact of each gene on melanoma patients with the help of univariate Cox regression models. Deeper feature selection was performed for genes that significantly affect the prognosis of melanoma patients, and this process was computed by the recursive feature elimination (RFE) method of random forest and the 10-fold cross-validation method included in the “caret” R package. Further, we obtained the gene expression profiles based on the above steps, and the principal components 1 and 2 obtained from PCA analysis were the basis of our feature score. The specific formula for this score system is referred to a previous study (Zhang et al., 2020; Chong et al., 2021b), m6Sig score = ∑(PC1i + PC2i).
Collect Genomic and Clinical Information for the ICI Cohort
The gene expression profiles of patients treated with ICI were retrieved in publicly available databases, focusing on matching with clinical information. Ultimately, we included metastatic melanoma treated with PD-1 (nivolumab or pembrolizumab) or PD-1 combined with CTLA-4 (ipilimumab) (Liu et al., 2019), and metastatic urothelial carcinoma (mUC) treated with atezolizumab (anti-PD-L1 mcAb) (Mariathasan et al., 2018) in this study. The gene expression profiles of the samples were converted in TPM format.
Statistical Analyses
All statistical analyses in the study were performed with R 3.6.1. Student’s t-test was performed for quantitative data conforming to a normal distribution, and Wilcoxon rank sum test was performed for non-normally distributed data. When more than two sets of analyses were performed, the nonparametric test was the Kruskal-Wallis test, while the parametric test was the analysis of variance (Hazra and Gogtay, 2016). The Fisher exact test was used for the calculation of contingency rates. Kaplan-Meier survival analysis and Cox regression analysis were performed using the “Survminer” package, and the m6Sig score subgroup stratum was “survival” package with the surv-cutpoint function completed. “timeROC” package completed the evaluation of the m6Sig score model, which plotted the corresponding subject operating characteristic curve (ROC) and calculated the area under the curve (AUC). In analyzing the relationship between patient’s clinical characteristics and the m6Sig score system, multivariate regression models were used to adjust for confounding factors in this. p < 0.05 was considered as statistical significance, and the Benjamini-Hochberg method was used to perform multiple hypothesis testing for false discovery rate (FDR) (Love et al., 2014).
Results
Mapping Genetic Variants of m6A Regulators in Melanoma
In our study, we explored the possible physiological roles of 23 m6A methylation-regulated genes in melanoma, including the “writers” CBLL1, KIAA1429, METTL14, METTL3, RBM15, RBM15B, WTAP, and ZC3H13; the “readers” ELAVL1, FMR1, HNRNPA2B1, HNRNPC, IGF2BP1, IGF2BP2, IGF2BP3, LRPPRC, YTHDC1, YTHDC2, YTHDF1, YTHDF2, and YTHDF3; and the “erasers” ALKBH5 and FTO. These m6A regulators not only recognize, remove, and add m6A modification sites but also, as revealed by GO enrichment analysis and Metascape analysis, can actually alter biological processes, such as regulating mRNA stability, RNA modifications, and RNA metabolism (Figure 1A). Among 467 melanoma patient samples with genomic sequencing, 133 (28.48%) had somatic mutations in m6A regulators, which mainly included missense mutations, nonsense mutations, and code-shifting mutations (Figure 1B). KIAA1429 had the highest mutation frequency, followed closely by IGF2BP1, and the next in the gradient were YTHDC1, LRPPRC, YTHDC2, ZC3H13, YTHDF1, and IGF2BP3. Interestingly, IGF2BP1, ZC3H13, and YTHDF1 had only missense mutations in the relatively high mutation frequencies. Analysis of the co-mutation profiles of the 23 m6A regulators revealed significant co-mutations between FMR1 and IGF2BP1, IGF2BP2 and IGF2BP1, ZC3H13 and LRPPRC, YTHDF3 and RBM15, ALKBH5 and METTL3, and ALKBH5 and FMR1 (Figure 1C). When performing CNV mutation analysis, we concluded that there was widespread CNV amplification in IGF2BP1, YTHDF1, and KIAA1429, while CNV deletion was more widespread in WTAP and RBM15 (Figure 1D). Comparing primary melanoma and metastases, we found that ALKBH5, ELAVL1, FMR1, HNRNPA2B1, HNRNPC, IGF2BP1/2/3, KIAA1429, LRPPRC, RBM15, YTHDC1/2, YTHDF1/3, and ZC3H13 were significantly upregulated in metastases, while RBM15B and METTL3 were significantly upregulated in primary melanoma (Figure 1E). The expression of m6A regulators with aberrant CNV amplification was also upregulated in metastases compared to primary melanoma (ALKBH5, FMR1, HNRNPA2B1, IGF2BP1/2/3, KIAA1429, YTHDC1, YTHDF1/3), and conversely, m6A regulators with aberrant CNV deletion were also downregulated (METTL3, RBM15B, YTHDC2), which are obtained by combining Figure 1D. The m6A regulator network mapped in Figure 1F showed the interaction relationships between 23 molecules that are interconnected and influence each other, which further modulates the prognosis of melanoma patients. This implies that there is a complex and well-organized crossover network between the regulators of writers, readers, and erasers, and this network allows the m6A modification pattern to further refine and take effect, influencing the development and metastasis of melanoma. Using the Spearman correlation test, we found that there is a mutual regulatory relationship between these m6A regulators. Interestingly, ALKBH5 was negatively correlated with most of the m6A regulators, while FMR1 and HNRNPA2B1 were positively correlated with most of them (Supplementary Figure S1A). We further analyzed the association between tumor purity and 23 m6A modification regulators (Figure 1G) and found that most of the m6A regulators were positively correlated with tumor cell purity, whereas WTAP has a negative association, suggesting that WTAP was enriched in non-tumor cell components. Forest plots with Cox regression model were employed to speculate the relationship between m6A regulators and the prognosis of melanoma patients. We found that samples with high expression of WTAP, FMR1, and METTL14 were associated with improved overall survival, while an opposite tendency was observed in RBM15B and ELAVL1 (Supplementary Figure S1B). Taken together, we integrated the genomic and transcriptomic landscapes of m6A regulators in melanoma, and noticed the changes in the expression levels and genetic variation of m6A regulators driving the development and progression of melanoma.
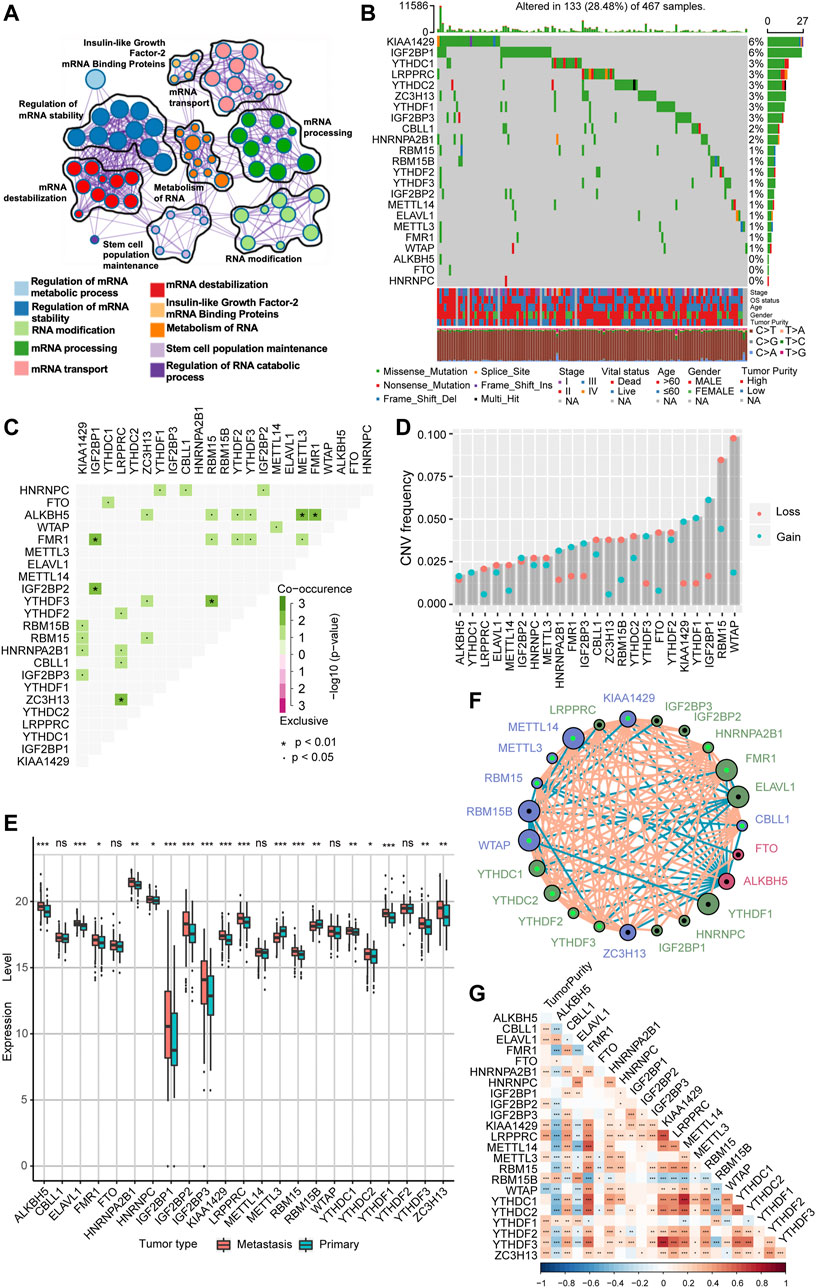
FIGURE 1. The landscape of genetic alterations of m6A regulators in melanoma. (A) Visualization of the Metascape enrichment network presenting similarities within and between clusters of terms. The same colors represent the same clustering terms. (B) Mutations in 23 m6A regulators were present in 133 of 467 melanoma patients (28.48%), with the most prevalent missense mutations, nonsense mutations, and frame shift deletion mutations. The numbers on the right side are representative of the mutation frequency of each regulator. Each column is one patient. (C) Visualization of co-occurrence and exclusion of 23 m6A regulator mutations. Green color represents co-occurrence, and purple color represents exclusion. (D) CNV mutations are present in all 23 m6A regulators. Column heights represent mutation frequencies. Pink dots represent loss mutations, and blue dots represent gain mutations. (E) Differential expression of mRNA of 23 m6A regulators in metastatic melanoma and primary melanoma. * represents p-values in statistics (*p < 0.05; **p < 0.01; ***p < 0.001). (F) Interaction network of the three m6A regulators in melanoma. Different colors represent different types of m6A regulators; green is a reader, blue is a writer, and red is an eraser. The connecting lines represent the correlation matrix; pink is positive correlation, while blue is negative correlation. Larger circles represent smaller p-values for prognostic analysis, and the shiny green dot in the center of the circle represents protective factors, while the black dot represents risk factors. (G) Visualization of tumor purity and 23 m6A regulator. Red color represents co-occurrence, and blue color represents exclusion.
The m6A Methylation Modification Pattern Consisting of 23 m6A Regulators Is Associated with Prognosis in Melanoma Patients.
Further, we stratified melanoma samples into three m6A modification patterns according to the expression of m6A regulators, a process based on consensus clustering analysis of the NMF algorithm (Supplementary Figure S2A,C). We named the three clusters as m6A-C1, m6A-C2, and m6A-C3, respectively (Figure 2A). The samples of m6A-C2 cluster had significantly different from the other two groups with regard to ELAVL1, RBM15B, YTHDF1/2/3, IGF2BP1/2/3, WTAP, METTL3, ZC3H13, RBM15, HNRNPA2B1, CBLL1, and LRPPRC. Besides, YTHDF1, IGF2BP3, METTL3, ZC3H13, and LRPPRC were significantly upregulated in the m6A-C1 subtype, WTAP and RBM15 were significantly upregulated in the m6A-C3 subtype, while ELAVL1, IGF2BP3, ZC3H13, and LRPPRC were significantly decreased in the m6A-C3 subtype. This conclusion was validated in the meta-GEO cohort consisting of five datasets, which include GSE19234, GSE22154, GSE50509, GSE59455, and GSE65904 (Supplementary Figure S2B). Patients in the m6A-C3 cluster have a significant survival advantage than other clusters in both TCGA and meta-GEO cohort (log-rank test, TCGA: p < 0.0001, Figure 2B; meta-GEO: p = 0.0015, Figure 2D). This model remained significant after multivariate Cox proportional risk regression analysis adjusted for clinicopathological factors of age, gender, and stage (TCGA: HR = 0.34 (0.21–0.51), p < 0.001; meta-GEO: HR = 0.51 (0.35–0.76), p < 0.001; Figures 2C,E).
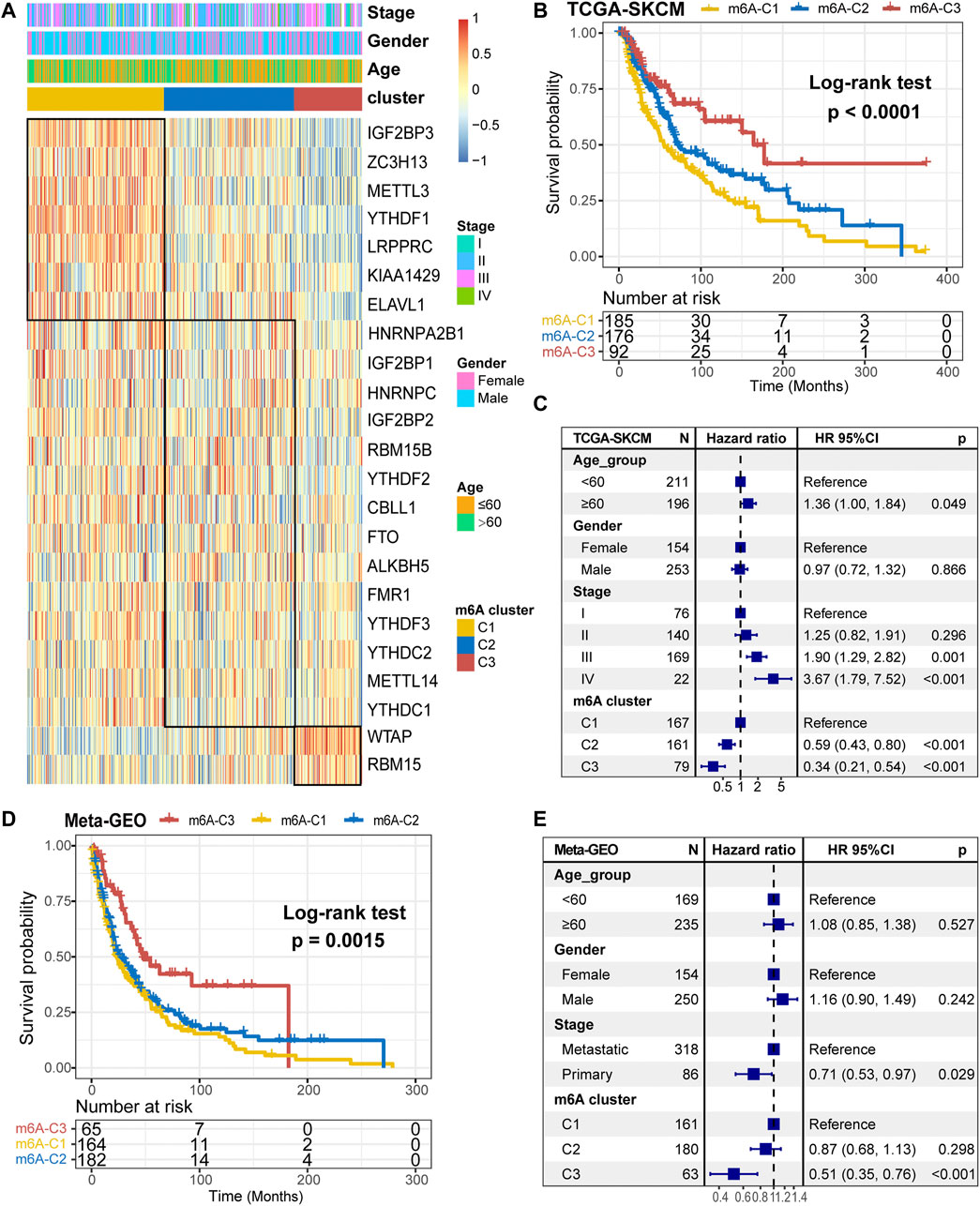
FIGURE 2. m6A methylation modification cluster and unsupervised clustering. (A) Results of unsupervised clustering of gene expression of 23 m6A moderators in the TCGA-SKCM cohort. (B) Kaplan-Meier curves of overall survival (OS) for different m6A clusters in the TCGA cohort. (C) Subgroup analysis for estimating clinical prognostic value of m6A modification subtype after adjusting for age, gender, and stage in the TCGA cohort. (D) Kaplan-Meier curves of overall survival (OS) for different m6A clusters in the meta-GEO cohort. (E) Subgroup analysis for estimating clinical prognostic value of m6A modification subtype after adjusting for age, gender, and stage in the meta-GEO cohort.
Characterization of the Immune Landscape With Three m6A Modification Clusters
We performed GSVA analysis against on Hallmarker gene set in an attempt to discover differences in the biological behavior of the three m6A modification clusters. As shown in Figure 3A, m6A-C1 cluster was associated with cell proliferation and differentiation and glucose transport, including oxidative phosphorylation, PI3K/AKT/mTOR signaling, DNA repair, and glycolysis. m6A-C2 cluster is distinguished by cancer and immune surveillance, involving epithelial mesenchymal transition, TGF-β signal, TNF-α signaling via NF-κB, and IL2/STAT5 signaling. As for m6A-C3, it was significantly enriched in signaling pathways related to inflammation and innate immune response, such as interferon-γ response, interferon-α response, allograft rejection, IL6/JAK/STAT3 signaling, and inflammatory response. The GSVA results further corroborate that these three m6A methylation modification clusters are directly related to different molecular mechanism, and m6A-C3 was strongly associated with antitumor immunity. In addition, we further evaluated the immune enrichment level of m6A methylation modification clusters using the ImmuneScore model constructed by ESTIMATE algorithm. The results showed significant differences in different clusters in both TCGA-SKCM cohort and the meta-GEO cohort (Supplementary Figure S3A,B). There is a coherence between the immune activation and survival time, which cluster with higher ImmuneScore having greater survival benefit for patients, like m6A-C3 in the TCGA-SKCM cohort and meta-GEO cohort. Thorsson et al. (Thorsson et al., 2018) divided the tumor immune landscape into six immune subtypes, represented with immune infiltration and stromal activation. Consistent with our findings, the m6A-C1 cluster is more inclined to the “Proliferation” and “Wound Healing” subtypes, m6A-C2 is highly expressed in “TGF-β Response,” and m6A-C3 is mainly dominated by “Lymphocyte Infiltration Signature Score,” “Macrophage Regulation,” and “IFN-γ Response” subtypes (Figure 3B). In addition, we performed a comparative analysis of immune checkpoint-related key genes (IDO1, CD274, TIM-3, PDCD1, CTLA-4, LAG3, and PDCD1LG2) among the three clusters. The results indicated the expression levels of seven key genes differ significantly between the three clusters, and the highest expression was all observed in the m6A-C3 cluster. TCGA-SKCM has established molecular typing based on the genomic landscape and transcriptomic profile. Although the proportion of m6A modification clusters among different mutational-based molecular subtype (BRAF-Mut, RAS-Mut, NF1-Mut, and Triple Negative) were not significant (Figure 3D), an obvious difference was found in transcriptomic-based subtype across three m6A modification clusters (Figure 3E). Samples with TCGA-Immune subtype account for 93.1% of the m6A-C3 cluster, followed by 64.8% in m6A-C2 subtype. However, the m6A-C1 were dominated by TCGA-Keratin (55.7%) and TCGA-MIFT-low (32.8%) subtype, which demonstrated the desert-related immune phenotype in m6A-C1. It is feasible to determine immune cell type abundance and expression from bulk tissues with digital cytometry (Newman et al., 2019). We also compared the immune cell infiltration level among the three m6A modification clusters in Figure 3F. The m6A-C1 cluster showed more myeloid-derived suppressor cell (MDSC), regulatory T cells, and T helper cell infiltration, while the m6A-C3 cluster exhibited infiltration of most types of T cells, natural killer cells, and dendritic cells. This suggested that the lower survival risk of melanoma patients with m6A-C3 clusters may be due to effective activation of the pre-existing immunity to inhibit tumor growth and malignant progression. Likewise, evaluation on immune cell abundance by CIBERSORT algorithm also corroborate our conclusions (Supplementary Figure S3C). In addition, the association between each m6A regulator and immune cell infiltration was also explored. As expressed in Supplementary Figure S3D, upregulation of WTAP and ALKBH5 was positively correlated with enhanced immune infiltration, while high expression of LRPPRC, METTL3, YTHDF1/3, and ZC3H13 was mostly associated with immunosuppression.
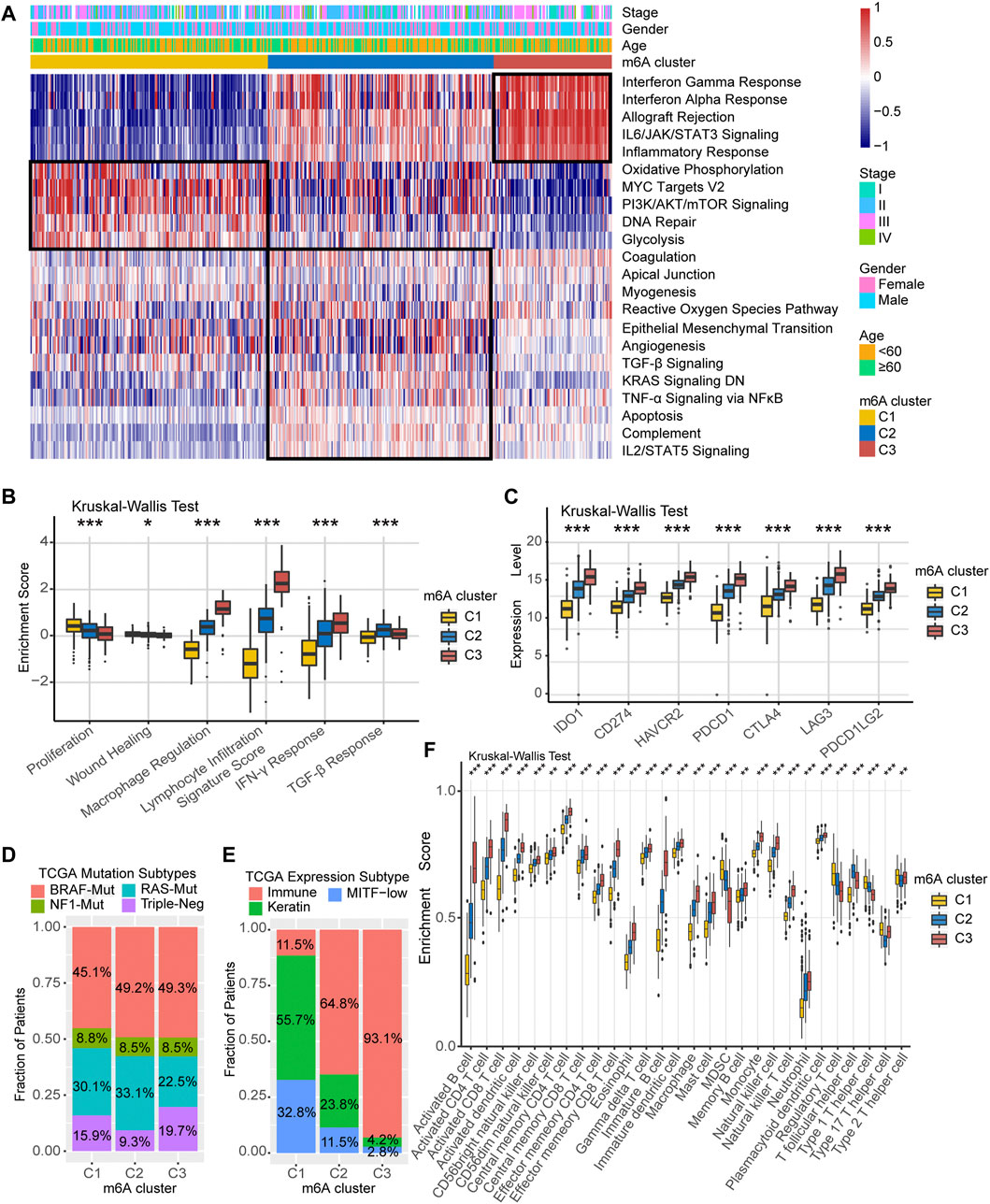
FIGURE 3. TME characteristics in distinct m6A modification clusters. (A) Heatmap of enriched pathways based on Hallmark gene set corresponding to different m6A modification clusters. (B) Relative distribution of six immune subtype in three different m6A clusters. (C) Expression level of immune checkpoint-related key genes among the three m6A clusters. (D) Association between TCGA genomic molecular typing and m6A clusters. (E) Association between TCGA transcriptome molecular typing and m6A clusters. (F) Relative infiltration level of 28 immune cell subsets among three distinct m6A modification clusters.
Differentially Expressed Genes Associated With m6A Methylation Modifications in Melanoma
Since RNA N6-methyladenosine (m6A) modification plays an important role in post-transcriptional regulation, we further examine the potential impact on gene expression change of each m6A modification cluster in melanoma. To clarify these queries, we employed the Bayesian-based method to identify differentially expressed genes (DEGs) that are differentially regulated across the three m6A methylation modification clusters. As illustrated in the Venn diagram of Figure 4A, there are a total of 636 DEGs which may play the crucial role in distinguishing the three m6A modification clusters (Supplementary Table S3). Based on these 636 representative DEGs of m6A signature, we further stratified the melanoma samples into three well typed transcriptomic phenotypes (denoted as m6Sig-SI, m6Sig-SII, and m6Sig-SIII) by unsupervised consensus clustering analysis (Supplementary Figure S4A). We also compared the m6A clusters and m6A signature-derived subtype, and found a significant association among these two-stratification method (Supplementary Table S4, adjusted χ2 test, p < 0.0001). Patients in m6Sig-SII were proved to be associated to better prognosis, while m6Sig-SI had a worse outcome (p < 0.001, log-rank test; Figure 4B). PD-L1 and ImmmuneScore were also highly expressed in m6Sig-SII subtype than the other subtypes (p < 0.0001, Kruskal Wallis test; Figures 4C,D). Mariathasan et al. (Mariathasan et al., 2018) summarized and formed a suit of gene set for assessing the activation of immune and stroma signaling pathway, whereby we adopted to evaluate the m6Sig signature. The m6Sig-SI subgroup was enriched in cell proliferation and DNA damage repair related pathways, m6Sig-SIII was characterized by CD8 T effector and antigen processing related pathway, whereas m6Sig-SII was focused in epithelial mesenchymal transition (EMT) related signaling pathway (Figure 4E). The expression level of 23 m6A regulators in three gene subgroups was also compared and shown in Supplementary Figure S4B. We observed significant differences of m6A regulator expression in the three m6A gene-signature subgroups, which was consistent with the expected results of the m6A methylation modification clusters.
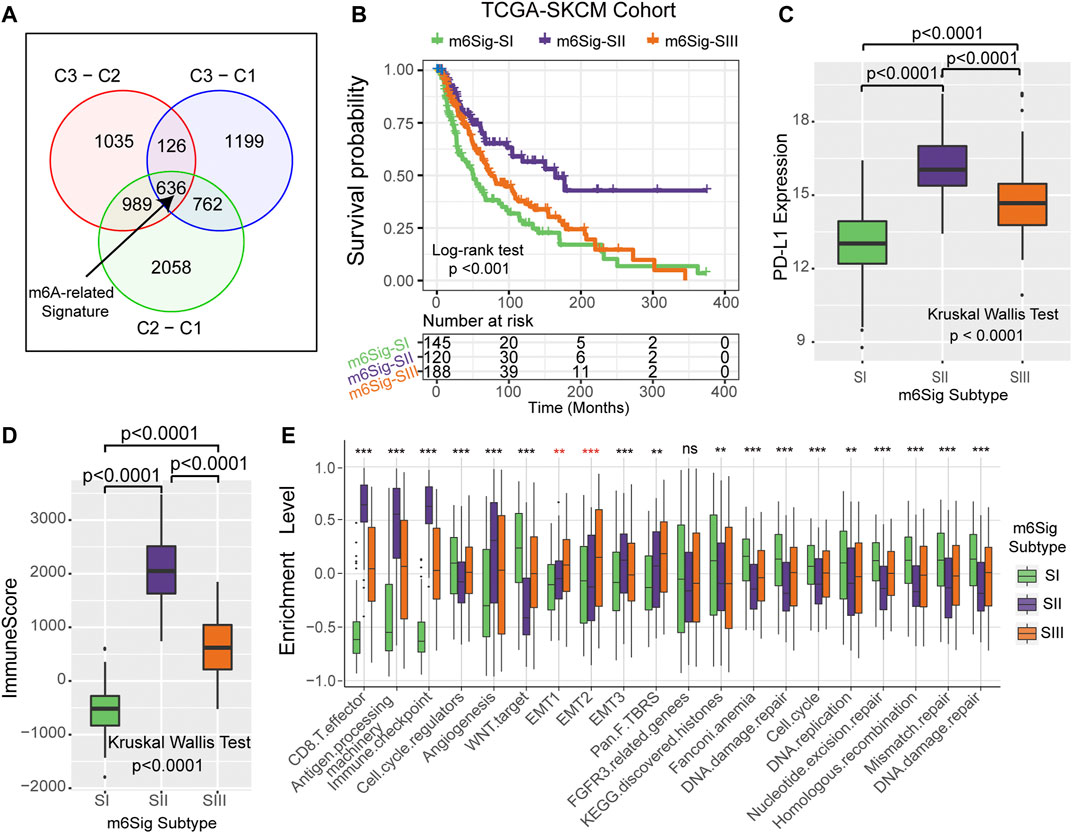
FIGURE 4. Construction of differential expression of m6A gene signatures and functional annotation. (A) The 636 differentially expressed genes between the three m6A clusters were recognized as m6A-related gene signature and shown in the Venn diagram. (B) Survival curves of m6A signature gene-based NMF unsupervised clustering in TCGA cohort. (C) Differences in PD-L1 expression among m6Sig subtype groups. (D) Differences in ImmuneScore between m6sig subtype groups. (E) Enrichment level of the three m6Sig subtypes in the classical signaling pathway constructed by Mariathasan et al.
The m6Sig Score System and Its Clinical Relevance
The results of the previous parts of the study can be concluded that m6A methylation modification has a strong association with prognosis and immune regulation in melanoma patients. In order to be able to accurately predict the m6A methylation modification cluster of tumors in individual melanoma patients, we developed the m6Sig score system to quantify the m6A modification cluster based on the identified m6A-related signature genes. In Figure 5A, the Sankey diagram uncovered the workflow of the m6Sig score system in melanoma. It can be found that m6A-C3 was linked to a higher m6Sig score, and lower for keratin and MITF-low subtypes. We sought to evaluate the significance of the m6Sig score system in predicting the prognosis of patients with melanoma through survival analysis. As shown in Figures 5B,C, the m6Sig score system clearly distinguished patient with different prognosis in both the TCGA-SKCM cohort and meta-GEO cohort (patients with high m6Sig scores had a better prognosis). We performed the Kruskal Wallis test and showed that the m6Sig score could be clearly distinguished in the immune and keratin subtypes (Figure 5D). Encouragingly, the m6Sig score system can be extremely well distinguished among the previous m6A clusters and m6Sig clusters (Supplementary Figure S5A,B), which are clusters constructed based on m6A methylation modification clusters and DEGs, respectively. Compared with clinicopathological staging, the m6Sig score system can better evaluate the prognosis of melanoma patients (AUCs for stage and m6Sig score are 0.613 and 0.681, respectively; Supplementary Figure S5C). In detail, multivariable cox regression model revealed that melanoma patients with low m6Sig scores had a worse survival outcome in TCGA (Supplementary Figure S5D) and meta-GEO cohort (Supplementary Figure S5E). This score system was also explored by ImmuneScore, and patients with high m6Sig scores had a higher ImmuneScore (p < 0.0001, Supplementary Figure S5F,G). Among the six immune subtypes, the m6Sig score was also differentially distributed. Samples with high scores were mostly clustered in the “Macrophage Regulation,” “Lymphocyte Infiltration Signature Score,” and “IFN-γ Response” subtypes (Supplementary Figure S5H), which was similar to the m6A-C3 cluster. Heatmap of correlation matrix demonstrated that the m6Sig score was markedly positively correlated with the immune activation process and negatively correlated with cell cycle and DNA damage repair (Supplementary Figure S5I). To better evaluate the effectiveness of our m6Sig score system in predicting the prognosis of melanoma patients, we introduced two independent cohorts (GSE22153 and GSE54437) to perform a survival analysis, and the results showed that patients with high m6Sig score had a better prognosis (Supplementary Figure S6A,B). Furthermore, a survival analysis after combining all patients involved in this study revealed that a high m6Sig score continued to indicate a survival benefit in melanoma patients (p < 0.0001, Supplementary Figure S6C). The TCGA-SKCM cohort also demonstrated that melanoma patients with high m6Sig score had prolonged disease-free survival (DFS, p = 0.0064, Supplementary Figure S6D). In addition, m6Sig score also negatively correlated with somatic copy number alternation (SCNA) level (r = −0.49, p < 0.0001, Figure 5E), which is a significant predictor of immunotherapy resistance in melanoma. The PD-L1 expression levels were also positively correlated with the m6Sig score (r = 0.75, p < 0.0001, Figure 5F), suggesting that melanoma patients may also benefit from the m6Sig score system for PD1/PD-L1 treatment regimens. More deeply, we performed significant mutation gene (SMG) analysis of melanoma samples based on m6Sig score, and the waterfall plot of mutation landscape noted that BRAF (54%/48%), SIRPB1(11%/5%), and KNSTRN (7%/2%) had higher somatic mutation rates in the high-score group, although BRAF was not statistically significant (Figure 5G). These data assist us to more comprehensively understand the m6Sig score system mapping to genomic variants, predicting that m6A methylation modification is closely linked to somatic mutations in melanoma patients.
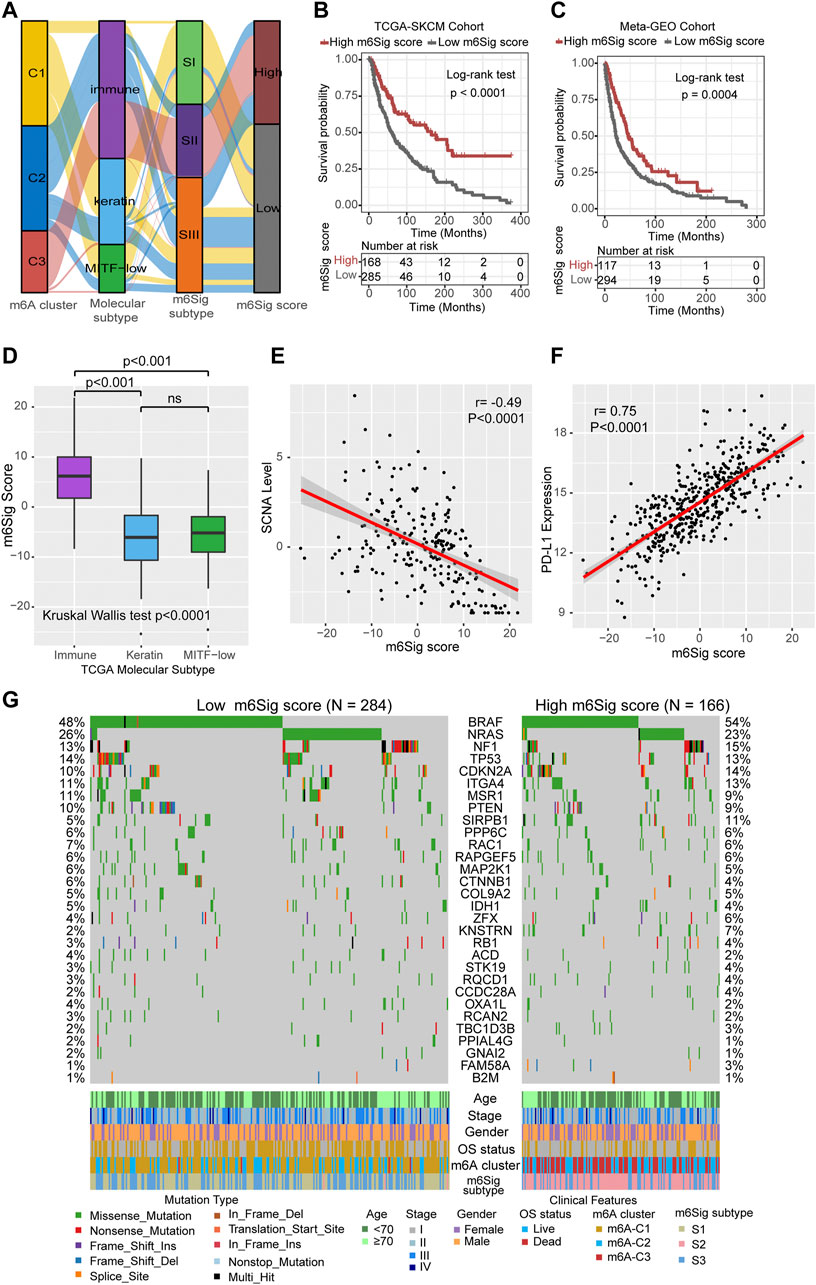
FIGURE 5. Construction of m6Sig score and explore the relevance of clinical features. (A) Alluvial diagram of m6A clusters in groups with different molecular subtypes (immune, keratin, and MITF-low), m6A-gene cluster, and m6Sig score. (B) Kaplan-Meier curves for high and low m6Sig score patient groups in TCGA cohort. (C) Kaplan-Meier curves for high and low m6Sig score patient groups in meta-GEO cohort. (D) The m6Sig score differed between the three TCGA molecular types. (E) The m6A score was negatively correlated with the SCNA mutational level. (F) The m6A score was positively correlated with PD-L1 expression level. (G) Mutation status of significantly mutated genes (SMGs) in the TCGA cohort, stratified by subgroups with low (left) versus high m6Sig scores (right). Each column represents one patient. Mutation types and clinical characteristics were annotated in different colors.
The m6Sig Score System Can Be a Better Predictor of the Effectiveness of Immunotherapy in Cancer
Cancer treatment regimens based on immune checkpoint inhibitors have provided a landmark innovation in the treatment of malignancies, mostly in melanoma. In addition to TML and PD-L1, TIDE and T cell-inflamed GEP have been recommended to predict immune response in recent years (Chen et al., 2019c; Chen et al., 2019d). We compared the established m6Sig score system with the T-cell inflamed gene expression profile (GEP) score and found that melanoma patients with high m6Sig score had elevated T-cell inflamed GEP score in both the TCGA-SKCM cohort and the Meta-GEO cohort (p < 0.0001, Figures 6A,B). In contrast, TIDE showed increased levels in patients with low m6Sig scores, implying that greater chance of tumor immune escape and resistance in low m6Sig scores subgroup (p < 0.0001, Figures 6C,D). These results further demonstrate that m6A modification clusters play a critical role in the immune response of tumors, thereby affecting the immune microenvironment of tumors.
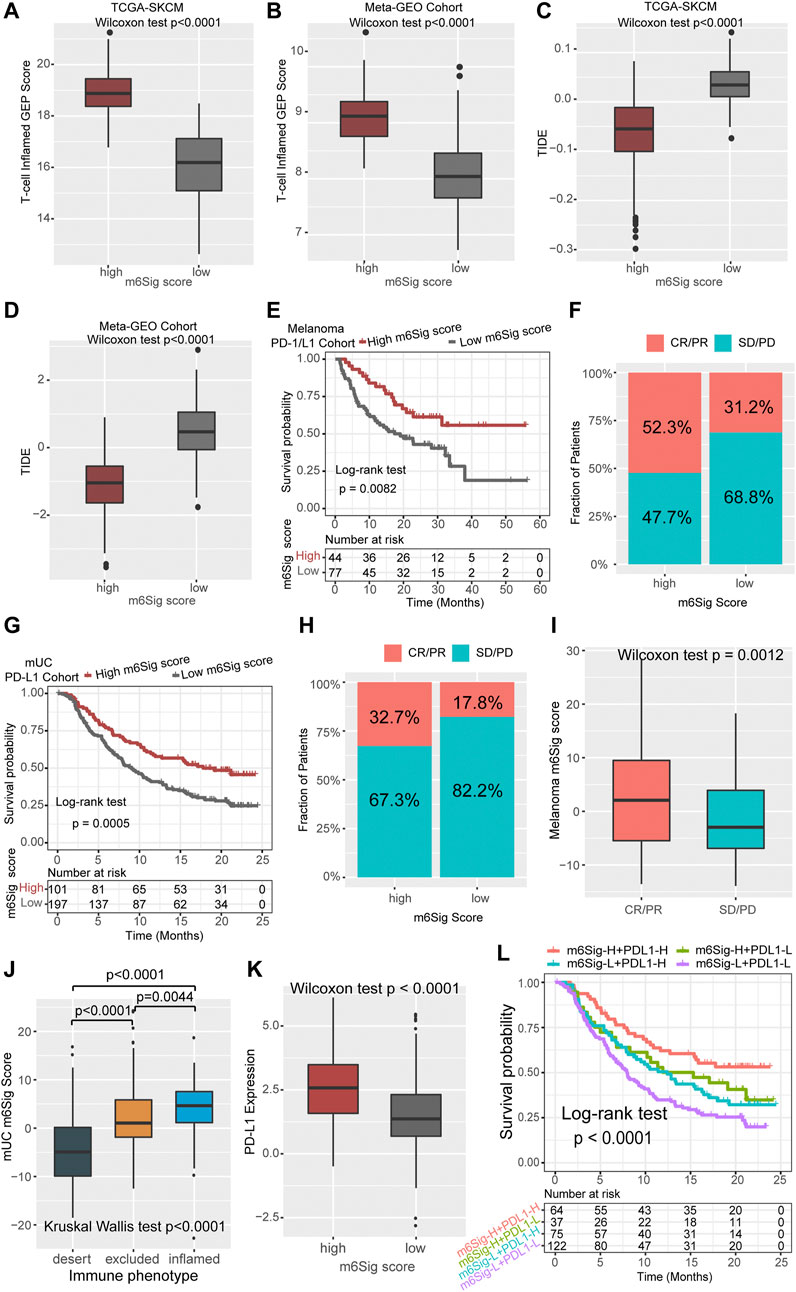
FIGURE 6. The m6Sig score predicts immunotherapeutic benefits. (A) Comparison of the relative distribution of T-cell inflamed GEP scores between the high and low m6Sig score groups in the TCGA cohort. (B) Comparison of the relative distribution of T-cell inflamed GEP scores between the high and low m6Sig score groups in the meta-GEO cohort. (C) Comparison of the relative distribution of TIDE between the high and low m6Sig score groups in the TCGA cohort. (D) Comparison of the relative distribution of TIDE between the high and low m6Sig score groups in the meta-GEO cohort. (E) Kaplan-Meier curves for high and low m6Sig score patient groups in the melanoma PD-1/CTLA-4 cohort. (F) The fraction of patients with clinical response to anti-PD-1/CTLA-4 immunotherapy in low or high m6Sig score groups. (G) Kaplan-Meier curves for high and low m6Sig score patient groups in the metastatic urothelial carcinoma (mUC) PD-L1 cohort. (H) The fraction of patients with clinical response to anti-PD-L1 immunotherapy in low or high m6Sig score groups of mUC cohort. (I) Distribution of m6Sig scores between immunotherapy response and non-response in melanoma PD-1/CTLA-4 cohort. (J) Distribution of mUC m6Sig scores among the three immune phenotypes. (K) The relationship between m6Sig score and PD-L1 expression level. (L) The m6Sig score combined with PD-L1 expression levels better predicted patient prognosis. CR, complete response; PR, partial response; SD, stable disease; PD, progressive disease.
Aforementioned data point to a strong association between m6A modification and immune response, we next investigated whether the m6Sig score could predict patients’ response to ICI treatment in independent immunotherapy cohorts. Patients with high m6Sig score exhibited significantly longer survival time (log-rank test, p = 0.0082, Figure 6E) and markedly clinical response to PD-1/CTLA-4 treatment in melanoma (response rate, high vs low m6Sig score subgroup, 52.3 vs 31.2%, Figure 6F). This result was also identified in an anti-PD-L1 metastatic uroepithelial cancer cohort (Mariathasan et al., 2018), in which patients with high m6Sig scores significantly benefited from PD-L1 immunotherapy (log-rank test, p = 0.0005, Figure 6G; response rate: high vs low m6Sig score subgroup, 32.7 vs 17.8%, Figure 6H). Furthermore, we found that patients with PD-1 immune response also had a higher m6Sig score (p = 0.0012, Figure 6I). Metastatic uroepithelial carcinoma patients with immune inflamed phenotype had a higher m6Sig score than immune excluded and desert phenotype (Figure 6J). A significant elevation of PD-L1 was identified in high m6Sig score subgroup (p < 0.0001, Figure 6K). Therefore, we divided the overall population into four subgroups according to the TMEsig-score and PD-L1 distribution, including TMEsig-score-H + PD-L1-H, TMEsig-score-H + PD-L1-L, TMEsig-score-L + PD-L1-H, and TMEsig-score-L + PD-L1-L. The TMEsig-score-H + PD-L1-H subgroup exhibited the best prognostic outcome compared with the other three subgroups (log-rank test, p < 0.0001, Figure 6L). Based on the results of the above analysis, our established m6Sig score system enables the prediction of responsiveness and prognosis to cancer immunotherapy.
Discussion
Recently, the dynamic and reversible process of m6A modification has been reported in participation of the innate immune, inflammatory response, and anti-tumor processes (Chen et al., 2019a; Shulman and Stern-Ginossar, 2020). Although numerous studies have recently revealed how m6A regulators are epigenetically regulated in the tumor immunogenicity, the association between m6A regulators and the overall tumor microenvironment has not yet been elucidated in melanoma. Thus, identifying distinct m6A modification clusters in the TME infiltration will contribute to advancing our understanding of anti-tumor immune response and facilitating more effective precision immunotherapy strategies.
In this study, we identified three different immunophenotypic m6A methylation modification clusters, which are characterized by different anticancer immune effects. The m6A-C1 phenotype is distinguished by promotion of cell proliferation and activation of PI3K/AKT/mTOR signaling pathway, and we prefer it to be the immune-desert phenotype. The m6A-C2 phenotype is more characteristic of cancer and immune surveillance, and it is associated with EMT, TGF-β, and TNF-α pathway activation and is an immune-excluded phenotype. The m6A-C3 phenotype, on the other hand, is associated with activation of pathways related to inflammatory response, innate immune response, and is an immune-inflamed phenotype. It has been shown that the tumor microenvironment plays a central role in tumorigenesis development and progression, and the levels of tumor-infiltrating CD4+/CD8+ T cells, M1 macrophages, NK cells, and inflammatory cytokines directly influence the onset of immune priming and adaptive immunity (Topalian et al., 2016; Galon and Bruni, 2019; Zeng et al., 2020). Interestingly, the m6A-C2 phenotype is associated with activation of the TGF-β signaling pathway and intermediate immune cell infiltration, and thus, we hypothesized that melanoma patients with m6A-C2 phenotype would benefit from the combination of immune checkpoint inhibitors and TGF-β blockers. There is evidence pointing out that activation of the TGF-β pathway hinders lymphocyte attack on “tumor barriers” (Tauriello et al., 2018). Moreover, inhibitors targeting TGF-β can effectively remodel the tumor microenvironment in the form of reprogrammed peritumor stromal fibroblasts, which can restore the body’s anti-tumor immunity (Mariathasan et al., 2018; Panagi et al., 2020). We found that the m6A-C3 phenotype was associated with enrichment of activated tumor-infiltrating lymphocytes, making this phenotype more likely responsive to ICI immunotherapy.
The m6A signature genes were derived from genes differentially expressed in three m6A modification subtypes. These m6A-related signature genes were further utilized to identify transcriptomic subtypes and tumor microenvironment landscapes in melanoma. Patients with m6Sig-SII subtype have higher PD-L1 expression levels and higher immuneScores, implying that patients with this subtype are better treated with immune checkpoint inhibitors for better therapeutic outcomes. For the sake of precision clinical practice, we optimized the m6Sig signatures into the m6Sig score scheme, a system that could be used to quantify the m6A modification level of individual tumors. The m6A modification clusters characterized by an immune-inflamed phenotype showed a higher m6Sig score, whereas the modification cluster characterized by an immune-desert phenotype had a lower score. The results based on survival analysis highlight that the m6Sig score system can effectively predict the prognosis of melanoma patients, and that this score system is strongly associated with TCGA molecular subtypes, genomic alternations, and PD-L1 expression levels. We also observed that m6Sig score was closely correlated with T-cell inflamed GEP score and TIDE, which are effective tools for prediction of immunotherapy benefit, further demonstrating that m6A RNA methylation modification can modulate the effect of immune response in melanoma. To identify the predictive value of m6Sig score system in immune response, we performed a series of analyses in two additional independent immunotherapy cohorts and validated the effect of this score system. In a nutshell, the m6A RNA methylation modification cluster can be used to determine the immune phenotype of melanoma patients, further guiding clinical treatment planning and effectively predicting the prognosis of patients.
We also noticed that certain m6A regulators play different roles in regulating tumorigenesis and tumor immunogenicity. Recent studies have confirmed that the mRNA stability and translation processes of the oncofetal IGF2 mRNA binding proteins (IGF2BPs) are regulated by RNA N6-methyladenosine (Huang et al., 2018). IGF2BP1, a member of the IGF2BPs family, was then identified as an oncogene that promotes cancer development by antagonizing cancer-suppressive miRNAs (Müller et al., 2018; Müller et al., 2019). In contrast, our results showed that IGF2BPs genes have higher expression level in patients with metastatic melanoma and m6A-C1 subtype. It has been demonstrated that IGF2BP2 promotes cancer progression by regulating the m6A-dependent glycolytic process and promotes cancer metastasis in the form of an RNA-protein ternary complex (Chen et al., 2019e). KIAA1429 is also well known as an m6A methyltransferase. In hepatocellular carcinoma, KIAA1429 promotes cancer metastasis and leads to poor patient prognosis by regulating post-transcriptional modifications (Lan et al., 2019). Our results also suggest this function of KIAA1429 to promote metastasis and highly expressed in m6A-C1 desert phenotype, but its prediction of patient survival may require the combination of RBM15, RBM15B, IGF2BP3, and HNRNPA2B1, with co-occurrence between them. YTHDCs and YTHDFs containing YTH domain act as “readers” in post-translational RNA methylation modification, and YTHDFs enhance aerobic glycolysis by degrading mRNA to further promote tumor formation (Wang et al., 2021; Xia et al., 2021). Our study confirms that both YTHDF1/3 and YTHDC1/2 are highly expressed in metastatic melanoma, and of interest, the high expression of YTHDF1 in patients with metastatic melanoma is accompanied by an indication of a poorer prognosis, which suggests a new direction for deeper studies of molecules containing YTH domain. In our study, we found that ELAVL1 was not only associated with metastasis of melanoma, but also reflected a poorer prognosis of patients, which may be related to the fact that ELAVL1 can stabilize oncogenic transcripts (Li et al., 2020b). In summary, the results of our analysis demonstrate the importance of a systematic and comprehensive consideration of m6A modification clusters, which are diverse in cancer across physiological processes.
Identification of significantly mutated genes underlying human cancers is a critical foundation for cancer diagnostics, therapeutics, and selection of rational therapies. In our study, we found a higher proportion of SMGs of BRAF, SIRPB1, and KNSTRN in the high m6Sig score subgroup, although BRAF was of marginal significance. In a pan-cancer study, it was noted that BRAF has a higher rate of specific driver mutations in leukocytes of cancer patients, a phenomenon associated with tumor-immune cell interactions (Thorsson et al., 2018). There is new evidence that BRAFi induces the occurrence of anti-tumor cell scorching immune responses, which may be a new strategy for the treatment of melanoma (Erkes et al., 2020). Signal regulatory protein beta 1 (SIRPB1) is a member of the signal regulatory protein (SIRP) family, which also belongs to the immunoglobulin superfamily, and is a negatively regulated receptor-type transmembrane glycoprotein involved in receptor tyrosine kinase-coupled signaling processes. SIRPB1 is associated with neutrophil migration across the epithelium, which provides a new target for drug design in immunotherapy (Ribeiro et al., 2019). It has been reported that KNSTRN mutations rarely occurred in other solid tumors and leukemias, which are relatively specific for skin-related cancers (Lee et al., 2016; Schmitz et al., 2019). These tumor driver mutations in different m6Sig scores not only are associated with malignant progression, metastasis, and recurrence of cancer but also play a role in the regulation of immune activity, demonstrating a complex and consequently clear interaction between m6A RNA methylation modifications and tumor immunogenomic.
The literature review helped us to integrate the well-known 23 m6A RNA methylation regulators for meta-analysis, but this still requires newly discovered regulators to be included to enhance the accuracy of the established m6A modification clusters. There is a relative lack of PD-L1-based regimens for melanoma patients, so we introduced a dataset of uroepithelial carcinoma treated with atezolizumab, but we still hope that the m6Sig score system can be analyzed and validated in melanoma immunotherapy with different immune checkpoint inhibitors. Moreover, all the data in this study were obtained from retrospective cohort, which would introduce some bias. Therefore, our next study focused on establishing a prospective cohort of melanoma patients with immune checkpoint inhibitors to validate and optimize the m6Sig score system. In addition, the current m6Sig score system does not yet incorporate the clinicopathological characteristics of the patients, which also lead to the drawbacks of the system.
In our study, we systematically assessed the m6A modification clusters of 1,020 melanoma patients and comprehensively analyzed the impact of m6A modification clusters generated by 23 m6A regulators on the cellular infiltration characteristics of the tumor microenvironment. The results of this integrative analysis confirm that RNA methylation is essential for the regulation of tumor immune response, and assessing the m6A modification clusters of patient tumors will help us better understand the immune microenvironment infiltration characteristics and provide new ideas for indications and protocol modifications for immunotherapy.
Data Availability Statement
Publicly available datasets were analyzed in this study. This data can be found here: Gene expression data and clinical information for melanoma patient samples were obtained from the GEO database (https://www.ncbi.nlm.nih.gov/geo/) and TCGA database (https://portal.gdc.cancer.gov/), which are publicly and freely available, including the GSE19234, GSE22154, GSE50509, GSE59455, GSE65904, GSE22153, GSE54437 and TCGA-SCKM datasets.
Author Contributions
Conception and design: XKL, FD, HL; Development of methodology: FD, HL, YLi, YLiu; Acquisition of data (provided data, acquired and managed patients, provided facilities, etc.): XYL, ND, QC, ZF, HW, XZ, JY; Analysis and interpretation of data (e.g., statistical analysis, biostatistics, computational analysis): FD, HL, YLi, YLiu, XKL; Writing, review, and/or revision of the manuscript: FD, HL, XKL; Administrative, technical, or material support (i.e., reporting or organizing data, constructing databases): FD, HL, YLi, YLiu; Study supervision: XKL.
Funding
This study was supported by grant from Key Research and Development Program of Shandong Province (No.2019JZZY010104; No.2019GSF108146), Academic promotion programme of Shandong First Medical University (2019QL021), Special Foundation for Taishan Scholars Program of Shandong Province (No.ts20190978), The Research Incubation Funding of Shandong Provincial Hospital, and Youth Training Program for High-level Projects of Jinan Central Hospital.
Conflict of Interest
The authors declare that the research was conducted in the absence of any commercial or financial relationships that could be construed as a potential conflict of interest.
Publisher’s Note
All claims expressed in this article are solely those of the authors and do not necessarily represent those of their affiliated organizations, or those of the publisher, the editors and the reviewers. Any product that may be evaluated in this article, or claim that may be made by its manufacturer, is not guaranteed or endorsed by the publisher.
Acknowledgments
We sincerely thank YY from Tianjin tumor hospital for analysis advice and CA for language editing.
Supplementary Material
The Supplementary Material for this article can be found online at: https://www.frontiersin.org/articles/10.3389/fcell.2021.761134/full#supplementary-material
References
Ayers, M., Lunceford, J., Nebozhyn, M., Murphy, E., Loboda, A., Kaufman, D. R., et al. (2017). IFN-γ-related mRNA Profile Predicts Clinical Response to PD-1 Blockade. J. Clin. Invest. 127 (8), 2930–2940. doi:10.1172/JCI91190
Binnewies, M., Roberts, E. W., Kersten, K., Chan, V., Fearon, D. F., Merad, M., et al. (2018). Understanding the Tumor Immune Microenvironment (TIME) for Effective Therapy. Nat. Med. 24 (5), 541–550. doi:10.1038/s41591-018-0014-x
Charoentong, P., Finotello, F., Angelova, M., Mayer, C., Efremova, M., Rieder, D., et al. (2017). Pan-cancer Immunogenomic Analyses Reveal Genotype-Immunophenotype Relationships and Predictors of Response to Checkpoint Blockade. Cel. Rep. 18 (1), 248–262. doi:10.1016/j.celrep.2016.12.019
Chen, D. S., and Mellman, I. (2017). Elements of Cancer Immunity and the Cancer-Immune Set point. Nature 541 (7637), 321–330. doi:10.1038/nature21349
Chen, H., Chong, W., Wu, Q., Yao, Y., Mao, M., and Wang, X. (2019). Association of LRP1B Mutation with Tumor Mutation Burden and Outcomes in Melanoma and Non-small Cell Lung Cancer Patients Treated with Immune Check-Point Blockades. Front. Immunol. 10, 1113. doi:10.3389/fimmu.2019.01113
Chen, H., Chong, W., Teng, C., Yao, Y., Wang, X., and Li, X. (2019). The Immune Response‐related Mutational Signatures and Driver Genes in Non‐small‐cell Lung Cancer. Cancer Sci. 110 (8), 2348–2356. doi:10.1111/cas.14113
Chen, H., Chong, W., Yang, X., Zhang, Y., Sang, S., Li, X., et al. (2020). Age-related Mutational Signature Negatively Associated with Immune Activity and Survival Outcome in Triple-Negative Breast Cancer. Oncoimmunology 9 (1), 1788252. doi:10.1080/2162402X.2020.1788252
Chen, H., Yang, M., Wang, Q., Song, F., Li, X., and Chen, K. (2019). The New Identified Biomarkers Determine Sensitivity to Immune Check-point Blockade Therapies in Melanoma. Oncoimmunology 8 (8), 1608132. doi:10.1080/2162402x.2019.1608132
Chen, R. X., Chen, X., Xia, L. P., Zhang, J. X., Pan, Z. Z., Ma, X. D., et al. (2019). N6-methyladenosine Modification of circNSUN2 Facilitates Cytoplasmic export and Stabilizes HMGA2 to Promote Colorectal Liver Metastasis. Nat. Commun. 10, 4695. doi:10.1038/s41467-019-12651-2
Chen, X.-Y., Zhang, J., and Zhu, J.-S. (2019). The Role of m6A RNA Methylation in Human Cancer. Mol. Cancer 18 (1), 103. doi:10.1186/s12943-019-1033-z
Chen, Y.-T., Shen, J.-Y., Chen, D.-P., Wu, C.-F., Guo, R., Zhang, P.-P., et al. (2020). Identification of Cross-Talk between m6A and 5mC Regulators Associated with Onco-Immunogenic Features and Prognosis across 33 Cancer Types. J. Hematol. Oncol. 13 (1), 22. doi:10.1186/s13045-020-00854-w
Chen, Z., Wu, L., Zhou, J., Lin, X., Peng, Y., Ge, L., et al. (2020). N6-methyladenosine-induced ERRγ Triggers Chemoresistance of Cancer Cells through Upregulation of ABCB1 and Metabolic Reprogramming. Theranostics 10 (8), 3382–3396. doi:10.7150/thno.40144
Chong, W., Shang, L., Liu, J., Fang, Z., Du, F., Wu, H., et al. (2021). m6A Regulator-Based Methylation Modification Patterns Characterized by Distinct Tumor Microenvironment Immune Profiles in colon cancerA Regulator-Based Methylation Modification Patterns Characterized by Distinct Tumor Microenvironment Immune Profiles in colon Cancer. Theranostics 11 (5), 2201–2217. doi:10.7150/thno.52717
Chong, W., Wang, Z., Shang, L., Jia, S., Liu, J., Fang, Z., et al. (2021). Association of Clock-like Mutational Signature with Immune Checkpoint Inhibitor Outcome in Patients with Melanoma and NSCLC. Mol. Ther. - Nucleic Acids 23, 89–100. doi:10.1016/j.omtn.2020.10.033
Dai, W., Li, Y., Mo, S., Feng, Y., Zhang, L., Xu, Y., et al. (2018). A Robust Gene Signature for the Prediction of Early Relapse in Stage I-III colon Cancer. Mol. Oncol. 12 (4), 463–475. doi:10.1002/1878-0261.12175
Davoli, T., Uno, H., Wooten, E. C., and Elledge, S. J. (2017). Tumor Aneuploidy Correlates with Markers of Immune Evasion and with Reduced Response to Immunotherapy. Science 355 (6322). doi:10.1126/science.aaf8399
Eddy, K., and Chen, S. (2020). Overcoming Immune Evasion in Melanoma. Ijms 21 (23), 8984. doi:10.3390/ijms21238984
Erkes, D. A., Cai, W., Sanchez, I. M., Purwin, T. J., Rogers, C., Field, C. O., et al. (2020). Mutant BRAF and MEK Inhibitors Regulate the Tumor Immune Microenvironment via Pyroptosis. Cancer Discov. 10 (2), 254–269. doi:10.1158/2159-8290.Cd-19-0672
Finotello, F., and Trajanoski, Z. (2018). Quantifying Tumor-Infiltrating Immune Cells from Transcriptomics Data. Cancer Immunol. Immunother. 67 (7), 1031–1040. doi:10.1007/s00262-018-2150-z
Galon, J., and Bruni, D. (2019). Approaches to Treat Immune Hot, Altered and Cold Tumours with Combination Immunotherapies. Nat. Rev. Drug Discov. 18 (3), 197–218. doi:10.1038/s41573-018-0007-y
Ghandi, M., Huang, F. W., Jané-Valbuena, J., Kryukov, G. V., Lo, C. C., McDonald, E. R., et al. (2019). Next-generation Characterization of the Cancer Cell Line Encyclopedia. Nature 569 (7757), 503–508. doi:10.1038/s41586-019-1186-3
Han, D., Liu, J., Chen, C., Dong, L., Liu, Y., Chang, R., et al. (2019). Anti-tumour Immunity Controlled through mRNA m6A Methylation and YTHDF1 in Dendritic Cells. Nature 566 (7743), 270–274. doi:10.1038/s41586-019-0916-x
Hänzelmann, S., Castelo, R., and Guinney, J. (2013). GSVA: Gene Set Variation Analysis for Microarray and RNA-Seq Data. BMC Bioinformatics 14, 7. doi:10.1186/1471-2105-14-7
Hazra, A., and Gogtay, N. (2016). Biostatistics Series Module 3: Comparing Groups: Numerical Variables. Indian J. Dermatol. 61 (3), 251–260. doi:10.4103/0019-5154.182416
He, L., Li, H., Wu, A., Peng, Y., Shu, G., and Yin, G. (2019). Functions of N6-Methyladenosine and its Role in Cancer. Mol. Cancer 18 (1), 176. doi:10.1186/s12943-019-1109-9
Hegde, P. S., Karanikas, V., and Evers, S. (2016). The where, the when, and the How of Immune Monitoring for Cancer Immunotherapies in the Era of Checkpoint Inhibition. Clin. Cancer Res. 22 (8), 1865–1874. doi:10.1158/1078-0432.Ccr-15-1507
Huang, X., Zhang, H., Guo, X., Zhu, Z., Cai, H., and Kong, X. (2018). Insulin-like Growth Factor 2 mRNA-Binding Protein 1 (IGF2BP1) in Cancer. J. Hematol. Oncol. 11, 88. doi:10.1186/s13045-018-0628-y
Jia, Q., Wu, W., Wang, Y., Alexander, P. B., Sun, C., Gong, Z., et al. (2018). Local Mutational Diversity Drives Intratumoral Immune Heterogeneity in Non-small Cell Lung Cancer. Nat. Commun. 9 (1), 5361. doi:10.1038/s41467-018-07767-w
Jiang, P., Gu, S., Pan, D., Fu, J., Sahu, A., Hu, X., et al. (2018). Signatures of T Cell Dysfunction and Exclusion Predict Cancer Immunotherapy Response. Nat. Med. 24 (10), 1550–1558. doi:10.1038/s41591-018-0136-1
Kandoth, C., McLellan, M. D., Vandin, F., Ye, K., Niu, B., Lu, C., et al. (2013). Mutational Landscape and Significance across 12 Major Cancer Types. Nature 502 (7471), 333–339. doi:10.1038/nature12634
Lan, T., Li, H., Zhang, D., Xu, L., Liu, H., Hao, X., et al. (2019). KIAA1429 Contributes to Liver Cancer Progression through N6-methyladenosine-dependent post-transcriptional Modification of GATA3. Mol. Cancer 18 (1), 186. doi:10.1186/s12943-019-1106-z
Lawrence, M. S., Stojanov, P., Polak, P., Kryukov, G. V., Cibulskis, K., Sivachenko, A., et al. (2013). Mutational Heterogeneity in Cancer and the Search for New Cancer-Associated Genes. Nature 499 (7457), 214–218. doi:10.1038/nature12213
Lee, J. H., Kim, M. S., Yoo, N. J., and Lee, S. H. (2016). Absence of KNSTRN Mutation, a Cutaneous Squamous Carcinoma-specific Mutation, in Other Solid Tumors and Leukemias. Pathol. Oncol. Res. 22 (1), 227–228. doi:10.1007/s12253-015-9993-9
Lewinska, A., Adamczyk-Grochala, J., Deregowska, A., and Wnuk, M. (2017). Sulforaphane-Induced Cell Cycle Arrest and Senescence Are Accompanied by DNA Hypomethylation and Changes in microRNA Profile in Breast Cancer Cells. Theranostics 7 (14), 3461–3477. doi:10.7150/thno.20657
Li, K., Huang, F., Li, Y., Li, D., Lin, H., Ni, R., et al. (2020). Stabilization of Oncogenic Transcripts by the IGF2BP3/ELAVL1 Complex Promotes Tumorigenicity in Colorectal Cancer. Am. J. Cancer Res. 10 (8), 2480–2494.
Li, X.-C., Jin, F., Wang, B.-Y., Yin, X.-J., Hong, W., and Tian, F.-J. (2019). The m6A Demethylase ALKBH5 Controls Trophoblast Invasion at the Maternal-Fetal Interface by Regulating the Stability of CYR61 mRNA. Theranostics 9 (13), 3853–3865. doi:10.7150/thno.31868
Li, X., Wen, D., Li, X., Yao, C., Chong, W., and Chen, H. (2020). Identification of an Immune Signature Predicting Prognosis Risk and Lymphocyte Infiltration in Colon Cancer. Front. Immunol. 11, 1678. doi:10.3389/fimmu.2020.01678
Liu, D., Schilling, B., Liu, D., Sucker, A., Livingstone, E., Jerby-Arnon, L., et al. (2019). Integrative Molecular and Clinical Modeling of Clinical Outcomes to PD1 Blockade in Patients with Metastatic Melanoma. Nat. Med. 25 (12), 1916–1927. doi:10.1038/s41591-019-0654-5
Love, M. I., Huber, W., and Anders, S. (2014). Moderated Estimation of Fold Change and Dispersion for RNA-Seq Data with DESeq2. Genome Biol. 15 (12), 550. doi:10.1186/s13059-014-0550-8
Mariathasan, S., Turley, S. J., Nickles, D., Castiglioni, A., Yuen, K., Wang, Y., et al. (2018). TGFβ Attenuates Tumour Response to PD-L1 Blockade by Contributing to Exclusion of T Cells. Nature 554 (7693), 544–548. doi:10.1038/nature25501
Martínez-Riaño, A., Bovolenta, E. R., Boccasavia, V. L., Ponomarenko, J., Abia, D., Oeste, C. L., et al. (2019). RRAS2 Shapes the TCR Repertoire by Setting the Threshold for Negative Selection. J. Exp. Med. 216 (10), 2427–2447. doi:10.1084/jem.20181959
Mayakonda, A., Lin, D.-C., Assenov, Y., Plass, C., and Koeffler, H. P. (2018). Maftools: Efficient and Comprehensive Analysis of Somatic Variants in Cancer. Genome Res. 28 (11), 1747–1756. doi:10.1101/gr.239244.118
Mazurkiewicz, J., Simiczyjew, A., Dratkiewicz, E., Ziętek, M., Matkowski, R., and Nowak, D. (2021). Stromal Cells Present in the Melanoma Niche Affect Tumor Invasiveness and its Resistance to Therapy. Ijms 22 (2), 529. doi:10.3390/ijms22020529
Müller, S., Bley, N., Glass, M., Busch, B., Rousseau, V., Misiak, D., et al. (2018). IGF2BP1 Enhances an Aggressive Tumor Cell Phenotype by Impairing miRNA-Directed Downregulation of Oncogenic Factors. Nucleic Acids Res. 46 (12), 6285–6303. doi:10.1093/nar/gky229
Müller, S., Glaß, M., Singh, A. K., Haase, J., Bley, N., Fuchs, T., et al. (2019). IGF2BP1 Promotes SRF-dependent Transcription in Cancer in a m6A- and miRNA-dependent Manner. Nucleic Acids Res. 47 (1), 375–390. doi:10.1093/nar/gky1012
Newman, A. M., Steen, C. B., Liu, C. L., Gentles, A. J., Chaudhuri, A. A., Scherer, F., et al. (2019). Determining Cell Type Abundance and Expression from Bulk Tissues with Digital Cytometry. Nat. Biotechnol. 37 (7), 773–782. doi:10.1038/s41587-019-0114-2
Pagès, F., Mlecnik, B., Marliot, F., Bindea, G., Ou, F. S., Bifulco, C., et al. (2018). International Validation of the Consensus Immunoscore for the Classification of colon Cancer: a Prognostic and Accuracy Study. Lancet 391 (10135), 2128–2139. doi:10.1016/S0140-6736(18)30789-X
Panagi, M., Voutouri, C., Mpekris, F., Papageorgis, P., Martin, M. R., Martin, J. D., et al. (2020). TGF-β Inhibition Combined with Cytotoxic Nanomedicine Normalizes Triple Negative Breast Cancer Microenvironment towards Anti-tumor Immunity. Theranostics 10 (4), 1910–1922. doi:10.7150/thno.36936
Ribeiro, G. E., Leon, L. E., Perez, R., Cuiza, A., Vial, P. A., Ferres, M., et al. (2019). Deletions in Genes Participating in Innate Immune Response Modify the Clinical Course of Andes Orthohantavirus Infection. Viruses 11 (8), 680. doi:10.3390/v11080680
Ritchie, M. E., Phipson, B., Wu, D., Hu, Y., Law, C. W., Shi, W., et al. (2015). Limma powers Differential Expression Analyses for RNA-Sequencing and Microarray Studies. Nucleic Acids Res. 43 (7), e47. doi:10.1093/nar/gkv007
Schmitz, L., Grinblat, B., Novak, B., Hoeh, A. K., Händschke, K., von Dobbeler, C., et al. (2019). Somatic Mutations in Kinetochore Gene KNSTRN Are Associated with Basal Proliferating Actinic Keratoses and Cutaneous Squamous Cell Carcinoma. J. Eur. Acad. Dermatol. Venereol. 33 (8), 1535–1540. doi:10.1111/jdv.15615
Shulman, Z., and Stern-Ginossar, N. (2020). The RNA Modification N6-Methyladenosine as a Novel Regulator of the Immune System. Nat. Immunol. 21 (5), 501–512. doi:10.1038/s41590-020-0650-4
Subramanian, A., Tamayo, P., Mootha, V. K., Mukherjee, S., Ebert, B. L., Gillette, M. A., et al. (2005). Gene Set Enrichment Analysis: a Knowledge-Based Approach for Interpreting Genome-wide Expression Profiles. Proc. Natl. Acad. Sci. 102 (43), 15545–15550. doi:10.1073/pnas.0506580102
Tauriello, D. V. F., Palomo-Ponce, S., Stork, D., Berenguer-Llergo, A., Badia-Ramentol, J., Iglesias, M., et al. (2018). TGFβ Drives Immune Evasion in Genetically Reconstituted colon Cancer Metastasis. Nature 554 (7693), 538–543. doi:10.1038/nature25492
Thorsson, V., Gibbs, D. L., Brown, S. D., Wolf, D., Bortone, D. S., Ou Yang, T. H., et al. (2018). The Immune Landscape of Cancer. Immunity 48 (4), 812–e14. doi:10.1016/j.immuni.2018.03.023
Topalian, S. L., Taube, J. M., Anders, R. A., and Pardoll, D. M. (2016). Mechanism-driven Biomarkers to Guide Immune Checkpoint Blockade in Cancer Therapy. Nat. Rev. Cancer 16 (5), 275–287. doi:10.1038/nrc.2016.36
Wang, H., Hu, X., Huang, M., Liu, J., Gu, Y., Ma, L., et al. (2019). Mettl3-mediated mRNA m6A Methylation Promotes Dendritic Cell Activation. Nat. Commun. 10 (1), 1898. doi:10.1038/s41467-019-09903-6
Wang, W., Shao, F., Yang, X., Wang, J., Zhu, R., Yang, Y., et al. (2021). METTL3 Promotes Tumour Development by Decreasing APC Expression Mediated by APC mRNA N6-methyladenosine-dependent YTHDF Binding. Nat. Commun. 12 (1), 3803. doi:10.1038/s41467-021-23501-5
Wang, Y., Wang, Y., Luo, W., Song, X., Huang, L., Xiao, J., et al. (2020). Roles of Long Non-coding RNAs and Emerging RNA-Binding Proteins in Innate Antiviral Responses. Theranostics 10 (20), 9407–9424. doi:10.7150/thno.48520
Xia, Z., Tang, M., Ma, J., Zhang, H., Gimple, R. C., Prager, B. C., et al. (2021). Epitranscriptomic Editing of the RNA N6-Methyladenosine Modification by dCasRx Conjugated Methyltransferase and Demethylase. Nucleic Acids Res. 49, 7361–7374. doi:10.1093/nar/gkab517
Yang, S., Wei, J., Cui, Y.-H., Park, G., Shah, P., Deng, Y., et al. (2019). m6A mRNA Demethylase FTO Regulates Melanoma Tumorigenicity and Response to Anti-PD-1 blockadeA mRNA Demethylase FTO Regulates Melanoma Tumorigenicity and Response to Anti-PD-1 Blockade. Nat. Commun. 10 (1), 2782. doi:10.1038/s41467-019-10669-0
Yoshihara, K., Shahmoradgoli, M., Martínez, E., Vegesna, R., Kim, H., Torres-Garcia, W., et al. (2013). Inferring Tumour Purity and Stromal and Immune Cell Admixture from Expression Data. Nat. Commun. 4, 2612. doi:10.1038/ncomms3612
Zaccara, S., Ries, R. J., and Jaffrey, S. R. (2019). Reading, Writing and Erasing mRNA Methylation. Nat. Rev. Mol. Cel. Biol. 20 (10), 608–624. doi:10.1038/s41580-019-0168-5
Zeng, D., Ye, Z., Wu, J., Zhou, R., Fan, X., Wang, G., et al. (2020). Macrophage Correlates with Immunophenotype and Predicts Anti-PD-L1 Response of Urothelial Cancer. Theranostics 10 (15), 7002–7014. doi:10.7150/thno.46176
Zhang, B., Wu, Q., Li, B., Wang, D., Wang, L., and Zhou, Y. L. (2020). m6A Regulator-Mediated Methylation Modification Patterns and Tumor Microenvironment Infiltration Characterization in Gastric cancerA Regulator-Mediated Methylation Modification Patterns and Tumor Microenvironment Infiltration Characterization in Gastric Cancer. Mol. Cancer 19 (1), 53. doi:10.1186/s12943-020-01170-0
Keywords: skin cutaneous melanoma, methylation of N6 adenosine modification, tumor microenvironment, immune profiles, immunotherapy
Citation: Du F, Li H, Li Y, Liu Y, Li X, Dang N, Chu Q, Yan J, Fang Z, Wu H, Zhang Z, Zhu X and Li X (2021) Identification of m6A Regulator-Associated Methylation Modification Clusters and Immune Profiles in Melanoma. Front. Cell Dev. Biol. 9:761134. doi: 10.3389/fcell.2021.761134
Received: 19 August 2021; Accepted: 25 October 2021;
Published: 21 December 2021.
Edited by:
Yicheng Long, Cornell University, United StatesReviewed by:
Cai Chen, Merck (United States), United StatesXueqian Zhuang, Memorial Sloan Kettering Cancer Center, United States
Mingqiang Wang, Stanford University, United States
Copyright © 2021 Du, Li, Li, Liu, Li, Dang, Chu, Yan, Fang, Wu, Zhang, Zhu and Li. This is an open-access article distributed under the terms of the Creative Commons Attribution License (CC BY). The use, distribution or reproduction in other forums is permitted, provided the original author(s) and the copyright owner(s) are credited and that the original publication in this journal is cited, in accordance with accepted academic practice. No use, distribution or reproduction is permitted which does not comply with these terms.
*Correspondence: Xiaokang Li, c2lsdWthbmdrYW5nQDE2My5jb20=
†These authors have contributed equally to this work