- 1Department of General Surgery, Shanghai General Hospital, Shanghai Jiao Tong University School of Medicine, Shanghai, China
- 2Department of Cardiology, Shanghai General Hospital, Shanghai Jiao Tong University School of Medicine, Shanghai, China
Background: Liver hepatocellular carcinoma (LIHC) is the third leading cause of cancer-related death and the sixth most common solid tumor worldwide. In the tumor microenvironment, the cross-talk between cancer cells, immune cells, and stromal cells exerts significant effects on neoplasia and tumor development and is modulated in part by chemokines. Chemokine (C-C motif) ligands (CCL) can directly target tumor cells and stromal cells, and they have been shown to regulate tumor cell proliferation, cancer stem-like cell properties, cancer invasiveness and metastasis, which directly and indirectly affect tumor immunity and influence cancer progression, therapy and patient outcomes. However, the prognostic values of chemokines CCL in LIHC have not been clarified.
Methods: In this study, we comprehensively analyzed the relationship between transcriptional chemokines CCL and disease progression of LIHC using the ONCOMINE dataset, GEPIA, UALCAN, STRING, WebGestalt, GeneMANIA, TRRUST, DAVID 6.8, LinkedOmics, TIMER, GSCALite, and Open Targets. We validated the protein levels of chemokines CCL through western blot and immunohistochemistry.
Results: The transcriptional levels of CCL5/8/11/13/15/18/20/21/25/26/27/28 in LIHC tissues were significantly elevated while CCL2/3/4/14/23/24 were significantly reduced. A significant correlation was found between the expression of CCL14/25 and the pathological stage of LIHC patients. LIHC patients with low transcriptional levels of CCL14/21 were associated with a significantly poor prognosis. The functions of differentially expressed chemokines CCL were primarily related to the chemokine signaling pathway, cytokine–cytokine receptor interactions, and TNF-α signaling pathway. Our data suggested that RELA/REL, NFKB1, STAT1/3/6, IRF3, SPI1, and JUN were key transcription factors for chemokines CCL. We found significant correlations among the expression of chemokines CCL and the infiltration of six types of immune cells (B cells, CD8+ T cells, CD4+ T cells, macrophages, neutrophils, and dendritic cells) and immune checkpoints (PD-1. PD-L1, and CTLA-4). The western blot and immunohistochemistry results showed that protein expression levels of CCL5 and CCL20 were upregulated in LIHC. CCL5 and CCL20 were significantly correlated with the clinical outcome of patients with LIHC, and could be negatively regulated by some drugs or small molecules.
Conclusions: Our results may provide novel insights for the potential suitable targets of immunological therapy and prognostic biomarkers for LIHC.
Introduction
Primary liver cancer is one of the most common malignant tumors of the digestive system. According to the latest statistics from the WHO, there are 906,000 newly diagnosed cases and 830,000 deaths worldwide in 2020, making it the sixth most common tumor in the world and the third cancer-related death in humans (Sung et al., 2021). Liver hepatocellular carcinoma (LIHC) is the most important pathological type of primary liver cancer, accounting for about 75–85% (Sung et al., 2021). At present, surgical resection and liver transplantation are still the most effective strategies for the treatment of LIHC, especially for patients in the early stage of the disease with a better prognosis (Forner et al., 2018). However, due to the late clinical symptoms of LIHC and the high aggressiveness of tumors, many patients with LIHC are already at an advanced stage when they are diagnosed, and they miss the best opportunity for surgical treatment (Llovet et al., 2008). In recent years, an increasing number of studies have shown that molecular targeted therapy and immunotherapy occupy an important position in the treatment options of LIHC patients (Llovet et al., 2018; Greten et al., 2019). The liver has been recognized as an immune privilege organ (Demetris et al., 2016; Tiegs and Lohse, 2010), therefore, and identifying more therapeutic targets and prognostic biomarkers related to the tumor microenvironment is an important consideration in the current clinical management of LIHC.
The chemokine system contains genes encoding 50 chemokine ligands and 20 homologous chemokine receptors (Zlotnik and Yoshie, 2000). Chemokines and their corresponding chemokine receptors are key regulators that chemoattract immune cells and drive the inflammatory response to specific triggers (Zimmermann and Tacke, 2011). In the tumor microenvironment, the cross-talk between cancer cells, immune cells, and stromal cells exerts significant effects on neoplasia and tumor development, and chemokines can be expressed by these cells and participate in its regulation (Nagarsheth et al., 2017). A large amount of research shows that the chemokines can directly target tumor cells and stromal cells, and they have been shown to regulate tumor cell proliferation, cancer stem-like cell properties, cancer invasiveness and metastasis, which directly and indirectly affect tumor immunity and influence cancer progression, tumor therapy and patient outcomes (Atretkhany et al., 2016; Nagarsheth et al., 2017; Mollica et al., 2019). The CC (β) subfamily of chemokines, a group of chemotactic cytokines known as CCL1–28, some of which have been confirmed to be related to the development and progression of LIHC (Schneider et al., 2012; Yang et al., 2012; Zhao et al., 2021). Zhu et al. have reported that CCL14 is significantly downregulated in LIHC tumor tissues compared with peritumor tissues (Zhu et al., 2019). As a tumor suppressor, CCL14 suppressed the proliferation and promoted the apoptosis of HCC cells via inhibiting the Wnt/β-catenin-signaling pathway. Furthermore, CCL2, CCL5, and CCL7 secreted by cancer-associated fibroblasts have been shown to promote HCC metastasis by activating the hedgehog and transforming growth factor-β (TGF-β) pathway (Liu et al., 2016). Similarly, CCL15 was found to be abundantly expressed in HCC, which could recruit inflammatory monocytes in the tumor microenvironment and lead to the metastasis of HCC cells (Liu et al., 2019). Therefore, the role of chemokines CCL in the LIHC tumor microenvironment is worthy of further exploration.
Although some studies have characterized the general expression profile and functional mechanism of some chemokine CCL in LIHC, determining the appropriate chemokine CCL as a therapeutic target and prognostic biomarker of LIHC is still an enormous issue that needs urgent attention. In this study, we will use the current rapidly developing sequencing technology and various databases, combined with bioinformatics analysis, to conduct an in-depth and comprehensive discussion on the expression of chemokines CCL in LIHC, and then evaluate them as potential therapeutic targets and prognostic markers. What we have done is to provide some additional recommendations for clinically related treatment strategies of LIHC, which are conducive to the prognosis and long-term survival of patients.
Materials and Methods
ONCOMINE
ONCOMINE (www.oncomine.org), an online database that integrates RNA and DNA-seq from Gene Expression Omnibus (GEO), The Cancer Genome Atlas (TCGA), and published literature sources, was used to evaluate the expression of chemokines CCL in LIHC (Rhodes et al., 2004). A p 0.05, a fold change of 2, and a gene rank in the top 10% were set as the significance thresholds, respectively. If the data conformed to the normal distribution, the Student’s t test or Welch’s t test was used to analyze the differences in the expression of chemokines CCL in HCC, otherwise the Mann-Whitney test was used.
GEPIA
GEPIA (Gene Expression Profiling Interactive Analysis, http://gepia.cancer-pku.cn/index.html) is currently a valuable and highly cited resource for gene expression analysis based on tumor and normal samples from TCGA and Genotype-Tissue Expression (GTEx) databases (Tang et al., 2017). In this study, based on the “LIHC” dataset, we used the single gene analysis and the multiple gene analysis module to analyze the mRNA expression levels of chemokines CCL and the prognostic correlation. The p value cutoff was set as 0.05. Log-rank test, a.k.a the Mantel-Cox test, and Kaplan–Meier curve were used for hypothesis test.
UALCAN
UALCAN (http://ualcan.path.uab.edu/analysis.html) is a comprehensive, user-friendly, and interactive web resource for analyzing cancer OMICS data. It is built on PERL-CGI with high quality graphics using javascript and CSS (Chandrashekar et al., 2017). We analyzed the mRNA expression levels of chemokines CCL using the “LIHC” dataset. The student’s t test was used in the database analysis and the p value cutoff was set as 0.05.
STRING
STRING (https://string-db.org/) is an online database for searching known protein interaction relationships, which aims to collect, score, and integrate all publicly available sources of protein–protein interaction (PPI) data, and to complement these with computational predictions of potential functions (Szklarczyk et al., 2019). We conducted a PPI network analysis of differentially expressed chemokines CCL to explore the interactions among them with STRING.
WebGestalt
WebGestalt (WEB-based Gene SeT AnaLysis Toolkit, http://www.webgestalt.org/) is a functional enrichment analysis web tool, which has on average 26,000 unique users from 144 countries and territories per year according to Google Analytics (Liao et al., 2019). In our study, the “PPI BIOGRID” of “Network Topology-based Analysis (NTA)” method was used to analyze the subnetwork and interactions among chemokines CCL.
GeneMANIA
GeneMANIA (http://www.genemania.org) is a flexible, user-friendly web interface for generating hypotheses about gene function, analyzing gene lists and prioritizing genes for functional assays (Warde-Farley et al., 2010).
David 6.8
DAVID 6.8 (https://david.ncifcrf.gov/home.jsp) is a comprehensive set of functional annotation tools for investigators to understand biological meaning behind large list of genes (Huang et al., 2009). In the study, the Gene Ontology (GO) enrichment analysis and Kyoto Encyclopedia of Genes and Genomes (KEGG) pathway enrichment analysis of differentially expressed chemokines CCL and their neighboring genes were performed using DAVID 6.8. Then we visualized the results through the “ggplot2,” “dplyr,” and “stringi” packages of R project and a p < 0.05. Biological processes (BP), cellular components (CC), and molecular function (MF) were included in the GO enrichment analysis.
TRRUST
TRRUST (https://www.grnpedia.org/trrust/) a manually curated database of human and mouse transcriptional regulatory networks. The current version of TRRUST contains 8,444 transcription factor (TF)-target regulatory relationships of 800 human TFs. The TRRUST database can provide information of mode of regulation (Han et al., 2018). The Fisher’s exact test and the Benjamini–Hochberg procedure were utilized in the database analysis.
TIMER
TIMER (https://cistrome.shinyapps.io/timer/) is a comprehensive resource for systematical analysis of immune infiltrates across diverse cancer types (Li et al., 2017). In this study, “Gene module,” “Survival module,” and “Correlation module” were used to evaluate the correlation among clinical outcome and the infiltration of immune cells and aberrant expressions of chemokines CCL in patient with LIHC. The Spearman’s rho value and estimated statistical significance were also shown in the database.
Open Targets
Open Targets provide a target-centric workflow to aid in identifying diseases potentially related to specific targets (Koscielny et al., 2017). Here, we used Open Targets to explore diseases associated with CCL5 and CCL20.
GSCALite
GSCALite (http://bioinfo.life.hust.edu.cn/web/GSCALite/) is a web-based analysis platform for gene set cancer analysis. Genomic aberrations influence clinical responses to treatment and are potential biomarkers for drug screening (Liu et al., 2018). We integrated the drug sensitivity and gene expression profile data of cancer cell lines in Genomics of Drug Sensitivity in Cancer (GDSC) and Therapeutics Response Portal (CTRP) for research. The expression of each gene in the genome and the small molecule/drug sensitivity (IC50) were analyzed by Spearman correlation. p values less than 0.05 were considered statistically significant.
Cell Culture
L02 cells, Huh7 cells, HCC-LM3 cells, HepG2 cells, and Hep3B cells were cultured using DMEM (Gibco, Thermo Fisher Scientific, Waltham, MA, USA) supplemented with 10% FBS (Gibco) and 1% penicillin-streptomycin, in 5% CO2 at 37°C.
Western Blot
LIHC tissues and adjacent normal tissues were obtained at Shanghai General Hospital from 2016 to 2018 and used in our study. Written informed consent was obtained from each patient. This study was approved by ethics committee of Shanghai General Hospital. Protein extracted from tissues or cell cultures were using RIPA buffer (Thermo Fisher Scientific) mixed with PMSF (Beyotime, Shanghai, China) for 30 min on ice, and then centrifuged at 12,000 rpm for 10 min at 4°C. Protein lysates were separated using SDS-PAGE and transferred to PVDF membranes. After incubating with 5% Bovine serum albumin (BSA) for 1 h at room temperature (RT), the membranes were incubated with primary antibodies (CCL5, 1:100, AF5151; CCL20, 1: 100, DF2238) (Affinity, Changzhou, China) overnight at 4°C, washed with Tris Buffered Saline with Tween®20 (TBST) for 3 times, and further incubated with secondary antibodies (Sangon Biotech, Shanghai, China) for 1 h at RT, and developed using ECL solutions (Beyotime).
Immunohistochemistry
All formalin-fixed paraffin-embedded tissues were prepared for use. After oven-drying, dewaxing, antigen retrieval, incubating with endogenous peroxidase, and blocking treatments, the slides were incubated with primary antibodies overnight at 4°C, followed by incubating with HRP-conjugated secondary antibodies at RT for 1 h. The stain was then visualized by incubation in DAB and counterstained with hematoxylin. Finally, the slides were covered with resinene and then the staining intensity was observed under the microscope (tumor tissue, n = 5; adjacent tissue, n = 5).
Results
Differential Expression of Chemokines CCL in Patients With LIHC
Twenty-four chemokines CCL were determined using the ONCOMINE database, excluding CCL6, CCL9, and CCL10. We then explored the transcriptional levels of chemokines CCL in LIHC and compared to normal liver tissues with ONCOMINE. As shown in Figure 1 and Table 1, the transcriptional levels of CCL5, CCL15, CCL18, CCL20, and CCL21 in LIHC tissues were significantly upregulated while the transcriptional levels of CCL2, CCL3, CCL14, and CCL23 were significantly downregulated in LIHC compared to normal liver tissue. These results are consistent with Chen et al. who found a significant downregulation of CCL2 and CCL3 in LIHC(X. Chen et al., 2002). Wurmbach et al. also reported that the level of CCL2(p = 0.001) and CCL3(p = 0.0007) in LIHC were reduced with fold changes of −3.398 and −3.255, respectively (Wurmbach et al., 2007). Similarly, Wurmbach et al. revealed that the expression of CCL14 and CCL23 were decreased in LIHC (Wurmbach et al., 2007), which was consistent with the downregulation of CCL4 expression in the Roessler’s dataset (Roessler et al., 2010). In contrast, Mas et al. found that the transcription levels of CCL5 and CCL21 were significantly upregulated in LIHC (Mas et al., 2009). The fold change of CCL15 expression in LIHC was 2.168 (p = 0.0012) and 2.395 (p<0.0001) in the datasets of Wurmbach (Wurmbach et al., 2007) and Mas (Mas et al., 2009), respectively. Moreover, Wurmbach et al. suggested that CCL18 was significantly elevated in LIHC (Wurmbach et al., 2007). And The results of Wurmbach (Wurmbach et al., 2007) and Roessler (Roessler et al., 2010) all suggested that CCL20 was remarkably higher in LIHC tumors than in normal samples.
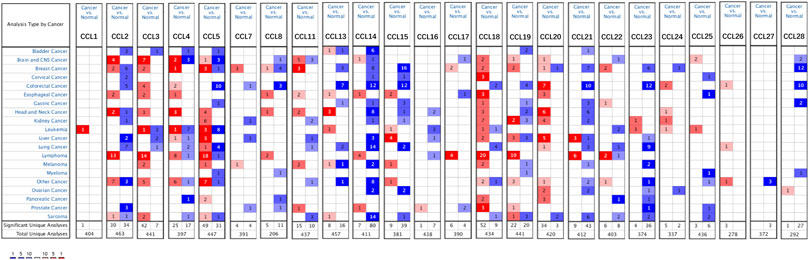
FIGURE 1. mRNA levels of chemokines CCL in LIHC (ONCOMINE). The figure shows the numbers of datasets with statistically significant mRNA upregulated (red) or downregulated expression (blue) of chemokines CCL.
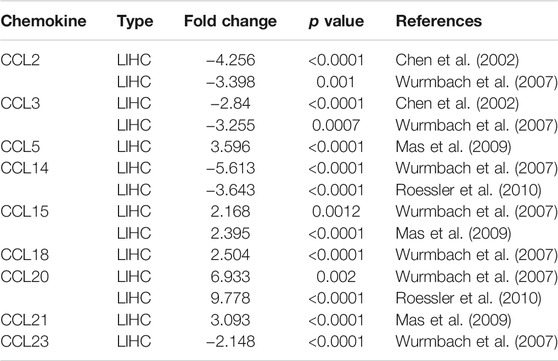
TABLE 1. The mRNA levels of aberrantly expressed chemokines CCL in LIHC tissues and normal liver tissues at transcriptome level (ONCOMINE).
We also used the UALCAN database to evaluate the expression levels of chemokines CCL in LIHC tissues and normal liver tissues. Consistent with the ONCOMINE data, the transcriptional levels of CCL3(p = 1.16E-04), CCL14(p = 1.77E-14), and CCL23(p = 3.65E-09) were significantly downregulated in LIHC compared to normal liver tissue, while the transcriptional levels of CCL15(p = 6.00E-15), CCL18(p = 4.68E-02), and CCL20(p = 1.04E-07) were significantly upregulated (Figure 2). In addition, the results of the UALCAN database showed that CCL4(p = 6.04E-04) and CCL24(p = 2.41E-02) were reduced remarkably and CCL8(p = 5.52E-05), CCL11(p = 2.04E-09), CCL13(p = 2.36E-08), CCL25(p = 1.70E-03), CCL26(p = 1.89E-08), CCL27(p = 1.80E-02), and CCL28(p = 2.97E-06) were elevated significantly in LIHC tumors compared with normal liver samples. Combining the results of the two databases, we have excluded those chemokines CCL whose expressions were not significantly different, including CCL1, CCL7, CCL12, CCL16, CCL17, CCL19, and CCL22.
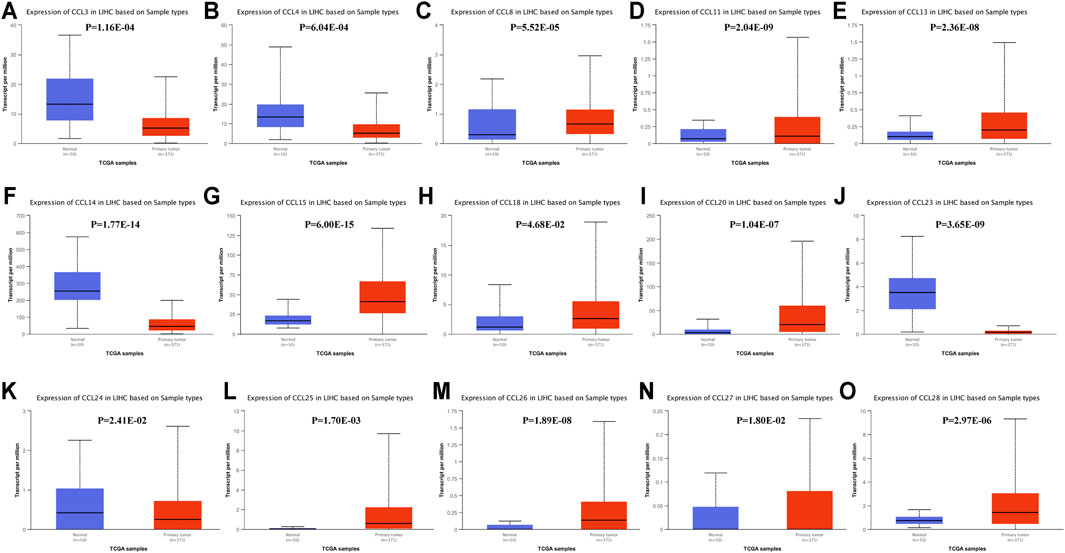
FIGURE 2. The transcription levels of chemokines CCL in LIHC (UALCAN). The transcriptional levels of (C) CCL8, (D) CCL11, (E) CCL13, (G) CCL15, (H) CCL18, (I) CCL20, (L) CXCL25, (M) CCL26, (N) CCL27, and (O) CCL28 in RCC tissues were significantly elevated while the transcriptional levels of (A) CCL3, (B) CCL4, (F) CCL14, (J) CCL23, and (K) CCL24 were remarkably reduced. p < 0.05 was considered statistically significant.
To identify chemokines CCL associated with tumorigenesis and progression in LIHC, we further explored the correlation between the expression of differentially expressed chemokines CCL and the pathological stage of LIHC patients. As expected, the expression of CCL14, CCL25, CCL26, and CCL28 were obviously related to the pathological stage (Figures 3A–D), and with the aggravation of tumor malignancy, the expression of CCL25, CCL26, and CCL28 were increased while CCL14 was decreased. As we have seen, there is a certain correlation between the expression of chemokines CCL and tumor progression of LIHC.
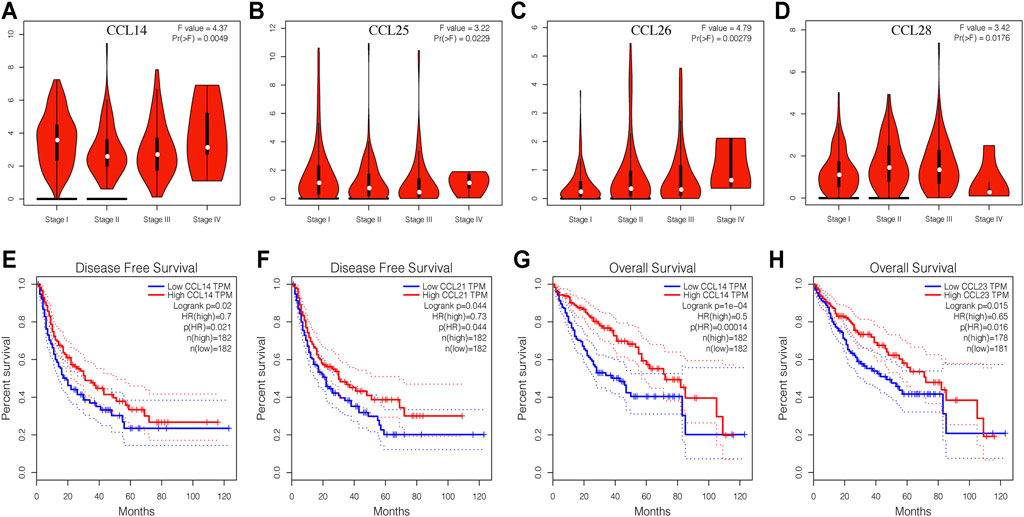
FIGURE 3. Correlation between aberrantly expressed chemokines CCL and the pathological stage, DFS, and OS of LIHC patients (GEPIA). (A–D) Correlation between the pathological stage and expression of CCL14, CCL25, CCL26, and CCL28, respectively. (E,F) The prognostic value of expression of CCL14 and CCL21 in LIHC patients in the DFS curve. (G,H) The prognostic value of expression of CCL14 and CCL23 in LIHC patients in the OS curve. p < 0.05 was considered statistically significant.
The Prognostic Value of Chemokines CCL in Patients With LIHC
We then used the GEPIA database to evaluate the correlation between the expression of differentially expressed chemokines CCL and the clinical prognosis of LIHC patients. As shown in Figures 3E,F, the high transcription levels of CCL14 (p = 0.021) and CCL21 (p = 0.044) in LIHC patients were significantly associated with better disease-free survival (DFS). More importantly, we also proved that LIHC patients with high CCL14 (Figure 3G) and CCL23 (Figure 3H) transcription levels have longer overall survival (OS).
PPI Network, and Interaction Analyses of Chemokines CCL in Patients With LIHC
To better understand the potential interactions between differentially expressed chemokines CCL, we used the STRING database to construct a PPI network (Figure 4A). The result revealed that there are 18 nodes and 84 edges in the PPI network. The functional enrichments in the network showed that these differentially expressed chemokines CCL was associated with the chemokine signaling pathway and the NF-κB signaling pathway. In addition, we further used WebGestalt to verify the interaction relationship of differentially expressed chemokines CCL (Figure 4B). The Top Ranking Neighbors associated with differentially expressed chemokines CCL including APP, ACKR2, VCAN, ACKR4, CCR3, SPATA20, NUDT3, CCR1, TGFB3, and CCR6. Moreover, GO enrichment analysis showed that the differential expression of chemokines CCL and their neighboring genes were related to chemotaxis and inflammatory response, G protein-coupled receptor signaling pathway, and cytokine-mediated signaling pathway (Figure 4C; Table 2). Similarly, GeneMANIA analysis demonstrated that the functions of chemokines CCL and their neighboring genes were mainly associated with cytokine activity, chemokine receptor binding, lymphocyte migration, response to tumor necrosis factor, etc. (Figure 4D).
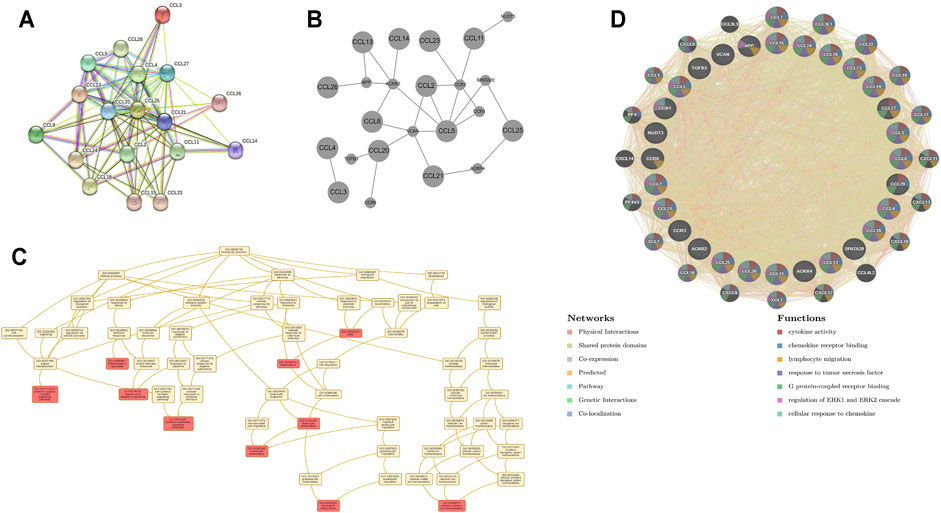
FIGURE 4. PPI network, neighboring gene network, interaction analyses and functional enrichment of aberrantly expressed chemokines CCL in LIHC patients. (A) PPI network of aberrantly expressed chemokines CCL. (B) Gene–gene interaction network of aberrantly expressed chemokines CCL and their most frequently altered neighboring genes. (C) GO enrichment of chemokines CCL and their neighboring genes. (D) PPI network and functional enrichment of chemokines CCL and their neighboring genes.
Transcription Factor Targets of Chemokines CCL in Patients With LIHC
Since the expression of chemokines CCL in LIHC tissues and normal liver tissues was significantly different, TRRUST was utilized to study the potential transcription factor targets of the differentially expressed chemokines CCL. All differentially expressed chemokines CCL were included in the TRRUST analysis, however, only 9 genes were involved, including CCL2, CCL3, CCL4, CCL5, CCL11, CCL13, CCL20, CCL21, and CCL26. This finding indicated that a total of 9 transcription factors (RELA, REL, NFKB1, STAT1/3/6, IRF3, SPI1, and JUN) were involved in the transcriptional regulation of chemokines CCL (Table 3). Among them, RELA and NFKB1 were pivotal transcription factors for CCL2, CCL3, CCL4, CCL5, CCL11, CCL13, and CCL20. Furthermore, REL, IRF3, SPI1, and JUN were pivotal transcription factors for CCL2 and CCL5. STAT1, STAT 3, STAT6 were the key transcription factors for CCL2 and CCL3, CCL2 and CCL11, CCL11 and CCL26, respectively.
Immune Cell Infiltration of Chemokines CCL in Patients With LIHC
In the tumor microenvironment, chemokines CCL are related to the inflammatory response and the regulation of immune cells, which affect the prognosis of patients with LIHC. Therefore, regarding the association between differential expression of chemokines CCL and immune cell infiltration, we performed a comprehensive exploration using the TIMER database. The expression of CCL2, CCL4, CCL5, CCL8, CCL11, CCL13, CCL18, CCL23, and CCL26 were positively associated with the infiltration of B cells, CD8+ T cells, CD4+ T cells, macrophages, neutrophils and dendritic cells (Figures 5A,C–G,J,M,P), while the expression of CCL15 was negatively associated with the infiltration of B cells, CD8+ T cells, CD4+ T cells, macrophages, neutrophils and dendritic cells (Figure 5I). The expression of CCL3 and CCL24 were positively associated with the infiltration of B cells, CD8+ T cells, macrophages, neutrophils and dendritic cells (all p < 0.05; Figures 5B,N). CCL14 expression was negatively associated with the infiltration of B cells, CD4+ T cells, macrophages, neutrophils and dendritic cells (all p < 0.05; Figure 5H). In contrast, CCL20 expression was positively associated with the infiltration of B cells, CD4+ T cells, macrophages, neutrophils and dendritic cells (all p < 0.05; Figure 5K). In addition, there was a positive correlation between CCL21 expression and the infiltration of B cells, CD8+ T cells, CD4+ T cells, macrophages, and dendritic cells (all p < 0.05; Figure 5L). CCL25 expression was negatively associated with the infiltration of macrophages (Cor = −0.111, p = 4.04e−2; Figure 5O). CCL27 expression was positively associated with the infiltration of CD8+ T cells (Cor = 0.125, p = 2.10e−2) and dendritic cells (Cor = 0.137, p = 1.13e−2; Figure 5Q). Except for B cells, the expression of CCL28 was positively correlated with the infiltration of CD8+ T cells, CD4+ T cells, macrophages, neutrophils and dendritic cells (all p < 0.05; Figure 5R). We also assessed the association of differentially expressed chemokines CCL and immune cells infiltration using multivariable Cox proportional hazard model. As shown in Table 4, B cells (p = 0.001), macrophages (p = 0.005), dendritic cells (p = 0), CCL2 expression (p = 0.04), CCL5 expression (p = 0.01), and CCL20 expression (p = 0.017) were significantly correlated with the clinical outcome of patients with LIHC.
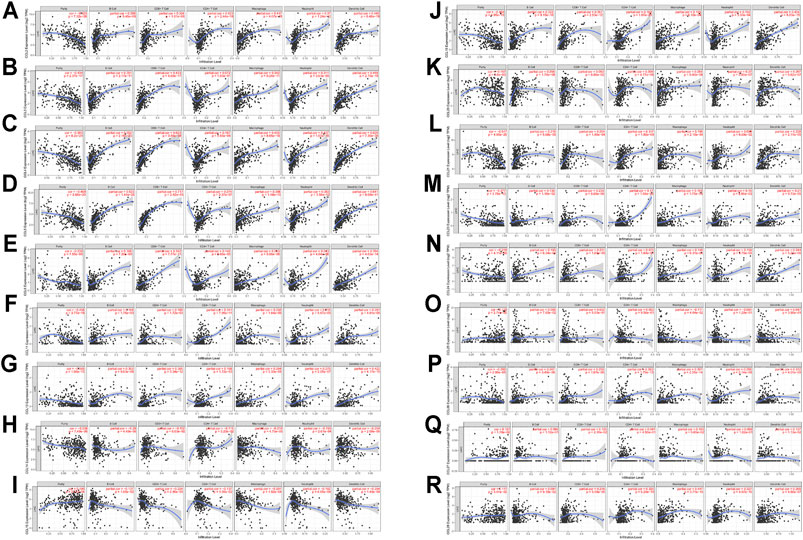
FIGURE 5. The correlation between aberrantly expressed chemokines CCL and immune cell infiltration (TIMER). The correlation between the numerous of immune cell and the expression of (A) CCL2, (B) CCL3, (C) CCL4, (D) CCL5, (E) CCL8, (F) CCL11, (G) CCL13, (H) CCL14, (I) CCL15, (J) CCL18, (K) CCL20, (L) CCL21, (M) CCL23, (N) CCL24, and (O) CCL25, (P) CCL26, (Q) CCL27, and (R) CCL28 in LIHC.
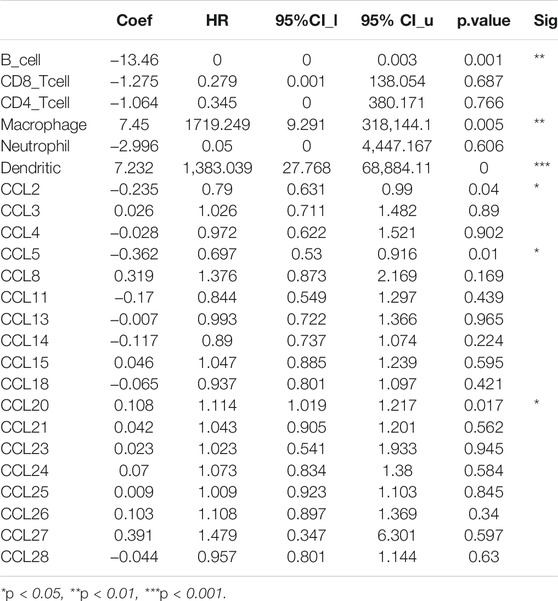
TABLE 4. The cox proportional hazard model of chemokines CCL and six tumor-infiltrating immune cells in LIHC (TIMER).
Correlation Between Immune Checkpoints and Chemokines CCL in Patients With LIHC
In order to explore the association between the chemokines CCL and immune checkpoints, we performed Correlation module analysis using the TIMER database. Based on the multivariable Cox proportional hazard model, we found that CCL2 and CCL5 were positively correlated with programmed cell death protein 1 (PD-1, also called PDCD1), programmed cell death-Ligand 1 (PD-L1, also called CD274), and cytotoxic T-lymphocyte-associated protein 4 (CTLA-4). In addition, CCL20 was also positively associated with PD-1 and CTLA-4 (Figure 6).
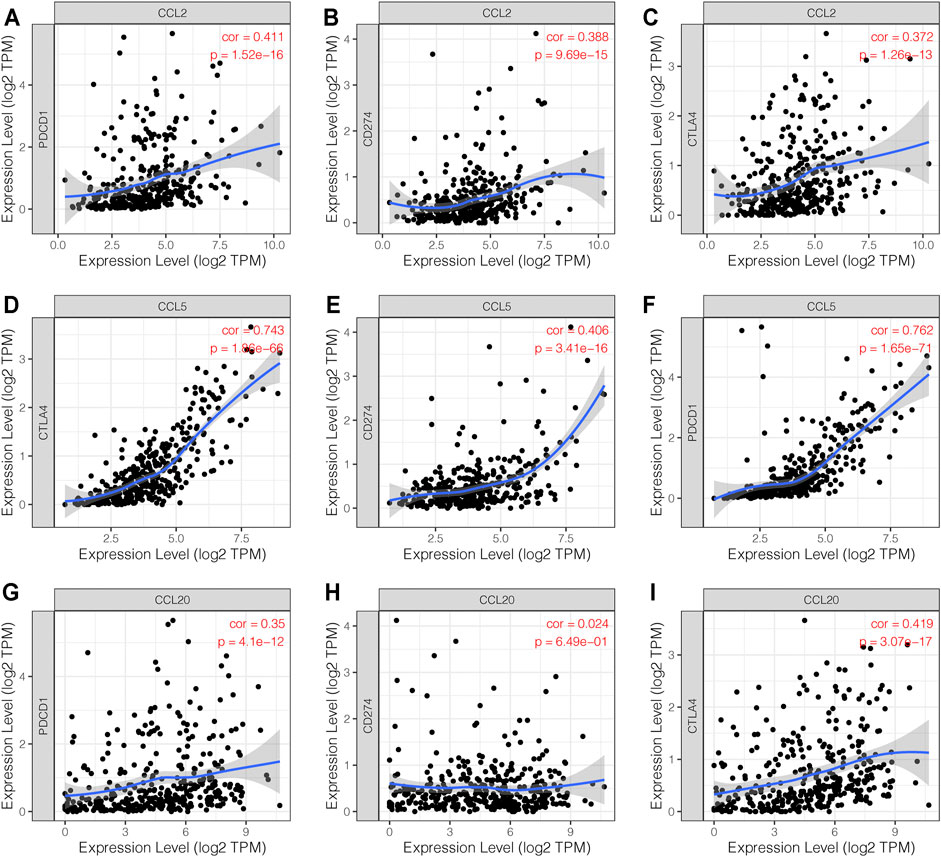
FIGURE 6. The correlation between chemokines CCL and immune checkpoints (TIMER). (A–F) The expression levels of CCL2 and CCL5 were positively associated with PD-1, PD-L1, and CTLA-4. (G–I) The expression level of CCL20 was positively associated with PD-1 and CTLA-4.
Functional Enrichment Analysis of Chemokines CCL in Patients With LIHC
To explore the specific mechanism of differentially expressed chemokines CCL and their neighboring genes in LIHC, we used DAVID 6.8 for functional enrichment analysis, which makes the most meaningful enrichment based on the significance of the p value (Figure 7). In the results, the GO BP category indicated that the disease progression of LIHC was related to chemokine-mediated signaling pathway, chemotaxis, cellular response to interferon-gamma, cellular response to interferon-1, and immune response. The extracellular space, extracellular region, and cell were the most highly enriched items in the GO CC category. Furthermore, the chemokine activity and chemokine receptor binding were primarily enriched items in the GO MF category. In addition, in the enrichment analysis of the KEGG pathway, the differentially expressed chemokines CCL and their neighboring genes were mainly enriched in chemokine signaling pathway, cytokine-cytokine receptor interaction, nuclear factor kappa B (NF-κB) signaling pathway, toll-like receptor (TLR) signaling pathway, and tumor necrosis factor (TNF) signaling pathway. It was worth noting that CCL5, and CCL20 were key regulators of the TNF signaling pathway.
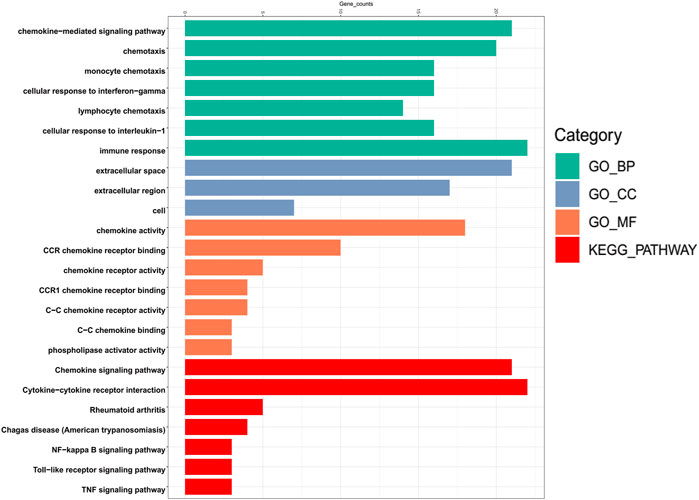
FIGURE 7. Bar plot of GO enrichment in cellular component terms, biological process terms, molecular function terms, and KEGG enriched terms of aberrantly expressed chemokines CCL and their most frequently altered neighboring genes in LIHC (David 6.8).
CCL5 and CCL20 Were Upregulated in Both HCC Cell Lines and Tumor Tissues
To test the protein expression levels of CCL5 and CCL20, we performed western blot and immunohistochemistry. As expected, CCL5 was dramatically upregulated in HCC cell lines, including Huh7, HepG2, HCC-LM3, and Hep3B, compared to human liver normal cell line L02 (Figure 8A). Similarly, the expression level of CCL20 was also significantly increased in Huh7, HepG2, and Hep3B cells compared to L02 cells (Figure 8A). Furthermore, the results of tissue samples have demonstrated that both CCL5 and CCL20 were markedly upregulated in HCC tumor tissues than in adjacent tissues (Figure 8B). Coincidentally, we also showed that the expressions of CCL5 and CCL20 were appreciably increased in HCC tumor tissues compared to adjacent tissues by immunohistochemistry (Figures 8C,D).
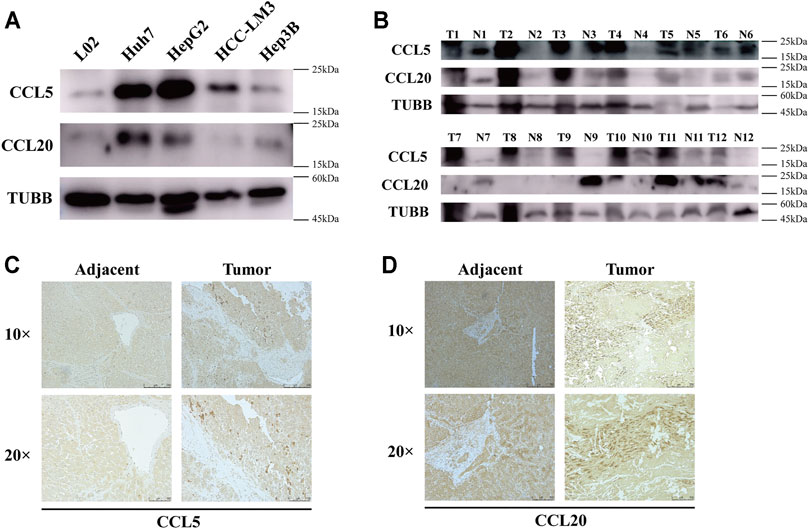
FIGURE 8. CCL5 and CCL20 expressions are increased in HCC cell lines and tumor tissues. (A) The protein levels of CCL5 and CCL20 in 4 HCC cell lines and human liver normal cell line determined by Western blot; TUBB was used as a control. (B) The protein levels of CCL5 and CCL20 in 12 HCC tissues and paired adjacent tissues determined by Western blot. (C) Representative images of CCL5 immunohistochemical staining in HCC tissues and paired adjacent tissues. Scale bar = 250 and 100 μm, respectively. (D) Representative images of CCL20 immunohistochemical staining in HCC tissues and paired adjacent tissues. Scale bar = 250 and 100 μm, respectively.
Disease Susceptibility and Drug Sensitivity Analysis of Aberrant Expression of Chemokines CCL
To further determine the disease caused by the aberrant expression of CCL5, and CCL20, we performed an analysis based on the Open Targets database. The findings showed that these chemokines CCL were significantly related to gastrointestinal disease, endocrine system disease, and the disease of cell proliferation disorder (Figures 9A,B). Among the GDSC database, the results indicated that there were 3 and 1 drugs or small molecules that could target the expression of CCL5 and CCL20, respectively (Figure 9C). Among them, CCL5 was negatively regulated by VNLG/124, KIN001-260, and ATRA, while CCL20 was negatively regulated by Trametinib. These analyses provided potential strategies for the clinical treatment of aberrant chemokines CCL expression in patients with LIHC.
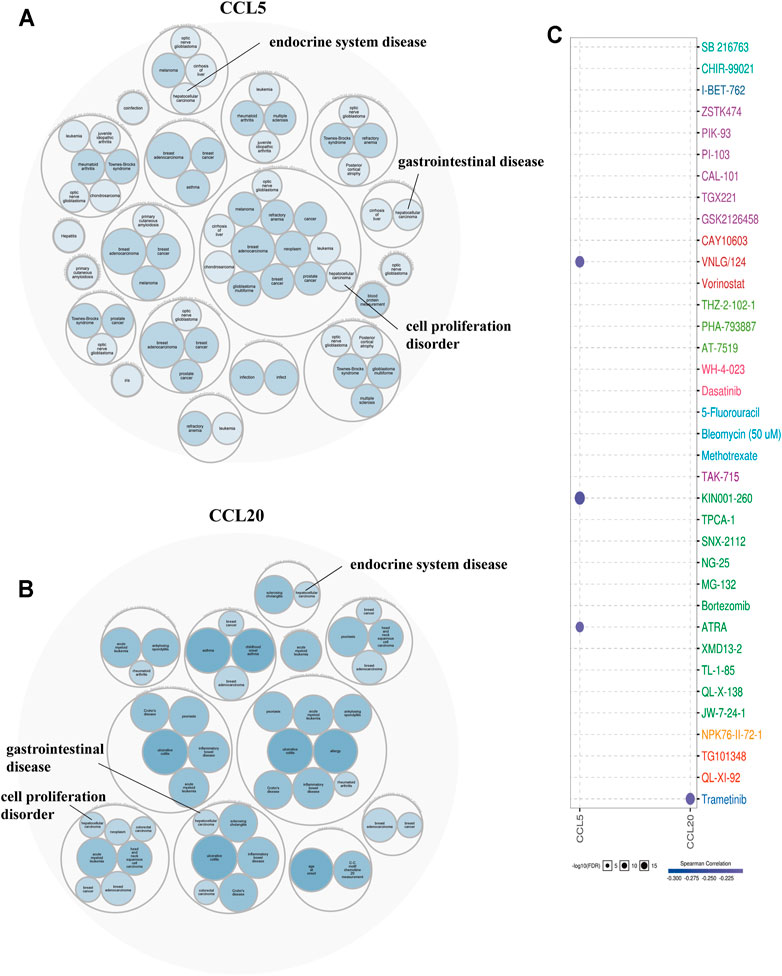
FIGURE 9. Disease correlation (Open Targets) and drug resistance analysis (GSCALite) of aberrantly expressed chemokines CCL. (A) Diseases related to CCL5, (B) Diseases related to CCL20. (C) Drug resistance analysis of chemokines CCL5 and CCL20.
Discussion
LIHC is considered the prototype of “inflammation-associated cancer,” because it usually originates in chronically inflamed tissue (Vucur et al., 2010). It is worth noting that chemokines CCL play a crucial role in inflammation and immunity, and they are also key mediators of cancer related inflammation being present at tumor site for pre-existing chronic inflammatory conditions but also being target of carcinogenic pathways (Mantovani et al., 2010). Previous studies have characterized the chemokines CCL play a vital regulatory role in tumor cell growth, tumor formation, invasion and metastasis (Chow and Luster, 2014; Yang et al., 2017). In addition, the role of chemokines CCL between the tumor microenvironment and cancer immunotherapy has gradually attracted the attention of researchers. However, the prognostic value and biological functions of chemokines CCL in LIHC urgently need to be further demonstrated.
Since the pathological stage of LIHC is critical to the prognosis of patients, we first explored the relationship between aberrantly expressed chemokines CCL and pathological stage in LIHC. Among the 18 aberrantly expressed genes in LIHC tissue compared with normal liver tissue (upregulation of CCL5/8/11/13/15/18/20/21/25/26/27/28; downregulation of CCL2/3/4/14/23/24), the transcriptional expression of CCL25, CCL26, and CCL28 were obviously elevated with the aggravation of tumor malignancy while CCL14 was reduced. Moreover, we have demonstrated that the high transcription levels of CCL14 and CCL21 in LIHC patients were significantly associated with better DFS, and high CCL14 and CCL23 transcription levels also brought longer OS. Therefore, all the findings proved that the differential expression of chemokines CCL was significantly associated with the progression and prognosis of LIHC.
Promiscuous interactions between chemokines and their receptors play a pivotal role in many pathological processes, including directly affecting tumor progression and therapeutic outcomes. To further clarify the interaction between these differentially expressed chemokines CCL in LIHC, PPI network and neighboring gene prediction were used for analysis. We found that the most significant functional interactions of these chemokines CCL included chemotaxis, cytokine activity, lymphocyte migration, and inflammatory response. As reported in previous studies, these functions could regulate immune cell infiltration and inflammation in the tumor environment (TME), which played an important role in tumorigenesis (Balkwill, 2004; Coussens and Werb, 2002; Koizumi et al., 2007).
In the tumor microenvironment, tumor cells can induce the recruitment and infiltration of various immune cells by expressing chemokines. We then performed a comprehensive exploration regarding the association between differential expression of chemokines CCL and immune cell infiltration. Accumulating evidence suggests that aberrant expressions of chemokines CCL regulate the recruitment of immune cells in tumors, such as CD8+ tumor infiltrating T cells and macrophages, which may ultimately affect the clinical outcome of cancer patients (Dangaj et al., 2019; Lee et al., 2017). As shown in our results, there was a positive or negative correlation between the expression of chemokines CCL and the infiltration of the six immune cell types, B cells, macrophages, neutrophils, dendritic cells, CD4+ and CD8+ T cells. What’s more, the multivariable Cox proportional hazard model indicated that the expressions of CCL2, CCL5, and CCL20 may be potential risk factors affecting the clinical outcome of patients with LIHC. Furthermore, chemokines have been proven to be important regulators of immune cell infiltration and immune checkpoint blocking efficacy in the TME (House et al., 2020; Karin, 2018). Similarly, our research suggested that CCL2 and CCL5 were positively correlated with immune checkpoints such as PD-1, PD-L1, and CTLA-4, while CCL20 was also positively correlated with PD-1 and CTLA-4.
In the analysis of potential transcription factor targets of the differentially expressed chemokines CCL, a total of 9 key transcription factors (RELA, REL, NFKB1, STAT1/3/6, IRF3, SPI1, and JUN) were involved in the transcriptional regulation of chemokines CCL (including CCL2, CCL3, CCL4, CCL5, CCL11, CCL13, CCL20, CCL21). RELA post-translational modification, particularly phosphorylation, has been proven to be critical for abnormal NF-κB activation (Lu and Yarbrough, 2015). Moreover, NFKB1 has been reported to play a crucial role in attenuating the activation of the NF-κB signaling pathway, potentially revealing a new therapeutic target in inflammatory diseases and cancer (Cartwright et al., 2016). Studies have also shown that phosphorylation of cellular JUN regulates the secretion of chemokines by macrophages in LIHC-bearing liver, and affects the recruitment of regulatory T cells and tumor progression (Hefetz-Sela et al., 2014). Therefore, these data indicate that the chemokine CCL mediates the progression of LIHC disease through a variety of signaling pathways, which also provides potential targets for clinical treatment strategies.
To more clarify the mechanism of the differentially expressed chemokines CCL in LIHC, we performed GO functional enrichment and KEGG signal pathway enrichment analyses. Among them, functional enrichment analysis showed that the aberrant expressed chemokines CCL and their similar neighboring genes were mainly related to chemokine-mediated signaling pathway, chemotaxis, NF-κB signaling pathway, TLR signaling pathway, and TNF signaling pathway. The findings were consistent with the previous studies demonstrating that chemokine-mediated signaling pathways play vital regulatory roles in oncogenic processes including tumor proliferation, apoptosis, epithelial–mesenchymal transition, immune evasion, and metastasis (Bian et al., 2019; Bikfalvi and Billottet, 2020; Zhuang et al., 2018). Furthermore, both the NF-κB signaling pathway (Dolcet et al., 2005) and TLR signaling pathway (Patra et al., 2020) have been confirmed to be involved in a variety of pathological processes in tumor progression.
In this study, we further verified that CCL5 and CCL20 were significantly upregulated in both HCC cell lines and tumor tissues compared with normal controls by Western blot and IHC, which were consistent with the transcriptional levels. We then paid attention to the TNF signaling pathway regulated by CCL5 and CCL20. TNF, the trigger of tumor cell apoptosis, was a major mediator of inflammation and immunity in tumor microenvironment, and there were strong evidences that this cancer-related inflammation contributes to the proliferation of malignant cells, stimulates angiogenesis and metastasis, regulates immune response, and affects clinical treatment strategies (Chen and Goeddel, 2002; Mantovani et al., 2008; Balkwill, 2009). Interestingly, TNF could also induce the activation of transcription factors c-jun and NF-κB, which ultimately activated JNK kinase activity and NF-κB signaling pathways, respectively (G. Chen et al., 2002). Besides, the disease susceptibility prediction found that aberrant expressed CCL5 and CCL20 were mainly related to gastrointestinal disease, endocrine system disease, and the disease of cell proliferation disorder (including LIHC). CCL5 expression was additionally negatively regulated by some small-molecule drugs, including VNLG/124, KIN001-260, ATRA, while CCL20 was negatively regulated by Trametinib. It is worth noting that immunotherapy has revolutionized the treatment of cancer in recent years. It has been reported that the upregulation of CCL5 may promote immune evasion through recruiting Treg cells into tumor lesion and anti-PD-L1 treatment could enhance CCL5-mediated anti-tumor effects in pancreatic ductal adenocarcinoma (Wang et al., 2020). We, therefore, demonstrated that CCL5 was positively correlated with PD-1, PD-L1, and CTLA-4, whereas CCL20 was positively correlated with PD-1 and CTLA-4. The collective evidence supports the potential of chemokines CCL as prognostic markers and clinical treatment targets for patients with LIHC.
Although bioinformatic approaches have been widely used in the research of human diseases, there are some limitations in our study. First, the results of gene expression presented by database analysis (such as Oncomine) are mainly mRNA levels. However, gene expression is regulated at multiple levels, including epigenetics, transcription, translation and post-translation. Especially in tumor-related research, we should also pay attention to the impact of gene protein expression levels on disease progression. Secondly, bioinformatics analysis is relatively mature today, which provides a broader perspective for the potential mechanisms and therapeutic targets of disease progression, whereas the experimental verifications on this basis are necessary, which is also the most critical limitation of our research. Finally, small sample size verification in this study is far from sufficient, and we look forward to large-sample clinical cohort studies and biological validation in vitro or in vivo that can be performed in the future to further verify the mechanism of LIHC progression.
In summary, our research findings hope to provide potential prognostic biomarkers for LIHC patients, and provide new insights for their therapeutic targets and clinical medications, which may help guide treatment strategies.
Data Availability Statement
The datasets presented in this study can be found in online repositories. The names of the repository/repositories and accession number(s) can be found in the article/supplementary material.
Ethics Statement
The studies involving human participants were reviewed and approved by the Ethics Committee of Shanghai General Hospital. The patients/participants provided their written informed consent to participate in this study.
Author Contributions
ZJ, CX, and PW wrote this manuscript and prepared the figure. LZ and XL devised and supervised this project. ZJ and CX assisted in the literature search and manuscript editing. All authors contributed to the article and approved the submitted version.
Funding
This study was partly supported by research grants from the National Natural Science Foundation of China (82070672).
Conflict of Interest
The authors declare that the research was conducted in the absence of any commercial or financial relationships that could be construed as a potential conflict of interest.
Publisher’s Note
All claims expressed in this article are solely those of the authors and do not necessarily represent those of their affiliated organizations, or those of the publisher, the editors and the reviewers. Any product that may be evaluated in this article, or claim that may be made by its manufacturer, is not guaranteed or endorsed by the publisher.
Acknowledgments
We thank the National Natural Science Foundation of China for the grant funding. We also acknowledge the contributions of the databases that we used in this study.
Abbreviations
BP, biological process; CC, cellular component; CCL, chemokine (C-C Motif) ligand; CTRP, Therapeutics Response Portal; CTLA-4, cytotoxic T-lymphocyte-associated protein 4; DFS, disease-free survival; GEPIA, Gene Expression Profiling Interactive Analysis; GEO, Gene Expression Omnibus; GDSC, Drug Sensitivity in Cancer; GO, Gene Ontology; HCC, hepatocellular carcinoma; KEGG, Kyoto Encyclopedia of Genes and Genomes; LIHC, liver hepatocellular carcinoma; MF, molecular function; NTA, Network Topology-based Analysis; NF-κB, nuclear factor kappa B; OS, overall survival; PD-L1 (CD274), programmed cell death-ligand 1; PPI, protein–protein interaction; TCGA, the cancer genome atlas; TF, transcription factor; PD-1(PDCD1), programmed cell death protein 1; TLR, toll-like receptor; TNF, tumor necrosis factor.
References
Atretkhany, K.-S. N., Drutskaya, M. S., Nedospasov, S. A., Grivennikov, S. I., and Kuprash, D. V. (2016). Chemokines, Cytokines and Exosomes Help Tumors to Shape Inflammatory Microenvironment. Pharmacol. Ther. 168, 98–112. doi:10.1016/j.pharmthera.2016.09.011
Balkwill, F. (2004). Cancer and the Chemokine Network. Nat. Rev. Cancer 4 (7), 540–550. doi:10.1038/nrc1388
Balkwill, F. (2009). Tumour Necrosis Factor and Cancer. Nat. Rev. Cancer 9 (5), 361–371. doi:10.1038/nrc2628
Bian, X., Xiao, Y.-T., Wu, T., Yao, M., Du, L., and Ren, S. (2019). Microvesicles and Chemokines in Tumor Microenvironment: Mediators of Intercellular Communications in Tumor Progression. Mol. Cancer 18(1), 50. doi:10.1186/s12943-019-0973-7
Bikfalvi, A., and Billottet, C. (2020). The CC and CXC Chemokines: Major Regulators of Tumor Progression and the Tumor Microenvironment. Am. J. Physiol. Cel Physiol 318 (3), C542–C554. doi:10.1152/ajpcell.00378.2019
Cartwright, T., Perkins, N. D., and Caroline, L, W. (2016). NFKB1: A Suppressor of Inflammation, Ageing and Cancer. FEBS J. 283 (10), 1812–1822. doi:10.1111/febs.13627
Chandrashekar, D. S., Bashel, B., Balasubramanya, S., Creighton, C. J., Ponce-Rodriguez, I., Chakravarthi, B., et al. (2017). UALCAN: A portal for Facilitating Tumor Subgroup Gene Expression and Survival Analyses. Neoplasia 19 (8), 649–658. doi:10.1016/j.neo.2017.05.002
Chen, G., and Goeddel, D. V. (2002). TNF-R1 Signaling: A Beautiful Pathway. Science 296 (5573), 1634–1635. doi:10.1126/science.1071924
Chen, X., Cheung, S. T., So, S., Fan, S. T., Barry, C., Higgins, J., et al. (2002). Gene Expression Patterns in Human Liver Cancers. Mol. Biol. Cel. 13 (6), 1929–1939. doi:10.1091/mbc.02-02-0023
Chow, M. T., and Luster, A. D. (2014). Chemokines in Cancer. Cancer Immunol. Res. 2 (12), 1125–1131. doi:10.1158/2326-6066.CIR-14-0160
Coussens, L. M., and Werb, Z. (2002). Inflammation and Cancer. Nature 420 (6917), 860–867. doi:10.1038/nature01322
Dangaj, D., Bruand, M., Grimm, A. J., Ronet, C., Barras, D., Duttagupta, P. A., et al. (2019). Cooperation between Constitutive and Inducible Chemokines Enables T Cell Engraftment and Immune Attack in Solid Tumors. Cancer Cell 35 (6), 885–900. doi:10.1016/j.ccell.2019.05.004
Demetris, A. J., Bellamy, C. O., Gandhi, C. R., Prost, S., Nakanuma, Y., and Stolz, D. B. (2016). Functional Immune Anatomy of the Liver-As an Allograft. Am. J. Transpl. 16 (6), 1653–1680. doi:10.1111/ajt.13749
Dolcet, X., Llobet, D., Pallares, J., and Matias-Guiu, X. (2005). NF-kB in Development and Progression of Human Cancer. Virchows Arch. 446 (5), 475–482. doi:10.1007/s00428-005-1264-9
Forner, A., Reig, M., and Bruix, J. (2018). Hepatocellular Carcinoma. Lancet 391 (10127), 1301–1314. doi:10.1016/S0140-6736(18)30010-2
Greten, T. F., Lai, C. W., Li, G., and Staveley-O'Carroll, K. F. (2019). Targeted and Immune-Based Therapies for Hepatocellular Carcinoma. Gastroenterology 156 (2), 510–524. doi:10.1053/j.gastro.2018.09.051
Han, H., Cho, J. W., Lee, S., Yun, A., Kim, H., Bae, D., et al. (2018). TRRUST V2: An Expanded Reference Database of Human and Mouse Transcriptional Regulatory Interactions. Nucleic Acids Res. 46 (D1), D380–D386. doi:10.1093/nar/gkx1013
Hefetz-Sela, S., Stein, I., Klieger, Y., Porat, R., Sade-Feldman, M., Zreik, F., et al. (2014). Acquisition of an Immunosuppressive Protumorigenic Macrophage Phenotype Depending on C-Jun Phosphorylation. Proc. Natl. Acad. Sci. U S A. 111 (49), 17582–17587. doi:10.1073/pnas.1409700111
House, I. G., Savas, P., Lai, J., Chen, A., Oliver, A. J., Teo, Z. L., et al. (2020). Macrophage-Derived CXCL9 and CXCL10 Are Required for Antitumor Immune Responses Following Immune Checkpoint Blockade. Clin. Cancer Res. 26 (2), 487–504. doi:10.1158/1078-0432.CCR-19-1868
Huang, D. W., Sherman, B. T., and Lempicki, R. A. (2009). Systematic and Integrative Analysis of Large Gene Lists Using DAVID Bioinformatics Resources. Nat. Protoc. 4 (1), 44–57. doi:10.1038/nprot.2008.211
Karin, N. (2018). Chemokines and Cancer: New Immune Checkpoints for Cancer Therapy. Curr. Opin. Immunol. 51, 140–145. doi:10.1016/j.coi.2018.03.004
Koizumi, K., Hojo, S., Akashi, T., Yasumoto, K., and Saiki, I. (2007). Chemokine Receptors in Cancer Metastasis and Cancer Cell-Derived Chemokines in Host Immune Response. Cancer Sci. 98 (11), 1652–1658. doi:10.1111/j.1349-7006.2007.00606.x
Koscielny, G., An, P., Carvalho-Silva, D., Cham, J. A., Fumis, L., Gasparyan, R., et al. (2017). Open Targets: A Platform for Therapeutic Target Identification and Validation. Nucleic Acids Res. 45 (D1), D985–D994. doi:10.1093/nar/gkw1055
Lee, J. M., Lee, M. H., Garon, E., Goldman, J. W., Salehi-Rad, R., Baratelli, F. E., et al. (2017). Phase I Trial of Intratumoral Injection of CCL21 Gene-Modified Dendritic Cells in Lung Cancer Elicits Tumor-specific Immune Responses and CD8(+) T-Cell Infiltration. Clin. Cancer Res. 23 (16), 4556–4568. doi:10.1158/1078-0432.CCR-16-2821
Li, T., Fan, J., Wang, B., Traugh, N., Chen, Q., Liu, J. S., et al. (2017). TIMER: A Web Server for Comprehensive Analysis of Tumor-Infiltrating Immune Cells. Cancer Res. 77 (21), e108–e110. doi:10.1158/0008-5472.CAN-17-0307
Liao, Y., Wang, J., Jaehnig, E. J., Shi, Z., and Zhang, B. (2019). WebGestalt 2019: Gene Set Analysis Toolkit with Revamped UIs and APIs. Nucleic Acids Res. 47 (W1), W199–W205. doi:10.1093/nar/gkz401
Liu, C. J., Hu, F. F., Xia, M. X., Han, L., Zhang, Q., and Guo, A. Y. (2018). GSCALite: A Web Server for Gene Set Cancer Analysis. Bioinformatics 34 (21), 3771–3772. doi:10.1093/bioinformatics/bty411
Liu, J., Chen, S., Wang, W., Ning, B. F., Chen, F., Shen, W., et al. (2016). Cancer-associated Fibroblasts Promote Hepatocellular Carcinoma Metastasis through Chemokine-Activated Hedgehog and TGF-Beta Pathways. Cancer Lett. 379 (1), 49–59. doi:10.1016/j.canlet.2016.05.022
Liu, L. Z., Zhang, Z., Zheng, B. H., Shi, Y., Duan, M., Ma, L. J., et al. (2019). CCL15 Recruits Suppressive Monocytes to Facilitate Immune Escape and Disease Progression in Hepatocellular Carcinoma. Hepatology 69 (1), 143–159. doi:10.1002/hep.30134
Llovet, J. M., Di Bisceglie, A. M., Bruix, J., Kramer, B. S., Lencioni, R., Zhu, A. X., et al. (2008). Design and Endpoints of Clinical Trials in Hepatocellular Carcinoma. J. Natl. Cancer Inst. 100 (10), 698–711. doi:10.1093/jnci/djn134
Llovet, J. M., Montal, R., Sia, D., and Finn, R. S. (2018). Molecular Therapies and Precision Medicine for Hepatocellular Carcinoma. Nat. Rev. Clin. Oncol. 15 (10), 599–616. doi:10.1038/s41571-018-0073-4
Lu, X., and Yarbrough, W. G. (2015). Negative Regulation of RelA Phosphorylation: Emerging Players and Their Roles in Cancer. Cytokine Growth Factor. Rev. 26 (1), 7–13. doi:10.1016/j.cytogfr.2014.09.003
Mantovani, A., Allavena, P., Sica, A., and Balkwill, F. (2008). Cancer-related Inflammation. Nature 454 (7203), 436–444. doi:10.1038/nature07205
Mantovani, A., Savino, B., Locati, M., Zammataro, L., Allavena, P., and Bonecchi, R. (2010). The Chemokine System in Cancer Biology and Therapy. Cytokine Growth Factor. Rev. 21 (1), 27–39. doi:10.1016/j.cytogfr.2009.11.007
Mas, V. R., Maluf, D. G., Archer, K. J., Yanek, K., Kong, X., Kulik, L., et al. (2009). Genes Involved in Viral Carcinogenesis and Tumor Initiation in Hepatitis C Virus-Induced Hepatocellular Carcinoma. Mol. Med. 15 (3-4), 85–94. doi:10.2119/molmed.2008.00110
Mollica, P. V., Massara, M., Capucetti, A., and Bonecchi, R. (2019). Chemokines and Chemokine Receptors: New Targets for Cancer Immunotherapy. Front. Immunol. 10, 379. doi:10.3389/fimmu.2019.00379
Nagarsheth, N., Wicha, M. S., and Zou, W. (2017). Chemokines in the Cancer Microenvironment and Their Relevance in Cancer Immunotherapy. Nat. Rev. Immunol. 17 (9), 559–572. doi:10.1038/nri.2017.49
Patra, M. C., Shah, M., and Choi, S. (2020). Toll-like Receptor-Induced Cytokines as Immunotherapeutic Targets in Cancers and Autoimmune Diseases. Semin. Cancer Biol. 64, 61–82. doi:10.1016/j.semcancer.2019.05.002
Rhodes, D. R., Yu, J., Shanker, K., Deshpande, N., Varambally, R., Ghosh, D., et al. (2004). ONCOMINE: A Cancer Microarray Database and Integrated Data-Mining Platform. Neoplasia 6 (1), 1–6. doi:10.1016/s1476-5586(04)80047-2
Roessler, S., Jia, H. L., Budhu, A., Forgues, M., Ye, Q. H., Lee, J. S., et al. (2010). A Unique Metastasis Gene Signature Enables Prediction of Tumor Relapse in Early-Stage Hepatocellular Carcinoma Patients. Cancer Res. 70 (24), 10202–10212. doi:10.1158/0008-5472.CAN-10-2607
Schneider, C., Teufel, A., Yevsa, T., Staib, F., Hohmeyer, A., Walenda, G., et al. (2012). Adaptive Immunity Suppresses Formation and Progression of Diethylnitrosamine-Induced Liver Cancer. Gut 61 (12), 1733–1743. doi:10.1136/gutjnl-2011-301116
Sung, H., Ferlay, J., Siegel, R. L., Laversanne, M., Soerjomataram, I., Jemal, A., et al. (2021). Global Cancer Statistics 2020: GLOBOCAN Estimates of Incidence and Mortality Worldwide for 36 Cancers in 185 Countries. CA Cancer J. Clin. 71 (3), 209–249. doi:10.3322/caac.21660
Szklarczyk, D., Gable, A. L., Lyon, D., Junge, A., Wyder, S., Huerta-Cepas, J., et al. (2019). STRING V11: Protein-Protein Association Networks with Increased Coverage, Supporting Functional Discovery in Genome-wide Experimental Datasets. Nucleic Acids Res. 47 (D1), D607–D613. doi:10.1093/nar/gky1131
Tang, Z., Li, C., Kang, B., Gao, G., Li, C., and Zhang, Z. (2017). GEPIA: A Web Server for Cancer and normal Gene Expression Profiling and Interactive Analyses. Nucleic Acids Res. 45 (W1), W98–W102. doi:10.1093/nar/gkx247
Tiegs, G., and Lohse, A. W. (2010). Immune Tolerance: What Is Unique about the Liver. J. Autoimmun. 34 (1), 1–6. doi:10.1016/j.jaut.2009.08.008
Vucur, M., Roderburg, C., Bettermann, K., Tacke, F., Heikenwalder, M., Trautwein, C., et al. (2010). Mouse Models of Hepatocarcinogenesis: What Can We Learn for the Prevention of Human Hepatocellular Carcinoma? Oncotarget 1 (5), 373–378. doi:10.18632/oncotarget.10090610.18632/oncotarget.170
Wang, X., Li, X., Wei, X., Jiang, H., Lan, C., Yang, S., et al. (2020). PD-L1 Is a Direct Target of Cancer-FOXP3 in Pancreatic Ductal Adenocarcinoma (PDAC), and Combined Immunotherapy with Antibodies against PD-L1 and CCL5 Is Effective in the Treatment of PDAC. Signal. Transduct Target. Ther. 5 (1), 38. doi:10.1038/s41392-020-0144-8
Warde-Farley, D., Donaldson, S. L., Comes, O., Zuberi, K., Badrawi, R., Chao, P., et al. (2010). The GeneMANIA Prediction Server: Biological Network Integration for Gene Prioritization and Predicting Gene Function. Nucleic Acids Res. 38 (Web Server issue), W214–W220. doi:10.1093/nar/gkq537
Wurmbach, E., Chen, Y. B., Khitrov, G., Zhang, W., Roayaie, S., Schwartz, M., et al. (2007). Genome-wide Molecular Profiles of HCV-Induced Dysplasia and Hepatocellular Carcinoma. Hepatology 45 (4), 938–947. doi:10.1002/hep.21622
Yang, P., Li, Q. J., Feng, Y., Zhang, Y., Markowitz, G. J., Ning, S., et al. (2012). TGF-beta-miR-34a-CCL22 Signaling-Induced Treg Cell Recruitment Promotes Venous Metastases of HBV-Positive Hepatocellular Carcinoma. Cancer Cell 22 (3), 291–303. doi:10.1016/j.ccr.2012.07.023
Yang, X. L., Liu, K. Y., Lin, F. J., Shi, H. M., and Ou, Z. L. (2017). CCL28 Promotes Breast Cancer Growth and Metastasis through MAPK-Mediated Cellular Anti-apoptosis and Pro-metastasis. Oncol. Rep. 38 (3), 1393–1401. doi:10.3892/or.2017.5798
Zhao, N., Dang, H., Ma, L., Martin, S. P., Forgues, M., Ylaya, K., et al. (2021). Intratumoral Gammadelta T-Cell Infiltrates, Chemokine (C-C Motif) Ligand 4/Chemokine (C-C Motif) Ligand 5 Protein Expression and Survival in Patients with Hepatocellular Carcinoma. Hepatology 73 (3), 1045–1060. doi:10.1002/hep.31412
Zhu, M., Xu, W., Wei, C., Huang, J., Xu, J., Zhang, Y., et al. (2019). CCL14 Serves as a Novel Prognostic Factor and Tumor Suppressor of HCC by Modulating Cell Cycle and Promoting Apoptosis. Cell Death Dis 10 (11), 796. doi:10.1038/s41419-019-1966-6
Zhuang, H., Cao, G., Kou, C., and Liu, T. (2018). CCL2/CCR2 axis Induces Hepatocellular Carcinoma Invasion and Epithelial-Mesenchymal Transition In Vitro through Activation of the Hedgehog Pathway. Oncol. Rep. 39 (1), 21–30. doi:10.3892/or.2017.6069
Zimmermann, H. W., and Tacke, F. (2011). Modification of Chemokine Pathways and Immune Cell Infiltration as a Novel Therapeutic Approach in Liver Inflammation and Fibrosis. Inflamm. Allergy Drug Targets 10 (6), 509–536. doi:10.2174/187152811798104890
Keywords: liver hepatocellular carcinoma, biomarker, chemokine, tumor microenvironment, bioinformatics analysis
Citation: Jiang Z, Xing C, Wang P, Liu X and Zhong L (2021) Identification of Therapeutic Targets and Prognostic Biomarkers Among Chemokine (C-C Motif) Ligands in the Liver Hepatocellular Carcinoma Microenvironment. Front. Cell Dev. Biol. 9:748269. doi: 10.3389/fcell.2021.748269
Received: 27 July 2021; Accepted: 17 November 2021;
Published: 06 December 2021.
Edited by:
Zhenqiang Sun, First Affiliated Hospital of Zhengzhou University, ChinaReviewed by:
Xiang Li, Henan Agricultural University, ChinaYuyan Zhu, The First Affiliated Hospital of China Medical University, China
Copyright © 2021 Jiang, Xing, Wang, Liu and Zhong. This is an open-access article distributed under the terms of the Creative Commons Attribution License (CC BY). The use, distribution or reproduction in other forums is permitted, provided the original author(s) and the copyright owner(s) are credited and that the original publication in this journal is cited, in accordance with accepted academic practice. No use, distribution or reproduction is permitted which does not comply with these terms.
*Correspondence: Xueni Liu, bHhseXNoQGhvdG1haWwuY29t; Lin Zhong, emhvbmdsXzMyODRAaG90bWFpbC5jb20=
†These authors have contributed equally to this work and share first authorship