- 1Department of Oncology, Beijing Friendship Hospital, Capital Medical University, Beijing, China
- 2Department of Intervention Therapy, Zezhou People’s Hospital, Jincheng, China
- 3Department of Biochemistry and Molecular Biology, School of Basic Medical Sciences, Shanxi Medical University, Taiyuan, China
- 4Chongqing Key Laboratory of Molecular Oncology and Epigenetics, The First Affiliated Hospital of Chongqing Medical University, Chongqing, China
The combination of immune-checkpoint blockade (ICB) and lenvatinib has demonstrated robust clinical effects that are superior to those of monotherapies, but the synergistic anti-tumor mechanisms remain unclear. Exploring the synergistic molecular mechanisms and early identifying potential application have key importance for clinical therapeutics. We firstly systematically reviewed published data of ICB in combination with lenvatinib for the treatment of cancer by meta-analysis. A subsequent bioinformatics analysis explored the mechanism of combined ICB and lenvatinib therapy in 33 cancer types. Transcriptomic analysis was conducted by RNA-seq, and genomic analysis was performed on gene mutations and copy-number alteration data. Tumor-related pathways and tumor immune micro-environment (TIME) were also investigated. The meta-analysis showed a 38.0% objective response rate (ORR) and 79% disease control rate (DCR) for ICB combined with lenvatinib. Multi-omics analysis revealed that ICB and lenvatinib target genes were highly expressed and showed driving alterations in six specific malignancies. Pathway-enrichment analysis found target genes were implicated in tumor development, angiogenesis, and immunoregulatory associated pathways. This study verified the potential synergistic mechanisms of ICB combined with lenvatinib at transcriptomics, genomics, protein, and cellular levels and recognized nine tumor types had ≥ 2 positive treatment-related molecular characteristics, which might benefit particularly from this combined strategy. The findings would help to provide clinical insights and theoretical basis for optimizing of targeted therapy-immunotherapy combinations, and for guiding individualized precision-medicine approaches for cancer treatment.
Introduction
Immune-checkpoint blockade (ICB) combined with molecular targeted therapy is an emerging trend in anticancer treatment. The blockade of inhibitory immune checkpoints, including programmed cell death-1 (PD-1) and its ligand programmed cell death ligand-1 (PD-L1), lymphocyte activation gene-3 (LAG-3), and cytotoxic T-lymphocyte–associated antigen 4 (CTLA-4), can reactivate the body’s immune system, thereby preventing the escape of tumor cells (Wei et al., 2018; He and Xu, 2020). In contrast, molecular targeted therapy reverses the malignant biological behaviors of tumors by blocking the tumorigenic components of cells at the molecular level (Druker, 2003). At present, it has been clinically found that the combined application of ICB and anti-angiogenic drugs or targeted therapy have synergistic effects in malignancy, but the molecular mechanism of this combination remains unclear.
The representative ICB therapy, pembrolizumab, is a PD-1 inhibitor, which has been approved for the treatment of colorectal cancer (Powles et al., 2020), non-small cell lung cancer (NSCLC) (Mok et al., 2019) (Abbreviations and full names of tumors and targets was shown in Supplementary Table 1) and other cancer types. Lenvatinib is a novel molecular targeted therapeutic drug that can inhibit a variety of tyrosine kinases, including vascular endothelial growth factor receptor (VEGFRs) 1–3, fibroblast growth factor receptor (FGFRs) 1–4, platelet derived growth factor receptor (PDGFR), stem cell factor receptor (c-kit) and rearranged during transfection (RET) proto-oncogene (Suyama and Iwase, 2018; Al-Salama et al., 2019). It exhibits potent antitumor, antiangiogenic, and immunomodulatory effects. Lenvatinib has been approved for unresectable liver hepatocellular cancer (LIHC) (Kudo et al., 2018), thyroid cancer (Schlumberger et al., 2015), and advanced renal cell carcinoma (RCC) (Motzer et al., 2015).
Nonetheless, the low remission rates with ICB monotherapies and the development of drug resistance with molecular targeted therapies have urged researchers to explore the potential of combination therapies. Data from previous studies showed that the median overall survival (mOS) of patients with advanced LIHC was 12.9 months with pembrolizumab monotherapy (Zhu et al., 2018) and 13.6 months with lenvatinib monotherapy (Kudo et al., 2018), whereas the mOS of patients treated with pembrolizumab and lenvatinib combination therapy reached 22.0 months (Finn et al., 2020). However, the therapeutic effect of ICB combined with lenvatinib is still poorly understood in some cancer types, and few investigations have addressed the mechanisms underlying the synergy of this combination.
Therefore, in this study, we first conducted a meta-analysis to analyze the efficacy of ICB and lenvatinib combination therapy. Bioinformatics analyses were subsequently performed to comprehensively analyze the synergistic mechanisms of ICB combined with lenvatinib and potential efficacies at the transcriptome (RNA-seq), genome (gene mutations, copy-number alterations [CNA]), protein, and immune cell levels.
Materials and Methods
Search Strategy and Selection Criteria for the Meta-Analysis
The PubMed, Embase, and Cochrane library databases were systematically queried by two authors of this study (Yuting Lu and Min Hu) for articles pertaining to ICB and lenvatinib. Abstracts from meetings of the European Society for Medical Oncology (ESMO) and the American Society of Clinical Oncology (ASCO) on the same topics were also searched. The latest data were used when the same trial was reported in multiple articles. The data-entry cutoff date was March 1, 2021. The search terms were: “lenvatinib” AND “immunotherapy OR immune therapy OR immune checkpoint inhibitor OR immune checkpoint blockade OR immune vaccine OR nivolumab OR ipilimumab OR pembrolizumab OR atezolizumab OR tremelimumab OR avelumab OR durvalumab” AND “combination OR combined with OR plus” AND “tumor OR cancer OR carcinoma OR neoplasm OR malignancy OR sarcoma.”
The inclusion criteria were as follows: (1) study design: prospective trial; (2) population: patients with pathologically confirmed advanced cancer; (3) intervention: lenvatinib + ICB; (4) study endpoints: objective-response rate (ORR), disease-control rate (DCR). The following information was independently extracted from the articles or abstracts identified by both authors (Yuting Lu and Min Hu): the authors’ names, the journal, the publication year, the tumor type, the treatment regimen, the line of treatment, the sample size per group, and the study endpoints. All information was crosschecked. All disagreements were resolved by discussion with a third reviewer (Qi Du).
Bioinformatics Data Mining
Expression and Correlation Analysis of ICB and Lenvatinib Targets
The target gene expression profile date of tumor samples and normal tissues was obtained from the cancer genome atlas (TCGA) in the UALCAN database1. The String database2 was employed to construct protein--protein-interaction (PPI) networks. The threshold was set to 0.4 to examine the relationships between the target proteins of ICB and lenvatinib. The UCSC Xena database3 was used to determine the Pearson correlation coefficient (r) values between the ICB and lenvatinib target genes in pan-cancer analysis. The GEPIA database4 was used to obtain the Pearson correlation coefficient of specific cancer types. Visualization was performed by the corrplot package of R (version 4.0.2).
Analysis of Genetic Alterations in ICB and Lenvatinib Target Genes
A total of 10967 samples from 32 studies from the TCGA in the cBioPortal database5 were used to analyze mutations and CNAs of ICB and lenvatinib target genes in different cancers. To identify likely functional variants, we used the driver-annotation resources OncoKB6 and Cancer Hotspots7. Passenger mutations, also known as variants of unknown significance, were filtered. The ggplot 2 and pheatmap packages of R (version 4.0.2) were used for visualization.
Gene Ontology (GO) and Kyoto Encyclopedia of Genes and Genomes (KEGG) Pathway Analyses of ICB and Lenvatinib Target Genes
To delineate the genetic and metabolic pathways of the ICB and lenvatinib target genes that affect tumor development, progression, and the immune microenvironment, the fifteen target genes were studied in terms of KEGG signaling pathways and GO pathway-enrichment analysis using the R packages clusterProfiler, org.Hs.eg.db, ggplot 2, enrichplot, and GOplot. P < 0.05 was set as the cut-off criterion. The enrichment analysis website Reactome8 was also used for validation.
Correlation Analysis Between ICB and Lenvatinib Target Genes and Tumor-Infiltrating Immune Cells
The TIMER database9 was used to obtain correlation coefficients between ICB and lenvatinib target genes, tumor infiltrates immune cells, and the associated pan-cancer survival data in TCGA. Datasets were selected from CIBERSORT (Myeloid-derived suppressor cells [MDSC] data were only found in the TIDE dataset). The pheatmap package of R (version 4.0.2) was used for visualization. The workflow is shown in Supplementary Figure 1.
Statistical Analysis
Statistical analysis was performed with Stata SE 16 (Stata Corporation, College Station, TX, United States) and R (version 4.0.2). We calculated the pooled ratio and 95% CI for ORR and DCR. I-squared (I2) tests were performed to evaluate heterogeneities between trials. In cases where an I2 of >50% was observed, which indicated the existence of heterogeneity, the randomized-effects model was used. Otherwise, the fixed effect model was adopted. Pearson correlation-coefficient analysis was performed to examine the relationship between ICB and lenvatinib target genes, and that between their targets and immune infiltrates. Kaplan–Meier analysis with a log-rank test was performed for immune infiltrates and genes to evaluate survival differences. The influences of gene-expression levels on survival were evaluated using the Cox proportional-hazards model. P < 0.05 was considered to reflect statistically significant differences.
Results
Meta-Analysis of Efficacy Data
We identified 412 original articles and 56 meeting abstracts during our electronic searches. After screening, four original articles and 12 conference abstracts met the criteria for inclusion in the meta-analysis (Supplementary Figure 2; Fernandez et al., 2020; Finn et al., 2020; Haugen et al., 2020; Kawazoe et al., 2020; Kudo et al., 2020; Lee et al., 2020; Li et al., 2020; Lin et al., 2020; Lwin et al., 2020; Makker et al., 2020; Taylor et al., 2020; Chung, 2021; Gomez-Roca et al., 2021; Motzer et al., 2021; Villanueva, 2021). The key characteristics of the 15 included studies are presented in Table 1. Across these studies, ICB combined with lenvatinib was associated with a benefit in ORR (pooled ratio, 0.38; 95% CI, 0.28–0.49; P < 0.001) and DCR (pooled ratio, 0.78; 95% CI, 0.72–0.84; P < 0.001) (Figure 1A). The results of the major endpoints with combined ICB and lenvatinib compared with ICB and lenvatinib monotherapy across different cancer types are summarized in Figure 1B (Boss et al., 2012; O’Day et al., 2013; Ribas et al., 2015; Bellmunt et al., 2017; Kudo et al., 2018; Adams et al., 2019; Burtness et al., 2019; Hida et al., 2019; Makker et al., 2019; Matulonis et al., 2019; Mok et al., 2019; Ott et al., 2019; Finn et al., 2020; Haugen et al., 2020; Iwasa et al., 2020; Kawazoe et al., 2020; Lin et al., 2020; Lwin et al., 2020; Powles et al., 2020; Gomez-Roca et al., 2021).
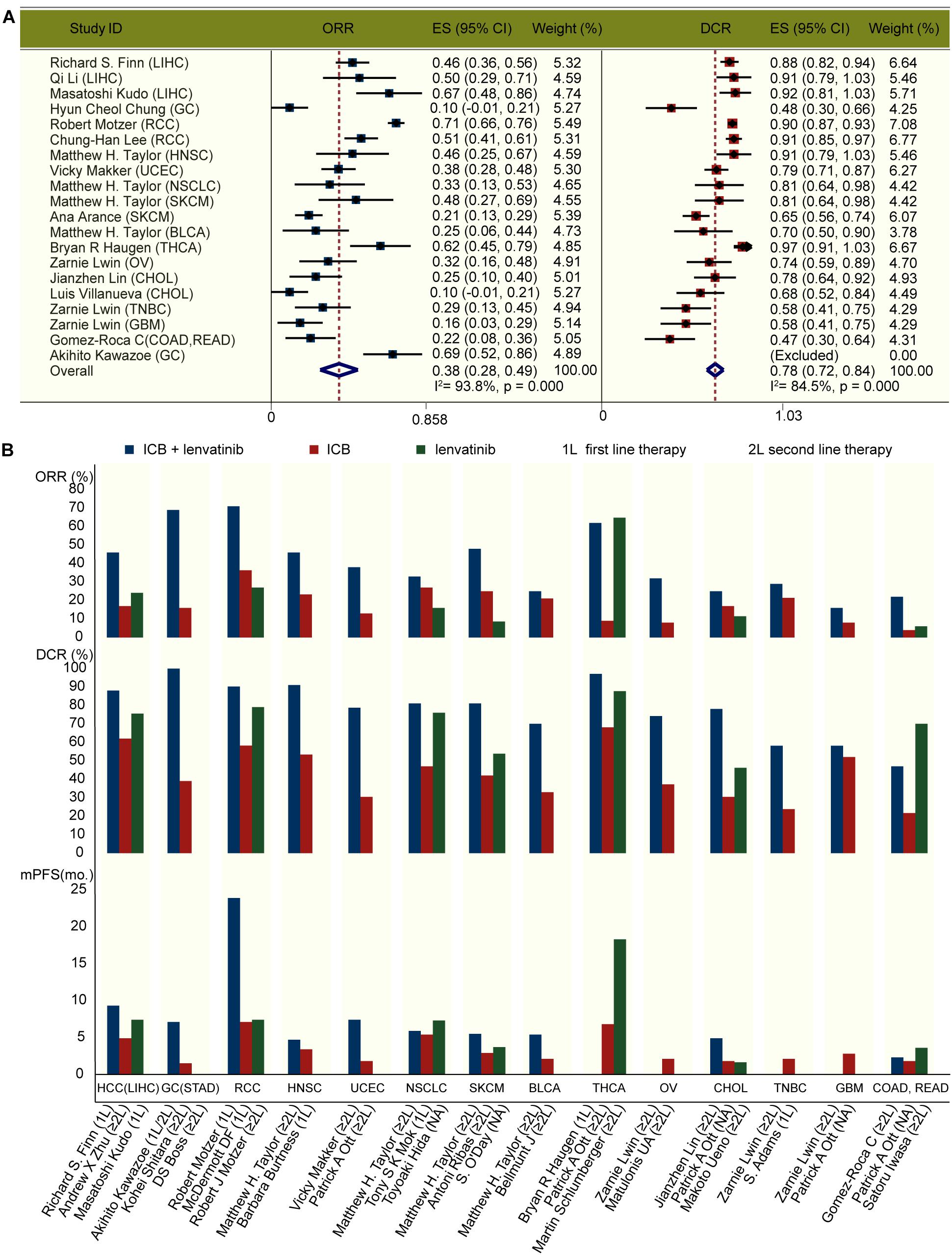
Figure 1. Clinical outcomes with ICB and lenvatinib. (A) Forest plot analysis of ORR and DCR with ICB combined with lenvatinib across different cancer types. (B) ORR, DCR, and mPFS with pembrolizumab and lenvatinib combined or as monotherapy across different cancer types. 1L, first line therapy; 2L, second line therapy; BLCA, bladder urothelial carcinoma; CHOL, cholangiocarcinoma; COAD, colon adenocarcinoma; ES, effect size; GBM, glioblastoma multiforme; GC, gastric cancer; HNSC, head and neck squamous cell carcinoma; LIHC, liver hepatocellular carcinoma; mo., month; NSCLC, non-small-cell lung cancer; OV, ovarian serous cystadenocarcinoma; RCC, renal cell carcinoma; READ, rectum adenocarcinoma; SKCM, skin cutaneous melanoma; THCA, thyroid carcinoma; TNBC, triple-negative breast carcinoma; UCEC, uterine corpus endometrial carcinoma.
Expression Analysis of ICB and Lenvatinib Targets
The bubble chart in Figure 2A shows that ICB and lenvatinib target genes are expressed in a broad range of cancers. In cholangiocarcinoma (CHOL), glioblastoma multiforme (GBM), head and neck squamous cell carcinoma (HNSC), kidney clear cell renal cell carcinoma (KIRC), LIHC, and stomach adenocarcinoma (STAD), both ICB and lenvatinib target genes were highly expressed (≥4 red bubbles for lenvatinib and ≥2 red bubbles for ICB, P < 0.05) (the red text in the abscissa). This suggests that the application of ICB combined with lenvatinib might be more effective in these malignancies. In contrast, in pancreatic adenocarcinoma (PAAD) and thymoma (THYM), the target genes of both therapies showed low expression (the blue text in the abscissa), which was suggested that this combination might be less effective in these tumors. The expression of ICB and lenvatinib target genes was inconsistent in breast invasive carcinoma (BRCA), colon adenocarcinoma (COAD), esophageal carcinoma (ESCA), lung adenocarcinoma (LUAD), lung squamous cell carcinoma (LUSC), pheochromocytoma and paraganglioma, thyroid carcinoma (THCA), and uterine corpus endometrial carcinoma (UCEC), with target genes for only one of the therapies showing higher expression (≥4 red bubbles for lenvatinib or ≥2 red bubbles for ICB, P < 0.05) (the green text in the abscissa). The combination of the two therapies may play a complementary or antagonistic role in these cancer types.
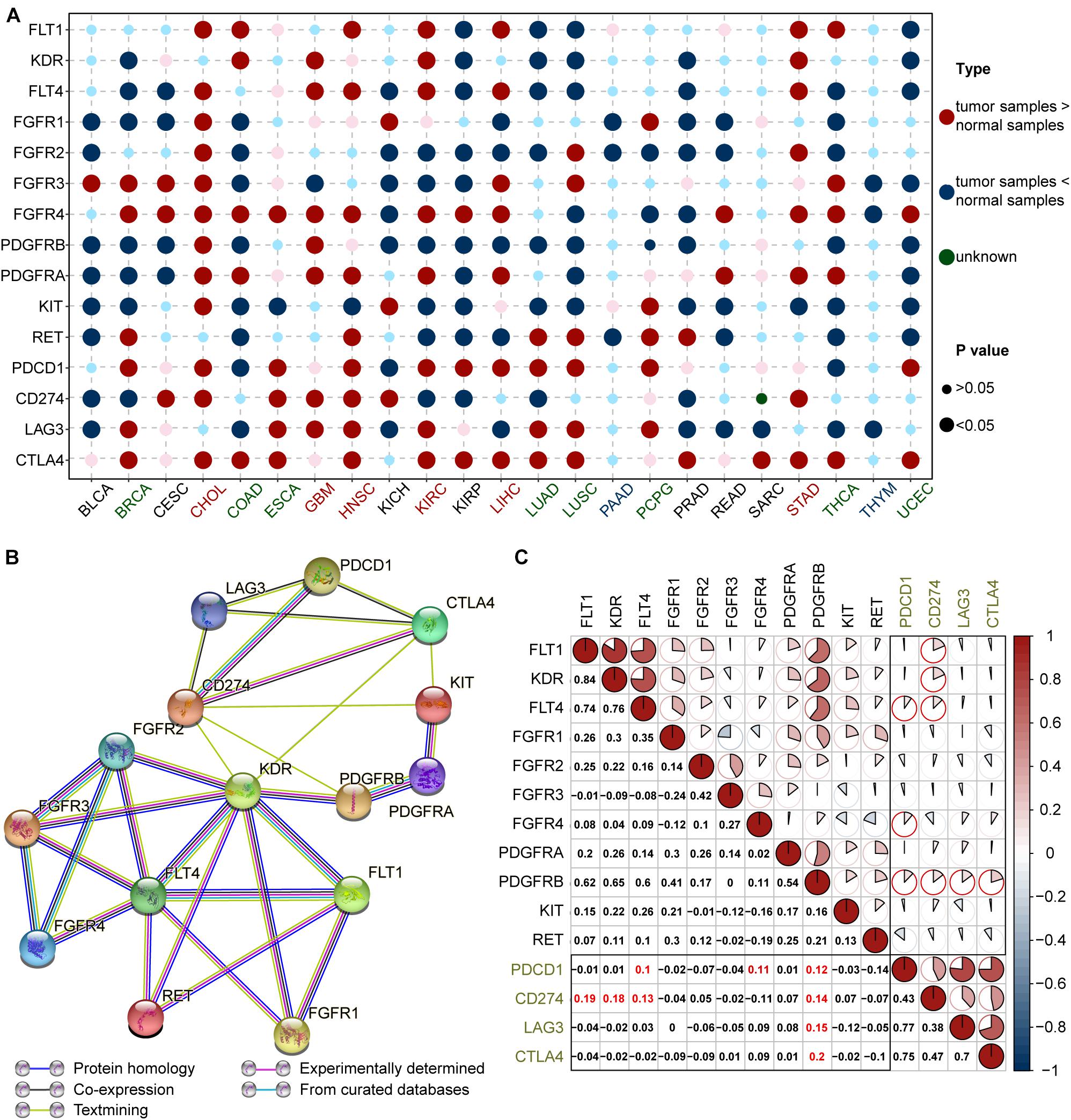
Figure 2. Expression and correlation of ICB and lenvatinib targets. (A) Comparison of ICB and lenvatinib target genes expression in tumor tissue and corresponding normal tissue. The cancer types in red text represent high expression of both lenvatinib (≥4 large red circles) and ICB (≥2 large red circles) target genes; green text represents moderate expression defined as higher expression of either lenvatinib (≥4 large red circles) or ICB (≥2 large red circles) target genes; blue text represents low expression of both lenvatinib and ICB target genes (no large red circles for either). (B) PPI network construction of lenvatinib and ICB target genes. (C) Correlation coefficient graph of ICB and lenvatinib target genes in pan-cancer. Numbers and the pie chart size correspond to the Pearson correlation coefficient (r value). The black boxes are the intersecting sections, and the red highlighted text and circles are r ≥ 0.1. BRCA, breast invasive carcinoma; CESC, cervical squamous cell carcinoma and endocervical adenocarcinoma; ESCA, esophageal carcinoma; KICH, kidney chromophobe; KIRC, kidney renal clear cell carcinoma; KIRP, kidney renal papillary cell carcinoma; LUAD, lung adenocarcinoma; LUSC, lung squamous cell carcinoma; PAAD, pancreatic adenocarcinoma; PCPG, pheochromocytoma and paraganglioma; PAAD, prostate adenocarcinoma; SARC, sarcoma; STAD, stomach adenocarcinoma; THYM, thymoma; ICB, immune-checkpoint blockade; FLT1 (VEGFR1), vascular endothelial growth factor receptor 1; KDR (VEGFR2), vascular endothelial growth factor receptor 2; FLT4 (VEGFR3), vascular endothelial growth factor receptor 3; FGFR, fibroblast growth factor receptor; PDGFR, platelet derived growth factor receptor; KIT, stem cell factor receptor; RET, the rearranged during transfection; PDCD1 (PD-1), programmed cell death 1; CD274 (PD-L1), programmed cell death ligand 1; LAG3, lymphocyte-activation protein 3; CTLA4, cytotoxic T-lymphocyte-associated antigen 4.
Correlation Analysis of ICB and Lenvatinib Targets
Protein–protein-interaction network analysis (Figure 2B) showed that the target proteins of ICB and lenvatinib displayed complex and extensive interactions, both directly and indirectly. Specifically, co-expression evidence was observed for the following proteins: VEGFR1, VEGFR2 and VEGFR3; FGFR2, FGFR3, and FGFR4; PD-1, PD-L1, LAG3, and CTLA4; and KIT and PDGFRA (Figure 2B, black lines). Analysis of scientific papers suggested that protein correlations exist between VEGFR2 and PD-L1 or CTLA4, between KIT and PD-L1 or CTLA4, and between PDGFRB and PD-L1 (Figure 2B, green lines). There were also protein correlations derived from experimental results (Figure 2B, purple lines) and curated databases (Figure 2B, blue lines).
Further correlation analysis showed that there was a common co-expression relationship between ICB and lenvatinib target genes in all cancers (Figure 2C). Specifically, strong positive correlations were observed between PDGFRB and CTLA4, LAG3, PDCD1 (PD-1), or CD274 (PD-L1); between FLT4 (VEGFR3) or FGFR4 and PD-1; and between FLT1 (VEGFR1) or KDR (VEGFR2) or VEGFR3 and PD-L1 (r ≥ 0.10) (highlighted in red). We calculated the number of positive correlation coefficients r ≥ 0.1 between lenvatinib and ICB target genes in specific cancer types. The upper half belonged to the high correlation group, e.g., COAD, READ, GBM, skin melanoma (SKCM) (green title in Supplementary Figure 3). Especially in LIHC, there was a strong positive correlation between PD-1 and FGFR1-4, PDGFRA, PDGFRB, or RET; between PD-L1 and FGFR1, PDGFRA, or PDGFRB; and between LAG3 and FGFR1 (Supplementary Figure 3 and Supplementary Data 1). The combination therapy may therefore have great potential in these tumor types. But, the types of cancers included in clinical trials are limited, and the clinical effects of some cancer types have yet to be confirmed. In contrast, the target genes of both therapies generally exhibited strong negative correlations in THCA, this suggests combination therapy may not be as effective as monotherapy for patients with this cancer. However, due to the complexities of the mechanisms of ICB combined with lenvatinib, the effectiveness of this combination strategy in specific cancer types cannot be fully revealed from the correlation of target genes alone, and further exploration is needed.
Analysis of Genetic Alterations in ICB and Lenvatinib Targets
Two-level pie charts were used to summarize the most common types of cancers with particularly ICB and lenvatinib target gene mutations, as well as the most common types of target mutations in each cancer type (Supplementary Data 2 and Figures 3A,B). For example, FGFR4 mutations were common in KIRC and SKCM (Figure 3A), whereas the common target mutations in STAD occurred in FGFR2 and VEGFR3 (Figure 3B). A heat map of the overall mutations in target genes showed that the 15 cancers with the highest mutational loads were SKCM, UCEC, LUSC, bladder urothelial carcinoma (BLCA), LUAD, STAD, uterine carcinosarcoma (UCS), COAD, rectum adenocarcinoma (READ), sarcoma (SARC), ESCA, ovarian serous cystadenocarcinoma (OV), GBM, HNSC, cervical squamous cell carcinoma and endocervical adenocarcinoma (CESC), and CHOL (Supplementary Figure 4 and Supplementary Data 3). This sort order of above-mentioned malignances was closely related to the clinical effect of ICB combined with lenvatinib.
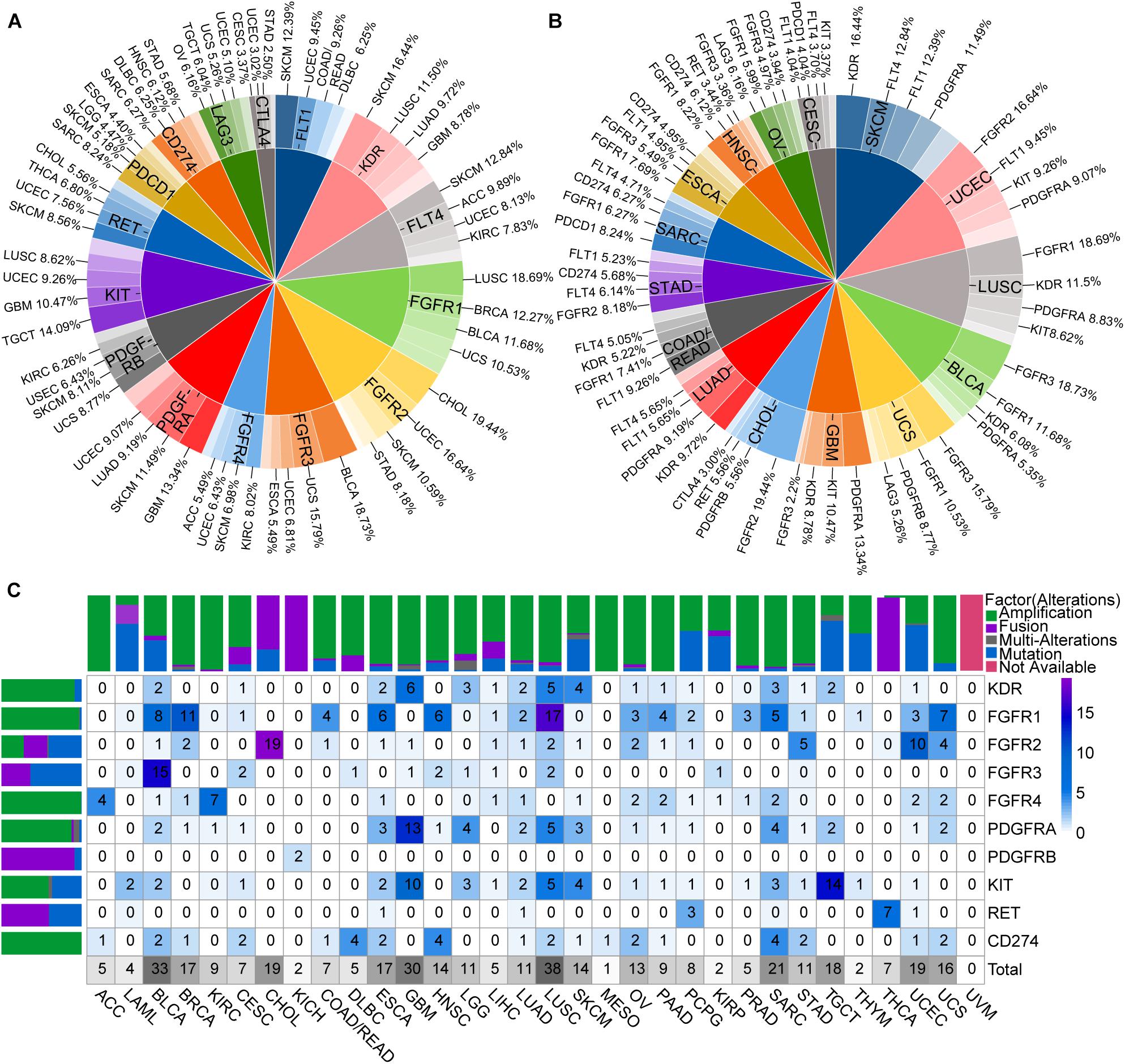
Figure 3. Genomic alterations of ICB and lenvatinib target genes. (A) The most common types of cancers with particularly ICB and lenvatinib target genes alterations. (B) The most common types of target alterations in each cancer type. (C) Driver alterations types and proportions of ICB and lenvatinib target genes in different cancer types. The color of the block represents the proportion of alterations, and the number represents the percentage of alterations. Above is a column chart of alteration types in different cancer types, and on the left is a column chart of alteration types in different targets. ACC, adrenocortical carcinoma; DBLC, diffuse large B-cell lymphoma; LGG, low grade glioma; LAML, acute myeloid leukemia; MESO, mesothelioma; TGCT, testicular germ cell tumors; UCS, uterine carcinosarcoma; UVM, uveal melanoma.
To further explore the driver alterations of target genes in different pathways, mutations and CNA of unknown significance defined by cBioPortal database were excluded as likely passenger alterations. Drive alterations (usually specific hotspot mutations or fusions, amplifications, and multiple alterations involving oncogenes defined by cBioPortal database) are shown in Figure 3C. VEGFR1, VEGFR3, PDCD1, LAG3, and CTLA4 were filtered because of insufficient evidence to drive mutations in cBioPortal database. Therefore, only CD274 (PD-L1) was left as an ICB-target gene. These results demonstrated that driver alterations of ICB and lenvatinib target genes were present in all included cancer types except for uveal melanoma. The cancer types with driver alterations in the lenvatinib target genes occurring at a frequency of ≥9% were as follows (ranked from the highest to the lowest frequency): LUSC, BLCA, GBM, CHOL, testicular germ cell tumors (TGCT), UCEC, SARC, BRCA, ESCA, UCS, SKCM, brain lower grade glioma (LGG), OV, LUAD, KIRC, HNSC, and STAD. The cancer types with a driver alteration in the ICB target gene PD-L1 (amplification in all cases) occurring at a frequency of ≥2% were as follows (ranked from the highest to the lowest frequency): lymphoid neoplasm diffuse large B-cell lymphoma (DLBC), HNSC, SARC, BLCA, CESC, ESCA, LUSC, OV, STAD, and UCS. We also observed that FGFR1 had a high mutation frequency in LUSC and BRCA; FGFR3 had the highest mutation frequency in BLCA. These gene mutations may be the mechanism loci where ICB and lenvatinib play a synergistic role, and explain the clinical efficacy and drug resistance diversity observed for ICB combined with lenvatinib.
Pathway-Enrichment Analysis of the ICB and Lenvatinib Target Genes
The results summarized in Figure 4 show that the ICB and lenvatinib target genes were implicated in multiple pathways associated with tumor development and progression (Supplementary Data 4), including the following terms: Ras signaling pathway, MAPK signaling pathway, PI3K-Akt signaling pathway, regulation of receptor signaling pathway via JAK-STAT, positive regulation of cell cycle. The target genes were also involved in the following antiangiogenic pathways: VEGFR signaling pathway, sprouting angiogenesis, and FGFR signaling pathway. In addition, the target genes of ICB and lenvatinib were significantly enriched in terms of the functional pathways of immune-related genes, including PD-L1 expression and PD-1 checkpoint pathway in cancer, T cell receptor signaling pathway, positive regulation of cell adhesion, T cell activation, and leukocyte proliferation. Finally, the target genes were involved in pathways associated with tumor metabolism, including central carbon metabolism in cancer and choline metabolism in cancer. In addition, this study explored the common pathway connections through which lenvatinib and ICB target genes play a role. KIT, CD274, and CTLA4 are all associated with leukocyte proliferation; KIT, PDCD1, CD274, LAG3, and CTLA4 co-regulatory T cell activation; KDR, RET, PDCD1, CD274, and CTLA4 positive regulation of cell adhesion; FGFR1CD, 274 and LAG3 negative regulation of cytokine production (red circles in Figure 4A). The pathway-network analysis revealed complex interactions among the pathways. Further analysis using the Reactome database (Supplementary Figure 5) showed that the ICB and lenvatinib target genes were significantly enriched in modules such as signal transduction, immune system, disease, hemostasis, metabolism, developmental biology, and extracellular matrix organization. It was further suggested that ICB and lenvatinib-related target genes play roles in multiple pathways related to tumor genesis and development and the immune microenvironment.
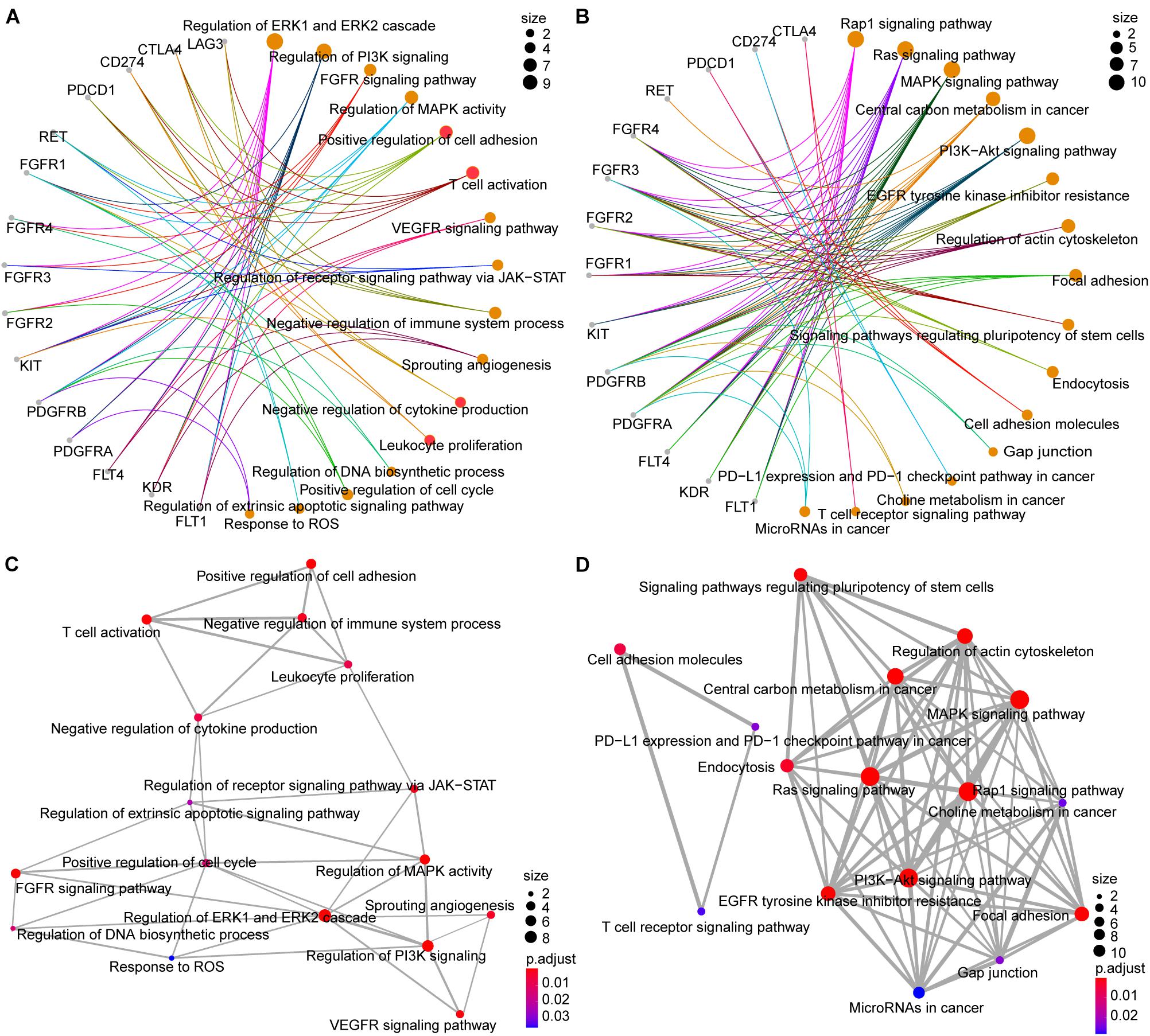
Figure 4. GO and KEGG pathway analysis of lenvatinib and ICB target genes. (A,B) Network diagrams between GO terms (A) KEGG pathways (B) and target genes. (C,D) The emapplot of GO terms (C) and KEGG pathways (D) shown the “pathway–pathway” network. The color of the circle represents the adjusted P-value, and the size of the circle represents the number of enriched genes.
Correlation Analysis Between ICB and Lenvatinib Target Genes, and the Tumor Microenvironment (TME)
Analysis using the TIMER database showed that the expression levels of ICB and lenvatinib target genes generally exhibited strong correlations with the levels of immune-cell infiltrates in the TME (Figure 5A and Supplementary Data 5). In most cancers, including adrenocortical carcinoma (ACC), CESC, COAD, DLBC, KIRC, LIHC, LUAD, prostate adenocarcinoma, SKCM and UCEC (the cancer types shown in the green box), the expression levels of lenvatinib target genes generally showed a negative correlation with the levels of CD8+ T cell infiltrates (≥8 blue squares and more than four of them have an r ≤ –0.15). However, the expression levels of lenvatinib target genes showed positive correlations with the infiltration levels of the immunosuppressive Tregs, M2 macrophages, and MDSCs. Specifically, strong positive correlations (≥8 red squares and more than 4 of them have r ≥ 0.15) were observed with levels of M2 macrophages in COAD, DLBC, ESCA, HNSC, KIRC, READ, STAD, TGCT, THYM, and UCS (the cancer types in orange box), and of MDSCs in ACC, kidney renal papillary cell carcinoma (KIRP), LIHC, OV and THCA (the cancer types in red box). ICB and lenvatinib target genes may jointly influence the level of immune cell infiltration through synergistic or antagonistic effects.
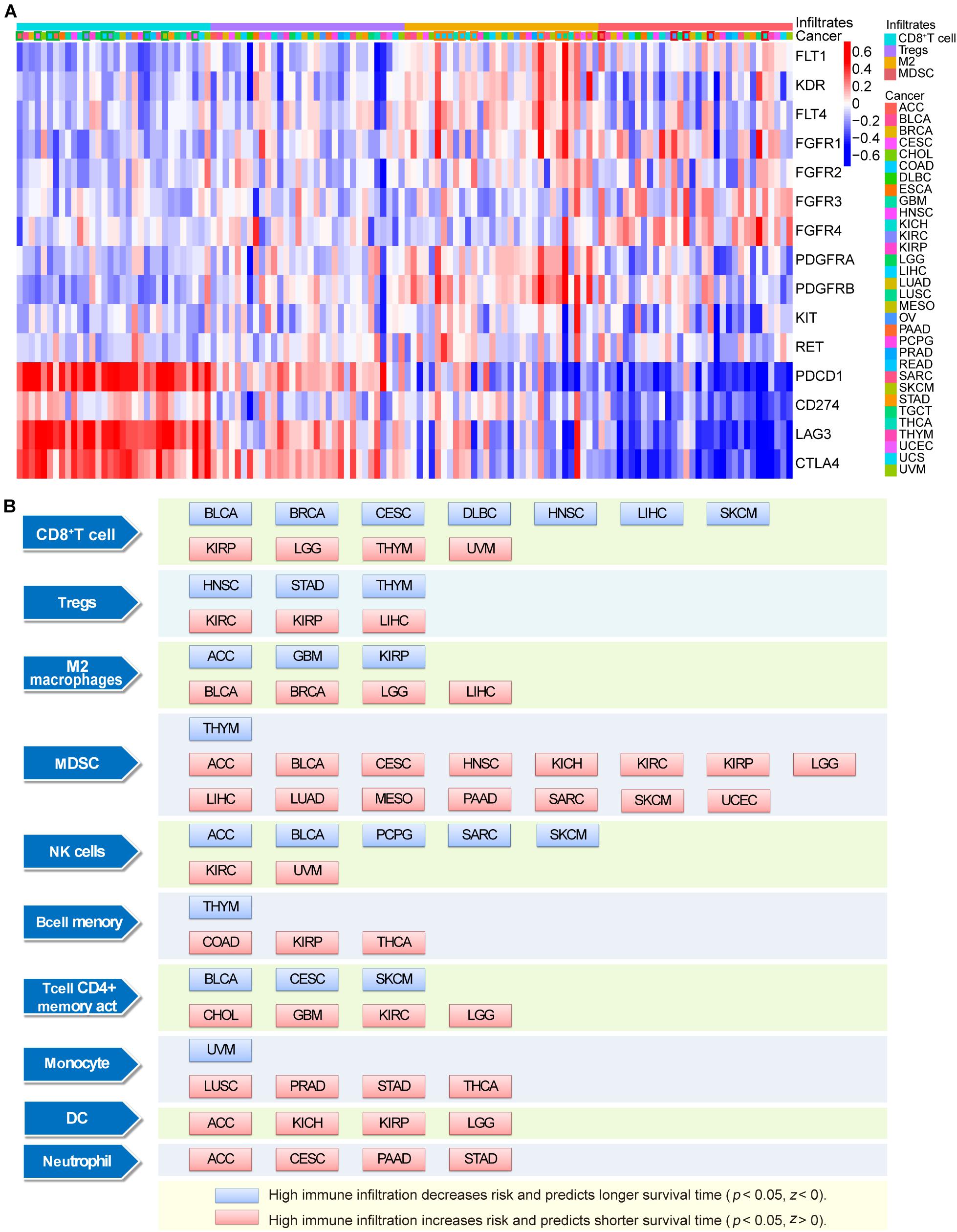
Figure 5. Correlation and survival analysis of immune infiltrating cells with ICB and lenvatinib target genes. (A) The correlation between ICB and lenvatinib target genes and tumor infiltration immune cells. The cancer types indicated with a green box if ≥8 positive correlations and more than 4 of them have an r ≤ –0.15, and the cancer types indicated with an orange or red box if ≥8 negative correlations and more than 4 of them have an r ≥ 0.15. (B) The relationship between immune infiltrates and survival outcomes in malignancy. The blue square represents high immune infiltration decreases risk and predicts longer survival time in certain tumors (P < 0.05, Z < 0), and the red square represents high immune infiltration increases risk and predicts shorter survival time in certain tumors (P < 0.05, Z > 0). Z represents normalized coefficient. DC, dendritic cells; M2, M2 macrophages; MDSC, myeloid-derived suppressor cells.
Survival analysis (Figure 5B) showed that the presence of CD8 + T cells was associated with a favorable prognosis in BLCA, BRCA, CESC, DLBC, HNSC, LIHC, and SKCM, and the highest infiltration levels were predictive of prolonged survival (blue square). The presence of immune cells was identified as a risk factor for poor prognosis in certain cancer types (red square), e.g., Tregs in KIRC, KIRP, and LIHC; M2 macrophages in BLCA, BRCA, LGG, and LIHC; and MDSCs in ACC, BLCA, CESC, HNSC, kidney chromophobe, KIRC, KIRP, LGG, LIHC, LUAD, mesothelioma, PAAD, SARC, SKCM, and UCEC, and immune cell infiltrates were predictive of shorter survival in these malignancies.
When the bioinformatics results were considered together, we found nine tumor types including LIHC, STAD, KIRC, UCEC, LUAD, SKCM, HNSC, CHOL, and GBM with ≥2 positive treatment-related molecular biological characteristics: high expression of ICB and lenvatinib target genes; high mutational loads and high frequency of driver alterations in lenvatinib and ICB target genes; negatively correlated with CD8+ T cell infiltration in lenvatinib target genes (Supplementary Table 2).
Discussion
Lenvatinib is a multitargeting kinase inhibitor that effectively inhibits the activities of a broad spectrum of targets (Supplementary Table 3). It also exhibits unique characteristics in terms of its IC50 value (Supplementary Table 4; Wang et al., 2004; Tian et al., 2011; Stjepanovic and Capdevila, 2014; Kudo, 2018; Lin et al., 2018; Xie et al., 2018) and type-V target-binding mode (Supplementary Table 5; Wang and Long, 2011; Cong-min et al., 2013; Watanabe Miyano et al., 2016; Mori et al., 2017; Roskoski, 2017; Apsel Winger et al., 2019; Kinoshita, 2019). These properties enable lenvatinib to significantly inhibit tumor growth in many types of malignant cancers. Clinical trials of lenvatinib are currently underway (Supplementary Table 6). ICB combined with antiangiogenic therapy is one of the most promising immunotherapeutic combinations. Findings from the present meta-analysis confirm that PD-L1/PD-1 inhibitors combined with lenvatinib result in high ORR, and DCR across patients with multiple different cancer types. However, the complementary and synergistic mechanisms between the two therapy types are currently poorly understood. In the present study we analyzed this topic in detail from a bioinformatics perspective.
The overexpression of lenvatinib target genes and inhibitory immune-checkpoint genes, the positive correlation between the expression levels of these genes, and extensive interactions among the target proteins have suggested the potential for combination therapy (Patel and Minn, 2018; Li et al., 2021). In our expression analysis, the target genes of both ICB and lenvatinib were highly expressed in CHOL, GBM, HNSC, KIRC, LIHC, SKCM, and STAD. This was consistent with the findings from our meta-analysis, which showed that the combination of ICB and lenvatinib achieved better therapeutic effects than either therapy alone in these cancers (Figure 1B). In PAAD and THYM, the target genes of both therapies were not highly expressed, suggesting that combination therapy might not be effective against these cancers, however, this still needs to be clarified in future clinical studies. Combined with a target gene correlation analysis, we found the lenvatinib target genes VEGFR1, VEGFR3, FGFR3, FGFR4, PDGFRA, and the ICB target gene PD-1 were all highly expressed in LIHC, and a relatively strong positive correlation was observed between PD-1 and FGFR3, FGFR4, or PDGFRA (r ≥ 0.15) (Supplementary Figure 3). Accordingly, in clinical trials conducted in unresectable LIHC, the efficacy of combination therapy was superior to that of monotherapy (Figure 1B; Kudo et al., 2018; Zhu et al., 2018; Finn et al., 2020). In THCA, there was an overall strong negative correlation between PD-1/PD-L1 and lenvatinib target genes. Data from different clinical trials in thyroid cancer have shown comparable ORRs with combination therapy (62.0%) and lenvatinib monotherapy (64.8%) (Figure 1B; Schlumberger et al., 2015; Haugen et al., 2020). However, whether lenvatinib monotherapy is inferior to combination therapy in THCA requires further verification in phase III randomized clinical trials. Therefore, it is evident that the expression levels and correlations among lenvatinib and ICB target genes and proteins are closely linked to clinical efficacy. Further studies are required to investigate whether these highly expressed and highly correlated targets can be used as biomarkers for predicting clinical efficacy.
Immune-checkpoint blockade and lenvatinib target genes in tumor-promoting pathways exhibit extensive driver alterations, which may explain the improved efficacy of combination therapy in across multiple cancer types (Le et al., 2015; Bielski et al., 2018). The total mutational loads of the lenvatinib and ICB target genes in pan-cancer are highly consistent with the tumor mutation burden and the degrees of immunogenicity of different types of cancer (Lawrence et al., 2013). The increased production of tumor neoantigens resulting from targeted therapy, together with the high mutational load, further increases the immunogenicity of a tumor. This promotes the extensive infiltration of immune cells and increases immune cell activity, both of which are key factors that enhance the clinical benefit of ICB (Cristescu et al., 2018; Chan et al., 2019). Therefore, cancers with a high number of driver alterations in lenvatinib target genes and a high total mutational load in lenvatinib and ICB target genes may be candidates for combination therapy, e.g., in SKCM, UCEC, LUSC, BLCA, LUAD, STAD, UCS, SARC, ESCA, OV, GBM, HNSC, and CHOL. Interestingly, the total mutational load of LIHC was not high, but lenvatinib targets exhibited extensive driver alterations and were generally highly expressed. Additionally, PD-1 displayed relatively strong positive correlations with FGFR3 and FGFR4 (r ≥ 0.15). These findings are consistent with the unsatisfactory therapeutic effects of single-agent immunotherapy and the enhanced efficacy of combination therapy observed in LIHC (Kudo et al., 2018; Zhu et al., 2018; Finn et al., 2020). In addition, PD-L1 and VEGFR2 in GBM, CTLA4 and PDGFRA in SKCM, as well as PD-L1 and FGFR2 in STAD were all highly expressed genes with driver alterations (Supplementary Table 7). These data may help to inform future explorations of dual-target therapeutic strategies or drug development. However, gene-expression levels, the presence of driver alterations, and the mutational load cannot precisely predict the efficacy of combination therapies for all cancers, as this is also affected by other factors, such as vascular anomalies (Tian et al., 2017), the abundance of immune cell infiltrates in the TME (Roelands et al., 2020), and the heterogeneity of tumor metabolism (Bergers and Fendt, 2021).
Pathway-enrichment analysis showed that the ICB and lenvatinib target genes were implicated in multiple pathways associated with tumor development and progression, angiogenesis, immunotherapy, and tumor metabolism. Additionally, complex interactions among the pathways were observed. The lenvatinib target genes were significantly enriched in multiple pathways associated with tumor development and progression, such as the RTK–RAS–MAPK signaling pathway, the PI-3 kinase signaling pathway, the JAK–STAT signaling pathway, the cell cycle pathway, and the FGFR–FGF signaling pathway. Blockade of these target genes should inhibit tumor cell proliferation or induce tumor cell apoptosis. Because the lenvatinib target genes are associated with signal-transduction pathways downstream of the TCR [e.g., the MAPK–MEK pathway (Ebert et al., 2016) and the PI3K-AKT-mTORpathway (Pollizzi and Powell, 2015)], targeted therapy can also modulate T cells and other immune cells. With regarding to pathways related to angiogenesis, the triple inhibition of VEGFR, FGFR, and PDGFR can inhibit angiogenesis and increase vascular permeability, which rebuilds the TME and increases the local infiltration of immune cells (Naoum et al., 2018; Cabanillas et al., 2019; Yi et al., 2019). Furthermore, the inhibition of FGFR1–4 can help overcome resistance to antiangiogenic drugs (Lee and Secord, 2014; Zheng et al., 2016). Among the immune-related pathways, T cell activation was regulated by KIT, PD-1, PD-L1, LAG3, and CTLA4; cell adhesion was upregulated by VEGFR2, RET, PD-1, PD-L1, and CTLA4; and leukocyte proliferation was regulated by KIT, PD-L1, and CTLA4 (red circles in Figure 4A). For tumor metabolism-related pathways, ICB and lenvatinib can inhibit tumor cell proliferation by downregulating glycolytic enzymes and reducing mTOR activity in tumor cells (Chang et al., 2015). It is evident that the antitumor effect of ICB combined with lenvatinib is modulated by several targets across multiple pathways.
In this study, we also explored the impact of ICB and lenvatinib target genes on the tumor immune microenvironment at the immune cell level. In most cancer types, the expression levels of lenvatinib target genes generally showed a negative correlation with the level of CD8+ T cell infiltration, suggesting that lenvatinib treatment may increase the degree of infiltration of CD8+ T cells. In ACC, KIRP, LIHC, OV, and THCA, the expression levels of lenvatinib target genes generally showed a negative correlation with MDSC infiltration levels, suggesting that lenvatinib treatment may reduce the degree of infiltration of immunosuppressive cells. The combined use of lenvatinib and ICB to promote CD8+ T cell infiltration and inhibit the number and function of Tregs, M2 macrophages, or MDSCs (in terms of IL10 or TGF-β secretion) has been confirmed in murine models of LIHC (Kimura et al., 2018; Kudo, 2019), COAD (Kato et al., 2019), and THCA (Gunda et al., 2019). Lenvatinib can also reduce tumor PD-L1 level to improve anti-PD-1 efficacy by blocking FGFR4 (Yi et al., 2021). The mechanisms of action of lenvatinib include (Figure 6): downregulating the expression of PD-1, CTLA-4, and TIM3 in T cells; blocking the binding of VEGFA and bFGF to their receptors, thereby inhibiting T-cell exhaustion (Deng et al., 2020); and destroying tumor cells, leading to the release of tumor antigens, which in turn promotes IFN-γ-mediated antigen presentation (Kato et al., 2019) and enhances the cytotoxicity of natural killer cells (Zhang et al., 2019). Lenvatinib also impedes angiogenesis and vascular anomalies by inhibiting the secretion of angiogenic factors, such as VEGF, FGF, and PDGF, thereby exerting an immunomodulatory effect (Matsuki et al., 2018). In summary, the synergistic antitumor effect of combined lenvatinib and ICB therapy is achieved by reversing the suppression of the body’s immune functions, increasing tumor immunogenicity, promoting tumor vascular normalization, as well as interact at the transcriptome, genome, and protein levels.
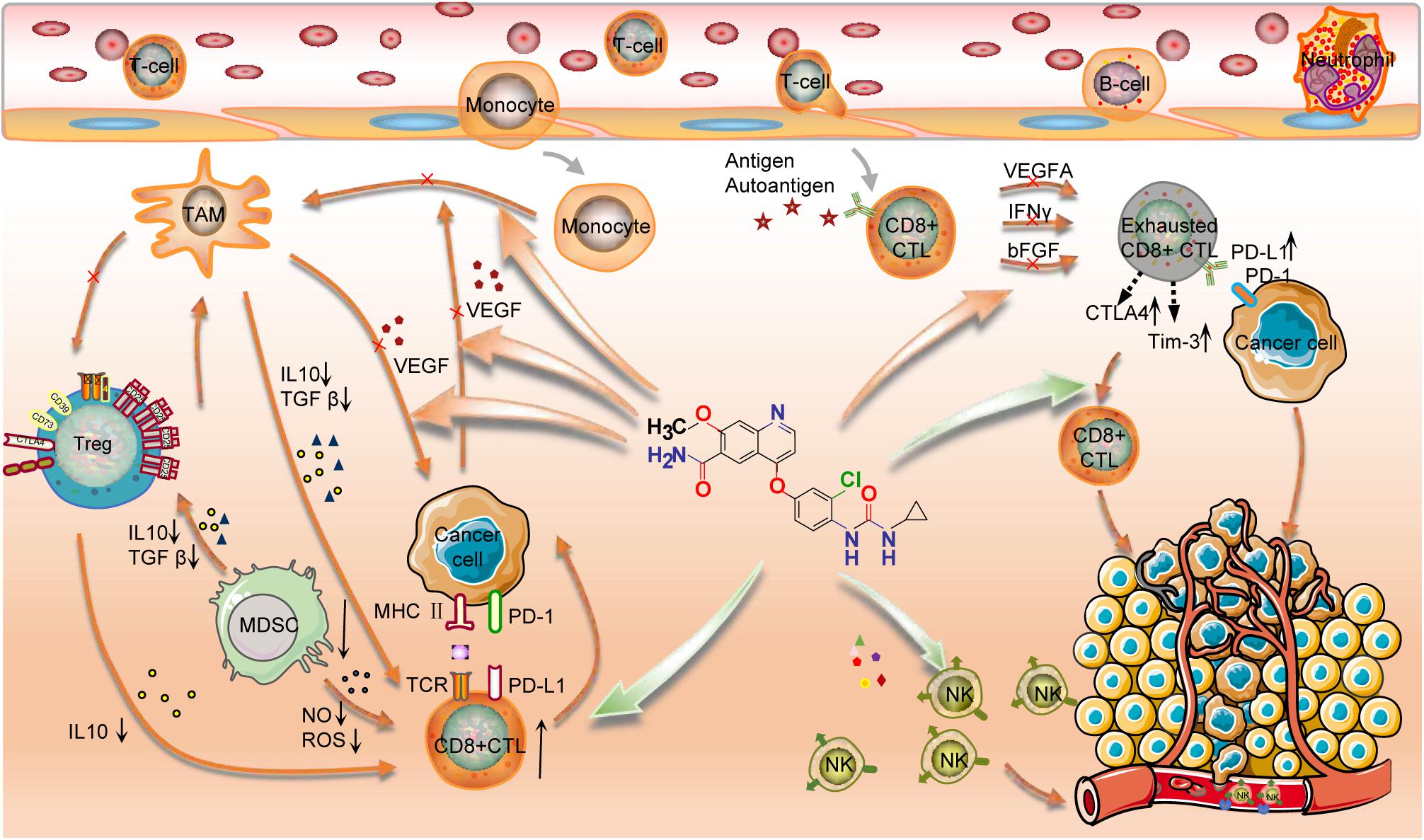
Figure 6. Mechanisms of action of lenvatinib in combination with ICB. Lenvatinib promoted the number and function of CD8+ T cells and inhibited the number and function of Tregs and Macrophages M2; Lenvatinib enhanced the cytotoxicity of NK cells; Lenvatinib decreased the expression of PD-1, CTLA-4, and TIM3 in T cells and inhibited the exhaustion of T cells; Lenvatinib also inhibits tumor angiogenesis and abnormalities by inhibiting the secretion of angiogenic factors, such as VEGF, FGF, and PDGF, etc. Finally, PD-1/PD-L1 Ab restores the exhausted T cell activity to kill the cancer cell. Therefore, this combination has a synergistic anti-tumor effect.
There were certain limitations to our study. Firstly, even though our study covered majority of cancer types, number of tumor types were excluded such as most hematologic cancers. Secondly, although our analyses included relatively large sample sets, larger sample sets are required for further exploration.
Conclusion
This meta-analysis confirmed that ICB combined with lenvatinib exerted greater clinical benefits than monotherapy, across multiple cancers. A multi-omics approach further identified complex and extensive networks connecting the ICB and lenvatinib targets by performing analyses at the gene, protein, and cellular levels, as well as correlation analysis between tumor and immune micro-environment. Most targets were mutually promotional and synergistic, but some of them displayed antagonistic effects. However, the relationships between some targets remained unclear. We found nine tumor types including LIHC, STAD, KIRC, UCEC, LUAD, SKCM, HNSC, CHOL, and GBM with ≥2 positive treatment-related molecular biological characteristics. These findings provide insights for the development of targeted therapy–immunotherapy combinations, and for guiding individualized precision-medicine approaches for cancer treatment.
Data Availability Statement
The datasets presented in this study can be found in online repositories. The names of the repository/repositories and accession number(s) can be found in the article/Supplementary Material.
Author Contributions
YL, QL, QD, and JJ conceptualized and designed the manuscript. YL, QD, YW, MW, MH, HL, and QL acquired, analyzed, and interpreted the data. YL wrote and edited the manuscript. YL, JJ, QD, YW, MW, MH, HL, and QL reviewed and revised the manuscript. All authors contributed to the article and approved the submitted version.
Funding
This work was supported by the National Natural Science Foundation of China (81301912); the Beijing Municipal Health and Scientific and Technological Achievements and Appropriate Technology Promotion Project (BHTPP202008); the “High-value Patent Cultivation” project of the Beijing Friendship Hospital Affiliated to the Capital Medical University (yyzscq202003); and the Research Foundation of Beijing Friendship Hospital (yyqdkt2019-40). The funders were not involved in the design of the study, the collection, analysis or interpretation of the data or the writing of the study.
Conflict of Interest
The authors declare that the research was conducted in the absence of any commercial or financial relationships that could be construed as a potential conflict of interest.
Publisher’s Note
All claims expressed in this article are solely those of the authors and do not necessarily represent those of their affiliated organizations, or those of the publisher, the editors and the reviewers. Any product that may be evaluated in this article, or claim that may be made by its manufacturer, is not guaranteed or endorsed by the publisher.
Acknowledgments
We thank Y. C. Tong and X. L. Wu for helpful writing comments and data checking in the manuscript, and we also thank the open-access databases for data sharing and processing.
Supplementary Material
The Supplementary Material for this article can be found online at: https://www.frontiersin.org/articles/10.3389/fcell.2021.730240/full#supplementary-material
Footnotes
- ^ http://ualcan.path.uab.edu/
- ^ https://string-db.org
- ^ https://xenabrowser.net/
- ^ http://gepia.cancer-pku.cn/detail.php
- ^ https://www.cbioportal.org/
- ^ www.oncokb.org
- ^ www.cancerhotspots.org
- ^ https://reactome.org/
- ^ http://timer.cistrome.org/
References
Adams, S., Schmid, P., Rugo, H. S., Winer, E. P., Loirat, D., Awada, A., et al. (2019). Pembrolizumab monotherapy for previously treated metastatic triple-negative breast cancer: cohort A of the phase II KEYNOTE-086 study. Ann. Oncol.: Off. J. Eur. Soc. Med. Oncol. 30, 397–404. doi: 10.1093/annonc/mdy517
Al-Salama, Z. T., Syed, Y. Y., and Scott, L. J. (2019). Lenvatinib: a review in hepatocellular carcinoma. Drugs 79, 665–674. doi: 10.1007/s40265-019-01116-x
Apsel Winger, B., Cortopassi, W. A., Garrido Ruiz, D., Ding, L., Jang, K., Leyte-Vidal, A., et al. (2019). ATP-competitive inhibitors midostaurin and avapritinib have distinct resistance profiles in exon 17-Mutant KIT. Cancer Res. 79, 4283–4292. doi: 10.1158/0008-5472.CAN-18-3139
Bellmunt, J., de Wit, R., Vaughn, D. J., Fradet, Y., Lee, J. L., Fong, L., et al. (2017). Pembrolizumab as second-line therapy for advanced urothelial carcinoma. N. Engl. J. Med. 376, 1015–1026. doi: 10.1056/NEJMoa1613683
Bergers, G., and Fendt, S. M. (2021). The metabolism of cancer cells during metastasis. Nat. Rev. Cancer 21, 162–180. doi: 10.1038/s41568-020-00320-2
Bielski, C. M., Donoghue, M. T. A., Gadiya, M., Hanrahan, A. J., Won, H. H., Chang, M. T., et al. (2018). Widespread selection for oncogenic mutant allele imbalance in cancer. Cancer Cell 34, 852.e4–862.e4. doi: 10.1016/j.ccell.2018.10.003
Boss, D. S., Glen, H., Beijnen, J. H., Keesen, M., Morrison, R., Tait, B., et al. (2012). A phase I study of E7080, a multitargeted tyrosine kinase inhibitor, in patients with advanced solid tumours. Br. J. Cancer 106, 1598–1604. doi: 10.1038/bjc.2012.154
Burtness, B., Harrington, K. J., Greil, R., Soulières, D., Tahara, M., de Castro, G., et al. (2019). Pembrolizumab alone or with chemotherapy versus cetuximab with chemotherapy for recurrent or metastatic squamous cell carcinoma of the head and neck (KEYNOTE-048): a randomised, open-label, phase 3 study. Lancet 394, 1915–1928. doi: 1
Cabanillas, M. E., Ryder, M., and Jimenez, C. (2019). Targeted therapy for advanced thyroid cancer: kinase inhibitors and beyond. Endocr. Rev. 40, 1573–1604. doi: 10.1210/er.2019-00007
Chan, T. A., Yarchoan, M., Jaffee, E., Swanton, C., Quezada, S. A., Stenzinger, A., et al. (2019). Development of tumor mutation burden as an immunotherapy biomarker: utility for the oncology clinic. Ann. Oncol. : Off. J. Eur. Soc. Med. Oncol. 30, 44–56. doi: 10.1093/annonc/mdy495
Chang, C. H., Qiu, J., O’Sullivan, D., Buck, M. D., Noguchi, T., Curtis, J. D., et al. (2015). Metabolic competition in the tumor microenvironment is a driver of cancer progression. Cell 162, 1229–1241. doi: 10.1016/j.cell.2015.08.016
Chung, H. C. (2021). LEAP-005: a phase II multicohort study of lenvatinib plus pembrolizumab in patients with previously treated selected solid tumors: results from the gastric cancer cohort. J. Clin. Oncol. 39:230. doi: 10.1016/j.annonc.2021.05.194
Cong-min, K., Ying-jie, D., and Qiao-yan, W. (2013). Small-molecule inhibitors of protein tyrosine kinases and their mechanisms of action. Chin. J. N. Drugs 22, 1170–1178.
Cristescu, R., Mogg, R., Ayers, M., Albright, A., Murphy, E., Yearley, J., et al. (2018). Pan-tumor genomic biomarkers for PD-1 checkpoint blockade-based immunotherapy. Science (New York, NY) 362:eaar3593. doi: 10.1126/science.aar3593
Deng, H., Kan, A., Lyu, N., Mu, L., Han, Y., Liu, L., et al. (2020). Dual vascular endothelial growth factor receptor and fibroblast growth factor receptor inhibition elicits antitumor immunity and enhances programmed cell Death-1 checkpoint blockade in hepatocellular carcinoma. Liver Cancer 9, 338–357. doi: 10.1159/000505695
Druker, B. J. (2003). David A. Karnofsky Award lecture. Imatinib as a paradigm of targeted therapies. J. Clin. Oncol.: Off. J. Am. Soc. Clin. Oncol. 21, (Suppl. 23) 239s–245s. doi: 10.1200/JCO.2003.10.589
Ebert, P. J. R., Cheung, J., Yang, Y., McNamara, E., Hong, R., Moskalenko, M., et al. (2016). MAP kinase inhibition promotes T cell and anti-tumor activity in combination with PD-L1 checkpoint blockade. Immunity 44, 609–621. doi: 10.1016/j.immuni.2016.01.024
Fernandez, A. M. A., O’Day, S. J., Merino, L. D. L. C., Petrella, T., Jamal, R., and Ny, L. (2020). LBA44 Lenvatinib (len) plus pembrolizumab (pembro) for advanced melanoma (MEL) that progressed on a PD-1 or PD-L1 inhibitor: initial results of LEAP-004. Ann. Oncol. 31:S1173. doi: 10.1016/j.annonc.2020.08.2274
Finn, R. S., Ikeda, M., Zhu, A. N. X., Sung, M. W., Baron, A. D., Kudo, M., et al. (2020). Phase Ib study of lenvatinib plus pembrolizumab in patients with unresectable hepatocellular carcinoma. J. Clin. Oncol. 38, 2960–2970. doi: 10.1200/JCO.20.00808
Gomez-Roca, C., Yanez, E., and Im, S. A. (2021). Eduardo castanon alvarez. LEAP-005: a phase II multicohort study of lenvatinib plus pembrolizumab in patients with previously treated selected solid tumors—Results from the colorectal cancer cohort. J. Clin. Oncol. 39:3564. doi: 10.1200/JCO.2021.39.15_suppl.3564
Gunda, V., Gigliotti, B., Ashry, T., Ndishabandi, D., McCarthy, M., Zhou, Z., et al. (2019). Anti-PD-1/PD-L1 therapy augments lenvatinib’s efficacy by favorably altering the immune microenvironment of murine anaplastic thyroid cancer. Int. J. Cancer 144, 2266–2278. doi: 10.1002/ijc.32041
Haugen, B., French, J., Worden, F. P., Konda, B., Sherman, E. J., Dadu, R., et al. (2020). Lenvatinib plus pembrolizumab combination therapy in patients with radioiodine-refractory (RAIR), progressive differentiated thyroid cancer (DTC): results of a multicenter phase II international thyroid oncology group trial. J. Clin. Oncol. 38:6512. doi: 10.1200/JCO.2020.38.15_suppl.6512
He, X., and Xu, C. (2020). Immune checkpoint signaling and cancer immunotherapy. Cell Res. 30, 660–669. doi: 10.1038/s41422-020-0343-4
Hida, T., Velcheti, V., Reckamp, K. L., Nokihara, H., Sachdev, P., Kubota, T., et al. (2019). A phase 2 study of lenvatinib in patients with RET fusion-positive lung adenocarcinoma. Lung Cancer 138, 124–130. doi: 10.1016/j.lungcan.2019.09.011
Iwasa, S., Okita, N., Kuchiba, A., Ogawa, G., Kawasaki, M., Nakamura, K., et al. (2020). Phase II study of lenvatinib for metastatic colorectal cancer refractory to standard chemotherapy: the LEMON study (NCCH1503). ESMO Open 5:e000776. doi: 10.1136/esmoopen-2020-000776
Kato, Y., Tabata, K., Kimura, T., Yachie-Kinoshita, A., Ozawa, Y., Yamada, K., et al. (2019). Lenvatinib plus anti-PD-1 antibody combination treatment activates CD8+ T cells through reduction of tumor-associated macrophage and activation of the interferon pathway. PLoS One 14:e0212513. doi: 10.1371/journal.pone.0212513
Kawazoe, A., Fukuoka, S., Nakamura, Y., Kuboki, Y., Wakabayashi, M., Nomura, S., et al. (2020). Lenvatinib plus pembrolizumab in patients with advanced gastric cancer in the first-line or second-line setting (EPOC1706): an open-label, single-arm, phase 2 trial. Lancet Oncol. 21, 1057–1065. doi: 10.1016/S1470-2045(20)30271-0
Kimura, T., Kato, Y., Ozawa, Y., Kodama, K., Ito, J., Ichikawa, K., et al. (2018). Immunomodulatory activity of lenvatinib contributes to antitumor activity in the Hepa1-6 hepatocellular carcinoma model. Cancer Sci. 109, 3993–4002. doi: 10.1111/cas.13806
Kinoshita, T. (2019). Protein allostery in rational drug design. Adv. Exp. Med. Biol. 1163, 45–64. doi: 10.1007/978-981-13-8719-7_3
Kudo, M. (2018). Lenvatinib may drastically change the treatment landscape of hepatocellular carcinoma. Liver Cancer 7, 1–19. doi: 10.1159/000487148
Kudo, M. (2019). Targeted and immune therapies for hepatocellular carcinoma: predictions for 2019 and beyond. World J. Gastroenterol. 25, 789–807. doi: 10.3748/wjg.v25.i7.789
Kudo, M., Finn, R. S., Qin, S., Han, K. H., Ikeda, K., Piscaglia, F., et al. (2018). Lenvatinib versus sorafenib in first-line treatment of patients with unresectable hepatocellular carcinoma: a randomised phase 3 non-inferiority trial. Lancet (London, England) 391, 1163–1173. doi: 10.1016/S0140-6736(18)30207-1
Kudo, M., Ikeda, M., Motomura, K., Okusaka, T., Kato, N., Dutcus, C. E., et al. (2020). A phase Ib study of lenvatinib (LEN) plus nivolumab (NIV) in patients (pts) with unresectable hepatocellular carcinoma (uHCC): study 117. J. Clin. Oncol. 38:513. doi: 10.1200/JCO.2020.38.4_suppl.513
Lawrence, M. S., Stojanov, P., Polak, P., Kryukov, G. V., Cibulskis, K., Sivachenko, A., et al. (2013). Mutational heterogeneity in cancer and the search for new cancer-associated genes. Nature 499, 214–218. doi: 10.1038/nature12213
Le, D. T., Uram, J. N., Wang, H., Bartlett, B. R., Kemberling, H., Eyring, A. D., et al. (2015). PD-1 blockade in tumors with mismatch-repair deficiency. N. Engl. J. Med. 372, 2509–2520. doi: 10.1056/NEJMoa1500596
Lee, C. H., Shah, A. Y., Hsieh, J. J., Rao, A., Pinto, A., Bilen, M. A., et al. (2020). Phase II trial of lenvatinib (LEN) plus pembrolizumab (PEMBRO) for disease progression after PD-1/PD-L1 immune checkpoint inhibitor (ICI) in metastatic clear cell renal cell carcinoma (mccRCC). J. Clin. Oncol. 38:2. doi: 10.1200/JCO.2020.38.15_suppl.5008
Lee, P. S., and Secord, A. A. (2014). Targeting molecular pathways in endometrial cancer: a focus on the FGFR pathway. Cancer Treat Rev. 40, 507–512. doi: 10.1016/j.ctrv.2013.11.004
Li, H., Kuang, X., Liang, L., Ye, Y., Zhang, Y., Li, J., et al. (2021). The beneficial role of sunitinib in tumor immune surveillance by regulating tumor PD-L1. Adv. Sci. (Weinheim, Baden-Wurttemberg, Germany) 8:2001596. doi: 10.1002/advs.202001596
Li, Q., Chen, M., Cao, M., Yuan, G., Hu, X., Dai, W., et al. (2020). 182P Lenvatinib (LEN) plus anti-PD-1 antibodies vs LEN alone for advanced hepatocellular carcinoma (HCC): a real-world study. Ann. Oncol. 31:S1310. doi: 10.1016/j.annonc.2020.10.203
Lin, J., Yang, X., Long, J., Zhao, S., Mao, J., Wang, D., et al. (2020). Pembrolizumab combined with lenvatinib as non-first-line therapy in patients with refractory biliary tract carcinoma. Hepatobiliary Surg. Nutr. 9, 414–424. doi: 10.21037/hbsn-20-338
Lin, Y. Y., Tan, C. T., Chen, C. W., Ou, D. L., Cheng, A. L., and Hsu, C. (2018). Immunomodulatory effects of current targeted therapies on hepatocellular carcinoma: implication for the future of immunotherapy. Semin. Liver Dis. 38, 379–388. doi: 10.1055/s-0038-1673621
Lwin, Z., Gomez-Roca, C., Saada-Bouzid, E., Yanez, E., Muñoz, F. L., Im, S. A., et al. (2020). LBA41 LEAP-005: phase II study of lenvatinib (len) plus pembrolizumab (pembro) in patients (pts) with previously treated advanced solid tumours. Ann. Oncol. 31:S1170. doi: 10.1016/j.annonc.2020.08.2271
Makker, V., Rasco, D., Vogelzang, N. J., Brose, M. S., Cohn, A. L., Mier, J., et al. (2019). Lenvatinib plus pembrolizumab in patients with advanced endometrial cancer: an interim analysis of a multicentre, open-label, single-arm, phase 2 trial. Lancet Oncol. 20, 711–718.
Makker, V., Taylor, M. H., Aghajanian, C., Oaknin, A., Mier, J., Lee Cohn, A., et al. (2020). Lenvatinib (LEN) plus pembrolizumab (PEMBRO) for early-line treatment of advanced/recurrent endometrial cancer (EC). J. Clin. Oncol. 38:6083. doi: 10.1200/JCO.2020.38.15_suppl.6083
Matsuki, M., Hoshi, T., Yamamoto, Y., Ikemori-Kawada, M., Minoshima, Y., Funahashi, Y., et al. (2018). Lenvatinib inhibits angiogenesis and tumor fibroblast growth factor signaling pathways in human hepatocellular carcinoma models. Cancer Med. 7, 2641–2653. doi: 10.1002/cam4.1517
Matulonis, U. A., Shapira-Frommer, R., Santin, A. D., Lisyanskaya, A. S., Pignata, S., Vergote, I., et al. (2019). Antitumor activity and safety of pembrolizumab in patients with advanced recurrent ovarian cancer: results from the phase II KEYNOTE-100 study. Ann. Oncol.: Off. J. Eur. Soc. Med. Oncol. 30, 1080–1087. doi: 10.1093/annonc/mdz135
Mok, T. S. K., Wu, Y. L., Kudaba, I., Kowalski, D. M., Cho, B. C., Turna, H. Z., et al. (2019). Pembrolizumab versus chemotherapy for previously untreated, PD-L1-expressing, locally advanced or metastatic non-small-cell lung cancer (KEYNOTE-042): a randomised, open-label, controlled, phase 3 trial. Lancet (London, England). 393, 1819–1830. doi: 10.1016/S0140-6736(18)32409-7
Mori, M., Kaneko, N., Ueno, Y., Yamada, M., Tanaka, R., Saito, R., et al. (2017). Gilteritinib, a FLT3/AXL inhibitor, shows antileukemic activity in mouse models of FLT3 mutated acute myeloid leukemia. Invest. N. Drugs 35, 556–565. doi: 10.1007/s10637-017-0470-z
Motzer, R. J., Hutson, T. E., Glen, H., Michaelson, M. D., Molina, A., Eisen, T., et al. (2015). Lenvatinib, everolimus, and the combination in patients with metastatic renal cell carcinoma: a randomised, phase 2, open-label, multicentre trial. Lancet Oncol. 16, 1473–1482. doi: 10.1016/S1470-2045(15)00290-9
Motzer, R. J., Porta, C., Eto, M., Powles, T., Grünwald, V., and Hutson, T. E. (2021). Phase 3 trial of lenvatinib (LEN) plus pembrolizumab (PEMBRO) or everolimus (EVE) versus sunitinib (SUN) monotherapy as a first-line treatment for patients (pts) with advanced renal cell carcinoma (RCC) (CLEAR study). Genitourinary Cancers Sympos.: Am. Soc. Clin. Oncol. 39:269. doi: 10.1200/JCO.2021.39.6_suppl.269
Naoum, G. E., Morkos, M., Kim, B., and Arafat, W. (2018). Novel targeted therapies and immunotherapy for advanced thyroid cancers. Mol. Cancer 17:51. doi: 10.1186/s12943-018-0786-0
O’Day, S., Gonzalez, R., Kim, K., Chmielowski, B., Kefford, R., Loquai, G. L., et al. (2013). A phase II study of the multitargeted kinase inhibitor lenvatinib in patients with advanced BRAF wild-type melanoma. J. Clin. Oncol. 31:abstr9026. doi: 10.1200/jco.2013.31.15_suppl.9026
Ott, P. A., Bang, Y. J., Piha-Paul, S. A., Razak, A. R. A., Bennouna, J., Soria, J. C., et al. (2019). T-Cell-Inflamed gene-expression profile, programmed death ligand 1 expression, and tumor mutational burden predict efficacy in patients treated with pembrolizumab across 20 cancers: keynote-028. J. Clin. Oncol.: Off. J. Am. Soc. Clin. Oncol. 37, 318–327. doi: 10.1200/JCO.2018.78.2276
Patel, S. A., and Minn, A. J. (2018). Combination cancer therapy with immune checkpoint blockade: mechanisms and strategies. Immunity 48, 417–433. doi: 10.1016/j.immuni.2018.03.007
Pollizzi, K. N., and Powell, J. D. (2015). Regulation of T cells by mTOR: the known knowns and the known unknowns. Trends Immunol. 36, 13–20. doi: 10.1016/j.it.2014.11.005
Powles, T., Plimack, E. R., Soulières, D., Waddell, T., Stus, V., Gafanov, R., et al. (2020). Pembrolizumab plus axitinib versus sunitinib monotherapy as first-line treatment of advanced renal cell carcinoma (KEYNOTE-426): extended follow-up from a randomised, open-label, phase 3 trial. Lancet Oncol. 21, 1563–1573. doi: 10.1016/S1470-2045(20)30436-8
Ribas, A., Puzanov, I., Dummer, R., Schadendorf, D., Hamid, O., Robert, C., et al. (2015). Pembrolizumab versus investigator-choice chemotherapy for ipilimumab-refractory melanoma (KEYNOTE-002): a randomised, controlled, phase 2 trial. Lancet Oncol. 16, 908–918. doi: 10.1016/S1470-2045(15)00083-2
Roelands, J., Hendrickx, W., Zoppoli, G., Mall, R., Saad, M., Halliwill, K., et al. (2020). Oncogenic states dictate the prognostic and predictive connotations of intratumoral immune response. J. Immunother. Cancer 8:e000617. doi: 10.1136/jitc-2020-000617
Roskoski, R. Jr. (2017). ROS1 protein-tyrosine kinase inhibitors in the treatment of ROS1 fusion protein-driven non-small cell lung cancers. Pharmacol. Res. 121, 202–212. doi: 10.1016/j.phrs.2017.04.022
Schlumberger, M., Tahara, M., Wirth, L. J., Robinson, B., Brose, M. S., Elisei, R., et al. (2015). Lenvatinib versus placebo in radioiodine-refractory thyroid cancer. N. Engl. J. Med. 372, 621–630. doi: 10.1056/NEJMoa1406470
Stjepanovic, N., and Capdevila, J. (2014). Multikinase inhibitors in the treatment of thyroid cancer: specific role of lenvatinib. Biol. Targets Therapy 8, 129–139. doi: 10.2147/BTT.S39381
Suyama, K., and Iwase, H. (2018). Lenvatinib: a promising molecular targeted agent for multiple cancers. Cancer Control: J. Moffitt Cancer Center 25:1073274818789361. doi: 10.1177/1073274818789361
Taylor, M. H., Lee, C.-H., Makker, V., Rasco, D., Dutcus, C. E., Wu, J., et al. (2020). Phase IB/II trial of lenvatinib plus pembrolizumab in patients with advanced renal cell carcinoma, endometrial cancer, and other selected advanced solid tumors. J. Clin. Oncol. 38, 1154–1163. doi: 10.1200/JCO.19.01598
Tian, L., Goldstein, A., Wang, H., Ching, L. H., Sun, K. I., and Welte, T. (2017). Mutual regulation of tumour vessel normalization and immunostimulatory reprogramming. Nature 544, 250–254. doi: 10.1038/nature21724
Tian, S., Quan, H., Xie, C., Guo, H., Lu, F., Xu, Y., et al. (2011). YN968D1 is a novel and selective inhibitor of vascular endothelial growth factor receptor-2 tyrosine kinase with potent activity in vitro and in vivo. Cancer Sci. 102, 1374–1380. doi: 10.1111/j.1349-7006.2011.01939.x
Villanueva, L. (2021). Lenvatinib plus pembrolizumab for patients with previously treated biliary tract cancers in the multicohort phase II LEAP-005 study. J. Clin. Oncol. 39:321. doi: 10.1200/JCO.2021.39.3_suppl.321
Wang, Y., Fei, D., Vanderlaan, M., and Song, A. (2004). Biological activity of bevacizumab, a humanized anti-VEGF antibody in vitro. Angiogenesis 7, 335–345. doi: 10.1007/s10456-004-8272-2
Wang, Y., and Long, Y. (2011). Advances in small-molecule inhibitors of protein tyrosine kinases. Chin. J. Organ. Chem. 31, 1595–1606.
Watanabe Miyano, S., Yamamoto, Y., Kodama, K., Miyajima, Y., Mikamoto, M., Nakagawa, T., et al. (2016). E7090, a novel selective inhibitor of fibroblast growth factor receptors, displays potent antitumor activity and prolongs survival in preclinical models. Mol. Cancer Ther. 15, 2630–2639. doi: 10.1158/1535-7163.MCT-16-0261
Wei, S. C., Duffy, C. R., and Allison, J. P. (2018). Fundamental mechanisms of immune checkpoint blockade therapy. Cancer Discov. 8, 1069–1086. doi: 10.1158/2159-8290.CD-18-0367
Xie, C., Wan, X., Quan, H., Zheng, M., Fu, L., Li, Y., et al. (2018). Preclinical characterization of anlotinib, a highly potent and selective vascular endothelial growth factor receptor-2 inhibitor. Cancer Sci. 109, 1207–1219. doi: 10.1111/cas.13536
Yi, C., Chen, L., Ling, Z., Liu, L., Shao, W., Zhang, R., et al. (2021). Lenvatinib targets FGFR4 to enhance antitumor immune response of Anti-PD-1 in hepatocellular carcinoma. Hepatology (Baltimore, Md) [Online ahead of print] doi: 10.1002/hep.31921
Yi, M., Jiao, D., Qin, S., Chu, Q., Wu, K., and Li, A. (2019). Synergistic effect of immune checkpoint blockade and anti-angiogenesis in cancer treatment. Mol. Cancer 18:60. doi: 10.1186/s12943-019-0974-6
Zhang, Q., Liu, H., Wang, H., Lu, M., Miao, Y., Ding, J., et al. (2019). Lenvatinib promotes antitumor immunity by enhancing the tumor infiltration and activation of NK cells. Am. J. Cancer Res. 9, 1382–1395.
Zheng, N., Wei, W., and Wang, Z. (2016). Emerging roles of FGF signaling in hepatocellular carcinoma. Translational Cancer Res. 5, 1–6.
Zhu, A. X., Finn, R. S., Edeline, J., Cattan, S., Ogasawara, S., Palmer, D., et al. (2018). Pembrolizumab in patients with advanced hepatocellular carcinoma previously treated with sorafenib (KEYNOTE-224): a non-randomised, open-label phase 2 trial. Lancet Oncol. 19, 940–952. doi: 10.1016/S1470-2045(18)30351-6
Keywords: immune-checkpoint blockade, lenvatinib, synergistic mechanism, multi-omics analysis, malignancy
Citation: Lu Y, Jin J, Du Q, Hu M, Wei Y, Wang M, Li H and Li Q (2021) Multi-Omics Analysis of the Anti-tumor Synergistic Mechanism and Potential Application of Immune Checkpoint Blockade Combined With Lenvatinib. Front. Cell Dev. Biol. 9:730240. doi: 10.3389/fcell.2021.730240
Received: 24 June 2021; Accepted: 16 August 2021;
Published: 09 September 2021.
Edited by:
Yi Zhang, First Affiliated Hospital of Zhengzhou University, ChinaReviewed by:
Macrina Beatriz Silva Cázares, Autonomous University of San Luis Potosí, MexicoJohn Morton, University of Colorado, United States
Copyright © 2021 Lu, Jin, Du, Hu, Wei, Wang, Li and Li. This is an open-access article distributed under the terms of the Creative Commons Attribution License (CC BY). The use, distribution or reproduction in other forums is permitted, provided the original author(s) and the copyright owner(s) are credited and that the original publication in this journal is cited, in accordance with accepted academic practice. No use, distribution or reproduction is permitted which does not comply with these terms.
*Correspondence: Qin Li, cWlubGkxMjgwMDNAY2NtdS5lZHUuY24=