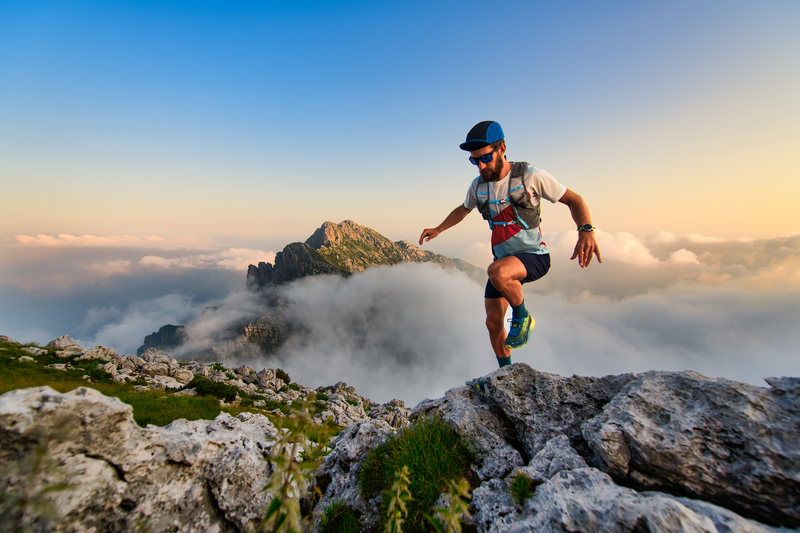
94% of researchers rate our articles as excellent or good
Learn more about the work of our research integrity team to safeguard the quality of each article we publish.
Find out more
ORIGINAL RESEARCH article
Front. Cell Dev. Biol. , 13 September 2021
Sec. Molecular and Cellular Oncology
Volume 9 - 2021 | https://doi.org/10.3389/fcell.2021.727935
This article is part of the Research Topic The Role of Tumor Microenvironment in Primary Liver Cancer Therapeutic Resistance View all 10 articles
Background: 5-Methylcytosine (m5C) plays essential roles in hepatocellular carcinoma (HCC), but the association between m5C regulation and immune cell infiltration in HCC has not yet been clarified.
Methods: In this study, we analysed 371 patients with HCC from The Cancer Genome Atlas (TCGA) database, and the expression of 13 m5C regulators was investigated. Additionally, gene set variation analysis (GSVA), unsupervised clustering analysis, single-sample gene set enrichment analysis (ssGSEA), correlation analysis, and immunohistochemical (IHC) staining were performed.
Results: Among the 371 patients, 41 had mutations in m5C regulators, the frequency of which was 11.26%. Compared with normal hepatic tissues, the expression of m5C regulators with copy number variations (CNVs) expansion was significantly higher than that in HCC tissues. Then, we identified three m5C modification patterns that had obvious tumour microenvironment (TME) cell infiltration characteristics. The prognostic analysis of the three major m5C modification subtypes showed that Cluster-2 had a clear survival advantage over the others. In addition, we found that DNMT1 was highly expressed in tumour tissues compared with normal tissues in a tissue microarray (TMA) and that it was positively correlated with many TME-infiltrating immune cells. High expression of the m5C regulator DNMT1 was related to a poor prognosis in patients with HCC. Furthermore, we developed three distinct Immu-clusters. Importantly, mRNAs related to the transcription of growth factor β (TGF-β)/EMT pathway were significantly up-regulated in Immu-cluster 2, indicating that this cluster is considered to be the immune rejection phenotype. Immu-cluster 3 showed elevated expression of mRNAs related to immune checkpoint genes.
Conclusion: Our work revealed the association between m5C modification and immune regulators in the TME. These findings also suggest that DNMT1 has great potential as a prognostic biomarker and therapeutic target for HCC.
Hepatocellular carcinoma (HCC) is the sixth most common cancer and the fourth leading cause of cancer-related death worldwide (Llovet et al., 2021). Risk factors for HCC include hepatitis B virus (HBV), hepatitis C virus (HCV), non-alcoholic fatty liver disease, obesity with diabetes, etc. Patients who are infected with HCV can be treated with antiviral therapies, while patients who are infected with HBV remain infected throughout life (European Association for the Study of the Liver, 2017). The survival of patients is driven by tumour stage, with a 5-year survival rate exceeding 70% for those with early-stage HCC compared to a median survival time of 1–1.5 years for those with advanced-stage HCC (Llovet et al., 2016). Most HCC patients are diagnosed at advanced stages, and limited effective therapeutic strategies are available (Hernandez-Gea et al., 2013).
Tumour cells are the driving cause of tumour development and progression. However, without the tumour microenvironment (TME), tumour cells cannot act alone in the progression of cancer. The TME includes the surrounding blood vessels, fibroblasts, immune cells, extracellular matrix, and signalling molecules. These elements contribute to the processes of carcinogenesis and progression, while it is still a major challenge to fully evaluate the complex TME (Hanahan and Coussens, 2012).
Epigenetic deregulation, such as aberrant DNA methylation and reversible chemical RNA modifications play a critical role in cancer (Davalos et al., 2018; García-Vílchez et al., 2019). Previous studies have mainly focused on m6A modification in regulating coding and non-coding RNA processing and function (Nombela et al., 2021). Emerging evidence has revealed the important role of 5-methylcytosine (m5C) in posttranscriptional regulation (Xue et al., 2020a). In addition, m5C modification was found to be abundant in mammalian cells, characterised by the addition of a methyl group at the carbon-5 position of the cytosine base (Bestor, 1988). m5C is mainly distributed in GC-rich areas. Over 10,000 potential sites of m5C modification have been detected in the whole human transcriptome (Bourgeois et al., 2015). The regulation of m5C is a dynamic process controlled by three major regulators, termed “writers” (add a special modification), “readers” (identify and bind modified nucleotides), and “erasers” (remove a special modification) (Yang et al., 2018).
Recently, targeting the TME has been an encouraging method for cancer treatment (Bejarano et al., 2021). Some studies showed a correlation between m6A and TME-infiltrating immune cells (Yi et al., 2020; Zhang et al., 2020a; Chong et al., 2021; Shen et al., 2021). However, due to technological limitations, the research above was restricted to one or two type of modification regulators or cell types, while anti-tumour effects involve multiple tumour suppressors interacting in a vitally cooperative way. Hence, a deep understanding of TME cell infiltration mediated by several regulators of gene modifications will help to enhance the perception of TME immune regulation, especially m5C modification.
In this study, we analysed 371 patients with HCC from The Cancer Genome Atlas (TCGA) database, and the samples were integrated to evaluate m5C modification patterns. Correlation analysis was performed between the m5C modification pattern and TME cell infiltration characteristics. Three different m5C modification patterns were discovered based on the expression of 13 m5C regulators. Besides, we found that distinct m5C modification patterns were closely associated with different enrichment pathways and immune cell infiltration characteristics, indicating that m5C modification might play an essential role in forming an individual TME.
Gene expression and clinical annotation data were downloaded from the TCGA database. Patients without complete survival data were excluded. The TCGA-Liver Hepatocellular Carcinoma (TCGA-LIHC) dataset was used for further analysis. Finally, a total of 371 patient in the TCGA-LIHC cohort were selected for this study.
For the TCGA dataset, the R package TCGA biolinks (Colaprico et al., 2016), which was developed to analyse Genomic Data Commons (GDC) data, was utilised to download the fragments per kilobase per million mapped reads (FPKM) values of gene expression from the GDC.1 FPKM values were further converted to transcripts per kilobase million (TPM) values. Batch effects generated by factors unrelated to any biological variations were corrected for using the parametric and non-parametric empirical Bayes framework algorithm from the sva package. Data related to somatic mutations were downloaded from the TCGA database. R (3.6.1) together with Bioconductor packages were employed in the study.
A total of 13 m5C regulators were extracted from 371 patients in the TCGA-LIHC cohort: 11 writers (NOP2, NSUN2, NSUN3, NSUN4, NSUN5, NSUN6, NSUN7, DNMT1, TRDMT1, DNMT3A, and DNMT3B), 1 eraser (TET2), and 1 reader (ALYREF). Unsupervised clustering analysis was employed to distinguish different m5C modifications, after which the classification of patients was conducted for subsequent analysis.
A consensus clustering algorithm (Hartigan and Wong, 1979) was employed to assure the number of clusters and their stability. The ConsensusClusterPlus package was applied to execute the workflow mentioned above, and the stability of the classification was accomplished by conducting 1000 repetitions (Wilkerson and Hayes, 2010).
To explore the disparity of biological processes in m5C modification patterns, the gene set variation analysis (“GSVA”) R package was used to perform GSVA. This package is based on a non-parametric and unsupervised algorithm and is widely used to estimate the variation in gene set enrichment in expression datasets (Hänzelmann et al., 2013). GSVA was implemented with “c2.cp.kegg.v6.2.symbols” gene sets obtained from the Molecular Signatures Database (MsigDB). An adjusted P-value of less than 0.05 was regarded as statistically significant. We applied the “ClusterProfiler” R package to functionally annotate m5C-related genes under the false discovery rate (FDR) threshold of <0.05.
The single-sample gene set enrichment analysis (ssGSEA) algorithm was used to determine the relative richness in cell infiltration in the TME. We obtained the gene set associated with each infiltrating immune cell type in the TME from Charoentong, who stores information on various human immune cells, including CD8 T cells, dendritic cells (DCs), natural killer (NK) T cells, macrophages, regulatory T cells, etc. (Barbie et al., 2009; Charoentong et al., 2017). ssGSEA was employed to determine the enrichment scores and define the relative abundance of each TME-infiltrating cell type in the corresponding sample.
With the aim of distinguishing m5C-related genes, all the patients were divided into three m5C modification patterns according to the expression of m5C regulators. The empirical Bayesian algorithm under the limma package in R was used to assure differentially expressed genes (DEGs) in heterogeneous modification patterns.
A set of genes was constructed by Mariathasan et al. (2018), Rosenberg et al. (2016) and Şenbabaoğlu et al. (2016), in which genes associated with certain biological processes are stored. Correlation analysis was employed to explore the association between the gene signature of m5C and biological pathways.
Human liver cell line Huh7 and paired normal human liver cell L02 were purchased from Chinese Academy of Sciences (Shanghai, China) and cultured in DMEM (Gibco, Carlsbad, CA, United States) supplemented with 10% fetal bovine serum (FBS; Gibco; Thermo Fisher Scientific) and 1% penicillin n (MP Biomedicals, Santa Ana, CA, United States). The cells were cultured at 37°C in atmosphere of 5% CO2.
Total RNA from Huh7 cell line was extracted with Rneasy Mini Kit (Qiagen, Valencia, CA, United States) and then reverse-transcribed into cDNA preformed using the PrimeScriptTM RT reagent Kit. GAPDH was used as the internal control. The expression levels of 11 writers (NOP2, NSUN2, NSUN3, NSUN4, NSUN5, NSUN6, NSUN7, DNMT1, TRDMT1, DNMT3A, and DNMT3B), 1 eraser (TET2), and 1 reader (ALYREF) were quantified using 2−ΔΔCt method by ABI7500fast PCR instrument. The primers are listed in Supplementary Table 1.
Human HCC tissue arrays and normal tissues (catalogue number: HlivH180Su15) were purchased from Shanghai Outdo Biotech Co., Ltd. (Shanghai, China). The method of immunohistochemical (IHC) staining has been reported previously. Briefly, antigen retrieval was performed by heating the tissue sections at 100°C for 30 min in target retrieval solution. Then, the tissue microarray (TMA) was incubated with a DNMT1 primary antibody [(EPR18453) (ab188453) Abcam, Cambridge, MA, United States], followed by incubation with an anti-rabbit secondary antibody. Two independent pathologists blindly assessed the IHC results according to the staining area and intensity (Zhang et al., 2020b).
Spearman and distance correlation analyses were performed to obtain the correlation coefficients of the TME-infiltrating immune cells and the corresponding expression of m5C regulators. Student’s T-test was used for comparisons two groups. One-way analysis of variance (ANOVA) and Kruskal–Wallis tests were performed to compare differences between three or more groups (Hazra and Gogtay, 2016). The Kaplan–Meier method was utilised to generate survival curves for the prognostic analysis, and the log-rank test was applied to identify significant differences. Univariate Cox regression was adopted to determine the hazard ratios of m5C regulators and genes related to specific m5C phenotypes. Multivariable Cox regression was utilised to identify independent prognostic risk factors. Patients with complete relevant data were subjected to further analysis with a multivariate model. The multivariate results were visualised with the forestplot package in R. Copy number variations (CNVs) in 13 m5C regulators were plotted with the Rcircos package (Mayakonda et al., 2018). All P-values were two-sided, with P < 0.05 considered statistically significant. The analysis was accomplished in R 3.6.1 software.
A total of 13 regulators of m5C were identified, including 11 writers, 1 eraser, and 1 reader. First, the incidence of CNVs and somatic mutations in regulators in HCC were summarised. In 364 samples, 41 showed mutations in m5C regulators, the occurrence of which was 11.26%. DNMT1 was found to be exposed to a higher frequency of mutations, followed by DNMT3A, while ALYREF, NSUN2, NSUN3, and NSUN5 were not (Figure 1A). CNVs were also detected in 13 other regulators upon exploration of their modification frequencies. Most of the modifications involved a copy number expansion, but TET2, NOP2, and NSUN4 had a broad occurrence of deletions (Figure 1B). The chromosome sites of the m5C regulators are shown in Figure 1C. Based on the expression of 13 m5C regulators in HCC patients, HCC samples could be thoroughly differentiated from normal samples (Figure 1D). To determine whether the expression of m5C regulators was influenced by the genetic mutations mentioned above, the mRNA expression of regulators was explored. We found that a change in m5C was an important factor leading to perturbations in the expression of m5C regulators. Compared with normal hepatic tissues, the expression of m5C regulators with a CNV expansion was significantly higher than that in HCC tissues (e.g., ALYREF and NSUN2) (Figures 1B,E). In addition, the expression of in HCC cell line Huh7 and normal control cell line L02 were detected by quantitative reverse-transcription PCR (qRT-PCR). Assistant with the expression in TCGA, the expression of ALYREF, DNMT1, DNMT3A, DNMT3B, NOP2, NSUN3, NSUN4, NSUN5, NSUN6, NSUN7, and TET2 were higher in HCC cell line Huh7 than in normal cell line L02. While the expression level of TRDMT1 and NSUN2 was lower in Huh7 than in L02 (Figure 1F). The analyses above showed that the genetic and expression alteration landscape of m5C regulators in normal tissues and HCC tissues is highly heterogeneous, suggesting that the expression imbalance of m5C regulators plays an important role in HCC occurrence and progression.
Figure 1. Copy number variations and somatic mutations in 13 m5C regulators in HCC. (A) Mutation frequencies of the top 9 m5C regulators. (B) CNV alterations among the 13 regulators. (C) Locations of mutations in the m5C regulators at the chromosome level. (D) Principal component analysis was used to distinguish tumour tissues and normal tissues based on the expression of m5C regulators. (E) The expression profiles of m5C regulator genes in tumour tissues and adjacent normal tissues. (F) qRT-PCR was used to determine the relative expression of NOP2, NSUN2, NSUN3, NSUN4, NSUN5, NSUN6, NSUN7, DNMT1, TRDMT1, DNMT3A, DNMT3B, TET2, and ALYREF in HCC cell line Huh7 and normal control cell line L02. *p < 0.05, **p < 0.01, ***p < 0.001.
Univariate Cox regression analysis showed that 13 m5C modulators have prognostic significance in HCC patients (Figure 2A). The m5C regulator network revealed m5C modulator interactions, modulator connections and their prognostic significance for patients (Figure 2B). The R package Consensus Cluster Plus was applied to classify patients with qualitatively different m5C alteration patterns according to the expression of 13 m5C regulators, and unsupervised clustering analysis was performed to identify a total of 3 different modification patterns (120 cases in modification pattern 1, 178 cases in modification pattern 2, and 73 cases in modification pattern 3; referred to as m5C Clusters 1–3, respectively) (Figure 2C and Supplementary Table 2). The prognostic analysis of the three major m5C modification subtypes showed that Cluster-2 had a clear survival advantage over the others (Figure 2D). The above results indicate that the regulators of m5C may play an important role in m5C alteration patterns and TME cell infiltration characteristics between individual tumours.
Figure 2. 5-Methylcytosine methylation alteration patterns and related biological characteristics. (A) Univariate Cox regression analysis of the 13 m5C regulators in patients with HCC. (B) The network of m5C regulators and their prognostic significance for HCC patients. (C) Unsupervised clustering analysis of 13 m5C regulators in HCC. (D) Survival analysis of HCC patients in the TCGA-LIHC cohort according to the three m5C clusters. (E,F) A heatmap of GSVA results shows the representative hallmark pathways associated with distinct m5C modification patterns.
To investigate the biological actions associated with m5C modification patterns, GSVA was conducted. As shown in Figure 2E and Supplementary Table 2, m5C Cluster-2 was remarkably enriched in carcinogenesis pathways, such as the ERBB signalling pathway, cell cycle signalling pathway, and adherens junction pathway. Cluster-1 was associated with many metabolism pathways, such as, oxidative phosphorylation, linoleic acid metabolism, arachidonic acid metabolism, arginine and proline metabolism, and nitrogen metabolism (Figure 2E). Cluster-3 was highly associated with spliceosome (Figure 2F). Further analysis of TME cell infiltration showed that Cluster-1
was significantly enriched in the infiltration of innate immune cells, including eosinophils, NK cells, macrophages, CD8 T cells, and mast cells (Figure 3A). Prior research has shown that tumours with an immune rejection phenotype exhibit large amounts of immune cells, and these immune cells are in the matrix around the tumour cell nest instead of inside the tissue (Chen and Mellman, 2017). GSVA showed that the modification of Cluster-1 was significantly related to matrix activation. Therefore, it was speculated that the Cluster-1 matrix serves as an activation inhibitor of the anti-tumour effect of immune cells. Further analysis showed that matrix activity was greatly upgraded in Cluster 1, activating the angiogenesis pathway. These results supported our hypothesis (Figure 3B).
Figure 3. Tumour microenvironment characteristics in different m5C modification patterns. (A) Comparison of the abundance of immune infiltrating cells in three clusters. (B) Differences in cellular biological pathways among the three clusters. *p < 0.05, **p < 0.01, ***p < 0.001.
To further explore the role of each m5C regulator in the TME, Spearman correlation analysis was applied to examine the correlation between each TME-infiltrating cell type and m5C regulators (Figure 4A). An emphasis was placed on the regulator DNMT1, an m5C methyltransferase, and we revealed its positive relationship with the infiltration of many TME immune cells. An estimation method was applied to determine the expression of DNMT1 and the infiltration of immune cells. The results showed that higher DNMT1 expression was related to a higher immune score, which means that a TME with high DNMT1 expression has significantly high immune cell infiltration (Figure 4B). Based on these results, the specific differences in 23 TME-infiltrating immune cells were explored between patients with high and low DNMT1 expression. We found that tumours exhibiting high DNMT1 expression had markedly more infiltration of 13 TME immune cells than those exhibiting low expression (Figure 4C). Recently, attention was drawn to the regulatory mechanisms of m5C modification on the activation of DCs, which are the bridge connecting innate immunity with adaptive immunity, the activation of which depends on upregulating the expression of MHC molecules, adhesion molecules, and costimulatory molecules (Figure 4D). As expected, subsequent enrichment analysis showed that tumours with high DNMT1 expression showed remarkable enrichment in immune activation pathways (Figure 4E). Therefore, it was speculated that m5C methylation modification mediated by DNMT1 may contribute to activated DCs in the TME, thus promoting the anti-tumour immune response in HCC.
Figure 4. Association of TME-infiltrating cells with the m5C regulator of DNMT1. (A) Correlation between m5C regulators and different immune cells using Spearman analysis. (B) Immune scores of the low DNMT1 group and the high DNMT1 group. (C) Comparison of the abundance of immune-infiltrating cells in the low DNMT1 group and high DNMT1 group. (D) Correlation between m5C regulators and the activation of dendritic cells. (E) High DNMT1 expression shows significant enhancement of the immune-activated pathway. *p < 0.05, **p < 0.01, ***p < 0.001.
Immunohistochemical staining was used to determine the expression pattern of DNMT1 on a TMA consisting of 90 pairs of HCC tissues and adjacent tissues. Representative micrographs illustrate the various degrees of DNMT1 expression (Figures 5A,B). The expression of DNMT1 was higher in tumour tissues than in control tissues (Figure 5C), which was consistent with the findings in the TCGA-LIHC cohort (Figure 1E). The correlation of DNMT1 expression with the clinicopathological characteristics of patients with HCC is shown in Supplementary Table 3. In addition, Kaplan–Meier curve analysis showed that patients with high DNMT1 expression had shorter overall survival (OS) than those with low DNMT1 expression (Figure 5D). Univariable and multivariable Cox regression analyses were used to determine whether the expression of DNMT1 was an independent risk factor. The univariable analysis revealed that DNMT1 expression was associated with tumour size and TB, AFP, and PD-L1 levels (P < 0.05, Supplementary Table 4). Further analysis demonstrated that DNMT1 might serve as a prognostic predictor for HCC.
Figure 5. Expression of DNMT1 in human HCC tumour tissues and control tissues. (A) Panoramic scanning of DNMT1 by IHC staining. (B) Representative IHC staining of DNMT1 in samples. (C) The expression of DNMT1 is higher in HCC tissues than in normal tissues. (D) Kaplan–Meier analysis showed that patients with higher levels of DNMT1 had shorter OS times than those with low levels of DNMT1. *p < 0.05.
For subsequent exploration of the biological behaviour of each m5C modification pattern, we ascertained 307 m5C phenotype-related DEGs with the limma package (Figure 6A). Cluster profiler was employed to implement enrichment analysis on the DEGs. Supplementary Table 5 summarises the significantly enriched pathways. As expected, we detected enrichment in biological processes that are notably related to m5C modification and immunity, which verified the important role that m5C modification plays in immune regulation in the TME (Figure 6B).
Figure 6. Identification of distinct Immu-clusters based on immune-related DEGs in m5C modification patterns. (A) A total of 307 m5C-related DEGs between three m5C clusters were identified, as shown in the Venn diagram. (B) Enrichment of biological processes significantly related to DEGs. (C) The selected genes were used to classify patients into different genomic subtypes by unsupervised clustering analysis. (D) Kaplan–Meier curves indicated that the genomic subtypes were correlated with the prognosis of patients with HCC. (E) Significant differences in the expression of m5C regulators. **p < 0.01, ***p < 0.001.
To further explain the association, we performed unsupervised clustering analysis to classify 307 m5C phenotype-related genes and extracting 27 immune-related genes: VIPR2, CCL7, RBP2, SLC10A2, FGF5, DEFA5, HTR3A, TRH, LCN15, AMBN, ADIPOQ, FGF3, CCK, NTF4, NDP, FGF9, PF4, CMA1, SFTPA2, CGB8, DEFA6, PF4V1, IL25, GH2, FGF8, SST, and
IAPP. Furthermore, we performed unsupervised clustering analysis based on these genes to categorise patients into different subtypes (Supplementary Figures 1A–D). In line with the clustering analysis of m5C modification patterns, unsupervised clustering analysis revealed three different m5C-modified phenotypes termed Immu-clusters 1–3, respectively. Thus, there are three different distinct immune-related m5C methylation patterns. We observed that tumours in Immu-clusters 2 and 3 were associated with poor differentiation and enriched in diffuse histological subtypes. The opposite pattern was observed in Immu-cluster 1. Patients whose survival status was known were mainly concentrated in Immu-cluster 1, while patients in clinical stage IV or with a high TNM grade were mainly concentrated in Immu-cluster 2 (Figure 6C). The analysis also showed that three different gene clusters had different feature genes (Figure 6C). In total, 114 of the 317 HCC patients clustered in Immu-cluster 1, which was associated with a better prognosis. The prognosis of patients in Immu-cluster 2 (110 patients) and Immu-cluster 3 (93 patients) was poor (Figure 6D). In the three immune clusters, a significant distinction in the expression of m5C regulatory factors emerged. This result was consistent with the m5C methylation modification patterns (Figure 6E).
To further explain the role that m5C-related phenotypes play in TME immune regulation, the levels of immune cells and expression of chemokines and cytokines in the three Immu-clusters were examined. The chosen cytokines and chemokines were taken from previously existing studies (Turley et al., 2015). Our analysis showed that activated CT4 T cells, immature B cells, regulatory T cells, NK cells, macrophages, mast cells, myeloid-derived suppressor cells (MDSCs), monocytes, neutrophils, and plasmacytoid DCs were significantly different among the Immu-clusters. Besides, the immunosuppressive cells (including MDSCs and regulatory T cells) were significantly upregulated in Immu-cluster 2 (Figure 7A). Tumour necrosis factor, interferon, CD8A, CXCL9, CXCL10, GZMA, GZMB, PRF1, and TBX2 were associated with immune activation transcription (Barbie et al., 2009; Zeng et al., 2019). The expression of TNF and TBX2 were different in this three Immu-clusters (Figure 7B). PD-L1, CD80, CD86, CTLA-4, HAVCR2, etc., were thought to be related to the transcription of immune checkpoints. We compared the transcription of these immune checkpoint genes in the three Immu-clusters and found that the expression of most of the immune checkpoint genes were remarkedly different (Figure 7C). ACTA2, CLDN3, VIM, COL4A1, SMAD9, TWIST1, TGFBR2, TGRB1, and ZEB1 are related to the transcription of growth factor β (TGF-β)/EMT pathway transformation and exhibited significant differences between the three Immu-clusters (Figure 7D). We found that mRNAs related to the TGF-β/EMT pathway were significantly upregulated in Immu-cluster 2, indicating that this cluster is the matrix-activated group and associated with immunosuppression. Immu-cluster 3 showed elevated expression of mRNAs related to immune checkpoint genes, suggesting that the patients in this group may respond better to immune checkpoint drugs, which requires further study.
Figure 7. Association between the expression of m5C regulators and immunoregulation in the TME. (A) Differences in immune cell infiltration in the three Immu-clusters. (B) Comparison of immune-related cytokine expression in the three Immu-clusters. (C) Comparison of the transcription of immune checkpoint genes in the three Immu-clusters. (D) Immu-clusters involved in the transcription of the TGF-β/EMT pathway. *p < 0.05, **p < 0.01, ***p < 0.001.
According to previous reports, tumours, including HCC, are mainly driven by genetic mutations. In recent years, epigenetic modifications have been found to play a critical role in the carcinogenesis and molecular pathogenesis of HCC (Xue et al., 2020a; Nombela et al., 2021). m5C is the most preventative and best understood DNA modification in eukaryotes (Piperi and Papavassiliou, 2011). In recent years, emerging evidence has revealed the important role of RNA m5C in posttranscriptional regulation. Several studies have revealed that m5C regulators and m5C methylation play essential roles in different cancer types, including HCC. He et al. (2020b) found that ALYREF and NSUN4 could be promising targets for HCC therapies. In addition, studies showed the map of m5C methylation based on HCC tissues and paired non-tumour tissues at the mRNA, lncRNA, and circRNA levels (He et al., 2020a,c; Zhang et al., 2020c). Recent studies showed that NSUN2 could promote tumour progression in HCC (Sun et al., 2020) and gastric cancer (Mei et al., 2020). Similar to our findings, Cui et al. (2021) and Xue et al. (2020b) found that DNMT1 played important roles in head and neck squamous cell carcinoma.
Recently, increasing evidence has shown interactions between the tumour immune-microenvironment (TIME) and m6A modifications. Yi et al. (2020) reported that copy number alterations in m6A methylation regulators affected immune cell infiltration in head and neck squamous cell carcinoma. Lin et al. (2020) also attempted to explore the relationship between m6A regulators and tumour-infiltrating immune cells by ssGSEA in glioma. Shen et al. (2021) found that m6A modification patterns were correlated with immune regulation in HCC and might provide novel immune therapeutic targets. However, as an important epigenetic modification, the role of m5C methylation in the immune regulation of HCC is still unclear. Here, we described the TME cell infiltration characteristics in different m5C modification patterns. Furthermore, we identified three distinct immune-related m5C methylation subtypes and investigated the levels of immune cells and expression of chemokines and cytokines in the three Immu-clusters. All the results indicate that the generation of immune-related m5C methylation subtypes contribute to understanding the molecular mechanisms of HCC and provide novel clues for predicting the prognosis of patients with HCC.
It has been demonstrated that DNMT1 is an essential methyltransferase for the maintenance of DNA methylation. Previous evidence has shown that DNMT1 is overexpressed in breast cancer (Wang et al., 2018), thyroid cancer cells (Zhang et al., 2018), and pancreatic cancer (Peng et al., 2005). Furthermore, high DNMT1 expression is significantly associated with a poor prognosis (Li et al., 2010; Hong et al., 2018). Consistent with our results, we found that DNMT1 expression was increased in tumour tissues compared with normal tissues in the TMA and TCGA cohort. In our study, Kaplan–Meier curve analysis and univariable and multivariable Cox regression analysis further demonstrated that the expression of DNMT1 is an independent risk factor for HCC. Therefore, DNMT1 might serve as a promising prognostic predictor and therapeutic target for HCC.
Taken together, our results showed the association between m5C modification and TME. Moreover, we found a key m5C modification regulator, DNMT1, which has great potential as a prognostic biomarker and therapeutic target for HCC.
The datasets presented in this study can be found in online repositories. The names of the repository/repositories and accession number(s) can be found in the article/Supplementary Material.
The studies involving human participants were reviewed and approved by The First Affiliated Hospital, College of Medicine, Zhejiang University. The patients/participants provided their written informed consent to participate in this study.
XG and HZo designed the whole study. QZ and JW conducted the statistical analysis. XG and QC draft the manuscript. QC made the relevant edits to the manuscript. XG and HZu revised the manuscript. All authors read and approved the final manuscript.
This study was supported by grants awarded by the National Science and Technology Major Project of China (No. 2018ZX10302206), Science and Technology Major Projects of Zhejiang Province (No. 2018C04016), and the Science and Technology Major Projects of Ningbo (No. 2016C51008).
The authors declare that the research was conducted in the absence of any commercial or financial relationships that could be construed as a potential conflict of interest.
All claims expressed in this article are solely those of the authors and do not necessarily represent those of their affiliated organizations, or those of the publisher, the editors and the reviewers. Any product that may be evaluated in this article, or claim that may be made by its manufacturer, is not guaranteed or endorsed by the publisher.
The Supplementary Material for this article can be found online at: https://www.frontiersin.org/articles/10.3389/fcell.2021.727935/full#supplementary-material
Supplementary Figure 1 | Consensus map of unsupervised clustering results used for subtype analysis.
Supplementary Table 1 | The primers for the qRT-PCR assay.
Supplementary Table 2 | Estimating the relative abundance of tumour cells in the TME in the TCGA-LIHC cohort by single-sample gene set enrichment analysis (ssGSEA).
Supplementary Table 3 | Activation states of biological pathways in distinct m5C modification patterns by GSVA.
Supplementary Table 4 | Correlation between DNMT1 expression and clinicopathological characteristics.
Supplementary Table 5 | Univariate and multivariate analyses of the factors correlated with the OS of HCC patients.
Supplementary Table 6 | Functional annotation of m5C phenotype-related genes.
m5C, 5-methylcytosine; HCC, hepatocellular carcinoma; TCGA, The Cancer Genome Atlas; GSVA, gene set variation analysis; ssGSEA, single-sample gene set enrichment analysis; IHC, immunohistochemical; TME, tumour microenvironment; TMA, tissue microarray; HBV, hepatitis B virus; HCV, hepatitis C virus; m6A, N6-methyladenosine; GDC, Genomic Data Commons; FPKM, fragments per kilobase per million mapped reads; TPM, transcripts per kilobase million; MsigDB, Molecular Signatures Database; FDR, false discovery rate; DCs, dendritic cells; NK, natural killer; DEGs, differentially expressed genes; ANOVA, one-way analysis of variance; CNVs, copy number variations; OS, overall survival; MDSCs, myeloid-derived suppressor cells; TIME, tumour immune-microenvironment.
Barbie, D. A., Tamayo, P., Boehm, J. S., Kim, S. Y., Moody, S. E., Dunn, I. F., et al. (2009). Systematic RNA interference reveals that oncogenic KRAS-driven cancers require TBK1. Nature 462, 108–112. doi: 10.1038/nature08460
Bejarano, L., Jordāo, M. J. C., and Joyce, J. A. (2021). Therapeutic targeting of the tumor microenvironment. Cancer Discov. 11, 933–959. doi: 10.1158/2159-8290.Cd-20-1808
Bestor, T. H. (1988). Cloning of a mammalian DNA methyltransferase. Gene 74, 9–12. doi: 10.1016/0378-1119(88)90238-7
Bourgeois, G., Ney, M., Gaspar, I., Aigueperse, C., Schaefer, M., Kellner, S., et al. (2015). Eukaryotic rRNA modification by yeast 5-methylcytosine-methyltransferases and human proliferation-associated antigen p120. PLoS One 10:e0133321. doi: 10.1371/journal.pone.0133321
Charoentong, P., Finotello, F., Angelova, M., Mayer, C., Efremova, M., Rieder, D., et al. (2017). Pan-cancer immunogenomic analyses reveal genotype-immunophenotype relationships and predictors of response to checkpoint blockade. Cell Rep. 18, 248–262. doi: 10.1016/j.celrep.2016.12.019
Chen, D. S., and Mellman, I. (2017). Elements of cancer immunity and the cancer–immune set point. Nature 541, 321–330. doi: 10.1038/nature21349
Chong, W., Shang, L., Liu, J., Fang, Z., Du, F., Wu, H., et al. (2021). m(6)A regulator-based methylation modification patterns characterized by distinct tumor microenvironment immune profiles in colon cancer. Theranostics 11, 2201–2217. doi: 10.7150/thno.52717
Colaprico, A., Silva, T. C., Olsen, C., Garofano, L., Cava, C., Garolini, D., et al. (2016). TCGAbiolinks: an R/Bioconductor package for integrative analysis of TCGA data. Nucleic Acids Res. 44:e71. doi: 10.1093/nar/gkv1507
Cui, J., Zheng, L., Zhang, Y., and Xue, M. (2021). Bioinformatics analysis of DNMT1 expression and its role in head and neck squamous cell carcinoma prognosis. Sci. Rep. 11:2267. doi: 10.1038/s41598-021-81971-5
Davalos, V., Blanco, S., and Esteller, M. (2018). SnapShot: messenger RNA modifications. Cell 174:46. doi: 10.1016/j.cell.2018.06.046
European Association for the Study of the Liver. (2017). EASL 2017 clinical practice guidelines on the management of hepatitis B virus infection. J. Hepatol. 67, 370–398. doi: 10.1016/j.jhep.2017.03.021
García-Vílchez, R., Sevilla, A., and Blanco, S. (2019). Post-transcriptional regulation by cytosine-5 methylation of RNA. Biochim. Biophys. Acta Gene Regul. Mech. 1862, 240–252. doi: 10.1016/j.bbagrm.2018.12.003
Hanahan, D., and Coussens, L. M. (2012). Accessories to the crime: functions of cells recruited to the tumor microenvironment. Cancer Cell 21, 309–322. doi: 10.1016/j.ccr.2012.02.022
Hänzelmann, S., Castelo, R., and Guinney, J. (2013). GSVA: gene set variation analysis for microarray and RNA-seq data. BMC Bioinform. 14:7. doi: 10.1186/1471-2105-14-7
Hartigan, J. A., and Wong, M. A. (1979). Algorithm AS 136: A K-means clustering algorithm. J. R. Stat. Soc. C 28, 100–108. doi: 10.2307/2346830
Hazra, A., and Gogtay, N. (2016). Biostatistics series module 3: comparing groups: numerical variables. Indian J. Dermatol. 61, 251–260. doi: 10.4103/0019-5154.182416
He, Y., Shi, Q., Zhang, Y., Yuan, X., and Yu, Z. (2020a). Transcriptome-wide 5-methylcytosine functional profiling of long non-coding RNA in hepatocellular carcinoma. Cancer Manag. Res. 12, 6877–6885. doi: 10.2147/cmar.S262450
He, Y., Yu, X., Li, J., Zhang, Q., Zheng, Q., and Guo, W. (2020b). Role of m(5)C-related regulatory genes in the diagnosis and prognosis of hepatocellular carcinoma. Am. J. Transl. Res. 12, 912–922.
He, Y., Zhang, Q., Zheng, Q., Yu, X., and Guo, W. (2020c). Distinct 5-methylcytosine profiles of circular RNA in human hepatocellular carcinoma. Am. J. Transl. Res. 12, 5719–5729.
Hernandez-Gea, V., Toffanin, S., Friedman, S. L., and Llovet, J. M. (2013). Role of the microenvironment in the pathogenesis and treatment of hepatocellular carcinoma. Gastroenterology 144, 512–527. doi: 10.1053/j.gastro.2013.01.002
Hong, L., Sun, G., Peng, L., Tu, Y., Wan, Z., Xiong, H., et al. (2018). The interaction between miR-148a and DNMT1 suppresses cell migration and invasion by reactivating tumor suppressor genes in pancreatic cancer. Oncol. Rep. 40, 2916–2925. doi: 10.3892/or.2018.6700
Li, A., Omura, N., Hong, S. M., and Goggins, M. (2010). Pancreatic cancer DNMT1 expression and sensitivity to DNMT1 inhibitors. Cancer Biol. Ther. 9, 321–329. doi: 10.4161/cbt.9.4.10750
Lin, S., Xu, H., Zhang, A., Ni, Y., Xu, Y., Meng, T., et al. (2020). Prognosis analysis and validation of m(6)A signature and tumor immune microenvironment in glioma. Front. Oncol. 10:541401. doi: 10.3389/fonc.2020.541401
Llovet, J. M., Kelley, R. K., Villanueva, A., Singal, A. G., Pikarsky, E., Roayaie, S., et al. (2021). Hepatocellular carcinoma. Nat. Rev. Dis. Primers 7:6. doi: 10.1038/s41572-020-00240-3
Llovet, J. M., Zucman-Rossi, J., Pikarsky, E., Sangro, B., Schwartz, M., Sherman, M., et al. (2016). Hepatocellular carcinoma. Nat. Rev. Dis. Primers 2:16018. doi: 10.1038/nrdp.2016.18
Mariathasan, S., Turley, S. J., Nickles, D., Castiglioni, A., Yuen, K., Wang, Y., et al. (2018). TGFβ attenuates tumour response to PD-L1 blockade by contributing to exclusion of T cells. Nature 554, 544–548. doi: 10.1038/nature25501
Mayakonda, A., Lin, D. C., Assenov, Y., Plass, C., and Koeffler, H. P. (2018). Maftools: efficient and comprehensive analysis of somatic variants in cancer. Genome Res. 28, 1747–1756. doi: 10.1101/gr.239244.118
Mei, L., Shen, C., Miao, R., Wang, J. Z., Cao, M. D., Zhang, Y. S., et al. (2020). RNA methyltransferase NSUN2 promotes gastric cancer cell proliferation by repressing p57(Kip2) by an m(5)C-dependent manner. Cell Death Dis. 11:270. doi: 10.1038/s41419-020-2487-z
Nombela, P., Miguel-López, B., and Blanco, S. (2021). The role of m6A, m5C and Ψ RNA modifications in cancer: novel therapeutic opportunities. Mol. Cancer 20:18. doi: 10.1186/s12943-020-01263-w
Peng, D. F., Kanai, Y., Sawada, M., Ushijima, S., Hiraoka, N., Kosuge, T., et al. (2005). Increased DNA methyltransferase 1 (DNMT1) protein expression in precancerous conditions and ductal carcinomas of the pancreas. Cancer Sci. 96, 403–408. doi: 10.1111/j.1349-7006.2005.00071.x
Piperi, C., and Papavassiliou, A. G. (2011). Strategies for DNA methylation analysis in developmental studies. Dev. Growth Differ. 53, 287–299. doi: 10.1111/j.1440-169X.2011.01253.x
Rosenberg, J. E., Hoffman-Censits, J., Powles, T., van der Heijden, M. S., Balar, A. V., Necchi, A., et al. (2016). Atezolizumab in patients with locally advanced and metastatic urothelial carcinoma who have progressed following treatment with platinum-based chemotherapy: a single-arm, multicentre, phase 2 trial. Lancet 387, 1909–1920. doi: 10.1016/s0140-6736(16)00561-4
Şenbabaoğlu, Y., Gejman, R. S., Winer, A. G., Liu, M., Van Allen, E. M., de Velasco, G., et al. (2016). Tumor immune microenvironment characterization in clear cell renal cell carcinoma identifies prognostic and immunotherapeutically relevant messenger RNA signatures. Genome Biol. 17:231. doi: 10.1186/s13059-016-1092-z
Shen, S., Yan, J., Zhang, Y., Dong, Z., Xing, J., and He, Y. (2021). N6-methyladenosine (m6A)-mediated messenger RNA signatures and the tumor immune microenvironment can predict the prognosis of hepatocellular carcinoma. Ann. Transl. Med. 9:59. doi: 10.21037/atm-20-7396
Sun, Z., Xue, S., Zhang, M., Xu, H., Hu, X., Chen, S., et al. (2020). Aberrant NSUN2-mediated m(5)C modification of H19 lncRNA is associated with poor differentiation of hepatocellular carcinoma. Oncogene 39, 6906–6919. doi: 10.1038/s41388-020-01475-w
Turley, S. J., Cremasco, V., and Astarita, J. L. (2015). Immunological hallmarks of stromal cells in the tumour microenvironment. Nat. Rev. Immunol. 15, 669–682. doi: 10.1038/nri3902
Wang, P., Chu, W., Zhang, X., Li, B., Wu, J., Qi, L., et al. (2018). Kindlin-2 interacts with and stabilizes DNMT1 to promote breast cancer development. Int. J. Biochem. Cell Biol. 105, 41–51. doi: 10.1016/j.biocel.2018.09.022
Wilkerson, M. D., and Hayes, D. N. (2010). Consensusclusterplus: a class discovery tool with confidence assessments and item tracking. Bioinformatics 26, 1572–1573. doi: 10.1093/bioinformatics/btq170
Xue, C., Zhao, Y., and Li, L. (2020a). Advances in RNA cytosine-5 methylation: detection, regulatory mechanisms, biological functions and links to cancer. Biomark Res. 8:43. doi: 10.1186/s40364-020-00225-0
Xue, M., Shi, Q., Zheng, L., Li, Q., Yang, L., and Zhang, Y. (2020b). Gene signatures of m5C regulators may predict prognoses of patients with head and neck squamous cell carcinoma. Am. J. Transl. Res. 12, 6841–6852.
Yang, Y., Hsu, P. J., Chen, Y. S., and Yang, Y. G. (2018). Dynamic transcriptomic m(6)A decoration: writers, erasers, readers and functions in RNA metabolism. Cell Res. 28, 616–624. doi: 10.1038/s41422-018-0040-8
Yi, L., Wu, G., Guo, L., Zou, X., and Huang, P. (2020). Comprehensive analysis of the PD-L1 and immune infiltrates of m(6)A RNA methylation regulators in head and neck squamous cell carcinoma. Mol. Ther. Nucleic Acids 21, 299–314. doi: 10.1016/j.omtn.2020.06.001
Zeng, D., Li, M., Zhou, R., Zhang, J., Sun, H., Shi, M., et al. (2019). Tumor microenvironment characterization in gastric cancer identifies prognostic and immunotherapeutically relevant gene signatures. Cancer Immunol. Res. 7, 737–750. doi: 10.1158/2326-6066.Cir-18-0436
Zhang, B., Wu, Q., Li, B., Wang, D., Wang, L., and Zhou, Y. L. (2020a). m(6)A regulator-mediated methylation modification patterns and tumor microenvironment infiltration characterization in gastric cancer. Mol. Cancer 19:53. doi: 10.1186/s12943-020-01170-0
Zhang, J. G., Zhou, H. M., Zhang, X., Mu, W., Hu, J. N., Liu, G. L., et al. (2020b). Hypoxic induction of vasculogenic mimicry in hepatocellular carcinoma: role of HIF-1 α. RhoA/ROCK and Rac1/PAK signaling. BMC Cancer 20:32. doi: 10.1186/s12885-019-6501-8
Zhang, Q., Zheng, Q., Yu, X., He, Y., and Guo, W. (2020c). Overview of distinct 5-methylcytosine profiles of messenger RNA in human hepatocellular carcinoma and paired adjacent non-tumor tissues. J. Transl. Med. 18:245. doi: 10.1186/s12967-020-02417-6
Keywords: HCC, DNMT1, m5C modification patterns, TME, prognosis
Citation: Gu X, Zhou H, Chu Q, Zheng Q, Wang J and Zhu H (2021) Uncovering the Association Between m5C Regulator-Mediated Methylation Modification Patterns and Tumour Microenvironment Infiltration Characteristics in Hepatocellular Carcinoma. Front. Cell Dev. Biol. 9:727935. doi: 10.3389/fcell.2021.727935
Received: 20 June 2021; Accepted: 24 August 2021;
Published: 13 September 2021.
Edited by:
Zhigang Ren, First Affiliated Hospital of Zhengzhou University, ChinaReviewed by:
Yuting He, First Affiliated Hospital of Zhengzhou University, ChinaCopyright © 2021 Gu, Zhou, Chu, Zheng, Wang and Zhu. This is an open-access article distributed under the terms of the Creative Commons Attribution License (CC BY). The use, distribution or reproduction in other forums is permitted, provided the original author(s) and the copyright owner(s) are credited and that the original publication in this journal is cited, in accordance with accepted academic practice. No use, distribution or reproduction is permitted which does not comply with these terms.
*Correspondence: Haihong Zhu, emh1aGg3MkB6anUuZWR1LmNu
†These authors have contributed equally to this work
Disclaimer: All claims expressed in this article are solely those of the authors and do not necessarily represent those of their affiliated organizations, or those of the publisher, the editors and the reviewers. Any product that may be evaluated in this article or claim that may be made by its manufacturer is not guaranteed or endorsed by the publisher.
Research integrity at Frontiers
Learn more about the work of our research integrity team to safeguard the quality of each article we publish.