- 1Department of Breast Surgery, Tongren Hospital, Shanghai Jiao Tong University School of Medicine, Shanghai, China
- 2Shanghai Key Laboratory of Tissue Engineering, Department of Plastic and Reconstructive Surgery, Shanghai 9th People’s Hospital, Shanghai Jiao Tong University School of Medicine, Shanghai, China
- 3Department of General Surgery, Jing’an District Center Hospital, Fudan University, Shanghai, China
Breast cancer (BC) is the most common cancer affecting women and the leading cause of cancer-related deaths worldwide. Compelling evidence indicates that microRNAs (miRNAs) are inextricably involved in the development of cancer. Here, we constructed a novel model, based on miRNA-seq and clinical data downloaded from The Cancer Genome Atlas (TCGA). Data from a total of 962 patients were included in this study, and the relationships among their clinicopathological features, survival, and miRNA-seq expression levels were analyzed. Hsa-miR-186 and hsa-miR-361 were identified as internal reference miRNAs and used to normalize miRNA expression data. A five-miRNA signature, constructed using univariate and multivariate Cox regression, was significantly associated with disease-specific survival (DSS) of patients with BC. Kaplan–Meier (KM) and receiver operating characteristic (ROC) analyses were conducted to confirm the clinical significance of the five-miRNA signature. Finally, a nomogram was constructed based on the five-miRNA signature to evaluate its clinical value. Cox regression analysis revealed that a five-miRNA signature was significantly associated with DSS of patients with BC. KM analysis demonstrated that the signature could efficiently distinguish high- and low-risk patients. Moreover, ROC analysis showed that the five-miRNA signature exhibited high sensitivity and specificity in predicting the prognosis of patients with BC. Patients in the high-risk subgroup who received adjuvant chemotherapy had a significantly lower incidence of mortality than those who did not. A nomogram constructed based on the five-miRNA signature was effective in predicting 5-year DSS. This study presents a novel five-miRNA signature as a reliable prognostic tool to predict DSS and provide theoretical reference significance for individualized clinical decisions for patients with BC.
Introduction
Globally, breast cancer (BC) is the leading cause of cancer mortality among women. It is estimated that 2.1 million new BC cases were diagnosed worldwide in 2018, accounting for almost one in four cancer cases (Bray et al., 2018). BC is clinically categorized into three types based on tumor hormonal status: luminal-like, human epidermal growth factor receptor 2 (HER2)-positive, and triple-negative BC. Luminal-like and HER2-positive BC are driven by the hormone receptors and HER2, while triple-negative BC does not express receptors for either hormones or human epidermal growth factor. The classification of BC by hormonal status has important therapeutic implications, as luminal-like cases may be treated using hormonal therapy, which is ineffective against HER2-positive and triple-negative breast cancer (Slamon et al., 1987; Fisher et al., 1989; Perou, 2011). Although multiple prognostic tools, including Oncotype Dx, MammaPrint, Theros, MapQuant Dx, and PAM50, have been developed for routine prognostic application in luminal-like BC, none is capable of predicting prognosis in all types of BC (Krop et al., 2017). While BC hormonal receptor status, HER2 status, tumor size, and the extent of lymph node metastases are independent prognostic indicators, these factors alone are inadequate guides for personalized BC treatment.
MicroRNAs (miRNAs) are small non-coding RNA molecules that regulate gene expression (Liu et al., 2014). Multiple studies have shown that miRNA function has a critical role in tumorigenesis, progression, and therapy (Rupaimoole and Slack, 2017). For instance, high expression levels of hsa-miR-21 are associated with specific BC clinical features, including advanced tumor stage, lymph node metastasis, and shortened patient survival (Yan et al., 2008). In addition, hsa-miR-210 and hsa-miR-221 have been linked with BC invasion and poorer prognosis of patients with BC (Volinia et al., 2012). Other miRNAs associated with prognosis in BC include hsa-miR-155, hsa-miR-206, hsa-miR-133b, hsa-miR-10b, let-7b, hsa-miR-30a, and hsa-miR-505 (Chen et al., 2012; Cheng et al., 2012; Ma et al., 2014; Parrella et al., 2014; Quan et al., 2018; Wang et al., 2019; Yao et al., 2019). However, many previous studies have been limited by small numbers of participants or a lack of extensive study scope, which has limited the translation of their findings into clinical application; for instance, the research results of the hsa-miR-30a only apply to triple-negative breast cancer (Cheng et al., 2012), and the study of the hsa-miR-10b has scarce representation in the population of cases classified as low risk by the Nottingham Prognostic Index (Parrella et al., 2014).
Recent research shows that multiple-miRNA signature (model) could predict the prognosis of some cancer; for instance, four-miRNA classifier is a reliable prognostic prediction tool for overall survival (OS) in lymph node-positive locoregional esophageal squamous cell carcinoma (Wen et al., 2021), a six-miRNA-based classifier is a reliable prognostic and predictive tool for disease recurrence in patients with stage II colon cancer (Zhang et al., 2013), and a robust six-miRNA prognostic signature (Zhao and Cui, 2020) and a novel seven-miRNA prognostic model are reliable to predict OS (Lu et al., 2019) for head and neck squamous cell carcinoma. It is worth mentioning that there were three multi-miRNA prognostic models for breast cancer. Lai et al. (2019) had constructed a novel six-microRNA-based model to predict OS, Tang et al. (2019) constructed a 17-miRNA-based model for OS and a 13-miRNA-based model for recurrence-free survival, and Lu et al. (2020) constructed a three-miRNA-based model for OS. However, all of the three multi-miRNA prognostic models for breast cancer lack a comprehensive analysis and do not identify the risk subgroup patients to tailor adjuvant chemotherapy.
The Cancer Genome Atlas (TCGA)1 project has applied extensive genomic sequencing and bioinformatics analyses to catalog human cancer-causing mutations in large cohorts, and the resulting datasets are publicly available (Tomczak et al., 2015). In this study, we took advantage of miRNA-seq and clinical datasets from TCGA for use in constructing a novel prognosis prediction model for disease-specific survival (DSS) in patients with BC.
Materials and Methods
MiRNA-Seq and Clinical Datasets
Level 3 miRNA-seq data and accompanying clinical datasets were obtained through TCGA Genomic Data Commons portal (GDC, see text footnote 1). Samples from 962 female patients with BC cataloged by TCGA, and for which clinical features and miRNA-seq data were available, were selected for further analyses. The BC dataset from TCGA used was last updated on June 13, 2018. The results reported here are completely based on data generated by TCGA Research Network.
Expression Data Normalization
Perl Critic software was used to merge miRNA-seq files and the Normalize Quantiles (edgeR, R package) was used to normalize miRNA expression levels. A total of 1,601 miRNAs were filtered out. Reference miRNA candidates were selected if they met all of the following inclusion criteria (Zhan et al., 2014):
(1) Mean (normal) > 100 and mean (tumor) > 100
(2) and
(3) and
Ultimately, hsa-miR-186 and hsa-miR-361 were chosen as internal reference molecules (Table 1) and used to normalize miRNA expression levels, based on the following formula:
The logarithm of the data is convenient for statistical analysis (Olivier et al., 2008; Sorrentino, 2010), which can reduce the absolute value of the data and facilitate calculation. Logarithm will not change the nature and correlation of the data but only compress the scale of the variables. Seventy-five differentially expressed miRNAs, including 14 up-regulated and 61 down-regulated, were screened out using edgeR (log2|fold change| > 1, adjusted P-value < 0.05).
Risk Prognostic Model Construction and Evaluation
The dataset (N = 962) was used to develop a clinical prediction model, and half of the dataset (N = 481) were randomly selected as a validation dataset, which was used for verification of the accuracy of the miRNA-based prognostic model as a predictor of DSS of patients with BC. Univariate and multivariate Cox proportional hazard models were constructed as risk prognostic models and area under the curve (AUC) analysis conducted to evaluate their accuracy.
Statistical Analyses
Statistical analyses were conducted using SPSS statistical suite version 25.0, ActivePerl version 5.26.3, and R version 3.6.1. Statistical significance was defined as a two-sided P-value or adjusted P-value < 0.05, except in univariate Cox proportional hazard analysis, where P < 0.1 was considered significant. The main outcome in this study was DSS, and the event time of DSS was defined according to the guidelines for time-to-event end point definitions in BC trials (Gourgou-Bourgade et al., 2015). The DSS event times for the individual patients enrolled in this retrospective study were manually retrieved from TCGA clinical records and a previous study (Thorsson et al., 2018). MiRNAs with prognostic value identified using univariate Cox proportional hazards models (P < 0.1) were further analyzed by multivariate Cox regression (default settings: backward, conditional, and entry 0.05; removal 0.10), and miRNAs identified by this analysis were used to construct a formula for calculation of prognostic risk scores. The results of univariate and multivariate Cox regression are presented as hazard ratio (HR) with 95% confidence interval (CI). Receiver operating characteristic (ROC) curve and distance on curve [DOC, equaling the square root of (1 − Sensitivity)2 + (1 − Specificity)2] were performed to calculate cut-off points. Next, BC cases were categorized as “low risk” or “high risk” based on risk score higher or lower than the cut-off point. Chi-square analysis was used to assess the correlation between BC clinicopathological features and risk subgroups. Kaplan–Meier (KM) analyses were applied to generate survival curves, and log rank test was used to establish the significance of differences between curves. A prognostic nomogram to predict individual survival based on an miRNA signature and clinical risk factors was constructed by Cox regression. The accuracy of the risk prognostic model was tested using AUC (95% CI) values.
Results
Identification of MiRNAs Associated With DSS in Patients With BC
A total of 1,601 miRNAs were filtered out, and 75 miRNAs were detected as differentially expressed using the edgeR package, including 14 up- and 61 down-regulated miRNAs (Figure 1A), and the expression levels of the 75 miRNAs were shown using the pheatmap package (Figure 1B). Further, univariate Cox proportional hazard analysis identified 23 miRNAs with prognostic value, five of which (hsa-miR-574, hsa-miR-30b, hsa-miR-224, hsa-miR-210, and hsa-miR-130a) were determined by multivariate Cox regression to be the optimum prognostic model for predicting DSS risk in patients with BC (Table 2). Risk scores were calculated using the formula:
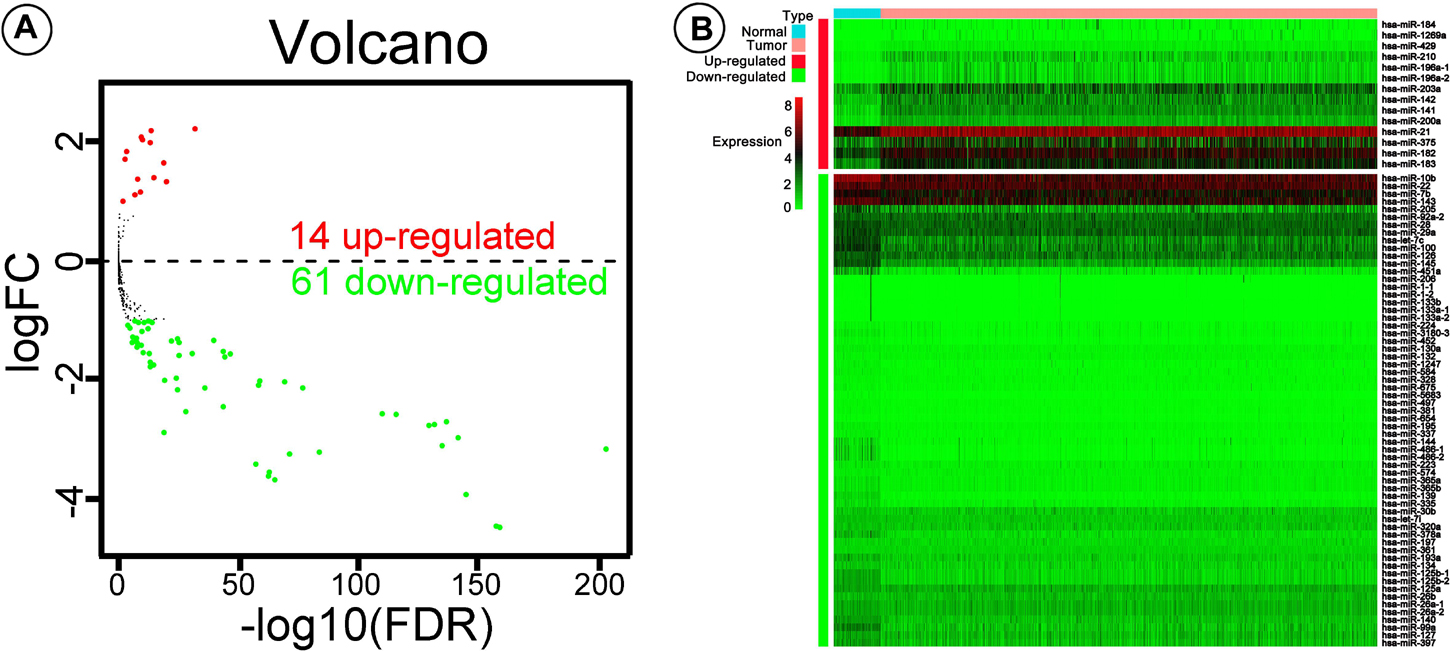
Figure 1. Volcano plot and pheatmap showing differentially expressed microRNAs (miRNAs). (A) Values plotted are mean normalized signal values (log10-scaled) for control (x-axis) and experimental (y-axis) groups. Red and green points correspond to twofold-change up/down, respectively (P < 0.05). (B) The pheatmap R package was used to plot expression levels of the differentially expressed miRNAs in breast cancer (BC) and adjacent tissue; red and green indicate that miRNA was expressed at high or low levels, respectively.
2.682 × hsa-miR-574 − 0.904 × hsa-miR-30b + 1.102 × hsa-miR-224 + 0.525 × hsa-miR-210 − 4.738 × hsa-miR-130a indicating that hsa-miRNA-574, hsa-miRNA-224, and hsa-miRNA-210 were associated with higher risk scores, whereas hsa-miRNA-30b and hsa-miRNA-130a were linked with lower risk scores. Expression levels of the five miRNAs are shown separately in Figure 2. The risk score, −0.197, was calculated as the cut-off point via ROC curve and DOC (DOC = 0.436) (Table 3). ROC curves for various risk score cut-off points are shown in Table 3. Using this model, patients were grouped into high-risk (N = 338) and low-risk (N = 624) groups, according to the cut-off point.
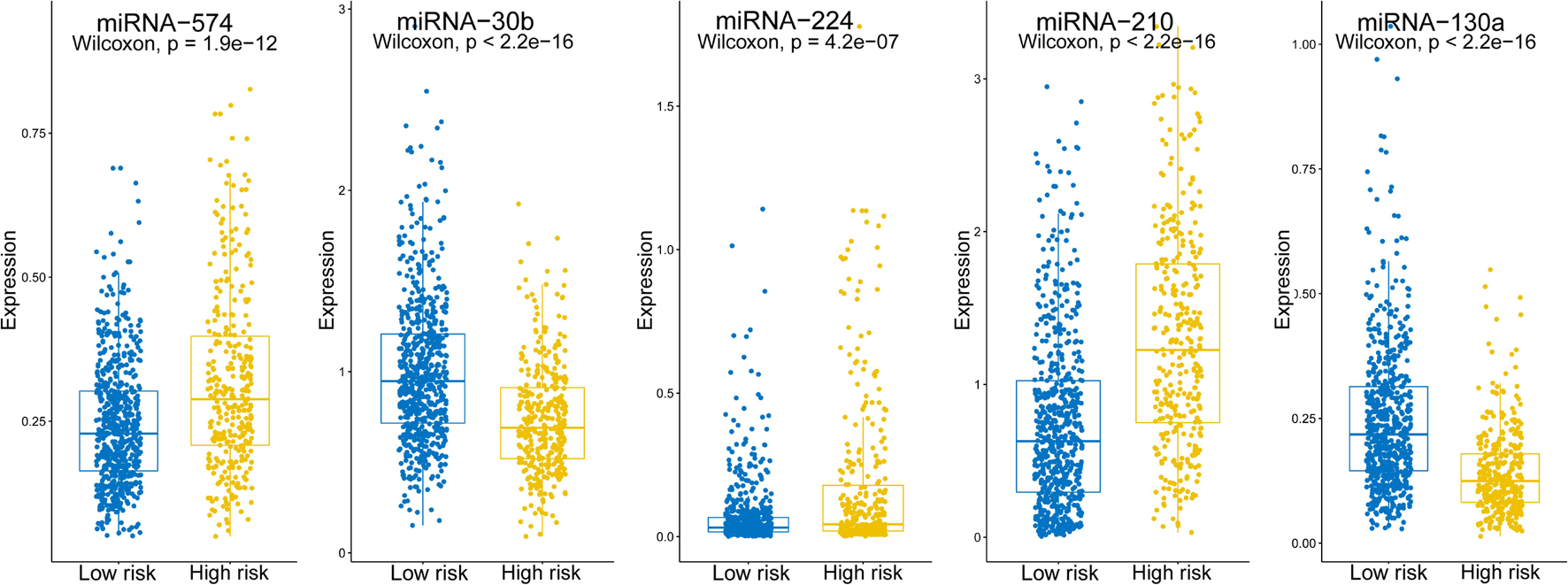
Figure 2. Expression levels of the five screened miRNAs. Boxplots show the expression levels of the five miRNAs in the high- and low-risk groups.
Clinicopathological Features
A total of 962 female cases with BC recorded in TCGA were extracted for analyses in this study. The median patient age was 58 years (range, 26–90 years), while the median DSS was 825 days. The 5-years DSS rate for all cases analyzed was 89.4%. BC tumor size, lymph node status, and metastasis status [tumor, node, metastasis (TNM) stage] was defined as outlined by the Eighth Edition American Joint Committee on Cancer (AJCC) Staging Manual (Amin et al., 2017), and molecular subtype (PAM50) was derived from a previous report by Thorsson et al. (2018). The proportion of HER2 subgroup patients in the low-risk group was significantly lower than that in the high-risk group (total dataset, χ2 = 92.295, P < 0.0001; validation dataset, χ2 = 45.400, P < 0.0001). Further, a larger tumor size was associated with a higher proportion of patients in the high-risk group for the total dataset (χ2 = 18.447, P < 0.0001), but not in the validation dataset (χ2 = 2.484, P = 0.478) (Table 4).
A Five-MiRNA Signature Associated With DSS of Patients With BC
Univariate and multivariate Cox proportional hazard regression analyses indicated that a higher five-miRNA risk score was correlated with higher incidences of clinical events (univariate analysis, HR 5.686, 95% CI: 3.065–10.550, P < 0.0001; multivariate analysis, HR 4.376, 95% CI 2.288–8.369, P < 0.0001) (Table 5). Moreover, KM survival curves showed that the high-risk group had worse prognosis in both the total (log rank P < 0.0001) and validation (log rank P < 0.0001) datasets (Figure 3).
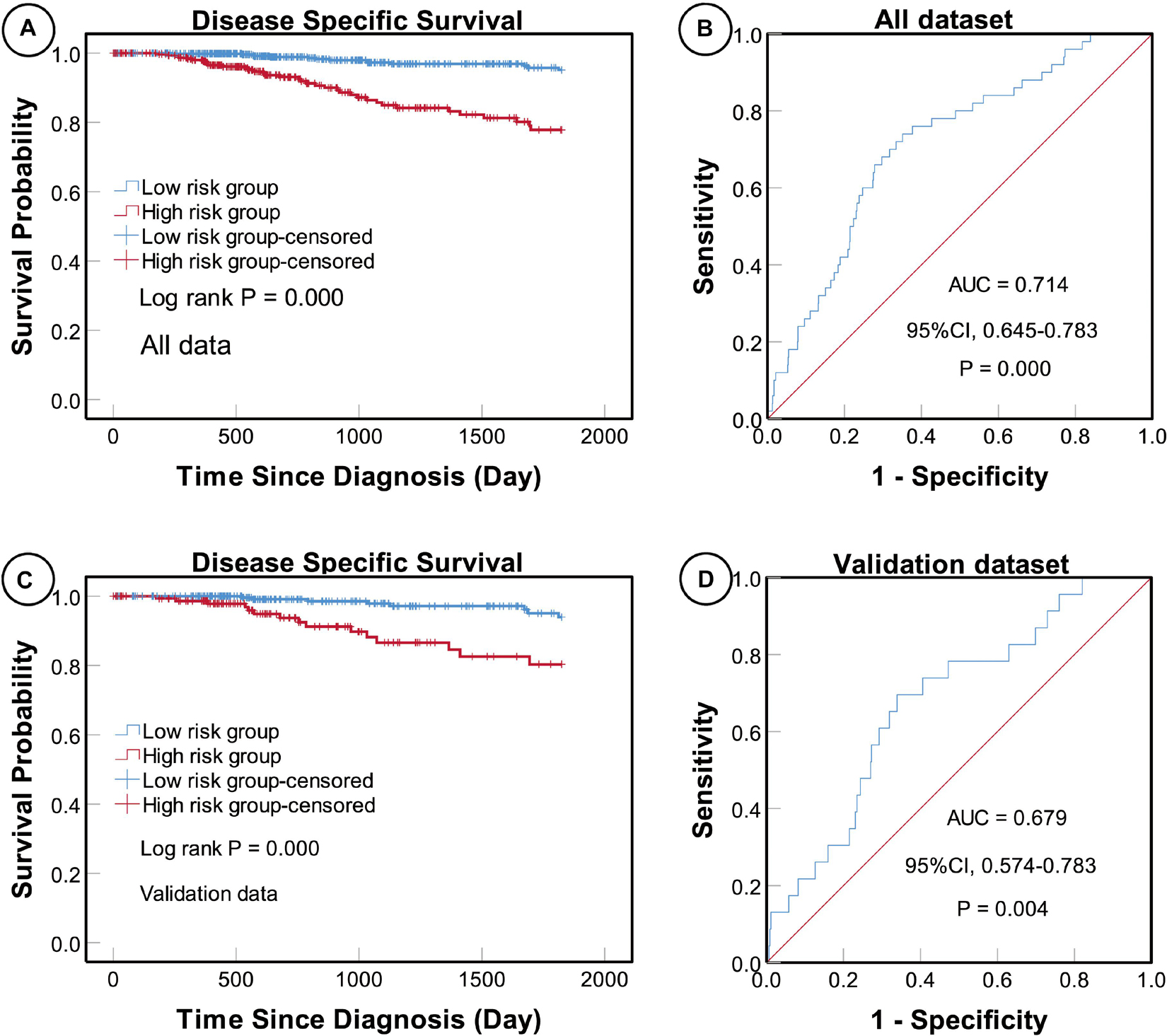
Figure 3. Kaplan–Meier (KM) and receiver operating characteristic (ROC) curves of disease-specific survival (DSS) according to the five-miRNA signature. KM curves of DSS for high- and low-risk groups in the total (A) and validation (C) datasets. The sensitivity and specificity of the five-miRNA signature for predicting DSS of patients in the total (B) and validation (D) datasets.
Evaluation of the Predictive Power of the Five-MiRNA Signature
To determine the sensitivity and specificity of the five-miRNA signature for predicting survival, we conducted ROC analyses of the total and validation datasets. The AUC value for the five-miRNA signature was 0.714 (95% CI 0.645–0.783, P < 0.0001) in the total dataset and 0.679 (95% CI 0.574–0.783, P = 0.004) in the validation dataset, respectively, suggesting that the five-miRNA signature was highly sensitive and specific (Figure 3).
According to the AJCC cancer staging manual (8th edition), TNM stage is correlated with cancer prognosis (Amin et al., 2017). Further, age and intrinsic molecular subtype (PAM50) are also closely linked to prognosis in patients with BC (Anders et al., 2009; Parker et al., 2009; Lænkholm et al., 2018). To validate the potential of the five-miRNA signature as a predictor of DSS of patients with BC, the entire TCGA BC dataset was stratified by cancer stage, age, and molecular subtype; BC cases were split into three age subgroups (≤ 40, 41–60, and ≥ 61 years old), four lymph node status subgroups (N0, N1, N2, and N3), four tumor size subgroups (T1, T2, T3, and T4), and five molecular subtype subgroups (LumA-like, LumB-like, HER2, basal-like, and normal-like). KM analysis indicated that patients in the low-risk group had significantly longer DSS in all three age subgroups (≤ 40 years, P = 0.008; 41–61 years P = 0.007; and ≥ 61 years, P < 0.0001). ROC curve analysis showed that the five-miRNA signature had good sensitivity and specificity for predicting survival in the 41–61 years (AUC = 0.652, 95% CI 0.532–0.771, P = 0.025) and the ≥ 61 years (AUC = 0.757, 95% CI 0.670–0.844, P < 0.0001) subgroups, but not in the ≤ 40 years (AUC = 0.714, 95% CI 0.490–0.937, P = 0.110); KM and ROC curves are presented in Figure 4. In analyses of lymph node, tumor size, molecular subtype, and metastasis status subgroups, KM curves also showed that patients in the low-risk group had significantly better prognosis than those in the high-risk group (N0 subgroup, KM analysis, P = 0.002; N1 subgroup, P < 0.001; N2 subgroup, P = 0.029; N3 subgroup, P = 0.013; T1 subgroup, P = 0.005; T2 subgroup, P < 0.001; T3 subgroup, P < 0.001; T4 subgroup, P < 0.506; LumA-like subgroup, P < 0.001; basal-like subgroup, P = 0.001; normal-like subgroup, P < 0.001; and M0 subgroup, P < 0.001), apart from the LumB-like and HER2 subgroups (LumB-like subgroup, P = 0.113; HER2 + subgroup, P = 0.067). ROC analysis demonstrated that the signature had good sensitivity and specificity for predicting DSS (N0 subgroup, AUC = 0.686, 95% CI 0.562–0.816, P = 0.020; N1 subgroup, AUC = 0.704, 95% CI 0.576–0.832, P = 0.002; N2 subgroup, AUC = 0.752, 95% CI 0.629–0.875, P = 0.013; N3 subgroup, AUC = 0.806, 95% CI 0.663–0.950, P = 0.009; T1 subgroup, AUC = 0.744, 95% CI 0.608–0.880, P = 0.009; T2 subgroup, AUC = 0.714, 95% CI 0.614–0.814, P = 0.001; T3 subgroup, AUC = 0.813, 95% CI 0.685–0.940, P = 0.003; LumA-like subgroup, AUC = 0.716, 95% CI 0.553–0.878, P = 0.008; basal-like subgroup, AUC = 0.666, 95% CI 0.553–0.779, P = 0.026; normal-like subgroup, AUC = 0.817, 95% CI 0.693–0.941, P = 0.001; and M0 subgroup, AUC = 0.733, 95% CI 0.661–0.805, P < 0.001), but not in the T4, LumB-like, and HER2 subgroups (T4 subgroup, AUC = 0.583, 95% CI 0.358–0.809, P = 0.472; LumB-like subgroup, AUC = 0.670, 95% CI 0.574–0.765, P = 0.197; HER2 + subgroup, AUC = 0.279, 95% CI 0.000–0.654, P = 0.140); KM and ROC curves are presented in Figures 5–7, and the results are summarized in Table 6. Overall, these analyses indicate that the five-miRNA signature has a good predictive value.
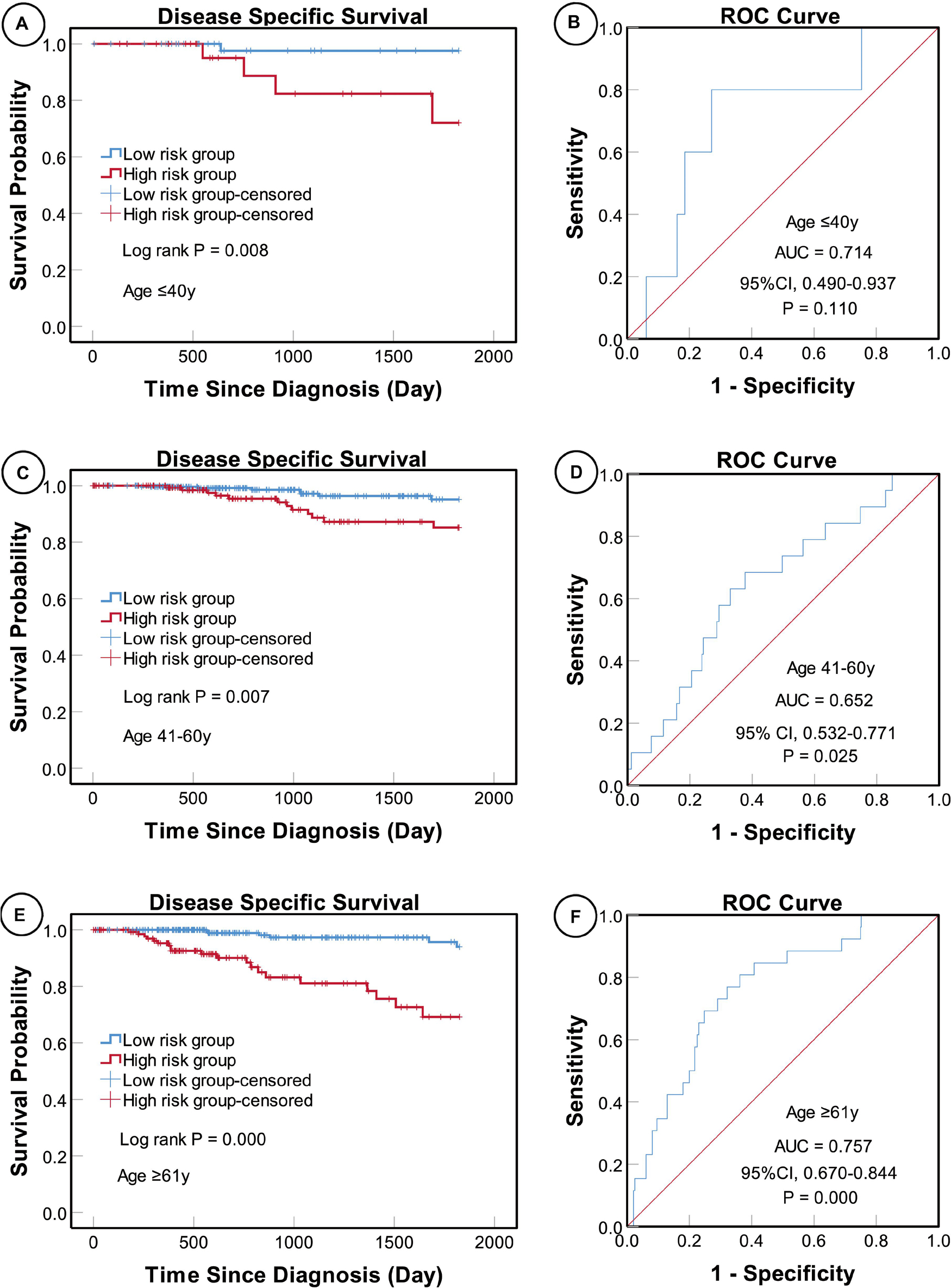
Figure 4. KM and ROC curve analyses of patients stratified by age. KM analysis showed that the patients in low-risk group had significantly better DSS in the ≤ 40 years (A), 41–61 years (C), and ≥ 61 years (E) subgroups. ROC analysis showed that the five-miRNA signature exhibited high sensitivity and specificity in predicting the prognosis of patients with BC in the ≤ 40 years (B), 41–61 years (D), and ≥ 61 years (F) subgroups.
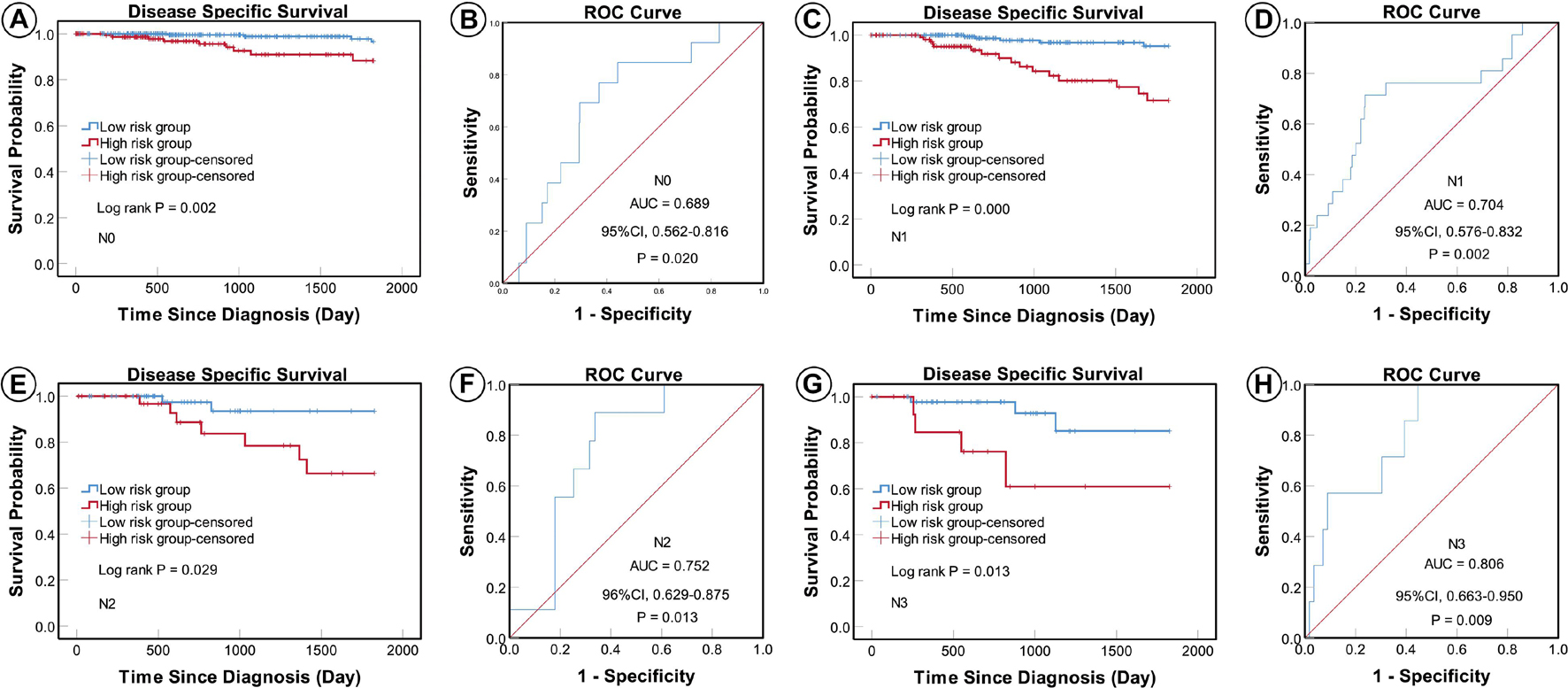
Figure 5. KM and ROC curves for patients stratified by lymph node status. KM analysis showed that patients in the low-risk group had significantly better DSS in the N0 (A), N1 (C), N2 (E), and N3 (G) subgroups. ROC analysis showed that the five-miRNA signature exhibited high sensitivity and specificity in predicting the prognosis of patients with BC in the N0 (B), N1 (D), N2 (F), and N3 (H) subgroups.
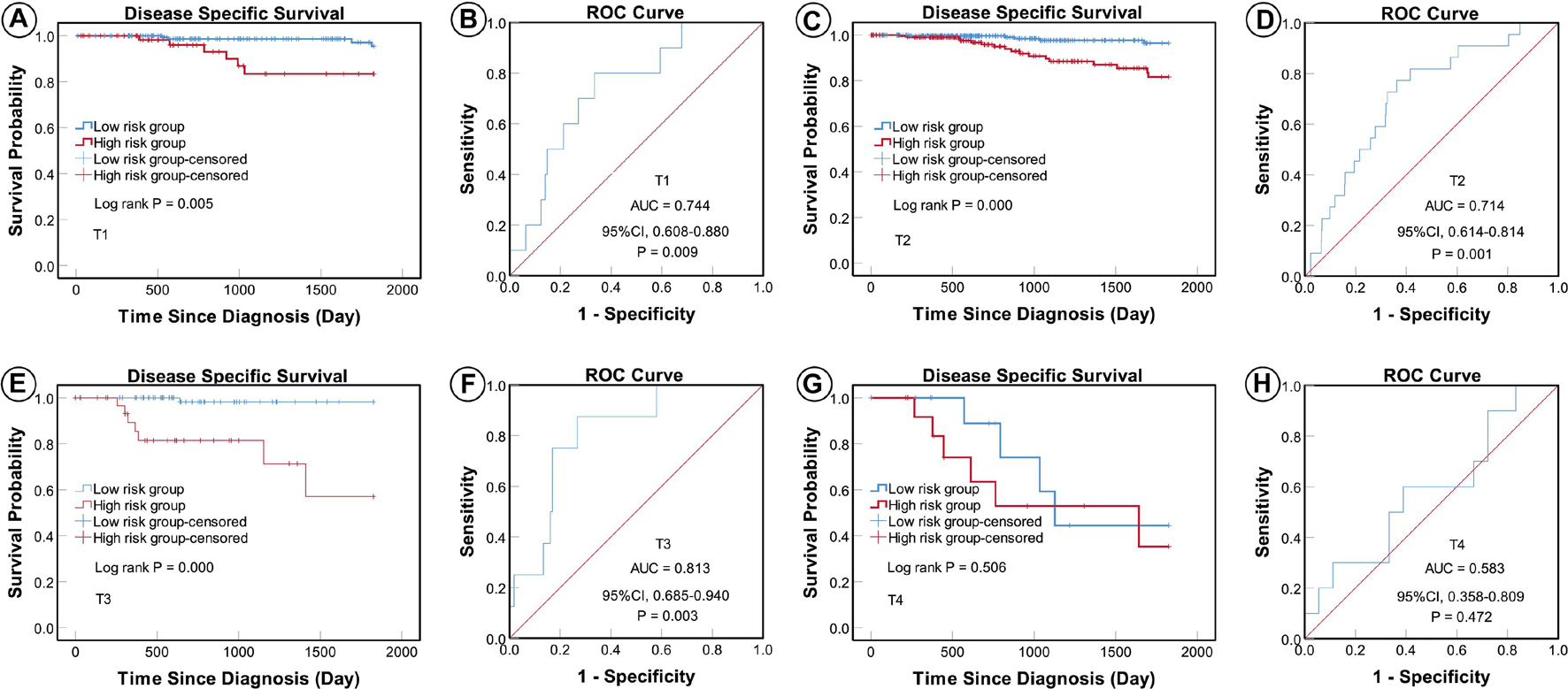
Figure 6. KM and ROC curves for patients stratified by tumor size. KM analysis showed that the patients in the low-risk group had significantly better DSS in the T1 (A), T2 (C), and T3 (E) subgroups, but not in the T4 (G) subgroup. ROC analysis showed that the five-miRNA signature exhibited high sensitivity and specificity in predicting the prognosis of patients with BC in the T1 (B), T2 (D), and T3 (F) subgroups, but not in the T4 (H) subgroup.
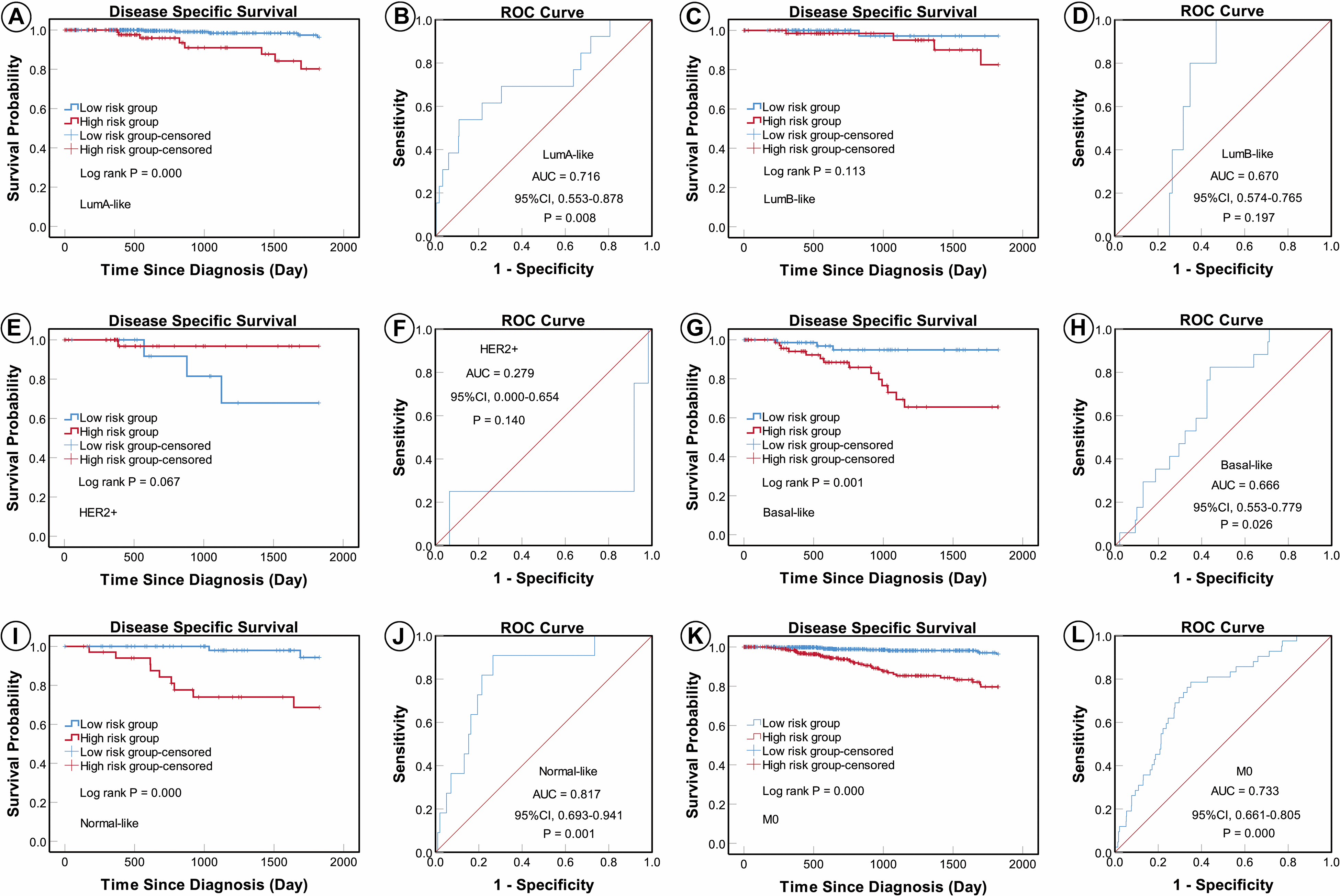
Figure 7. KM and ROC curves for patients stratified by molecular subtype and metastasis status. KM analysis showed that patients in the low-risk group had significantly better DSS in the LumA-like (A), basal-like (G), normal-like (I), and M0 (K) subgroups, but not in the LumB-like (C) or HER2 (E) subgroups. ROC analysis showed that the five-miRNA signature exhibited high sensitivity and specificity in predicting the prognosis of patients with BC in the LumA-like (B), basal-like (H), normal-like (J), and M0 (L) subgroups, but not the LumB-like (D) or HER2 (F) subgroups.
Relevance of the MiRNA Signature in Clinical Decision-Making
We found that patients in the high-risk group who underwent adjuvant chemotherapy had significantly better prognosis than those who did not (P = 0.004), while no such difference was detected in the low-risk group (P = 0.466) (Figure 8). These results suggest that patients in the high-risk group could benefit from adjuvant chemotherapy, while those in the low-risk group may not.
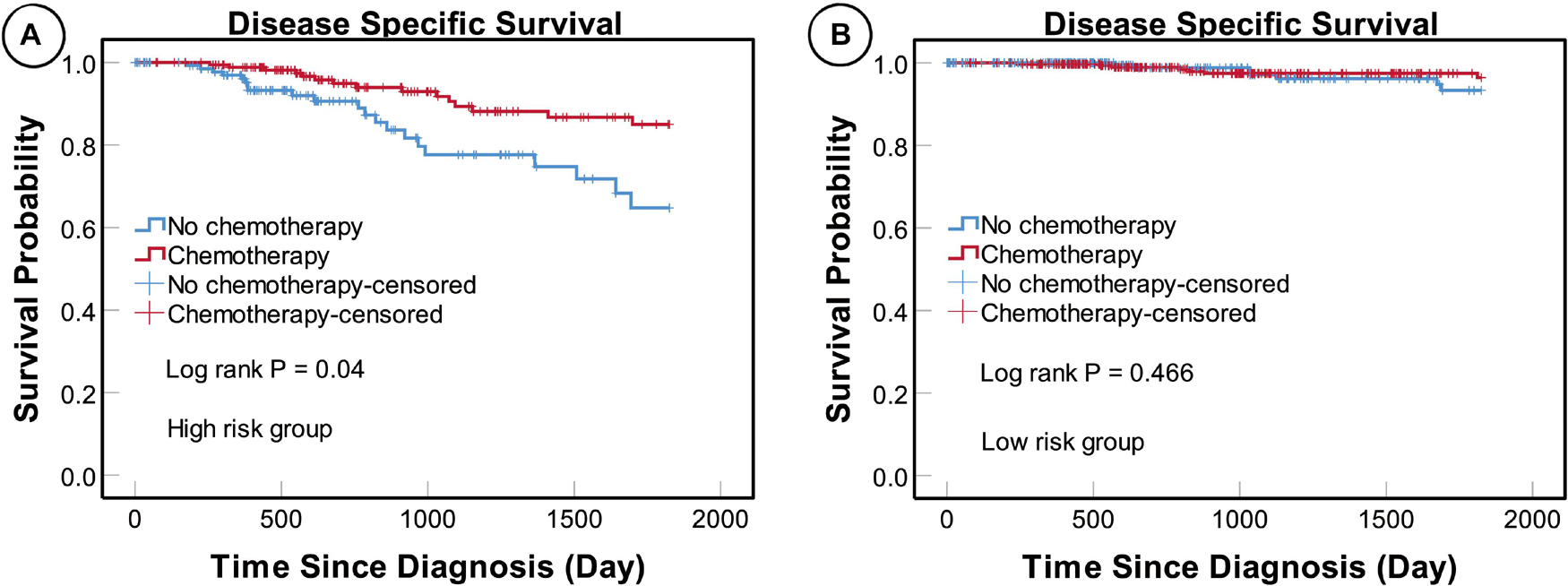
Figure 8. KM curves for patients in different risk subgroups. KM analysis showing that patients in the high-risk (A) but not the low-risk (B) subgroup who underwent adjuvant chemotherapy had significantly better prognosis than those who did not receive adjuvant chemotherapy.
Nomogram Development
To apply the five-miRNA signature in clinical settings, we combined it with conventional clinical predictors of prognosis (age, tumor size, lymph node, metastasis, and molecular subtype) to create a nomogram (Figure 9). Each risk factor corresponds to a designated point determined by drawing a line perpendicular to the points axis. The corresponding sum of risk factor points located on the total points represents the probability of 5-year DSS by reading straight down to the 5-year DSS axis.
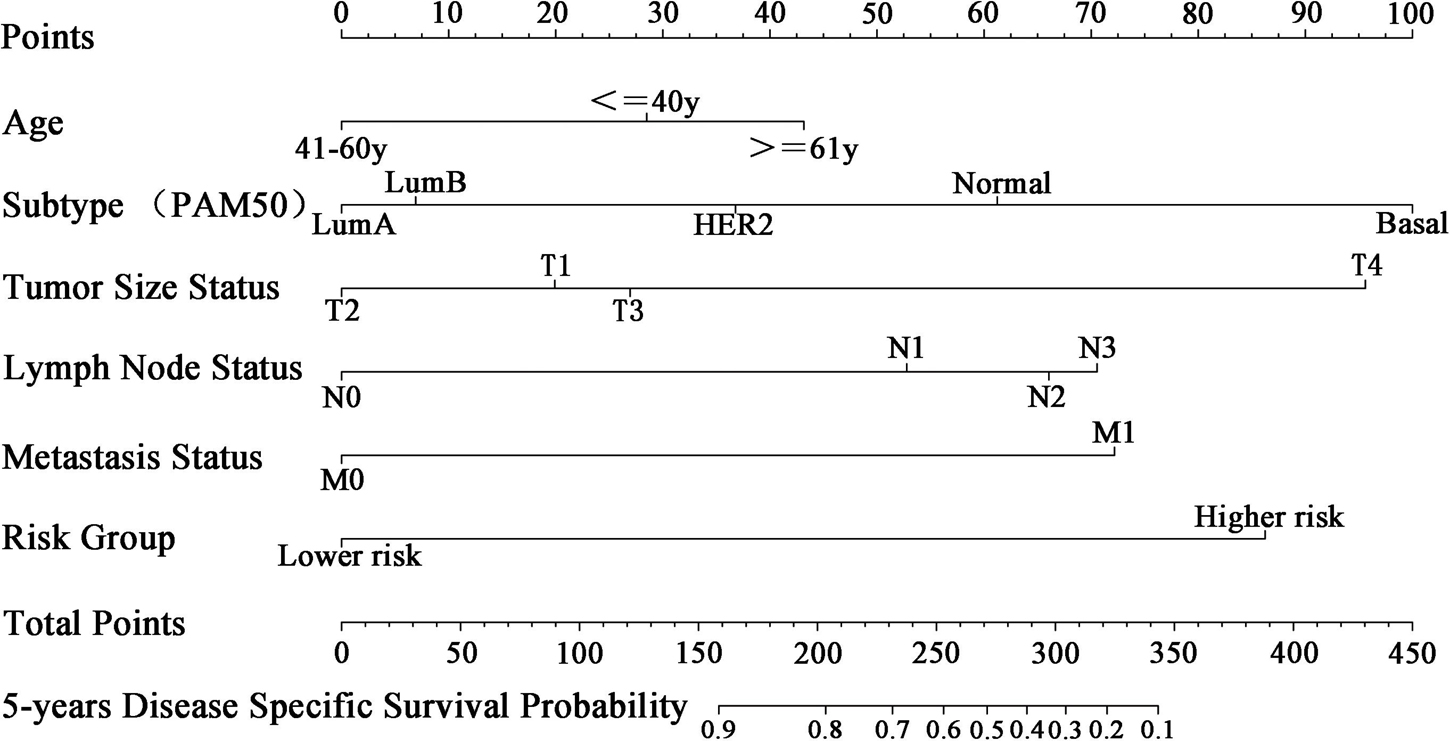
Figure 9. Nomogram for predicting 5-year DSS of patients with BC. Each clinical predictive factor corresponds to a designated point shown by a line drawn perpendicular to the point’s axis. The corresponding sum of risk factor points, shown as total points, can be used to estimate the probability of 5-year DSS by drawing a line straight down to the 5-year DSS axis.
Discussion
Although, numerous molecular predictors of prognosis have been developed to guide clinical decisions in the management of patients with BC, the scope for application of predictors has been somewhat limited; for example, Oncotype Dx was developed for prediction of prognosis only in cases with ER-positive, HER2-negative, node-negative, and early stage BC (Paik et al., 2004). Numerous studies have demonstrated that miRNAs have potentially important functions in breast tumorigenesis, and multiple miRNAs have been identified as candidate biomarkers for prediction of prognosis in patients with BC (Yan et al., 2008; Chen et al., 2012; Cheng et al., 2012; Zhou et al., 2014). The results of multigene analyses demonstrate that combinations of multiple biomarkers can achieve higher sensitivity and specificity, relative to single-gene biomarkers (Paik et al., 2004; Cardoso et al., 2016).
In the present study, a five-miRNA signature was identified as significantly associated with DSS. Further analyses demonstrated the utility of this five-miRNA signature as a powerful predictor of prognosis in patients with BC. A nomogram constructed by combining the five-miRNA signature and conventional prognostic factors had high value for prediction of 5-year DSS of patients with BC. In the high-risk group, patients who underwent adjuvant chemotherapy had better prognosis than those who did not, but this was not the case in the low-risk group, indicating that patients in the high-risk group could benefit from adjuvant chemotherapy more than those in the low-risk group.
The biological functions of the five miRNAs have been elucidated in numerous experiments, and they are associated with BC prognosis or pathological features. Hsa-miR-30b has been validated as a tumor suppressor that inhibits bone metastasis in BC (Croset et al., 2018). Up-regulation of hsa-miR-210 promotes BC stem cell metastasis, proliferation, and self-renewal by targeting E-cadherin (Tang et al., 2018). Further, hsa-miR-224 promotes tumorigenesis through down-regulation of caspase-9 in triple-negative BC (Zhang et al., 2019), hsa-miR-574 enhances doxorubicin resistance by down-regulating SMAD4 in BC cells (Sun et al., 2018), and hsa-miR-130a suppresses BC cell migration and invasion (Chen et al., 2018; Kong et al., 2018) and reduces drug resistance in BC (Huang et al., 2019).
Recent research shows that multiple-miRNA signature (model) could predict the prognosis of some cancer, for instance, four-miRNA classifier for esophageal squamous cell carcinoma (Wen et al., 2021), a six-miRNA-based classifier for stage II colon cancer (Zhang et al., 2013), a robust six-miRNA prognostic signature (Zhao and Cui, 2020), and a novel seven-miRNA prognostic model (Lu et al., 2019) for head and neck squamous cell carcinoma. In breast cancer, three multi-miRNA prognostic models have been developed to date (Lai et al., 2019; Tang et al., 2019; Lu et al., 2020). However, our model has several advantages as a predictor of prognosis in patients with BC. First, our study included 962 female cases with BC, while excluding males and cases with missing clinical information, which avoided the possibility of sex-specific effects and ensured more credible results. Second, DSS was chosen as a clinical outcome, rather than OS, as OS is less sensitive for BC-specific mortality. Third, we identified two internal reference miRNAs (hsa-miR-186 and hsa-miR-361), which were essential to normalize expression data for other miRNAs. Quantification methods for miRNA expression levels include relative and absolute expression quantification. Although absolute quantification could detect exact miRNA expression, small changes in an experiment may cause huge relative quantification differences. The purpose of relative quantification is the degree of change in the expression level of the target miRNA relative to the expression level of the reference miRNA, and its function is to correct aspects of operation differences to ensure the accuracy of the experimental results. Also, hsa-miR-186 and hsa-miR-361 were screened by following strict criteria that have been reported by Zhan et al. (2014), they had certain and stable expression levels in tumor and normal tissue, and there was no statistical difference between tumor and normal tissue. So, relative quantification appears to be more robust than absolute quantification approaches. Fourth, patients with high-risk scores using our model were shown to benefit from adjuvant chemotherapy, which could inform clinical decision-making regarding appropriate treatment strategies for patients with BC.
Notwithstanding these strengths, the study had a number of shortcomings and limitations, which should be acknowledged. First, a real-world validation dataset was lacking. Our breast center has already started to establish a validation dataset of BC to verify the findings in this research. However, limited by the follow-up time and the number of cases, it may take a long time. If the validation dataset is consistent with the results of this study, the finding will be applied to a prospective clinical study. Second, the biological functions of the five miRNAs remain to be fully elucidated. Third, KM curve or ROC subgroup analyses did not reveal any significant difference in the subgroups: T4 (N = 28), LumB-like (N = 164), HER2 (N = 67), age ≤ 40 years (N = 87), and M1 (N = 14); therefore, further study to identify more accurate molecular models for these patient subgroups is warranted. Fourth, while our analyses show that patients with high-risk scores could benefit from adjuvant chemotherapy, which indicates that the five-miRNA signature has theoretical reference significance for individualized clinical decision-making, more clinical studies are necessary to confirm this observation. Fifth, our study lacks an independent validation dataset. In the early stage of study design, we had considered that the full dataset be randomly divided into training and validation datasets by the proportions of 1:1, 3:2, 7:3, and 4:1, but the larger the sample size of the dataset, the higher the credibility of the model established, absolutely selecting full dataset for training and modeling. Although the verification dataset was randomly selected from the total dataset with the overlap of the sample points, it can also verify the reliability of the model.
Conclusion
In conclusion, we identified a novel five-miRNA prognostic model significantly associated with DSS in patients with BC and developed a nomogram based on the five-miRNA signature with high prognostic prediction value. Moreover, our analyses indicated that patients with high-risk scores using our model could benefit from adjuvant chemotherapy, indicating that the five-miRNA signature has theoretical reference significance for individualized clinical decision-making.
Data Availability Statement
All datasets generated and analyzed in this study are available from the corresponding author on reasonable request. TCGA datasets analyzed in this study are available at https://cancergenome.nih.gov/ (TCGA repository).
Ethics Statement
Ethical review and approval was not required for the study on human participants in accordance with the local legislation and institutional requirements. Written informed consent for participation was not required for this study in accordance with the national legislation and the institutional requirements.
Author Contributions
BT and MH collected, analyzed, interpreted TCGA data, and participated in writing the manuscript. KZ critically revised the manuscript for intellectual content. XQ, YD, and YG controlled the quality of tables and figures. JW and XY designed this study and reviewed the manuscript. All authors contributed to the article and approved the submitted version.
Funding
This study was supported by the Shanghai Jing’an District Science and Technology Committee and the Shanghai Jing’an District Municipal Health Commission (Grant No. 2020MS03).
Conflict of Interest
The authors declare that the research was conducted in the absence of any commercial or financial relationships that could be construed as a potential conflict of interest.
Publisher’s Note
All claims expressed in this article are solely those of the authors and do not necessarily represent those of their affiliated organizations, or those of the publisher, the editors and the reviewers. Any product that may be evaluated in this article, or claim that may be made by its manufacturer, is not guaranteed or endorsed by the publisher.
Acknowledgments
This study was performed using data from the Cancer Genome Atlas Research Network: https://cancergenome.nih.gov/.
Abbreviations
AJCC, American Joint Committee on Cancer; AUC, area under the curve; BC, breast cancer; CI, confidence intervals; DOC, distance on curve; DSS, disease-specific survival; GDC, Genomic Data Commons; HER2, human epidermal growth factor receptor 2; HR, hazard ratio; KM, Kaplan–Meier; miRNAs, microRNAs; OS, overall survival; ROC, receiver operating characteristic; TCGA, The Cancer Genome Atlas; TNM, tumor size, lymph node status, and metastasis status.
Footnotes
References
Amin, M. B., Greene, F. L., Edge, S. B., Compton, C. C., Gershenwald, J. E., Brookland, R. K., et al. (2017). AJCC Cancer Staging Manual, 8th Edn. New York, NY: Springer.
Anders, C. K., Johnson, R., Litton, J., Phillips, M., and Bleyer, A. (2009). Breast cancer before age 40 years. Sem. Oncol. 36, 237–249. doi: 10.1053/j.seminoncol.2009.03.001
Bray, F., Ferlay, J., Soerjomataram, I., Siegel, R. L., Torre, L. A., and Jemal, A. (2018). Global cancer statistics 2018: GLOBOCAN estimates of incidence and mortality worldwide for 36 cancers in 185 countries. CA Cancer J. Clin. 68, 394–424. doi: 10.3322/caac.21492
Cardoso, F., van’t Veer, L. J., Bogaerts, J., Slaets, L., Viale, G., Delaloge, S., et al. (2016). 70-Gene signature as an aid to treatment decisions in early-stage breast cancer. New Engl. J. Med. 375, 717–729. doi: 10.1056/NEJMoa1602253
Chen, J., Wang, B. C., and Tang, J. H. (2012). Clinical significance of microRNA-155 expression in human breast cancer. J. Surg. Oncol. 106, 260–266. doi: 10.1002/jso.22153
Chen, X., Zhao, M., Huang, J., Li, Y., Wang, S., Harrington, C. A., et al. (2018). microRNA-130a suppresses breast cancer cell migration and invasion by targeting FOSL1 and upregulating ZO-1. J. Cell. Biochem. 119, 4945–4956. doi: 10.1002/jcb.26739
Cheng, C. W., Wang, H. W., Chang, C. W., Chu, H. W., Chen, C. Y., Yu, J. C., et al. (2012). MicroRNA-30a inhibits cell migration and invasion by downregulating vimentin expression and is a potential prognostic marker in breast cancer. Breast Cancer Res. Treat. 134, 1081–1093. doi: 10.1007/s10549-012-2034-4
Croset, M., Pantano, F. A.-O., Kan, C. W. S., Bonnelye, E., Descotes, F., Alix-Panabières, C., et al. (2018). miRNA-30 family members inhibit breast cancer invasion, osteomimicry, and bone destruction by directly targeting multiple bone metastasis-associated genes. Cancer Res. 78, 5259–5273.
Fisher, B., Costantino, J., Redmond, C., Poisson, R., Bowman, D., Couture, J., et al. (1989). A randomized clinical trial evaluating tamoxifen in the treatment of patients with node-negative breast cancer who have estrogen-receptor-positive tumors. N. Engl. J. Med. 320, 479–484.
Gourgou-Bourgade, S., Cameron, D., Poortmans, P., Asselain, B., Azria, D., Cardoso, F., et al. (2015). Guidelines for time-to-event end point definitions in breast cancer trials: results of the DATECAN initiative (Definition for the Assessment of Time-to-event Endpoints in CANcer trials). Ann. Oncol. 26, 2505–2506. doi: 10.1093/annonc/mdv478
Huang, J., Zhao, M., Hu, H., Wang, J., Ang, L., and Zheng, L. (2019). MicroRNA-130a reduces drug resistance in breast cancer. Int. J. Clin. Exp. Pathol. 12, 2699–2705.
Kong, X., Zhang, J., Li, J., Shao, J., and Fang, L. (2018). MiR-130a-3p inhibits migration and invasion by regulating RAB5B in human breast cancer stem cell-like cells. Biochem. Biophys. Res. Commun. 501, 486–493. doi: 10.1016/j.bbrc.2018.05.018
Krop, I., Ismaila, N., Andre, F., Bast, R. C., Barlow, W., Collyar, D. E., et al. (2017). Use of biomarkers to guide decisions on adjuvant systemic therapy for women with early-stage invasive breast cancer: american society of clinical oncology clinical practice guideline focused update. J. Clin. Oncol. 35, 2838–2847. doi: 10.1200/JCO.2017.74.0472
Lai, J., Wang, H., Pan, Z., and Su, F. (2019). A novel six-microRNA-based model to improve prognosis prediction of breast cancer. Aging (Albany NY) 11, 649–662. doi: 10.18632/aging.101767
Liu, B., Li, J., and Cairns, M. J. (2014). Identifying miRNAs, targets and functions. Brief. Bioinformatics 15, 1–19. doi: 10.1093/bib/bbs075
Lu, D. C., Han, W., and Lu, K. (2020). Identification of key microRNAs involved in tumorigenesis and prognostic microRNAs in breast cancer. Mathem. Biosci. Eng. MBE 17, 2923–2935. doi: 10.3934/mbe.2020164
Lu, L., Wu, Y., Feng, M., Xue, X., and Fan, Y. (2019). A novel seven-miRNA prognostic model to predict overall survival in head and neck squamous cell carcinoma patients. Mol. Med. Rep. 20, 4340–4348. doi: 10.3892/mmr.2019.10665
Lænkholm, A.-V., Jensen, M.-B., Eriksen, J. O., Rasmussen, B. B., Knoop, A. S., Buckingham, W., et al. (2018). PAM50 risk of recurrence score predicts 10-Year distant recurrence in a comprehensive danish cohort of postmenopausal women allocated to 5 years of endocrine therapy for hormone receptor–positive early breast cancer. J. Clin. Oncol. 36, 735–740. doi: 10.1200/JCO.2017.74.6586
Ma, L., Li, G. Z., Wu, Z. S., and Meng, G. (2014). Prognostic significance of let-7b expression in breast cancer and correlation to its target gene of BSG expression. Med. Oncol. 31:773. doi: 10.1007/s12032-013-0773-7
Olivier, J., Johnson, W. D., and Marshall, G. D. (2008). The logarithmic transformation and the geometric mean in reporting experimental IgE results: what are they and when and why to use them? Ann. Allergy Asthma Immunol. 100, 333–337. doi: 10.1016/S1081-1206(10)60595-9
Paik, S., Shak, S., Tang, G., Kim, C., Baker, J., Cronin, M., et al. (2004). A multigene assay to predict recurrence of tamoxifen-treated, node-negative breast cancer. New England J. Med. 351, 2817–2826. doi: 10.1056/NEJMoa041588
Parker, J. S., Mullins, M., Cheang, M. C. U., Leung, S., Voduc, D., Vickery, T., et al. (2009). Supervised risk predictor of breast cancer based on intrinsic subtypes. J. Clin. Oncol. 27, 1160–1167. doi: 10.1200/JCO.2008.18.1370
Parrella, P., Barbano, R., Pasculli, B., Fontana, A., Copetti, M., Valori, V. M., et al. (2014). Evaluation of microRNA-10b prognostic significance in a prospective cohort of breast cancer patients. Mol. Cancer 13:142. doi: 10.1186/1476-4598-13-142
Perou, C. M. (2011). Molecular stratification of triple-negative breast cancers. Oncologist 16, 61–70. doi: 10.1634/theoncologist.2011-S1-61
Quan, Y., Huang, X., and Quan, X. (2018). Expression of miRNA-206 and miRNA-145 in breast cancer and correlation with prognosis. Oncol. Lett. 16, 6638–6642. doi: 10.3892/ol.2018.9440
Rupaimoole, R., and Slack, F. J. (2017). MicroRNA therapeutics: towards a new era for the management of cancer and other diseases. Nat. Rev. Drug Discov. 16, 203–222. doi: 10.1038/nrd.2016.246
Slamon, D. J., Clark, G. M., Wong, S. G., Levin, W. J., Ullrich, A., and McGuire, W. L. (1987). Human breast cancer: correlation of relapse and survival with amplification of the HER-2/neu oncogene. Science 235:177.
Sorrentino, R. P. (2010). Large standard deviations and logarithmic-normality: the truth about hemocyte counts in Drosophila. Fly 4, 327–332. doi: 10.4161/fly.4.4.13260
Sun, F. D., Wang, P. C., Luan, R. L., Zou, S. H., and Du, X. (2018). MicroRNA-574 enhances doxorubicin resistance through down-regulating SMAD4 in breast cancer cells. Eur. Rev. Med. Pharmacol. Sci. 22, 1342–1350. doi: 10.26355/eurrev_201803_14476
Tang, J., Ma, W., Zeng, Q., Tan, J., Cao, K., and Luo, L. (2019). Identification of miRNA-Based signature as a novel potential prognostic biomarker in patients with breast cancer. Dis. Markers 2019, 3815952–3815952. doi: 10.1155/2019/3815952
Tang, T., Yang, Z., Zhu, Q., Wu, Y., Sun, K., Alahdal, M., et al. (2018). Up-regulation of miR-210 induced by a hypoxic microenvironment promotes breast cancer stem cells metastasis, proliferation, and self-renewal by targeting E-cadherin. FASEB J. doi: 10.1096/fj.201801013R Online ahead of print.
Thorsson, V., Gibbs, D. L., Brown, S. D., Wolf, D., Bortone, D. S., Ou Yang, T.-H., et al. (2018). The immune landscape of cancer. Immunity 48, 812–830.e14. doi: 10.1016/j.immuni.2018.03.023
Tomczak, K., Czerwińska, P., and Wiznerowicz, M. (2015). The cancer genome atlas (TCGA): an immeasurable source of knowledge. Contemporary Oncol. (Poznan, Poland) 19, A68–A77. doi: 10.5114/wo.2014.47136
Volinia, S., Galasso, M., Sana, M. E., Wise, T. F., Palatini, J., Huebner, K., et al. (2012). Breast cancer signatures for invasiveness and prognosis defined by deep sequencing of microRNA. Proc. Natl. Acad. Sci. U S A. 109, 3024–3029. doi: 10.1073/pnas.1200010109
Wang, J., Liu, H., and Li, M. (2019). Downregulation of miR-505 promotes cell proliferation, migration and invasion, and predicts poor prognosis in breast cancer. Oncol. Lett. 18, 247–254. doi: 10.3892/ol.2019.10334
Wen, J., Wang, G., Xie, X., Lin, G., Yang, H., Luo, K., et al. (2021). Prognostic value of a Four-miRNA signature in patients with lymph node positive locoregional esophageal squamous cell carcinoma undergoing complete surgical resection. Ann. Surg. 273, 523–531. doi: 10.1097/SLA.0000000000003369
Yan, L.-X., Huang, X.-F., Shao, Q., Huang, M.-Y., Deng, L., Wu, Q.-L., et al. (2008). MicroRNA miR-21 overexpression in human breast cancer is associated with advanced clinical stage, lymph node metastasis and patient poor prognosis. RNA (New York, N.Y.) 14, 2348–2360. doi: 10.1261/rna.1034808
Yao, Y., Liu, R., Gao, C., Zhang, T., Qi, L., Liu, G., et al. (2019). Identification of prognostic biomarkers for breast cancer based on miRNA and mRNA co-expression network. J. Cell. Biochem. 120, 15378–15388. doi: 10.1002/jcb.28805
Zhan, C., Yan, L., Wang, L., Jiang, W., Zhang, Y., Xi, J., et al. (2014). Identification of reference miRNAs in human tumors by TCGA miRNA-seq data. Biochem. Biophys. Res. Commun. 453, 375–378. doi: 10.1016/j.bbrc.2014.09.086
Zhang, J.-X., Song, W., Chen, Z.-H., Wei, J.-H., Liao, Y.-J., Lei, J., et al. (2013). Prognostic and predictive value of a microRNA signature in stage II colon cancer: a microRNA expression analysis. Lancet Oncol. 14, 1295–1306. doi: 10.1016/s1470-2045(13)70491-1
Zhang, L., Zhang, X., Wang, X., He, M., and Qiao, S. (2019). MicroRNA-224 promotes tumorigenesis through downregulation of Caspase-9 in triple-negative breast cancer. Dis. Markers 2019:7378967. doi: 10.1155/2019/7378967
Zhao, X., and Cui, L. (2020). A robust six-miRNA prognostic signature for head and neck squamous cell carcinoma. J. Cell. Physiol. 235, 8799–8811. doi: 10.1002/jcp.29723
Keywords: breast cancer, miRNA, prognostic model, biomarker, signature, TCGA
Citation: Tian B, Hou M, Zhou K, Qiu X, Du Y, Gu Y, Yin X and Wang J (2021) A Novel TCGA-Validated, MiRNA-Based Signature for Prediction of Breast Cancer Prognosis and Survival. Front. Cell Dev. Biol. 9:717462. doi: 10.3389/fcell.2021.717462
Received: 31 May 2021; Accepted: 19 August 2021;
Published: 13 September 2021.
Edited by:
Benjamin Kidder, Wayne State University, United StatesReviewed by:
Gianvito Urgese, Politecnico di Torino, ItalyKristina Andrijauskaite, The University of Texas Health Science Center at San Antonio, United States
Copyright © 2021 Tian, Hou, Zhou, Qiu, Du, Gu, Yin and Wang. This is an open-access article distributed under the terms of the Creative Commons Attribution License (CC BY). The use, distribution or reproduction in other forums is permitted, provided the original author(s) and the copyright owner(s) are credited and that the original publication in this journal is cited, in accordance with accepted academic practice. No use, distribution or reproduction is permitted which does not comply with these terms.
*Correspondence: Jie Wang, wangjie2655@139.com
†These authors have contributed equally to this work and share first authorship