- State Key Laboratory of Cancer Biology, Xijing Hospital of Digestive Diseases, The Fourth Military Medical University, Xi’an, China
Hepatocellular carcinoma (HCC), a highly aggressive tumor, has high incidence and mortality rates. Recently, immunotherapies have been shown to be a promising treatment in HCC. The results of either the CheckMate-040 or IMbrave 150 trials demonstrate the importance of immunotherapy in the systemic treatment of liver cancer. Thus, in this study, we tried to establish a reliable prognostic model for liver cancer based on immune-related genes (IRGs) and to provide a new insight for immunotherapy of HCC. In this study, we used four datasets that incorporated 851 HCC samples, including 340 samples with complete clinical information from the cancer genome atlas (TCGA) database, to establish an effective model for predicting the prognosis of HCC patients based on the differential expression of IRGs and validated the prognostic model using the data from International Cancer Genome Consortium (ICGC). The top 6 characteristic IRGs identified by protein-protein interaction (PPI) network analysis, MMP9, FOS, CAT, ESR1, ANGPTL3, and KLKB1, were selected for further study. In addition, we assessed the correlations of the six characteristic IRGs with the tumor immune microenvironment, clinical stage, and sensitivity to anti-cancer drugs. We also explored whether the differential expression of the characteristic IRGs was specific to HCC or present in pan-cancer. The expression levels of the six characteristic IRGs were significantly different between most tumor tissues and adjacent normal tissues. In addition, these characteristic IRGs showed a strong association with immune cell infiltration in HCC patients. We found that MMP9 and ESR1 were independent prognostic factors for HCC, while CAT, ESR1, and KLKB1 were associated with the clinical stage. We collected HCC paraffin sections from 24 patients from Xijing hospital to identify the differential expression of the five genes (MMP9, ESR1, CAT, FOS, and KLKB1). Finally, the results of decision curve analysis (DCA) and nomogram revealed that our models provided a prognostic benefit for most HCC patients and the predicted overall survival (OS) was consistent with the actual OS. In conclusion, we systemically constructed a novel prognostic model that provides new insights into HCC.
Introduction
Hepatocellular carcinoma (HCC), a common primary malignant tumor, accounts for almost 85% of all liver cancers (Sung et al., 2021). The worldwide incidence and mortality rates of HCC rank sixth and third, and in 2020 approximately 906,000 new cases of HCC and 830,000 related deaths were estimated to occur (Sung et al., 2021). The incidence and mortality rates of HCC depend on the patient’s race, region, age, sex, and risk factors related to tumor progression (Kulik and El-Serag, 2019; Sung et al., 2021). Current treatment options of HCC such as surgery, chemoradiotherapy, liver transplantation, and radiofrequency ablation will benefit a very small percentage of patients, and a median overall survival (OS) of untreated HCC patients was <9 months (Giannini et al., 2015; Wang et al., 2018). With the development of immunotherapy, the OS of patients with unresectable HCC were prolonged. Previous studies found that the prognosis of patients with HCC is associated with their immune microenvironment and clinical pathological features (Ayuso et al., 2018; Zhang et al., 2019). Notably, there is no effective prognostic model for HCC that systematically assesses immune-related genes (IRGs) together with clinicopathological features.
Application of immunotherapy regimens in patients with HCC has led to encouraging results in terms of both safety and efficacy, and immunological mechanisms have been demonstrated to be playing a key role in the epigenetic mechanism, pathogenesis and development of HCC (Keenan et al., 2019; Jayant et al., 2020). Recently, a multicenter, randomized and phase III study which is called IMbrave 150 evaluated combined atezolizumab and bevacizumab treatment vs. sorafenib in patients with unresectable HCC, met its coprimary endpoint and both progression free survival (PFS) and OS were improved (Finn et al., 2020). However, the HCC tumor microenvironment (TME), which includes antigen-presenting cells (APCs), myeloid-derived suppressive cells (MDSCs), and neutrophils, is complex, thereby resulting in immunotherapy resistance in HCC patients. Due to the complexity and immune suppression mechanisms of the liver immune microenvironment, immune checkpoint inhibitors (ICPI) treatment has limited benefits for a small number of patients with HCC. A high-throughput study of patient tissue samples revealed that 25% of the samples exhibited a transcriptomic hallmark of proinflammatory responses associated with adaptive or exhausted immunity (Sia et al., 2017). The immune response is improved by immunotherapies targeting coinhibitory receptors such as PD-1 and CTLA4 which inhibit the immunosuppressive mechanisms in several tumors (Wolchok, 2015; Chikuma, 2017). However, a substantial amount of work is need to make immunotherapies successful for patients with HCC. In addition, the clinical relevance and expression profiles of IRGs in HCC have not been explored systematically.
Previous studies have explored the immunotherapeutic effects on most cancers, and the relationship between immune cell infiltration and HCC prognosis has been the focus of a few studies (Zhou et al., 2019). The expression of 7 IRGs was detected in 374 HCC samples. Nevertheless, the conclusions are not very reliable due to a lack of prognostic model correction and to the inclusion of few clinical variables (Wang et al., 2020). Studies have shown that vascular normalization and antitumor immune responses are promoted by the inhibition of PD-1 in HCC (Garris et al., 2018; Sharpe and Pauken, 2018). However, these studies included small sample sizes, and the correlation between HCC patient prognosis and immunotherapy needs to be further assessed. Past studies have paid little attention to the sensitivities of IRGs to anti-cancer drugs and a systematic analysis of the associations of IRGs with the prognosis of HCC patients is urgently needed.
In this research, we utilized a multigene expression cohort of 851 HCC samples to develop and validate an individualized IRG set based on HCC prognostic signatures. The correlations of the characteristic IRGs with immune infiltrating cells, clinical stage, clinical prognosis, and drug sensitivity were analyzed, with the aim of providing a sufficient amount of data to improve the prognosis and immunotherapeutic responses of HCC patients. In addition, we performed a comprehensive analysis that incorporated clinical characteristics to identify potential prognostic biomarkers and molecular targets for HCC.
Materials and Methods
Ethics Statement
Twenty-four paraffin sections of HCC tissue which were used to perform immunohistochemistry (IHC) were obtained at the Xijing Hospital. This study was approved by the Xijing Hospital’s Ethics Committee and was conducted in accordance with the ethical standards as laid down in the 1964 Declaration of Helsinki and its later amendments.
Data Source and Preprocessing
The RNA-sequencing data and expression levels of microRNAs (miRNAs) in HCC patient samples were downloaded from The Cancer Genome Atlas (TCGA),1 and the dataset included 340 HCC samples that included clinical information. The fragments per kilobase million (FPKM) values were transformed to transcripts per kilobase million (TPM) values for further study. The clinical features of the patients, such as their clinical stage, sex, and age, were obtained from the UCSC Xena website.2 The gene expression data for different tissues and cell lines were downloaded from the TCGA and Cancer Cell Line Encyclopedia (CCLE) databases.
Hepatocellular carcinoma gene chip data (GSE14520, GSE101685, and GSE36376) were downloaded from Gene Expression Omnibus (GEO) datasets, and all data were from Homo sapiens (Roessler et al., 2010). GSE14520 was based on the GPL571 (HG-U133A_2) Affymetrix Human Genome U133A 2.0 Array and the GPL3921 (HT_HG-U133A) Affymetrix HT Human Genome U133A Array platform and contained information on 247 tumor samples and 241 normal liver samples. GSE101685 was based on the GPL570 (HG-U133_Plus_2) Affymetrix Human Genome U133 Plus 2.0 Array platform and contained information on 24 HCC samples and 8 normal liver samples. GSE36376 was based on the GPL10558 Illumina HumanHT-12 V4.0 expression beadchip platform and included information on 240 HCC samples and 193 normal liver samples. Tumor-associated IRGs were downloaded from the ImmPort database.3 All data used in this study were freely available online.
Identification of Immune-Related Genes in Hepatocellular Carcinoma
Principal component analysis (PCA) was conducted to assess the fundamental differences between HCC tissues and adjacent normal tissues (Luo et al., 2014). The analysis of differentially expressed genes (DEGs) was performed using the “DESeq2” package, with a corrected P < 0.05 and a | Log fold change(FC)| ≥ 1.0 serving as the thresholds (Love et al., 2014). The differential analysis results are presented as heatmaps and volcano plots. The heatmaps were produced by using the pheatmap package and the volcano plots were generated with R software. The Venn diagram web-tool was adopted to identify the common DEGs, and IRGs were associated with HCC among the common DEGs and tumor-related IRGs from the ImmPort database were identified.
Enrichment Analysis of Immune-Related Genes
Both Gene Ontology (GO) enrichment and Kyoto Encyclopedia of Genes and Genomes (KEGG) pathway analyses were performed by using the “clusterprofiler” package (Yu et al., 2012). GO terms were identified with a strict cutoff of p < 0.01 and a false discovery rate (FDR) of <0.05. KEGG signaling pathways were visualized in the form of a network map drawn with Cytoscape 3.7.2 software (Shannon et al., 2003).
The potential functions of IRGs were explored by gene set enrichment analysis (GSEA) with the R package ‘‘clusterprofiler.’’ We downloaded the gene set ‘‘c2.cp.kegg.v6.2.symbols’’ from the Molecular Signatures Database (MSigDB)4 for the GSEA. Gene set variation analysis (GSVA) was conducted by using the gene set “msigdb.v7.0.symbols,” from the MSigDB database (Hänzelmann et al., 2013). Differences with p < 0.05, were statistically significant.
Identification of Characteristic Immune-Related Genes
Search Tool for the Retrieval of Interacting Genes/Proteins (STRING),5 a free online tool, was used to identify and predict interactions between proteins or genes. A PPI network of IRGs was constructed with a cutoff standard of a combined score >0.4 (Szklarczyk et al., 2019). The PPI network map of the IRGs was visualized with Cytoscape software. CytoHubba, a plug-in of Cytoscape, was used to explore essential nodes in the network. The six characteristic IRGs with the best scores were identified by the maximal clique centrality (MCC) method and selected for further study (Chin et al., 2014).
The miRNA-mRNA interaction data were downloaded from miRTarBase,6 miRDB,7 and TargetScan,8 and the three miRNA databases were then integrated by using NetworkAnalyst.9 Subsequently, a regulatory network was constructed via correlation analysis of the mRNA-miRNAs (Lewis et al., 2003, 2005; Chen and Wang, 2020; Huang et al., 2020). The construction of the network was performed by Cytoscape (v3.7.2) to validate the reliability of the characteristic IRGs at the mRNA expression level.
Correlations of the Expression Levels of the Characteristic Immune-Related Genes With Anti-cancer Drug Sensitivity and Clinical Stage
The CellMiner database10 which is a web resource allows the rapid retrieval of data related to the impact of 20,503 chemical compounds, including 102 drugs, on 22,379 gene transcripts and 360 miRNA (Reinhold et al., 2012). Gene expression data and half maximal growth inhibition (GI50) values for different anti-cancer drugs were downloaded to analyze the correlations between the characteristic IRGs and anti-cancer drug sensitivity. Moreover, we grouped the patients into four groups according to the clinical stage, namely stage I, stage II, stage III, and stage IV; to compare the differences in the expression levels of the characteristic IRGs among the groups.
Correlations of the Characteristic Immune-Related Genes With the Infiltration of Immune Cells and Immune Checkpoint Genes in Hepatocellular Carcinoma Patients
TIMER, a comprehensive resource, that includes 10,897 samples across 39 cancer types, was used to systematically analyze of immune infiltrates (Li et al., 2016, 2017).11 We explored the correlations of the expression levels of the characteristic IRGs with the abundance of infiltrating immune cells via gene modules. The left-most panel shows the gene expression levels according to tumor purity. We also used the GEPIA website (Gene Expression Profiling Interactive Analysis)12 to analyze the correlation of the two prognostic IRGs (MMP9 and ESR1) with the immune checkpoint genes (Tang et al., 2017).
Construction and Validation of the Clinical Prognostic Model
We aimed to construct a prognostic HCC model based on the characteristic IRGs together with clinicopathological features. Cox regression analysis was adopted to determine the risk score of patients in terms of OS. A nomogram for survival prediction was then constructed with the TCGA cohort. Harrell’s concordance index (C-index) was measured to quantify the differentiation performance of the constructed nomogram. A calibration curve was used to evaluate the performance of the nomogram, and to compare the predicted values of the nomogram with the observed actual survival rates. Decision curve analysis (DCA) was performed to evaluate the clinical efficacy of the prognostic model (Van Calster et al., 2018). And the data which included 229 HCC samples from the International Cancer Genome Consortium (ICGC) database were used for the external validation of prognostic models.
Immunohistochemistry
Human liver tissues were obtained from the Xijing Hospital and the tumor sections were incubated with commercial rabbit polyclonal antibodies against MMP9 (1:400 dilution, Servicebio Biotechnology), ESR1 (1:1,000 dilution, Servicebio Biotechnology), KLKB1 (1:50 dilution, Abcam Biotechnology), CAT (1:800 dilution, Abcam Biotechnology), and FOS (1:400 dilution, Servicebio Biotechnology) overnight at 4°C. Then, the sections were treated with an appropriated secondary conjugated to horseradish peroxidase (HRP) at room temperature for 1 h, and the bound antibodies were visualized with 3, 3′-diaminobenzidine (DAB). We used a scoring method for the semi-quantitative analysis of IHC images. The tissue sections were scored by degree of staining and range of positivity by three individuals under a light microscope, and were repeated three times independently, the mean values were taken, and the final scores were summed and compared.
Statistical Analysis
The log-rank test was used for Kaplan-Meier survival analysis, to assess the differences in survival. The survival ROC package and pROC package were applied to construct the receiver operating characteristic (ROC) curve and to calculate the area under the curve (AUC) (Robin et al., 2011; Rizvi et al., 2019). To calculate the correlation coefficients among characteristic IRGs, Pearson correlation analysis was performed. Cox regression analysis was used to identify the independent prognostic factors. The p-value threshold for inclusion of multivariate cox regression analysis was 0.2 (Kang et al., 2013). The chi-square test was used to compare and analyze the significance of differences between the two sets of categorical variables. To compare two sets of continuous variables, independent Student’s t-tests were conducted, and the differences between the non-normally distributed variables were analyzed by the Wilcoxon rank sum test. In this study, R v4.0.2 was used for all statistical analyses (*p < 0.05; **p < 0.01; ***p < 0.001).
Results
Identification of 20 Immune-Related Genes in Hepatocellular Carcinoma Patients
The flow chart for this study is included in the Supplementary Figure 1. To analyze the key genes associated with HCC, we first performed PCA of the four datasets, revealing significant differences between HCC tissues and normal tissues (Supplementary Figure 2).
Subsequently, we analyzed the DEGs in HCC samples from the four datasets separately. In the TCGA HCC dataset, 3,328 genes were significantly upregulated and 1,241 genes were significantly downregulated (Figures 1A,B); in the GSE14520 dataset, 274 genes were significantly upregulated, and 488 genes were significantly downregulated (Figures 1C,D); in the GSE101685 dataset, 538 genes were significantly upregulated, and 843 genes were significantly downregulated (Figures 1E,F); and in the GSE36376 dataset, 89 genes were significantly upregulated and 360 genes were significantly downregulated (Figures 1G,H).
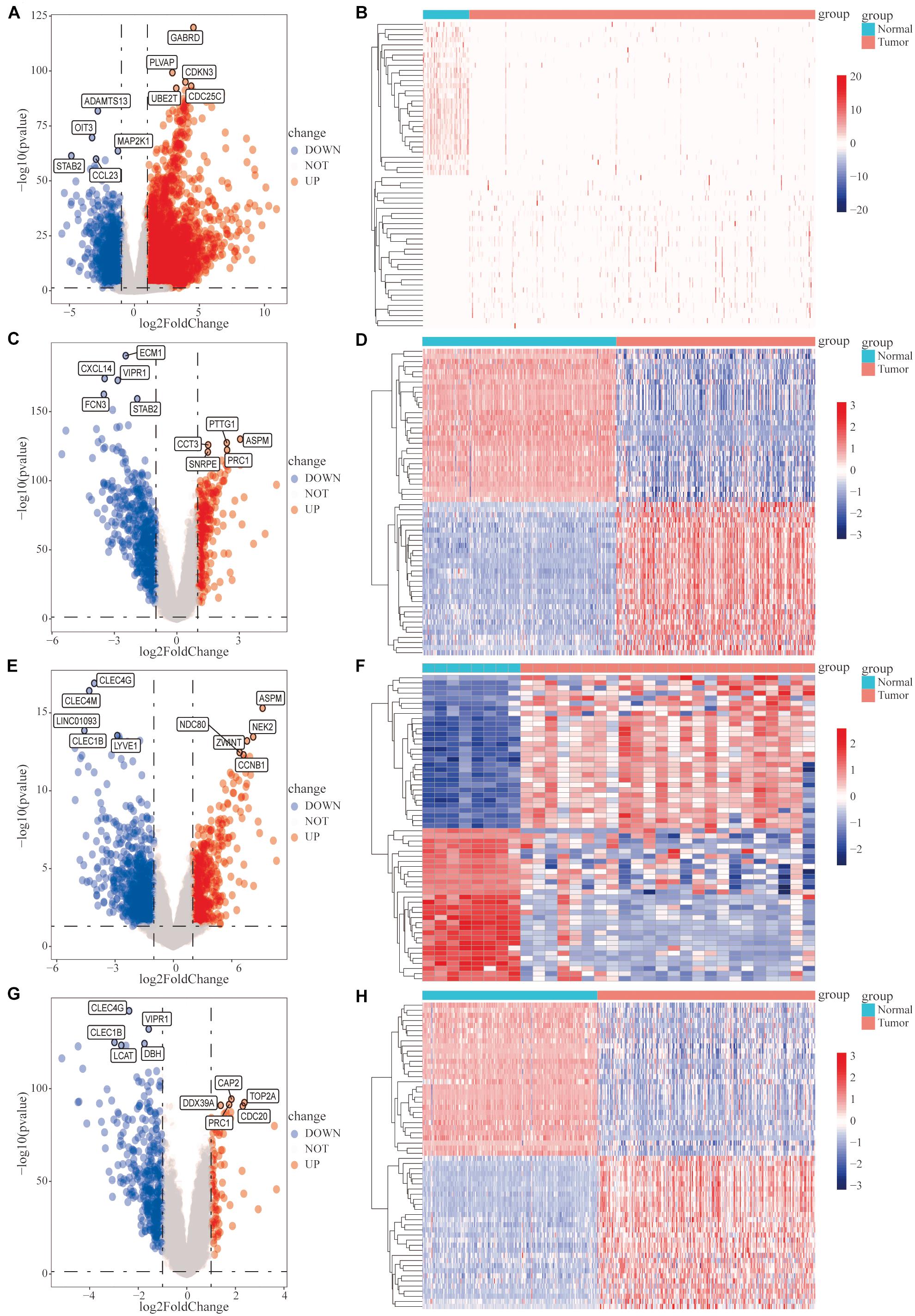
Figure 1. Analysis of differentially expressed genes (DEGs) between hepatocellular carcinoma (HCC) tumor tissues and adjacent normal tissues. Differential expression analysis was performed, based on the gene expression data for HCC patients, and the analysis results are presented as volcano plots and heat maps. (A,B) The cancer genome atlas (TCGA)-HCC dataset, (C,D) GSE14520 dataset, (E,F) GSE101685 dataset, and (G,H) GSE36376 dataset.
The Venn diagram revealed 206 common DEGs among the four datasets (Supplementary Figure 3A). In total, we downloaded 1,811 tumor-associated IRGs from the ImmPort database. A Venn diagram revealed 20 differentially expressed IRGs among the 206 DEGs (Supplementary Figure 3B).
Functional Enrichment Analysis of the 20 Immune-Related Genes
We performed GO functional enrichment analysis of the 20 IRGs revealing that the IRGs were closely associated with biological processes such as the response to metal ions, the response to cadmium ions, the growth hormone receptor complex, collagen trimers, and peptide binding (Figures 2A–D). The detailed GO analysis results are available in Supplementary Table 1. KEGG functional analysis results suggested that the 20 IRGs mainly affect the IL-17, endocrine resistance, TNF, and estrogen signaling pathways (Figures 2E,F). The IL-17 and endocrine resistance signaling pathways are demonstrated in detail (Figures 2G,H).
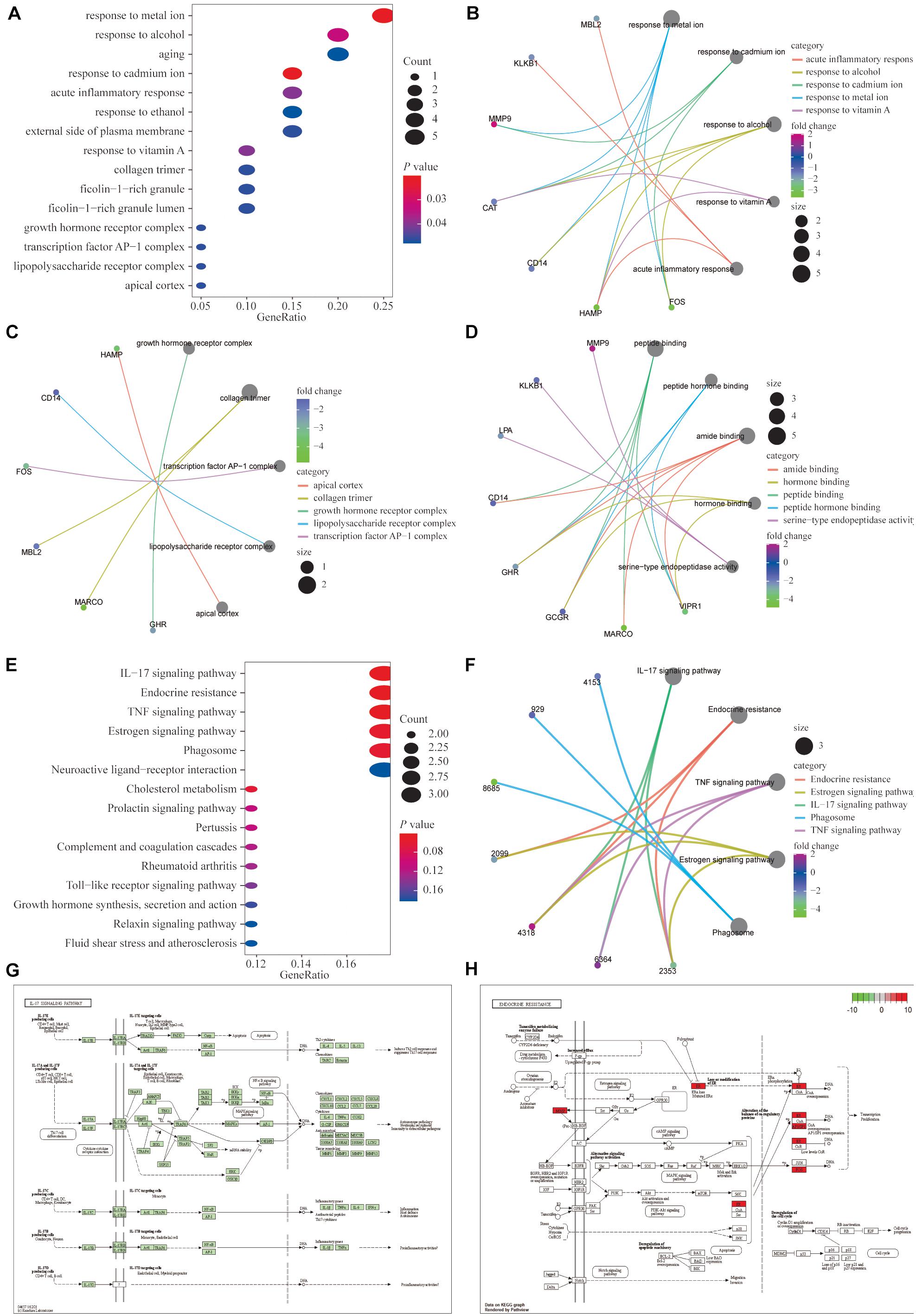
Figure 2. Functional enrichment analysis of the 20 differentially expressed IRGs. (A–D) Gene ontology (GO) analysis showed that the differentially expressed genes (DEGs) were closely associated with biological processes such as responses to metal ion, response to cadmium ion, growth hormone receptor complex, collagen trimer, and peptide binding. (E,F) Kyoto encyclopedia of genes and genomes (KEGG) functional analysis suggested that differentially expressed immune genes mainly affected the IL-17, endocrine resistance, TNF and estrogen signaling pathways. (G,H) Detailed demonstrations of the IL-17 and endocrine resistance pathways.
Moreover, the results of GSEA in the TCGA database showed that the enrichment of the ribosome, the complement and coagulation cascade, and the peroxisome proliferators-activated receptor (PPAR) signaling pathways were significant (Supplementary Figure 4). The specific enrichments of the related pathways are shown in Supplementary Table 2.
To assess the variations in different pathways in patients with HCC, GSVA was performed to analyze the enrichment scores of patients with HCC in each of the four datasets, and the relevant signaling pathways with significant differential expression are shown as heat maps (Supplementary Figure 5). The GSVA results were consistent with the GSEA results.
Identification of the Characteristic Immune-Related Genes
The 20 differentially expressed IRGs were added to the STRING database to construct the PPI network thereby identify characteristic IRGs that are closely related to HCC (Supplementary Figure 6A). MMP9, FOS, CAT, ESR1, ANGPTL3, and KLKB1 were identified as characteristic IRGs based on the MCC algorithm and were selected for further analysis (Supplementary Figure 6B). When we got the characteristic IRGs, we did the GSEA for each characteristic IRG (Supplementary Figure 7).
An interaction network including differential IRGs and miRNAs was constructed (Supplementary Figure 8A). Moreover, we selected differentially expressed transcription factors related to the expression of the 20 IRGs, with the thresholds of a Pearson’s coefficient > 0.4 and a p-value < 0.01, and imported the highly expressed and correlated transcription factors into Cytoscape to construct the transcription factor regulatory network (Supplementary Figure 8B).
Analysis at the mRNA and protein levels, revealed that the characteristic IRGs play key roles in the development of HCC. In addition, IHC analysis was performed to assess the MMP9, ESR1, CAT, FOS, and KLKB1 expression in HCC patients and the expression level of characteristic IRGs (MMP9, ESR1, CAT, FOS, and KLKB1) of IHC were scored blindly and compare to each other using a semi-quantitative analysis method (Figure 3). The MMP9 protein was highly expressed in HCC tissues while the ESR1, CAT, FOS, and KLKB1 proteins were expressed at lower levels in HCC tissues than in adjacent normal tissues. The results of the IHC analyses of patient samples were consistent with the results of the differential expression analysis of the TCGA cohort.
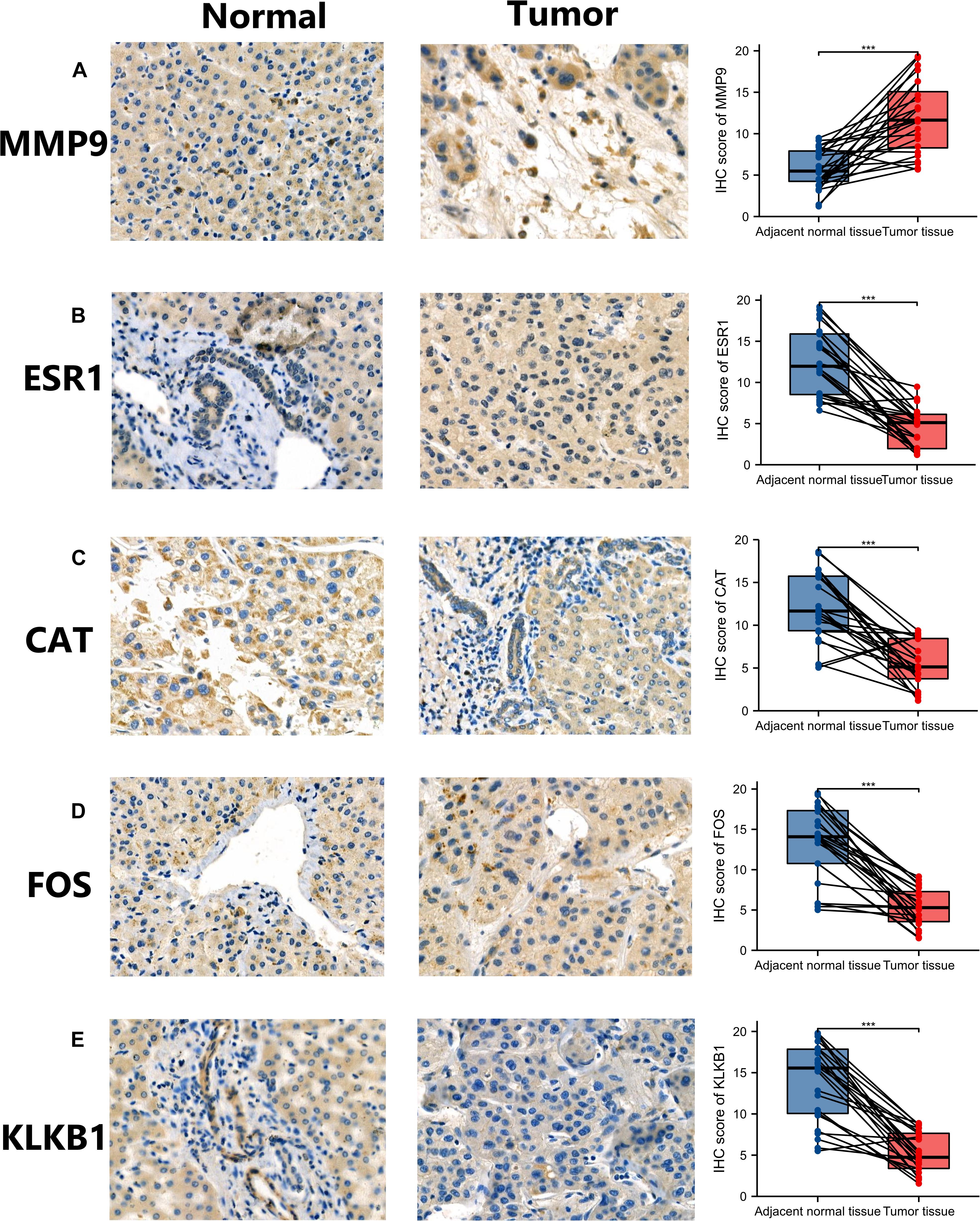
Figure 3. Validation of the expression of characteristic immune-related genes (IRGs) in hepatocellular carcinoma (HCC) by immunohistochemistry (IHC). Panels (A–E) are the results of IHC and semi-quantitative analysis of MMP9, ESR1, CAT, FOS, and KLKB1 in HCC tissues and adjacent normal tissues, respectively. *p < 0.05; **p < 0.01; ***p < 0.001.
Pan-Cancer Analysis of Characteristic Immune Gene
We analyzed the differential expression of 6 characteristic IRGs in 33 tumors from the TCGA database. In the vast majority of tumors, the expression levels of the characteristic IRGs were significantly different, compared to adjacent normal tissues (Supplementary Figure 9). Moreover, the mRNA expression levels of the characteristic IRGs in 1,457 tumor cell lines were determined using the CCLE database (Supplementary Figure 10). The results showed that the six characteristic IRGs may act key roles in most cancers not only in HCC. It provided potential targets for the treatment of cancers.
Associations of the Characteristic Immune-Related Genes With the Immune Microenvironment and Drug Sensitivity
To assess the abilities of the expression levels of the six characteristic IRGs obtained by PPI network analysis to discriminate between HCC tissues and adjacent normal tissues in the TCGA dataset, ROC curve analysis was conducted. All of the characteristic IRGs were able to separate HCC tissues from adjacent normal tissues (Supplementary Figure 11).
We further analyzed the effects of these characteristic IRGs on the immune microenvironment in HCC patients (Figure 4). The six characteristic IRGs showed strong correlations with B cells, CD4+ T cells, CD8+ T cells, neutrophils, macrophages, and dendritic cells (DCs), however, the correlations of FOS with B cells, CAT with neutrophils, and KLKB1 with CD8+ T cells were not statistically significant (P < 0.05).
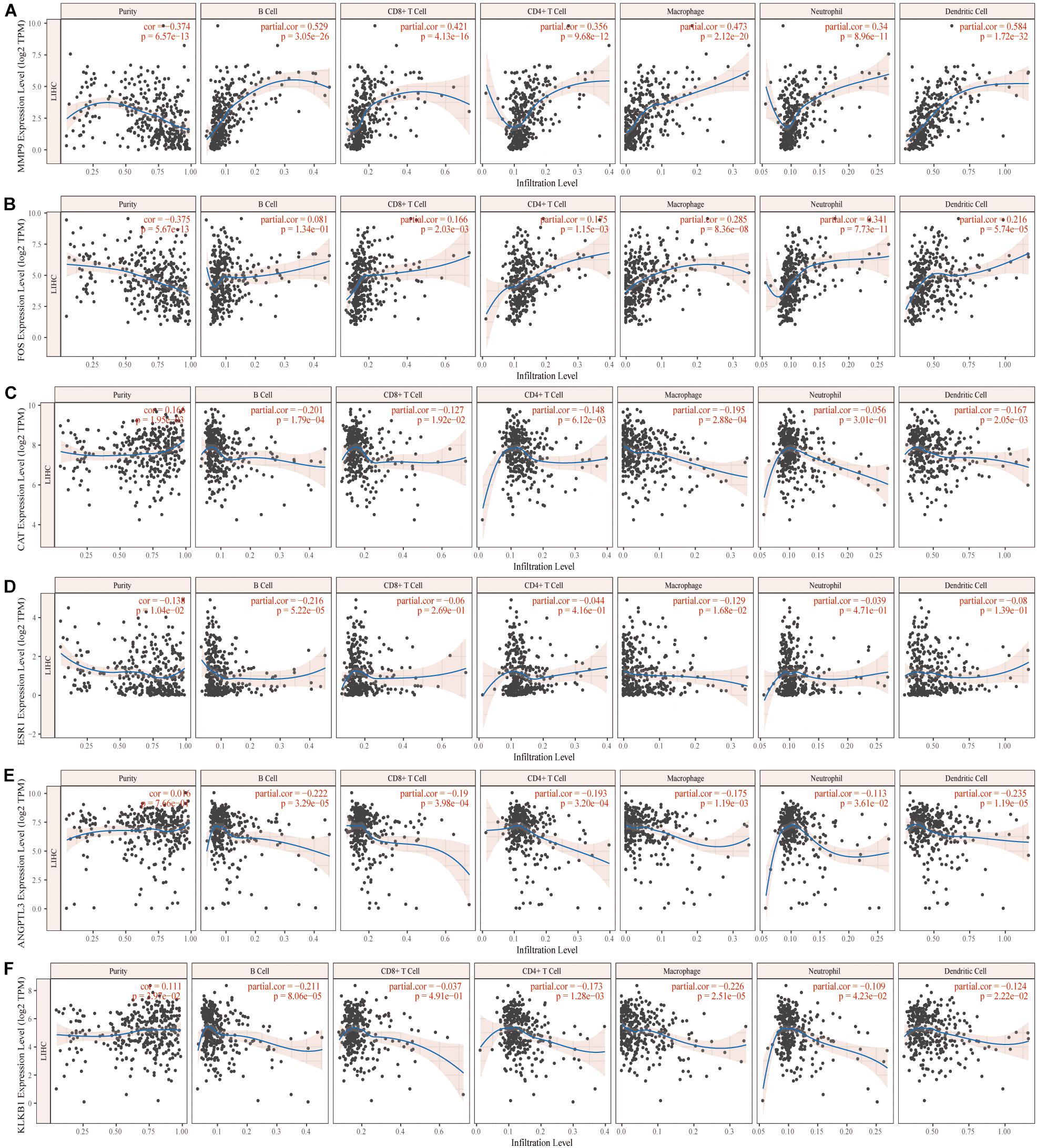
Figure 4. Correlation of the characteristic immune-related genes (IRGs) and the immune microenvironment in hepatocellular carcinoma (HCC) patients (A–F).
Moreover, we further analyzed the associations of these characteristic IRGs with sensitivities to various anti-cancer chemotherapeutic drugs using the CellMiner database (Supplementary Figure 12). A significant positive correlation was observed between the expression of the ESR1 gene and sensitivity to fulvestrant, raloxifene, etc., and between the expression of MMP9 and sensitivity to rebimastat, etc., detailed information is shown in Table 1. This study may provide new insight for the clinical treatment of HCC.
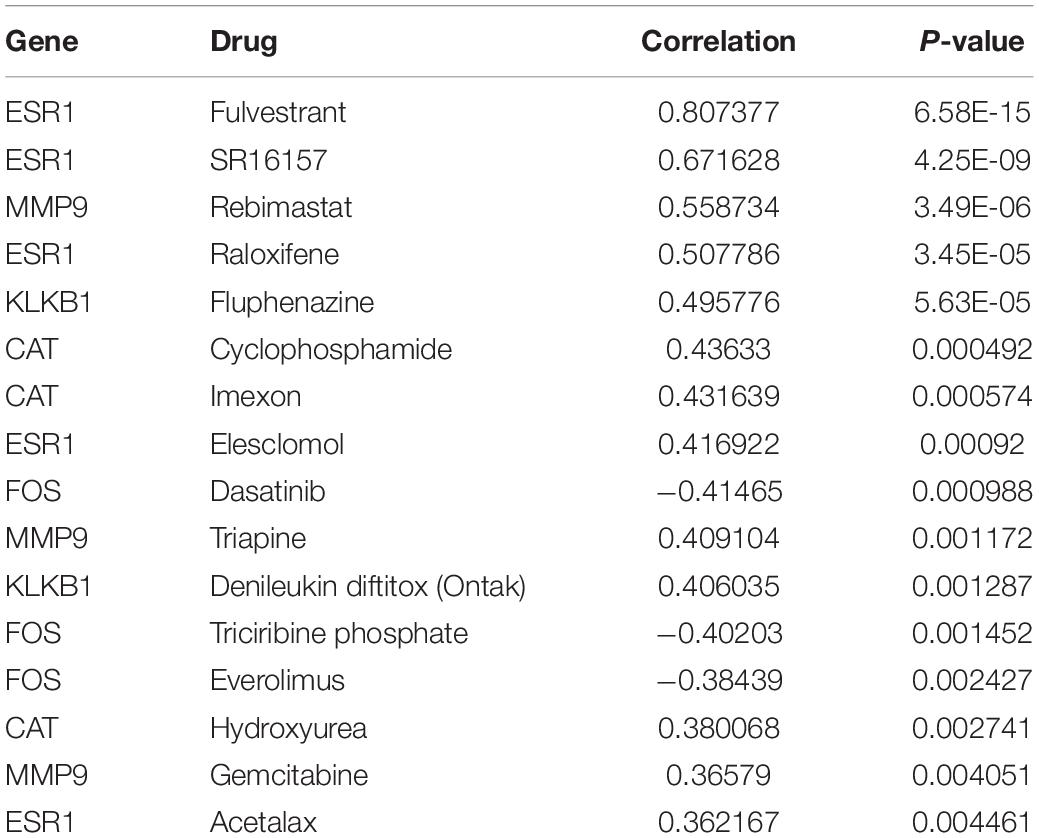
Table 1. Correlations of the six characteristic immune-related genes (IRGs) with anti-cancer drugs sensitivity based on the CellMiner database.
Clinical Correlation Analysis of the Characteristic Immune-Related Genes
Differential expression analysis of the TCGA database showed that among the six characteristic IRGs, the MMP9 gene was significantly expressed in tumor tissues compared with adjacent normal tissues (Figure 5A). In contrast, the remaining five genes were expressed at lower levels in tumor tissues (Figures 5B–F).
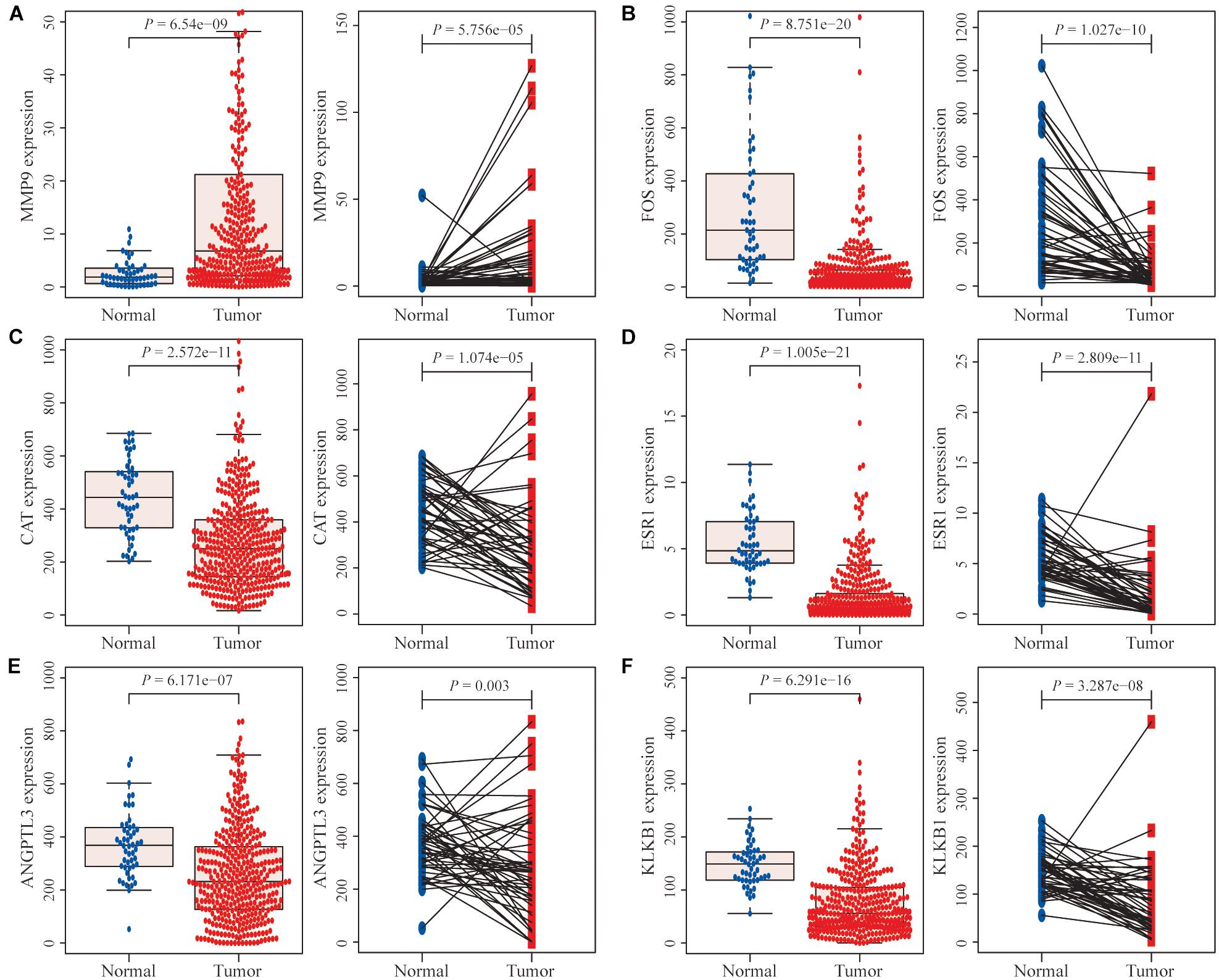
Figure 5. Differential expression analysis of immune-related genes (IRGs). (A) The MMP9 gene was expressed at significantly higher levels in hepatocellular carcinoma (HCC) tissues than in normal HCC tissues and paired adjacent normal tissues. (B–F) The expression levels of the remaining five genes were significantly lower in tumor tissues than in normal tissues and paired adjacent normal tissues.
In addition, the effects of the six IRGs on the prognosis of HCC patients were further studied. High MMP9 gene expression, and low ESR1 gene expression, were closely associated with poor prognosis in HCC patients (log-rank P < 0.001; Figures 6A,D), whereas the expression levels of the other genes had no significant effects on the prognosis of HCC patients (Figures 6B,C,E,F). Moreover, the expression levels of the CAT, ESR1, and KLKB1 genes were significantly correlated with the HCC stage (P < 0.05), while MMP9 was not significantly correlated with the tumor stage (Figure 7).
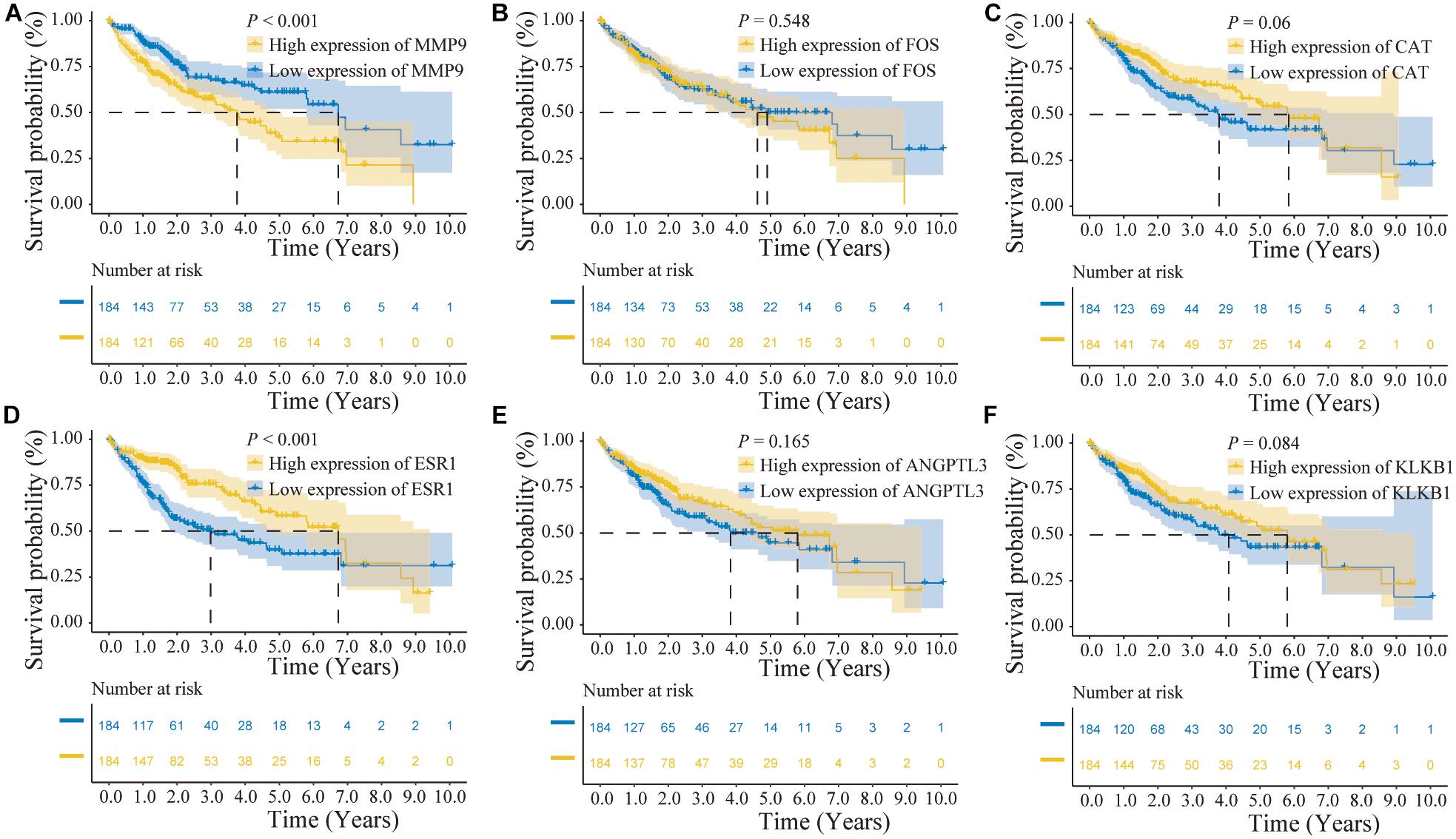
Figure 6. Prognostic analysis of the characteristic immune-related genes (IRGs) in the cancer genome atlas (TCGA) database. (A) High MMP9 gene expression suggested poor patient prognosis (log-rank P < 0.001). (B,C) The expression levels of the FOS and CAT genes were not significantly correlated with the prognosis of hepatocellular carcinoma (HCC) patients. (D) Low ESR1 gene expression suggested poor patient prognosis (log-rank P < 0.001). (E,F) The expression levels of the ANGPTL3 and KLKB1 genes were not significantly correlated with the prognosis of HCC patients.
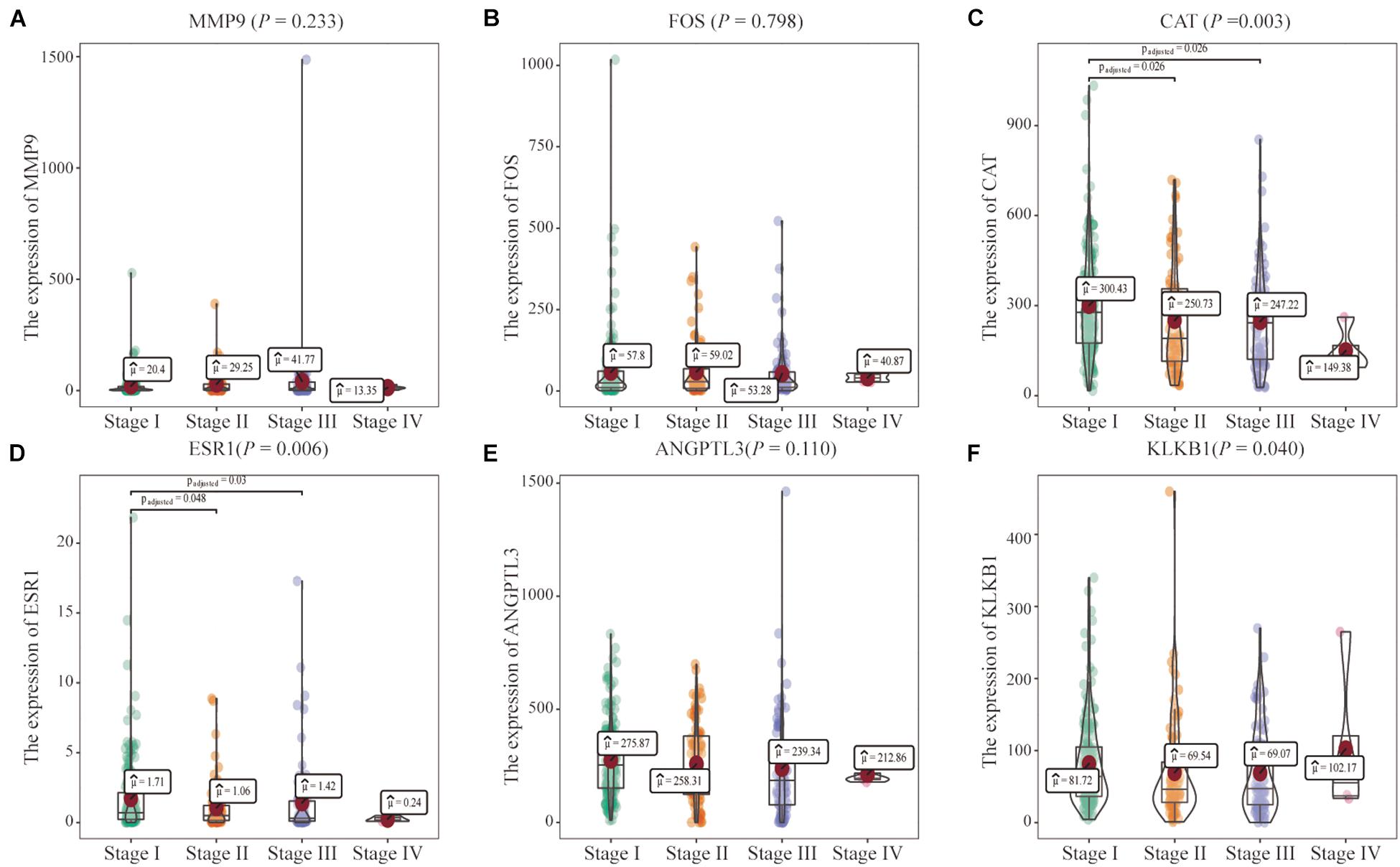
Figure 7. Correlations of the six characteristic immune-related genes (IRGs) with clinical stage. (A–F) The correlation between six characteristics IRGs and clinical stage were analyzed, respectively.
In the multivariate Cox regression analysis, p < 0.2 on univariate analysis were included in multivariable model and we included gender, stage, AFP, hepatitis, and other clinically relevant factors which were important for prognosis, and the expression of MMP9 and ESR1 to determine whether MMP9, ESR1 was an independent factor for prognosis of HCC. The results revealed that the MMP9 and ESR1 genes were independent prognostic predictors for HCC patients (P = 0.011; P = 0.015). While MMP9 expression was identified as a risk factor, ESR1 expression exerted a protective effect on HCC patients (Figure 8). Then we analyzed the correlation of MMP9, ESR1 with PD-1, PD-L1, and CTLA-4 which were called the immune checkpoint genes. We found that the expression of MMP9 has a positive and significant correlation with PD-1 and CTLA-4. While the expression of ESR1 has a negative and significant correlation with PD-L1 and CTLA-4 (Supplementary Figure 13).
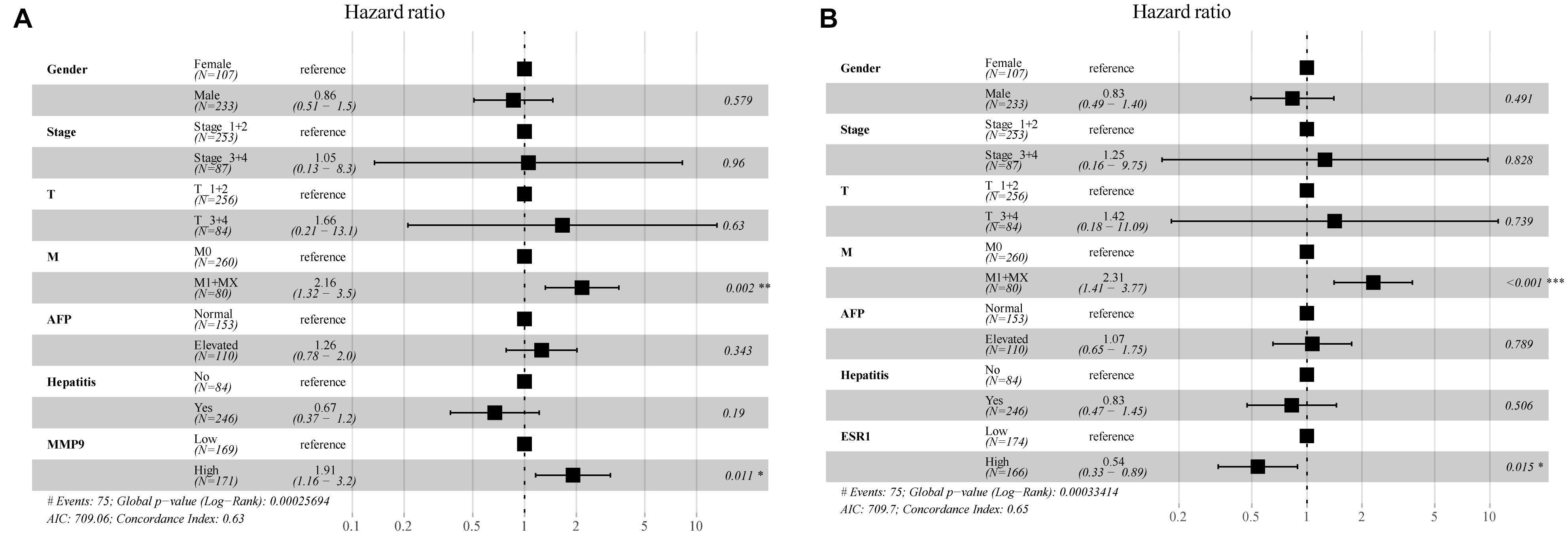
Figure 8. Multifactorial Cox analysis of MMP9 and ESR1 expression in hepatocellular carcinoma (HCC) patients. (A) The results of multifactorial Cox analysis suggested that MMP9 gene was an independent risk factor for the prognosis of HCC patients. (B) The expression of the ESR1 gene was an independent protective factor for the prognosis of HCC patients. *p < 0.05; **p < 0.01; ***p < 0.001.
Next, we incorporated the expression levels of two genes (MMP9 and ESR1) and clinicopathological features into the model (Figures 9A,B). A nomogram was then constructed to predict the OS of patients with HCC (Figures 9C,E). The C-index was used to calculate the discriminatory power of the nomogram, which showed a certain degree of discrimination [MMP9: 0.654 (0.601–0.706); ESR1: 0.658 (0.605–0.710)]. Moreover, the calibration curves showed good agreement between the 1-, 2-, and 3-year OS estimates from the prediction of the nomogram and the actual OS rates of the patients (Figures 9D,F). In addition, the DCA results showed a prognostic benefit for approximately 15–95% of patients as determined by the model (Figures 9G,H).
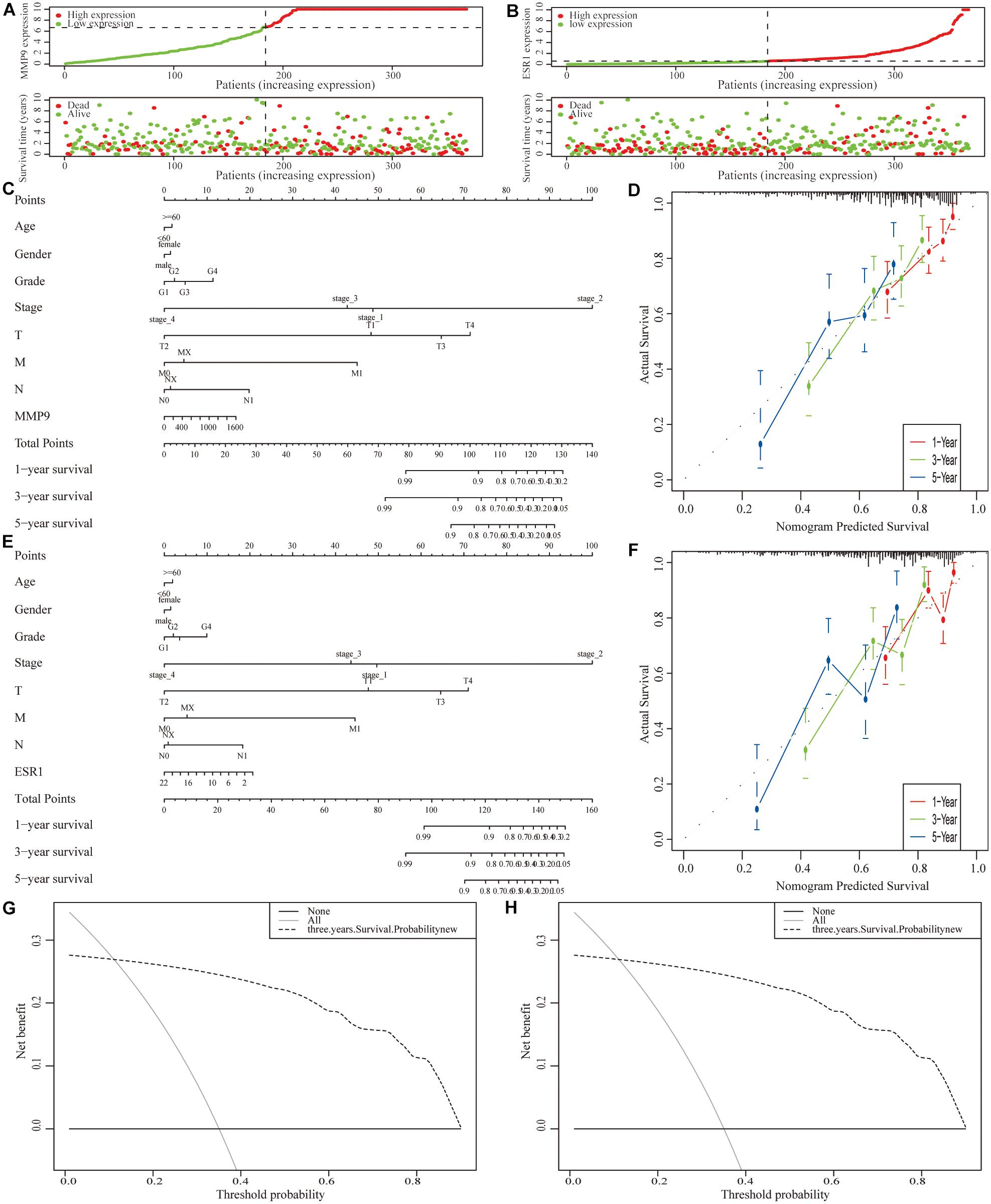
Figure 9. Construction of a clinical prognostic model for patients with hepatocellular carcinoma (HCC). (A,B) Risk factor correlation diagram of the differentially expressed immune-related genes (IRGs). (C) A nomogram was constructed by combining the expression level of MMP9 with clinicopathological features. (D) Calibration curves of the nomogram constructed using the MMP9 gene; the horizontal coordinates are the survival statistics predicted by the nomogram and the vertical coordinates are the actual observed survival situation, each curve was repeated 1,000 times. The curves showed that the nomogram had a good ability to predict prognosis of patients at 1-, 2-, and 3-year. (E) A nomogram was constructed by ESR1 expression with clinicopathological features. (F) Calibration curve of the nomogram constructed using the ESR1 gene. (G,H) Decision curve analysis (DCA) of the nomograms.
We also used the ICGC-JP dataset as an external validation to measure the accuracy and stability of the prognostic model (Supplementary Figure 14).
Discussion
Hepatocellular carcinoma, an aggressive cancer, has a poor long-term prognosis, and ranks as the third leading cause of cancer-related death. In 2017, Food and Drug Administration (FDA) approved the nivolumab for the treatment of HCC patients who have been previously treated with sorafenib, immunotherapy has become a standard of care treatment option for HCC (El-Khoueiry et al., 2017). With the development of immunotherapy, the OS of HCC patients, especially those with advanced liver cancer, has been prolonged. It suggests that immune research is promising in HCC. However, the relationship between IRGs and HCC remains unknown. In this study, we aimed to systematically analyze the relationship between IRGs and clinical stage, and prognosis in HCC patients, and then establish a prognostic model and validate using external dataset. In addition, we first investigated the associations of these IRGs with immune cell infiltration and drug sensitivity in patients with HCC. Compared to other studies of immune-related prognostic models, this study incorporates a larger study population and includes more characteristic IRGs. Besides, our prognostic model is analyzed by multi-omics level and validated by external dataset from ICGC to ensure the credible results and has high AUC values.
The Venn diagrams of DEGs from the four datasets, and the subsequent comparison of commonly altered genes with 1,811 tumor immune genes, revealed a total of 20 IRGs. KEGG pathway analysis showed that IRGs were mainly enriched in the IL-17, endocrine resistance, TNF, and estrogen signaling pathways in HCC patients. IL-17, a cytokine that is involved in the immune response, binds to the IL-17 receptor on the cell membrane. A study published in the Journal of Hepatology in 2020 identified IL-17a as a potential therapeutic target for HCC (Ma et al., 2020). Insulin resistance, TNF and estrogen promote the progression of HCC, and these factors are attracting increased attention from researchers. Inhibitors of G-protein-coupled estrogen receptor (GPER1) that have been characterized could be used to treat or prevent liver cancer (Park et al., 2010; Chaturantabut et al., 2019; Jindal et al., 2019). GSEA revealed that IRGs, in HCC patients, were mainly enriched in the ribosome, complement and coagulation cascade, and PPAR signaling pathways. A previous study reported that many lncRNAs, that bind to the ribosomal protein S6 in cancer cells, promote HCC progression by regulating cell proliferation and migration, and their levels are correlated with poor prognosis in HCC patients (Pang et al., 2020). The complement system has many functions in vivo, such as functioning as a proteolytic cascade in serum, mediating innate immunity, and providing extra protection against pathogens. Regardless of the factors driving HCC, the liver immune microenvironment is the main factor associated with chronic inflammation, the progression of liver fibrosis, and cirrhosis. The complement cascade, which is related to these immunological mechanisms, is central to the network and tightly regulates humoral and cellular responses to external stimuli (Malik et al., 2020). PPARs, nuclear hormone receptors, could be activated by fatty acids and their derivatives and PPAR agonists are beneficial for the treatment of non-alcoholic fatty liver disease (NAFLD), liver fibrosis, and HCC (Cao et al., 2009). Recent, studies demonstrated that PPARs participate in the progression of gut microbiota inhabitation and adaptation which is related to the pathogenesis of HCC (Yu et al., 2020). These signaling pathways were shown to be associated with HCC progression in our analysis and were consistent with those reported in published literature.
We incorporated 20 IRGs into the PPI network and obtained 6 characteristic IRGs, namely, MMP9, FOS, CAT, ESR1, ANGPTL3, and KLKB1, by applying the MCC algorithm. MMP9 plays key roles in local extracellular matrix proteolysis and in leukocyte migration. A previous study showed that HCC progression was inhibited by 7-methoxy-1-tetralone, possibly via the modulation of proliferation- and migration-related mediators, including MMP9 (Wen et al., 2020). The FOS gene encodes leucine zipper proteins that, dimerize with proteins of the JUN family, to form the AP-1, transcription factor complex. FOS proteins have been associated with the regulation of cell proliferation, differentiation, and transformation. FOS gene expression has been associated with apoptotic cell death and liver carcinogenesis is related to FOS-dependent inflammation (Bakiri et al., 2017). ESR1, also known as NR3A1, is one of the two main types of estrogen receptors. A previous study demonstrated that the expression of ESR1 gene is decreased by >90%, in almost 50% of HCC patients (Hishida et al., 2013). Decreased expression of this gene was significantly related to a high liver damage score, pathological invasion of the intrahepatic portal vein, tumor size and hepatitis B virus infection, showed that ESR1 gene is a candidate protective gene in HCC (Hishida et al., 2013). ANGPTL3 encodes secreted proteins that were expressed predominantly in the liver and plays a role in angiogenesis. ANGPTL3 expression and serum levels could act as novel biomarkers in the diagnosis of chronic hepatitis and HCC, and ANGPTL3 expression could be useful for discriminating HCC from chronic hepatitis in patients (El-Shal et al., 2017). In addition, we showed for the first time that CAT, and KLKB1 may be potential therapeutic targets in HCC patients. The differential expression of MMP9, ESR1, CAT, FOS, KLKB1 in HCC patients were verified by IHC.
In addition, the HCC microenvironment includes parenchymal cells, which are complex immune-related cells; however, the success of immune checkpoint suppression in solid tumors highlights the key role of the TME in tumor progression. TIMER was utilized to explore the effects of six characteristic IRGs on the infiltration of immune cells, revealing that MMP9, ESR1, and ANGPTL3 were closely associated with B cells, CD4+ T cells, CD8+ T cells, neutrophils, macrophages, and DCs. All six characteristic IRGs showed strong associations with immune cells, but the associations of the FOS gene with B cells, the CAT gene with neutrophils, and the KLKB1 gene with CD8 cells were not significant. Thus, we characterized the associations of these IRGs with the levels of immune cell infiltration in HCC patients, thereby providing a new reference for immunotherapy. However, immune cells such as T cells and DCs have different subtypes and further studies needed to confirm the detailed mechanisms of IRGs and different immune cell subtypes.
Notably, ROC curve analysis was performed to confirm the expression of the six characteristic IRGs that sufficiently distinguished HCC tissues from adjacent normal tissues. We analyzed the differential expression of the six characteristic IRGs in HCC patient tissues; MMP9 was shown to be expressed at significantly higher levels in HCC tissues than in normal tissues, while the other five genes were expressed at significantly lower levels in HCC tissues than in adjacent normal tissues. Further analysis of the prognostic impacts of the six characteristic IRGs on HCC patients revealed significant correlations of high MMP9 gene expression and low ESR1 gene expression with a poor prognosis in HCC patients. Recent studies revealed the inhibition of MMP9 which is regulated by α1-ACT, could suppress liver cancer development (Zhu et al., 2017). Previous studies on ESR1 focused on breast cancer, and breast cancer patients highly expressing the ESR1 gene were shown to have a better prognosis; however, recent studies showed that ESR1 is also associated with breast cancer liver metastasis (De Santo et al., 2019; Tian et al., 2021). We further found that MMP9 and ESR1 were independent predictors of HCC patient prognosis by performing multifactorial Cox regression analysis and we found that there are strong and significant correlation between MMP9 and ESR1 with CTLA-4. In addition, we first revealed that the gene expression levels of CAT, ESR1, and KLKB1 were significantly associated with the HCC clinical stage. For the first time, we also analyzed the associations of IRGs with therapeutic sensitivity to anti-cancer drugs. The expression of the ESR1 gene had a significant positive correlation with sensitivity to fulvestrant, raloxifene, etc. While the expression of the MMP9 gene had a significant positive correlation with sensitivity to rebimastat. Previous studies showed that fulvestrant inhibited the proliferation of HepG2 cell, via the ERα and non-canonical Wnt pathways, and indicated that it may be a promising therapeutic for HCC (Wang et al., 2014). Another study demonstrated that the effective delivery of raloxifene had proapoptotic and cytotoxic effects on HCC cell lines (Almutairi et al., 2019). However, studies on HCC and rebimastat have not been reported.
This study does have some limitations. First, subgroup analysis of IRGs, together with more clinical characteristics of HCC patients should be performed to comprehensively identify the factors and effects influencing HCC prognosis. Second, 851 liver cancer patient tissues were included in the analysis, but complete clinical information was available for only 340 samples. In future studies, cross-validation with internal datasets and increased sample sizes from other databases are needed. Third, in this study, we analyzed the association between IRGs with the sensitivity to different anti-cancer drugs, but the association between IRGs with the sensitivity to immunotherapeutic drugs need to pay attention. Finally, because obtaining fresh HCC samples was difficult, we used the paraffin-embedded sections from only HCC patients to validate our findings by IHC, and more data obtained by additional techniques such as qPCR are needed.
Conclusion
In summary, the 20 identified DEGs were mainly enriched for numerous immune-related GO terms and KEGG pathways, and the top six IRGs (MMP9, FOS, CAT, ESR1, ANGPTL3, and KLKB1) were selected for further study. We then analyzed the effect of the six characteristic IRGs on the HCC immune microenvironment and found positive correlations between their expression levels and the infiltration of a variety of immune cells. Two characteristic IRGs (MMP9 and ESR1) were significantly associated with the prognosis of HCC, and multifactorial Cox regression analysis showed that they were independent prognostic factors. We are the first to demonstrate that characteristic IRGs (CAT, ESR1, and KLKB1) are significantly associated with the clinical stage of HCC. Moreover, for the first time, we investigated the associations of characteristic IRGs with anti-cancer drug sensitivity, and found that fulvestrant, raloxifene, SR16157, and rebimastat have potential therapeutic effects in HCC. Besides, we found a significant correlation between MMP9, ESR1, and the immune checkpoint gene CTLA4, suggesting that it may be a potential molecular target. Most importantly, we successfully established two prognostic models for HCC based on independent prognostic IRGs (MMP9, ESR1) that provided prognostic benefit to approximately 15–95% of HCC patients and the predicted OS rates were in good agreement with the actual observed rates. In addition, we validated the prognostic models using the data from ICGC database.
Data Availability Statement
The datasets presented in this study can be found in online repositories. The names of the repository/repositories and accession number(s) can be found in the article/Supplementary Material.
Ethics Statement
The studies involving human participants were reviewed and approved by the Xijing Hospital’s Ethics Committee. The patients/participants provided their written informed consent to participate in this study.
Author Contributions
YH, JBW, and XMZ conceived and designed the experiments. XPD, YNH, FFY, and GYX collected the data. JHY, SYT, MZ, YSL, and SYM analyzed the data. JHY, MZ, and SYT drafted the manuscript. All authors critically reviewed the manuscript.
Funding
This study was funded by the National Natural Science Foundation of China (Nos. 81770569, 81870421, and 81773072), the International Cooperation and Exchange of the National Natural Science Foundation of China (No. 81820108005).
Conflict of Interest
The authors declare that the research was conducted in the absence of any commercial or financial relationships that could be construed as a potential conflict of interest.
Publisher’s Note
All claims expressed in this article are solely those of the authors and do not necessarily represent those of their affiliated organizations, or those of the publisher, the editors and the reviewers. Any product that may be evaluated in this article, or claim that may be made by its manufacturer, is not guaranteed or endorsed by the publisher.
Supplementary Material
The Supplementary Material for this article can be found online at: https://www.frontiersin.org/articles/10.3389/fcell.2021.700553/full#supplementary-material
Footnotes
- ^ https://portal.gdc.cancer.gov/
- ^ http://xena.ucsc.edu/
- ^ https://www.immport.org/
- ^ http://software.broadinstitute.org/gsea/msigdb/index.jsp
- ^ http://www.string-db.org/
- ^ https://mirtarbase.cuhk.edu.cn/
- ^ http://www.mirdb.org/
- ^ http://www.targetscan.org/vert_71/
- ^ https://www.networkanalyst.ca/
- ^ https://discover.nci.nih.gov/cellminer/home.do
- ^ https://cistrome.shinyapps.io/timer/
- ^ http://gepia.cancer-pku.cn/index.html
References
Almutairi, F. M., Abd-Rabou, A. A., and Mohamed, M. S. (2019). Raloxifene-encapsulated hyaluronic acid-decorated chitosan nanoparticles selectively induce apoptosis in lung cancer cells. Bioorgan. Med. Chem. 27, 1629–1638. doi: 10.1016/j.bmc.2019.03.004
Ayuso, C., Rimola, J., Vilana, R., Burrel, M., Darnell, A., and García-Criado, Á, et al. (2018). Diagnosis and staging of hepatocellular carcinoma (HCC): current guidelines. Eur. J. Radiol. 101, 72–81. doi: 10.1016/j.ejrad.2018.01.025
Bakiri, L., Hamacher, R., Graña, O., Guío-Carrión, A., Campos-Olivas, R., Martinez, L., et al. (2017). Liver carcinogenesis by FOS-dependent inflammation and cholesterol dysregulation. J. Exper. Med. 214, 1387–1409. doi: 10.1084/jem.20160935
Cao, L. Q., Wang, X. L., Wang, Q., Xue, P., Jiao, X. Y., Peng, H. P., et al. (2009). Rosiglitazone sensitizes hepatocellular carcinoma cell lines to 5-fluorouracil antitumor activity through activation of the PPARgamma signaling pathway. Acta Pharmacol. Sin. 30, 1316–1322. doi: 10.1038/aps.2009.119
Chaturantabut, S., Shwartz, A., Evason, K. J., Cox, A. G., Labella, K., Schepers, A. G., et al. (2019). Estrogen activation of G-Protein-coupled estrogen receptor 1 regulates Phosphoinositide 3-Kinase and mTOR signaling to promote liver growth in Zebrafish and proliferation of human hepatocytes. Gastroenterology 156, 1788–1804.e13. doi: 10.1053/j.gastro.2019.01.010
Chen, Y., and Wang, X. (2020). miRDB: an online database for prediction of functional microRNA targets. Nucleic Acids Res. 48, D127–D131. doi: 10.1093/nar/gkz757
Chikuma, S. (2017). CTLA-4, an essential immune-checkpoint for T-cell activation. Curr. Top. Microbiol. Immunol. 410, 99–126. doi: 10.1007/82_2017_61
Chin, C. H., Chen, S. H., Wu, H. H., Ho, C. W., Ko, M. T., and Lin, C. Y. (2014). cytoHubba: identifying hub objects and sub-networks from complex interactome. BMC Syst. Biol. 8, (Suppl. 4):S11. doi: 10.1186/1752-0509-8-S4-S11
De Santo, I., McCartney, A., Migliaccio, I., Di Leo, A., and Malorni, L. (2019). The emerging role of ESR1 mutations in luminal breast cancer as a prognostic and predictive biomarker of response to endocrine therapy. Cancers 11:1894. doi: 10.3390/cancers11121894
El-Khoueiry, A. B., Sangro, B., Yau, T., Crocenzi, T. S., Kudo, M., Hsu, C., et al. (2017). Nivolumab in patients with advanced hepatocellular carcinoma (CheckMate 040): an open-label, non-comparative, phase 1/2 dose escalation and expansion trial. Lancet 389, 2492–2502. doi: 10.1016/s0140-6736(17)31046-2
El-Shal, A. S., Zidan, H. E., Rashad, N. M., and Wadea, F. M. (2017). Angiopoietin-like protein 3 and 4 expression 4 and their serum levels in hepatocellular carcinoma. Cytokine 96, 75–86. doi: 10.1016/j.cyto.2017.03.006
Finn, R. S., Qin, S., Ikeda, M., Galle, P. R., Ducreux, M., Kim, T. Y., et al. (2020). Atezolizumab plus bevacizumab in unresectable hepatocellular carcinoma. N. Engl. J. Med. 382, 1894–1905. doi: 10.1056/NEJMoa1915745
Garris, C. S., Arlauckas, S. P., Kohler, R. H., Trefny, M. P., Garren, S., Piot, C., et al. (2018). Successful Anti-PD-1 cancer immunotherapy requires T cell-dendritic cell crosstalk involving the cytokines IFN-γ and IL-12. Immunity 49, 1148–1161.e7. doi: 10.1016/j.immuni.2018.09.024
Giannini, E., Farinati, F., Ciccarese, F., Pecorelli, A., Rapaccini, G., Di Marco, M., et al. (2015). Prognosis of untreated hepatocellular carcinoma. Hepatology 61, 184–190. doi: 10.1002/hep.27443
Hänzelmann, S., Castelo, R., and Guinney, J. (2013). GSVA: gene set variation analysis for microarray and RNA-seq data. BMC Bioinform. 14:7. doi: 10.1186/1471-2105-14-7
Hishida, M., Nomoto, S., Inokawa, Y., Hayashi, M., Kanda, M., Okamura, Y., et al. (2013). Estrogen receptor 1 gene as a tumor suppressor gene in hepatocellular carcinoma detected by triple-combination array analysis. Intern. J. Oncol. 43, 88–94. doi: 10.3892/ijo.2013.1951
Huang, H. Y., Lin, Y. C., Li, J., Huang, K. Y., Shrestha, S., Hong, H. C., et al. (2020). miRTarBase 2020: updates to the experimentally validated microRNA-target interaction database. Nucleic Acids Res. 48, D148–D154. doi: 10.1093/nar/gkz896
Jayant, K., Habib, N., Huang, K., Podda, M., Warwick, J., and Arasaradnam, R. (2020). Immunological basis of genesis of hepatocellular carcinoma: unique challenges and potential opportunities through immunomodulation. Vaccines 8:247. doi: 10.3390/vaccines8020247
Jindal, A., Thadi, A., and Shailubhai, K. (2019). Hepatocellular carcinoma: etiology and current and future drugs. J. Clin. Exper. Hepatol. 9, 221–232. doi: 10.1016/j.jceh.2019.01.004
Kang, S. J., Cho, Y. R., Park, G. M., Ahn, J. M., Han, S. B., Lee, J. Y., et al. (2013). Predictors for functionally significant in-stent restenosis: an integrated analysis using coronary angiography, IVUS, and myocardial perfusion imaging. JACC Cardiovasc. Imag. 6, 1183–1190. doi: 10.1016/j.jcmg.2013.09.006
Keenan, B., Fong, L., and Kelley, R. (2019). Immunotherapy in hepatocellular carcinoma: the complex interface between inflammation, fibrosis, and the immune response. J. Immunother. Cancer 7:267. doi: 10.1186/s40425-019-0749-z
Kulik, L., and El-Serag, H. B. (2019). Epidemiology and management of hepatocellular carcinoma. Gastroenterology 156, 477–491.e1. doi: 10.1053/j.gastro.2018.08.065
Lewis, B. P., Burge, C. B., and Bartel, D. P. (2005). Conserved seed pairing, often flanked by adenosines, indicates that thousands of human genes are microRNA targets. Cell 120, 15–20. doi: 10.1016/j.cell.2004.12.035
Lewis, B. P., Shih, I. H., Jones-Rhoades, M. W., Bartel, D. P., and Burge, C. B. (2003). Prediction of mammalian microRNA targets. Cell 115, 787–798. doi: 10.1016/s0092-8674(03)01018-3
Li, B., Severson, E., Pignon, J. C., Zhao, H., Li, T., Novak, J., et al. (2016). Comprehensive analyses of tumor immunity: implications for cancer immunotherapy. Genome Biol. 17:174. doi: 10.1186/s13059-016-1028-7
Li, T., Fan, J., Wang, B., Traugh, N., Chen, Q., Liu, J. S., et al. (2017). TIMER: a web server for comprehensive analysis of tumor-infiltrating immune cells. Cancer Res. 77, e108–e110. doi: 10.1158/0008-5472.CAN-17-0307
Love, M. I., Huber, W., and Anders, S. (2014). Moderated estimation of fold change and dispersion for RNA-seq data with DESeq2. Genome Biol. 15:550. doi: 10.1186/s13059-014-0550-8
Luo, S., Chen, Y., Su, X., and Chu, H. (2014). mmeta: an R package for multivariate meta-analysis. J. Statist. Softw. 56:11.
Ma, H. Y., Yamamoto, G., Xu, J., Liu, X., Karin, D., Kim, J. Y., et al. (2020). IL-17 signaling in steatotic hepatocytes and macrophages promotes hepatocellular carcinoma in alcohol-related liver disease. J. Hepatol. 72, 946–959. doi: 10.1016/j.jhep.2019.12.016
Malik, A., Thanekar, U., Amarachintha, S., Mourya, R., Nalluri, S., Bondoc, A., et al. (2020). “Complimenting the complement”: mechanistic insights and opportunities for therapeutics in hepatocellular carcinoma. Front. Oncol. 10:627701. doi: 10.3389/fonc.2020.627701
Pang, Y., Liu, Z., Han, H., Wang, B., Li, W., Mao, C., et al. (2020). Peptide SMIM30 promotes HCC development by inducing SRC/YES1 membrane anchoring and MAPK pathway activation. J. Hepatol. 73, 1155–1169. doi: 10.1016/j.jhep.2020.05.028
Park, E. J., Lee, J. H., Yu, G. Y., He, G., Ali, S. R., Holzer, R. G., et al. (2010). Dietary and genetic obesity promote liver inflammation and tumorigenesis by enhancing IL-6 and TNF expression. Cell. 140, 197–208. doi: 10.1016/j.cell.2009.12.052
Reinhold, W. C., Sunshine, M., Liu, H., Varma, S., Kohn, K. W., Morris, J., et al. (2012). CellMiner: a web-based suite of genomic and pharmacologic tools to explore transcript and drug patterns in the NCI-60 cell line set. Cancer Res. 72, 3499–3511. doi: 10.1158/0008-5472.CAN-12-1370
Rizvi, A. A., Karaesmen, E., Morgan, M., Preus, L., Wang, J., Sovic, M., et al. (2019). gwasurvivr: an R package for genome-wide survival analysis. Bioinformatics 35, 1968–1970. doi: 10.1093/bioinformatics/bty920
Robin, X., Turck, N., Hainard, A., Tiberti, N., Lisacek, F., Sanchez, J. C., et al. (2011). pROC: an open-source package for R and S+ to analyze and compare ROC curves. BMC Bioinform. 12:77. doi: 10.1186/1471-2105-12-77
Roessler, S., Jia, H. L., Budhu, A., Forgues, M., Ye, Q. H., Lee, J. S., et al. (2010). A unique metastasis gene signature enables prediction of tumor relapse in early-stage hepatocellular carcinoma patients. Cancer Res. 70, 10202–10212. doi: 10.1158/0008-5472.can-10-2607
Shannon, P., Markiel, A., Ozier, O., Baliga, N. S., Wang, J. T., Ramage, D., et al. (2003). Cytoscape: a software environment for integrated models of biomolecular interaction networks. Genome Res. 13, 2498–2504. doi: 10.1101/gr.1239303
Sharpe, A. H., and Pauken, K. E. (2018). The diverse functions of the PD1 inhibitory pathway. Nat. Rev. Immunol. 18, 153–167. doi: 10.1038/nri.2017.108
Sia, D., Jiao, Y., Martinez-Quetglas, I., Kuchuk, O., Villacorta-Martin, C., Castro de Moura, M., et al. (2017). Identification of an immune-specific class of hepatocellular carcinoma, based on molecular features. Gastroenterology 153, 812–826. doi: 10.1053/j.gastro.2017.06.007
Sung, H., Ferlay, J., Siegel, R. L., Laversanne, M., Soerjomataram, I., Jemal, A., et al. (2021). Global cancer statistics 2020: GLOBOCAN estimates of incidence and mortality worldwide for 36 cancers in 185 countries. CA Cancer J. Clin. 71, 209–249. doi: 10.3322/caac.21660
Szklarczyk, D., Gable, A. L., Lyon, D., Junge, A., Wyder, S., Huerta-Cepas, J., et al. (2019). STRING v11: protein-protein association networks with increased coverage, supporting functional discovery in genome-wide experimental datasets. Nucleic Acids Res. 47, D607–D613. doi: 10.1093/nar/gky1131
Tang, Z., Li, C., Kang, B., Gao, G., Li, C., and Zhang, Z. (2017). GEPIA: a web server for cancer and normal gene expression profiling and interactive analyses. Nucleic Acids Res. 45, W98–W102. doi: 10.1093/nar/gkx247
Tian, C., Liu, S., Wang, Y., and Song, X. (2021). Prognosis and genomic landscape of liver metastasis in patients with breast cancer. Front. Oncol. 11:588136. doi: 10.3389/fonc.2021.588136
Van Calster, B., Wynants, L., Verbeek, J. F. M., Verbakel, J. Y., Christodoulou, E., Vickers, A. J., et al. (2018). Reporting and interpreting decision curve analysis: a guide for investigators. Eur. Urol. 74, 796–804. doi: 10.1016/j.eururo.2018.08.038
Wang, C. J., Guo, D. K., You, T. G., Shen, D. W., Wang, C., Tang, L., et al. (2014). Inhibition of hepatocellular carcinoma by fulvestrant involves the estrogen receptor α and Wnt pathways in vitro and in patients. Mol. Med. Rep. 10, 3125–3131. doi: 10.3892/mmr.2014.2595
Wang, J., Ha, J., Lopez, A., Bhuket, T., Liu, B., and Wong, R. J. (2018). Medicaid and uninsured hepatocellular carcinoma patients have more advanced tumor stage and are less likely to receive treatment. J. Clin. Gastroenterol. 52, 437–443. doi: 10.1097/mcg.0000000000000859
Wang, W. J., Wang, H., Hua, T. Y., Song, W., Zhu, J., Wang, J. J., et al. (2020). Establishment of a prognostic model using immune-related genes in patients with hepatocellular carcinoma. Front. Genet. 11:55. doi: 10.3389/fgene.2020.00055
Wen, Y., Cai, X., Chen, S., Fu, W., Chai, D., Zhang, H., et al. (2020). 7-Methoxy-1-tetralone induces apoptosis, suppresses cell proliferation and migration in hepatocellular carcinoma via regulating c-Met, p-AKT, NF-κB, MMP2, and MMP9 expression. Front. Oncol. 10:58. doi: 10.3389/fonc.2020.00058
Yu, G., Wang, L. G., Han, Y., and He, Q. Y. (2012). clusterProfiler: an R package for comparing biological themes among gene clusters. OMICS J. Integr. Biol. 16, 284–287. doi: 10.1089/omi.2011.0118
Yu, Q., Wu, L., Ji, J., Feng, J., Dai, W., Li, J., et al. (2020). Gut microbiota, peroxisome proliferator-activated receptors, and hepatocellular carcinoma. J. Hepatocell. Carcinoma 7, 271–288. doi: 10.2147/jhc.s277870
Zhang, Q., He, Y., Luo, N., Patel, S. J., Han, Y., Gao, R., et al. (2019). Landscape and dynamics of single immune cells in hepatocellular carcinoma. Cell 179, 829–845.e20. doi: 10.1016/j.cell.2019.10.003
Zhou, Z. J., Xin, H. Y., Li, J., Hu, Z. Q., Luo, C. B., and Zhou, S. L. (2019). Intratumoral plasmacytoid dendritic cells as a poor prognostic factor for hepatocellular carcinoma following curative resection. Cancer Immunol. Immunother. 68, 1223–1233. doi: 10.1007/s00262-019-02355-3
Keywords: prognostic model, gene expression omnibus (GEO), the cancer genome atlas (TCGA), immune-related genes, hepatocellular carcinoma, ICGC
Citation: Yu J, Ma S, Tian S, Zhang M, Ding X, Liu Y, Yang F, Hu Y, Xuan G, Zhou X, Wang J and Han Y (2021) Systematic Construction and Validation of a Prognostic Model for Hepatocellular Carcinoma Based on Immune-Related Genes. Front. Cell Dev. Biol. 9:700553. doi: 10.3389/fcell.2021.700553
Received: 26 April 2021; Accepted: 08 September 2021;
Published: 04 October 2021.
Edited by:
Dechun Feng, National Institute on Alcohol Abuse and Alcoholism (NIAAA), United StatesReviewed by:
Haitao Zhao, Peking Union Medical College Hospital (CAMS), ChinaAlexis Leal, University of Colorado, Denver, United States
Copyright © 2021 Yu, Ma, Tian, Zhang, Ding, Liu, Yang, Hu, Xuan, Zhou, Wang and Han. This is an open-access article distributed under the terms of the Creative Commons Attribution License (CC BY). The use, distribution or reproduction in other forums is permitted, provided the original author(s) and the copyright owner(s) are credited and that the original publication in this journal is cited, in accordance with accepted academic practice. No use, distribution or reproduction is permitted which does not comply with these terms.
*Correspondence: Ying Han, hanying1@fmmu.edu.cn; Jingbo Wang, jimberw@163.com; Xinmin Zhou, zhouxmm@fmmu.edu.cn
†These authors have contributed equally to this work