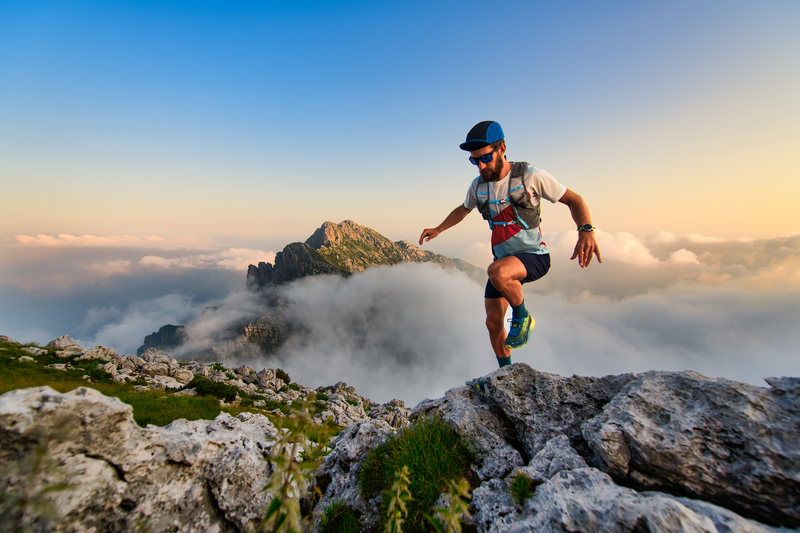
95% of researchers rate our articles as excellent or good
Learn more about the work of our research integrity team to safeguard the quality of each article we publish.
Find out more
ORIGINAL RESEARCH article
Front. Cell Dev. Biol. , 30 September 2021
Sec. Epigenomics and Epigenetics
Volume 9 - 2021 | https://doi.org/10.3389/fcell.2021.661381
This article is part of the Research Topic Role of Genetics and Epigenetics in Major Structural Malformations View all 28 articles
Down's syndrome (DS) is one of the most commonly known disorders with multiple congenital disabilities. Besides severe cognitive impairment and intellectual disability, individuals with DS also exhibit additional phenotypes of variable penetrance and severity, with one or more comorbid conditions, including Alzheimer's disease, congenital heart disease, or leukemia. Various vital genes and regulatory networks had been studied to reveal the pathogenesis of the disease. Nevertheless, very few studies have examined alternative splicing. Alternative splicing (AS) is a regulatory mechanism of gene expression when making one multi-exon protein-coding gene produce more than one unique mature mRNA. We employed the GeneChip Human Transcriptome Array 2.0 (HTA 2.0) for the global gene analysis with hiPSCs from DS and healthy individuals. Examining differentially expressed genes (DEGs) in these groups and focusing on specific transcripts with AS, 466 up-regulated and 722 down-regulated genes with AS events were identified. These genes were significantly enriched in biological processes, such as cell adhesion, cardiac muscle contraction, and immune response, through gene ontology (GO) analysis of DEGs. Candidate genes, such as FN1 were further explored for potentially playing a key role in DS. This study provides important insights into the potential role that AS plays in DS.
Down's syndrome (DS) or trisomy 21 (OMIM #190685) is a well-recognized and studied complex genetic condition caused by a chromosomal disorder, namely the presence of a total or partial trisomy of chromosome 21 (HSA21). It occurs in ~1:700–1:1,000 newborns globally (Weijerman and de Winter, 2010), and it is the most commonly known genetic etiology associated with moderate to severe intellectual disability. Furthermore, individuals with DS also exhibit additional phenotypes of variable penetrance and severity, with one or more comorbid conditions, including Alzheimer's disease, congenital heart disease, or leukemia (Ballard et al., 2016). Further understanding of the relationship between redundant chromosome 21 and its associated diseases is expected to provide theoretical support for revealing the pathogenesis, and developing therapeutic approaches and drugs to treat DS (Ballard et al., 2016). Moreover, due to the supernumerary copy of chromosome 21 (HSA21), some researchers hypothesized that the most dosage-sensitive genes in chromosome 21 are likely to contribute to the DS phenotype, also known as the “gene dosage effect” hypothesis (Korenberg et al., 1994). Some results support the “gene dosage effect” hypothesis that most of the Down's syndrome phenotypes are related to alterations in gene expression due to the extra chromosome 21 (HSA21). However, some researchers doubted that the DS phenotype could merely be explained by gene dosage effects (Jiang et al., 2013), the hypothesis of favor among medical geneticists. The factors influencing DS phenotypes include chromosome 21 DNA, functional elements and variability of chromosome 21, the variability of other chromosomes, chromatin structure, epigenetic modifications, stochastic events, and the environment (Antonarakis, 2017).
Nevertheless, very few studies on DS to date have examined alternative splicing (AS), a widespread regulatory mechanism of gene expression and makes one multi-exon protein-coding gene capable of producing more than one unique mature mRNA. AS provides transcriptional plasticity by controlling which RNA isoforms are expressed at a given time point in a given cell type. Alternative splicing affects about 95% of mammalian genes (Pan et al., 2008).
The emergence of induced pluripotent stem cells (iPSCs) provided important research tools for human disease research and drug screening (Takahashi et al., 2007; Yu et al., 2007). DS patient-derived induced pluripotent stem cells (DS hiPSCs) exhibit DS-like characteristics when they are induced to differentiate into somatic cells in vitro (Chou et al., 2012; Shi et al., 2012; Briggs et al., 2013) and are ideal models to study the genetic mechanisms underlying DS, as well as its associated diseases (Weick et al., 2013). Previous studies have shown that the proliferative ability of DS hiPSCs is much lower than that of normal human iPSCs (hiPSCs). This suggests that the extra chromosome 21 may also affect the biological characteristics of DS hiPSCs. However, the evidence at the molecular level is still lacking (Omori et al., 2017). In order to further explore the critical role that key genes may play in the proliferative and developmental differences between DS hiPSCs and normal hiPSCs, as well as to reveal the related molecular mechanism, we used whole genome expression profiles to analyze and screen the differentially expressed genes, and global aberrant alternative splicing events between DS hiPSCs and normal hiPSCs.
To delve into whether the extra chromosome 21 affects the maintenance of pluripotency of DS hiPSCs, we primarily checked the cell morphology of DS hiPSCs and normal hiPSCs. The results showed that the DS hiPSCs exhibited pluripotent stem cells' typical characteristics with a large nucleus and compact clone (Figure 1A). A strong positive expression for alkaline phosphatase staining (Figure 1B) and the results of cell immunofluorescence assays (Figure 1C) showed no significant difference in cell morphology and surface marker expression between DS hiPSCs and normal hiPSCs. Overall, these results indicated that the maintenance of pluripotency of DS hiPSCs was similar to that of normal hiPSCs and was not significantly affected by the redundant chromosome 21.
Figure 1. Pluripotency of hiPSCs, (A) Morphological observation of DS and N (Normal) hiPSCs (100×, all scale bars are 500 μm), (B) Alkaline phosphatase staining of DS and N (Normal) hiPSCs, (C) Immunofluorescence images showing DAPI (blue), LIN28A (green), and TRA1-60 (red) expression in DS and N (Normal) hiPSCs (100×, all scale bars are 500 μm).
As it is known that trisomy 21 causes alterations to both stem and precursor cells (Liu et al., 2015), it is also possible that the alteration of the proliferative ability of DS hiPSCs is caused by the differences in expression of genes and the aberrant AS events. To determine if this is the case, we used the GeneChip Human Transcriptome Array 2.0 (HTA 2.0) for the global gene analysis with hiPSCs from DS and healthy individuals. We examined differentially expressed genes (DEGs) in these groups focusing on specific transcripts with AS events. The quality control analyses of the HTA 2.0 data highlight the correct segregation of samples from each cell line (Figure 2).
Figure 2. Quality control for the HTA 2.0 data, (A) the correlation matrix heatmap of samples, (B) the heatmap of differential expression probe sets.
A more detailed analysis of HTA 2.0 datasets revealed, the total differentially expressed genes are 466 up-regulated and 722 down-regulated genes (in total 1,188 significantly differentially expressed genes) in DS hiPSCs, compared with normal hiPSCs (Figure 3). By summarizing the distribution of differentially expressed genes on each chromosome and the proportion of coding genes in the chromosome, we found that the proportion of up-regulated genes on chromosome 21 (5.20%) was significantly higher than that on the other chromosomes (0–1.79%), which showed a gene dosage effect of the genes on chromosome 21. This result is consistent with previous studies of DS somatic cells that demonstrated that the redundant chromosome 21 leads to gene dosage effects (Moldrich et al., 2007; Nawa et al., 2019).
Figure 3. Analysis of global gene expression profile, (A) Differentially expressed genes (DS vs. Normal iPSCs, red and green represent up-regulated and down-regulated genes, respectively), the expression analysis settings: Gene-Level Fold Change < −2 or > 2, Gene-Level P-Value < 0.01, (B) proportion of Coding and Non-Coding in the Up-Regulated and Down-Regulated genes, Filter criteria: Fold Change: > 2 or < −2, P-value: < 0.01, (C) Volcano map of differentially expressed genes (DS vs. Normal iPSCs, red and violet represent up-regulated and down-regulated genes, respectively).
Tables 1 and 2 list the top 10 up-regulated and down-regulated genes in DS hiPSCs sorted by the P-value, respectively. The up-regulated H1-6 is a member of the histone H1 family. The linker histone, H1, interacts with linker DNA between nucleosomes and functions in chromatin compaction into higher-order structures. The down-regulated H3C11 and H4C13 are members of the histone H3 and H4 families, respectively, which are essential nuclear proteins responsible for the nucleosome structure of the chromosomal fiber in eukaryotes. The up- and down-regulation of these genes may affect the compaction of chromatin and the higher-order structures of chromosomes in DS hiPSCs, leading to the instability of chromosomes and alternative modifications of histones resulting in the aberrant regulation of gene expression. DYRK1A, a gene coding for a kinase known to regulate splicing factors that maps to chromosome 21 (Qian et al., 2011), was identified as an up-regulated gene in DS hiPSCs. The aberrant expression of this regulator of splicing factors may lead to splicing changes in the trisomic cells.
We tested multiple splicing algorithms to identify differences in splicing between DS and normal hiPSCs and chose EventPointer (see Methods). Using this algorithm with the filter criteria: Exon Splicing Index > 2 or < −2 and Exon P-value < 0.01, we identified 1,862 annotated genes with splicing changes when comparing DS with normal hiPSCs, half of which are the Cassette Exon events and more than one third are Alternative 5′ Donor Site events and the Alternative 3′ Acceptor Site (Figure 4A).
Figure 4. Alternative splicing analysis, (A) Proportion of AS events, (B) Biological function enrichment analysis of the identified 1,862 annotated genes with splicing changes.
To explore the effect of splicing changes on the DS hiPSCs, we performed the gene ontology (GO) and KEGG signaling pathway analysis on all selected genes with alternative splicing events (Figure 4B). The results showed that 1,593 genes (87.8% of 1,862 annotated genes with splicing changes) are enriched in 188 biological processes, while 1,675 genes (92.3%) were enriched in 84 cellular components. We observed enrichment for 1,601 genes (88.2%) in 75 molecular functions, as well as 707 genes (39%) in 49 KEGG signaling pathways. The top 10 enrichment items are listed in Figure 4B. Fifty-six genes with splicing changes are enriched in the cell division process; this might be the driving force behind the phenomenon that the proliferative ability of DS hiPSCs and normal hiPSCs is different.
Figure 5 demonstrates the visualization of three genes as examples of splicing changes, namely the gene RPL39L (Ribosomal Protein L39 Like, OMIM: 607547), PARP2 (Poly(ADP-Ribose) Polymerase 2, OMIM: 607725), and FN1 (Fibronectin 1, OMIM: 135600).
Figure 5. Visualization of the alternative splice events of three selected genes, (A) RPL39L, (B) PARP2 and (C) FN1.
To explore the effect of differentially expressed genes on the DS hiPSCs, we further performed GO functional enrichment and KEGG signaling pathway analysis of 466 up-regulated and 722 down-regulated genes, respectively. The results show that 466 up-regulated genes are enriched in 64 biological processes, 34 cellular components, 18 molecular functions, and 10 KEGG signaling pathways. The 722 down-regulated genes are enriched in 50 biological processes, 27 cellular components, 16 molecular functions, and 8 KEGG signaling pathways. The top 10 enrichment items in up- and down-regulated genes are listed in Tables 3 and 4, respectively. Eight up-regulated genes were significantly enriched in the negative regulation of growth, which may be related to somatic cells' lower proliferative ability and stem cells from patients with DS (Kimura et al., 2005). Overexpression of genes related to negative growth regulation may inhibit cell proliferation, suggesting that the proliferation of DS hiPSCs may also be impaired. Nine up-regulated genes are significantly enriched in proteins targeting the mitochondria, which may affect ATP synthesis by damaging mitochondrial function, resulting in the inhibition of select cell functions (Valenti et al., 2018). Fifteen up-regulated genes were significantly enriched in nucleosome assembly, implying that the redundant chromosome 21 may increase chromosome synthesis and assembly stress. Twenty-seven down-regulated genes were significantly enriched in cell adhesion. The impaired cell adhesion ability affects cell growth and neural cell migration during embryonic development in DS patients (Huo et al., 2018). In nervous system development there were 17 down-regulated genes that were enriched, suggesting that the molecular regulation might be abnormal before the differentiation of DS neurocytes (Liu et al., 2015). Moreover, in focal adhesion there were 16 down-regulated genes that were significantly enriched. This is consistent with the enrichment in cell adhesion of the GO analysis. Another 16 down-regulated genes were enriched in the PI3K Akt signaling pathway. The hyperactivity of this pathway promotes carcinogenesis (Yang et al., 2019). The silencing of this signaling pathway in DS hiPSCs may be the critical cause of the low incidence of solid tumors in DS patients. The disorder of the whole-genome expression profile indicates that other biological characteristics of DS hiPSCs, such as proliferation and cell adhesion, are also affected.
Table 3. GO and KEGG analysis of global up-regulated genes (Sort by P-value, and only the top ten GO items/pathways were listed in the table if there were more than 10).
Table 4. GO and KEGG analysis of global down-regulated genes (Sort by P-value, and only the top ten GO items/pathways were listed in the table if there were more than 10).
To test the reliability of gene expression microarray results, several genes were selected and were validated by real time qPCR. The relative quantifications of the expression of these genes in DS hiPSCs and normal hiPSCs are represented in Figure 6.
Figure 6. qRT-PCR of (A) H1-6, (B) FN1, (C) H4C13, and (D) RPL13 in normal iPSCs (N) and DS hiPSCs (DS). Data are represented as mean ± s.e.m. * stand for P < 0.05 and *** for P < 0.001 by one-way followed with Tukeys test from n = 3.
In compare with normal hiPSCs, DS hiPSCs exhibited a 2.1-fold increase of H1-6 (Figure 6A), a linker histone, which interacts with DNA between nucleosomes and plays an important role in the compaction of chromatin into higher order structures. In DS hiPSCs, the expressions of FN1 (Figure 6B) and H4C13 (Figure 6C) were reduced 4- and 2.5-fold, respectively. The expression of the gene RPL13 (Figure 6D) was not significant changed in DS hiPSCs in comparison with the normal hiPSCs. All of these results are consistent with the DEG analysis of the microarray dataset.
Although RNA-seq, a preferred platform for studying differentially expressed genes and alternative splicing nowadays, has some inherent advantages in comparison with microarrays, such as the ability to identify novel exons and splice junctions in an unbiased manner, the HTA 2.0 platform can nevertheless detect more weakly expressed events, which are missed in the RNA-seq results (Fumagalli et al., 2014; Wang et al., 2014; Romero et al., 2018). Furthermore, the modern microarrays, HTA 2.0, can still outperform RNA-seq for the analysis of gene expression in terms of cost, reproducibility and time as well as memory resources for treating data (Nazarov et al., 2017; Romero et al., 2018). For these reasons, we have chosen the HAT 2.0 platform as the major research approach for the AS studies. The reliability of gene expression microarray results was tested by the relative quantification of the expression of several selected genes. All of these genes showed the same change direction as they were exhibited in the DEG analysis of the microarray dataset (Figure 6).
The triploidy of chromosome 21 is the proximal cause of DS. Multi-omics studies of DS somatic cells revealed that the transcriptome and epigenetic modification disorders, leads to the disequilibrium of disease-related gene expression as a possible underlying mechanism contributing to the comorbidities associated with DS (Letourneau et al., 2014; Waugh et al., 2019). Therefore, the studies on DS's molecular regulatory mechanism can provide novel insight that could improve the clinical management of these patients. In this study, we examined differentially expressed genes (DEGs) in hiPSCs derived from DS and healthy individuals and focused on specific transcripts with AS. 1,188 differentially expressed genes and 1,862 annotated genes with splicing changes were analyzed by GO function enrichment and KEGG signaling pathway analysis, in order to dissect out the cause of the difference between DS and normal hiPSCs.
To more accurately analyze the possible dosage effects of chromosome 21 genes on DS hiPSCs, those genes determined to be up-regulated were analyzed. The biological functions and KEGG signaling pathways of the up-regulated genes on chromosome 21 suggested that dysfunctional neurodevelopment and metabolisms must have occurred in the early embryonic development of DS patients. However, the gene dosage effect can not fully explain the pathogenesis of the various comorbidities of DS patients. Bioinformatics analysis of differentially expressed genes in the whole-genome showed that the up-regulated genes were primarily involved in cell growth regulation, nucleosome assembly, and chromosome separation, suggesting that the formation and separation blockage of chromosome 21 might be the main reason for the abnormal cell cycle. KEGG signaling pathway analysis showed that the down-regulated genes are enriched in focal adhesion and extracellular matrix (ECM) receptor interaction, indicating that the migration and adhesion of DS hiPSCs may be impaired, and migration and colonization of both nervous and reproductive systems occurred during early embryonic development (Huo et al., 2018). In addition, abnormal cell adhesion was also found in the bioinformatics analysis of other DS hiPSCs (Hibaoui et al., 2014; Gonzales et al., 2018). These results are consistent with the other studies, in which the RNA-seq are used as the major research platform (Hibaoui et al., 2014; Gonzales et al., 2018; Perepitchka et al., 2020).
Key genes identified from the HTA 2.0 dataset analysis are histone coding genes involved in nucleosome formation and gene expression regulation. It appeared that alternation of histone-related genes, such as mutations in histone-modified genes, can lead to disorders related to DS, such as congenital heart disease (Zaidi et al., 2013). Some of the key genes identified in this study are involved in disorders of neurodevelopment and neurogenesis. For example, H3C2 plays an essential role in brain development in early embryonic development (Ren and van Nocker, 2016), and H2BC12 is involved in Alzheimer's disease (Pedrero-Prieto et al., 2019). H1-2, on the other hand, plays a critical role in the stabilization of chromatin and is involved in cell cycles, apoptosis induced by DNA damage, and the stabilization of autophagy protein and fibrin (Konishi et al., 2003; Sancho et al., 2008; Roque et al., 2015; Wang et al., 2017). The up-regulated H1-6 and down-regulated H3C11 and H4C13 are also essential for the stabilization and structure of chromatin and chromosomes. The alteration of the expression of these genes could also lead to variations of chromatin states and chromosome structure. These genes are worthy of further analysis to determine the reasonable correlations to pathological knowledge.
We observed trisomy-dependent splicing changes in the DS hiPSCs. Our identification of splicing changes resonance with the alternative splicing events in a selected set of genes in fetal DS tissue that has been previously reported (Toiber et al., 2010). AS in DS endothelial progenitor cells has also been analyzed via RNA-seq, although no confirmatory studies have yet been performed (Costa et al., 2011). The large majority of alternative splicing events we identified occur in genes located outside of chromosome 21, implying that these AS differences were not directly affected by gene dosage. It is more likely that altered expression or altered splicing activity could cause the splicing dysregulation in DS hiPSCs. Alternatively, up-regulation of the chromosome 21 gene, such as DYRK1A, a kinase known to regulate splicing factors (Qian et al., 2011), could contribute to the alternative splicing events we identified. It has been reported that over-expression of DYRK1A in mice led to mimicry of splicing aberrations in DS (Toiber et al., 2010). The role of DYRK1A or the other specific splicing factors in the alternative splicing changes we identified will require additional studies. The gene dosage effect and expression levels of these genes should be normalized in the DS hiPSCs backgrounds.
DS hiPSC (ATCC® ACS-1003™) and normal hiPSC (ATCC® ACS-1011™) were from the ATCC (American Type Culture Collection) cell bank. HiPSCs were cultured using Gibco's StemFlex™ medium (ThermoFisher Scientific, USA). The petri dishes were pre-treated with Matrigel, and the medium was changed daily. After culturing for 4–5 days, the iPSCs were digested, and the suspended cell clusters were collected and subcultured at a ratio of 1:6 at 37°C, 5% CO2 (Aalders et al., 2019).
After removing the culture medium, hiPSCs were washed twice with PBS, adding blocking solution (PBS solution containing 0.6% BSA) within 30 min, a membrane breaker (PBS solution containing 0.02% Triton X-100) was added to break the cell membrane. The diluted primary antibody (1:250) [TRA1-60 primary antibody (ThermoFisher Scientific, USA) and LIN28A primary antibody (Cell Signaling, USA)] were added and incubated at 4°C overnight. The cells were washed twice with PBS the next day and then incubated with the fluorescent secondary antibody (1:500) [FITC-labeled goat anti-rabbit IgG (H + L), Cy3 labeled goat anti-mouse IgG (H + L)], respectively, at room temperature, avoiding light for 2 h. Finally, the anti-fluorescence quenching mount solution (containing DAPI) was added and incubated at room temperature for 10 min before the cells were observed under a fluorescence microscope (Weltner et al., 2018).
According to the instructions of the BCIP/NBT alkaline phosphatase staining kit's manufacturer (Beyotime Biotechnology, Shanghai, China), the protocol involved mixing 3 mL of alkaline phosphatase staining buffer with 10 μL of BCIP solution (300×) and 20 μL of NBT solution (150×) to prepare the working solution. Cells in 6-well plate were washed twice with PBS, then an appropriate amount of working solution was added to a single well of the 6-well plate while avoiding light exposure for 5–30 min until the color displayed to the expected depth. The working solution was then removed, and the cells were washed twice with distilled water to stop coloring. Before the photographs were taken, the stained cells were dried at room temperature and keep away from light.
DS hiPSC and normal hiPSC were seeded in a 6-well plate, each cultured for 3 days, and then digested to harvest about 5 × 105 cells. The experiment was repeated three times. The total RNA was extracted with TRIzol™ reagent (ThermoFisher Scientific, USA) and was first detected by the Agilent 2100 bioanalyzer with the Agilent RNA 6000 Nano Kit (Agilent Technologies, Waldbronn, Germany) according to the instruction of the manufacture. Total RNAs with an RNA integrity index (RIN) >7.0 were used for subsequent experiments.
By using WT Amplification Kit Module 1 and WT Amplification Kit Module 2 in the GeneChip™ WT PLUS Kit (ThermoFisher Scientific, USA), 100 ng of total RNA as input was performed in vitro transcription (IVT) to synthesize cRNA, and then reverse transcription and purification to obtain single-stranded cDNA (sscDNA). The GeneChip™ WT end labeling kit was used to fragment and label sscDNA, which was hybridized with the human transcriptome array (HTA 2.0), and the hybridization signals were detected on a chip scanner to obtain CEL files for DS and normal hiPSCs.
The SuperScript™ IV reverse transcriptase (Invitrogen™, USA) was used for the synthesis of first stand cDNA from the isolated RNA. 1 μg of total RNAs were reverse transcribed according to the manufacturer's instructions. cDNA was real time polymerase chain (PCR) amplified in a LightCycler® 96 System (Roche, USA) using the FastStart Essential DNA Green Master (Roche, USA). The LightCycler® 96 SW 1.1 software was used for raw data collection and gene expression comparisons (2−ΔΔCT method). The R packages “Rmisc” and “multcomp” are used for the data analysis and “ggplot2” for the visualization of the results.
All data were reprocessed from raw images. Signal intensities, quality control, data normalization, and gene expression values for these samples were processed together with the RMA processing algorithm using the “oligo” package in R (Carvalho and Irizarry, 2010). The DEG analysis was performed with Fold change (FC) < −2 or > 2, P-value < 0.01.
The alternative splicing events analysis was performed using the “EventPointer” package in R (Romero et al., 2016) with the splicing index < −2 or > 2 and exon-Level P-value < 0.01.
GO enrichment was performed using DAVID (Huang et al., 2009a,b) (http://david.abcc.ncifcrf.gov/). A hypergeometric test with the Benjamini and Hochberg false discovery rate (FDR) was performed using the default parameters to adjust the P-value (Benjamini and Hochberg, 1995).
The datasets presented in this study can be found in online repositories. The names of the repository/repositories and accession number(s) can be found at: https://www.ncbi.nlm.nih.gov/geo/query/acc.cgi?acc=GSE168111.
YW, QM, YZe and FZ were the main contributors to designing experiments. YW, QM, and FZ interpreted results, wrote and revised the manuscript. ZL and QM performed microarray experiments and analyzed data. GY, LC and YZha performed cell culture experiments and analyzed data. FY analyzed microarray data. All authors agree to be accountable for the content of the work.
The research was funded by National Key R&D Program of China (2016YFC1000503 and 2019YFA0801402) and Key disciplines of top priority in Shanghai (2017ZZ02019).
The authors declare that the research was conducted in the absence of any commercial or financial relationships that could be construed as a potential conflict of interest.
Aalders, J., Van den Vreken, N., Popovic, M., Mishra, S., Heindryckx, B., and van Hengel, J. (2019). Robust protocol for feeder-free adaptation of cryopreserved human pluripotent stem cells. In Vitro Cell Dev. Biol. Anim. 55, 777–783. doi: 10.1007/s11626-019-00413-9
Antonarakis, S. E. (2017). Down syndrome and the complexity of genome dosage imbalance. Nat. Rev. Genet. 18, 147–163. doi: 10.1038/nrg.2016.154
Ballard, C., Mobley, W., Hardy, J., Williams, G., and Corbett, A. (2016). Dementia in Down's syndrome. Lancet Neurol. 15, 622–636. doi: 10.1016/S1474-4422(16)00063-6
Benjamini, Y., and Hochberg, Y. (1995). Controlling the false discovery rate: a practical and powerful approach to multiple testing. J. R. Stat. Soc. B Methodol. 57, 289–300. doi: 10.1111/j.2517-6161.1995.tb02031.x
Briggs, J. A., Sun, J., Shepherd, J., Ovchinnikov, D. A., Chung, T. L., Nayler, S. P., et al. (2013). Integration-free induced pluripotent stem cells model genetic and neural developmental features of down syndrome etiology. Stem Cells 31, 467–478. doi: 10.1002/stem.1297
Carvalho, B. S., and Irizarry, R. A. (2010). A framework for oligonucleotide microarray preprocessing. Bioinformatics 26, 2363–2367. doi: 10.1093/bioinformatics/btq431
Chou, S. T., Byrska-Bishop, M., Tober, J. M., Yao, Y., Vandorn, D., Opalinska, J. B., et al. (2012). Trisomy 21-associated defects in human primitive hematopoiesis revealed through induced pluripotent stem cells. Proc. Natl. Acad. Sci. U.S.A. 109, 17573–17578. doi: 10.1073/pnas.1211175109
Costa, V., Angelini, C., D'Apice, L., Mutarelli, M., Casamassimi, A., Sommese, L., et al. (2011). Massive-scale RNA-Seq analysis of non ribosomal transcriptome in human trisomy 21. PLoS ONE 6:e18493. doi: 10.1371/journal.pone.0018493
Fumagalli, D., Blanchet-Cohen, A., Brown, D., Desmedt, C., Gacquer, D., Michiels, S., et al. (2014). Transfer of clinically relevant gene expression signatures in breast cancer: from affymetrix microarray to illumina RNA-sequencing technology. BMC Genomics 15:1008. doi: 10.1186/1471-2164-15-1008
Gonzales, P. K., Roberts, C. M., Fonte, V., Jacobsen, C., Stein, G. H., and Link, C. D. (2018). Transcriptome analysis of genetically matched human induced pluripotent stem cells disomic or trisomic for chromosome 21. PLoS ONE 13:e0194581. doi: 10.1371/journal.pone.0194581
Hibaoui, Y., Grad, I., Letourneau, A., Sailani, M. R., Dahoun, S., Santoni, F. A., et al. (2014). Modelling and rescuing neurodevelopmental defect of Down syndrome using induced pluripotent stem cells from monozygotic twins discordant for trisomy 21. EMBO Mol. Med. 6, 259–277. doi: 10.1002/emmm.201302848
Huang, D. W., Sherman, B. T., and Lempicki, R. A. (2009a). Bioinformatics enrichment tools: paths toward the comprehensive functional analysis of large gene lists. Nucleic Acids Res. 37, 1–13. doi: 10.1093/nar/gkn923
Huang, D. W., Sherman, B. T., and Lempicki, R. A. (2009b). Systematic and integrative analysis of large gene lists using DAVID bioinformatics resources. Nat. Protoc. 4, 44–57. doi: 10.1038/nprot.2008.211
Huo, H. Q., Qu, Z. Y., Yuan, F., Ma, L., Yao, L., Xu, M., et al. (2018). Modeling Down syndrome with patient iPSCs reveals cellular and migration deficits of GABAergic neurons. Stem Cell Rep. 10, 1251–1266. doi: 10.1016/j.stemcr.2018.02.001
Jiang, J., Jing, Y., Cost, G. J., Chiang, J. C., Kolpa, H. J., Cotton, A. M., et al. (2013). Translating dosage compensation to trisomy 21. Nature 500, 296–300. doi: 10.1038/nature12394
Kimura, M., Cao, X., Skurnick, J., Cody, M., Soteropoulos, P., and Aviv, A. (2005). Proliferation dynamics in cultured skin fibroblasts from Down syndrome subjects. Free Radic. Biol. Med. 39, 374–380. doi: 10.1016/j.freeradbiomed.2005.03.023
Konishi, A., Shimizu, S., Hirota, J., Takao, T., Fan, Y., Matsuoka, Y., et al. (2003). Involvement of histone H1.2 in apoptosis induced by DNA double-strand breaks. Cell 114, 673–688. doi: 10.1016/S0092-8674(03)00719-0
Korenberg, J. R., Chen, X. N., Schipper, R., Sun, Z., Gonsky, R., Gerwehr, S., et al. (1994). Down syndrome phenotypes: the consequences of chromosomal imbalance. Proc. Natl. Acad. Sci. U.S.A. 91, 4997–5001. doi: 10.1073/pnas.91.11.4997
Letourneau, A., Santoni, F. A., Bonilla, X., Sailani, M. R., Gonzalez, D., Kind, J., et al. (2014). Domains of genome-wide gene expression dysregulation in Down's syndrome. Nature 508, 345–350. doi: 10.1038/nature13200
Liu, W., Zhou, H., Liu, L., Zhao, C., Deng, Y., Chen, L., et al. (2015). Disruption of neurogenesis and cortical development in transgenic mice misexpressing Olig2, a gene in the Down syndrome critical region. Neurobiol. Dis. 77, 106–116. doi: 10.1016/j.nbd.2015.02.021
Moldrich, R. X., Dauphinot, L., Laffaire, J., Rossier, J., and Potier, M. C. (2007). Down syndrome gene dosage imbalance on cerebellum development. Prog. Neurobiol. 82, 87–94. doi: 10.1016/j.pneurobio.2007.02.006
Nawa, N., Hirata, K., Kawatani, K., Nambara, T., Omori, S., Banno, K., et al. (2019). Elimination of protein aggregates prevents premature senescence in human trisomy 21 fibroblasts. PLoS ONE 14:e0219592. doi: 10.1371/journal.pone.0219592
Nazarov, P. V., Muller, A., Kaoma, T., Nicot, N., Maximo, C., Birembaut, P., et al. (2017). RNA sequencing and transcriptome arrays analyses show opposing results for alternative splicing in patient derived samples. BMC Genomics 18:443. doi: 10.1186/s12864-017-3819-y
Omori, S., Tanabe, H., Banno, K., Tsuji, A., Nawa, N., Hirata, K., et al. (2017). A pair of maternal chromosomes derived from meiotic nondisjunction in trisomy 21 affects nuclear architecture and transcriptional regulation. Sci. Rep. 7:764. doi: 10.1038/s41598-017-00714-7
Pan, L., Woodruff, E., Liang, P., and Broadie, K. (2008). Mechanistic relationships between Drosophila fragile X mental retardation protein and metabotropic glutamate receptor A signaling. Mol. Cell. Neurosci. 37, 747–760. doi: 10.1016/j.mcn.2008.01.003
Pedrero-Prieto, C. M., Flores-Cuadrado, A., Saiz-Sanchez, D., Ubeda-Banon, I., Frontinan-Rubio, J., Alcain, F. J., et al. (2019). Human amyloid-beta enriched extracts: evaluation of in vitro and in vivo internalization and molecular characterization. Alzheimers Res. Ther. 11:56. doi: 10.1186/s13195-019-0513-0
Perepitchka, M., Galat, Y., Beletsky, I. P., Iannaccone, P. M., and Galat, V. (2020). Down syndrome iPSC model: endothelial perspective on tumor development. Oncotarget 11, 3387–3404. doi: 10.18632/oncotarget.27712
Qian, W., Liang, H., Shi, J., Jin, N., Grundke-Iqbal, I., Iqbal, K., et al. (2011). Regulation of the alternative splicing of tau exon 10 by SC35 and Dyrk1A. Nucleic Acids Res. 39, 6161–6171. doi: 10.1093/nar/gkr195
Ren, M., and van Nocker, S. (2016). In silico analysis of histone H3 gene expression during human brain development. Int. J. Dev. Biol. 60, 167–173. doi: 10.1387/ijdb.150334sv
Romero, J. P., Muniategui, A., De Miguel, F. J., Aramburu, A., Montuenga, L., Pio, R., et al. (2016). EventPointer: an effective identification of alternative splicing events using junction arrays. BMC Genomics 17:467. doi: 10.1186/s12864-016-2816-x
Romero, J. P., Ortiz-Estévez, M., Muniategui, A., Carrancio, S., de Miguel, F. J., Carazo, F., et al. (2018). Comparison of RNA-seq and microarray platforms for splice event detection using a cross-platform algorithm. BMC Genomics 19:703. doi: 10.1186/s12864-018-5082-2
Roque, A., Sortino, R., Ventura, S., Ponte, I., and Suau, P. (2015). Histone H1 favors folding and parallel fibrillar aggregation of the 1–42 amyloid-beta peptide. Langmuir 31, 6782–6790. doi: 10.1021/la504089g
Sancho, M., Diani, E., Beato, M., and Jordan, A. (2008). Depletion of human histone H1 variants uncovers specific roles in gene expression and cell growth. PLoS Genet. 4:e1000227. doi: 10.1371/journal.pgen.1000227
Shi, Y., Kirwan, P., Smith, J., MacLean, G., Orkin, S. H., and Livesey, F. J. (2012). A human stem cell model of early Alzheimer's disease pathology in Down syndrome. Sci. Transl. Med. 4:124ra29. doi: 10.1126/scitranslmed.3003771
Takahashi, K., Tanabe, K., Ohnuki, M., Narita, M., Ichisaka, T., Tomoda, K., et al. (2007). Induction of pluripotent stem cells from adult human fibroblasts by defined factors. Cell 131, 861–872. doi: 10.1016/j.cell.2007.11.019
Toiber, D., Azkona, G., Ben-Ari, S., Torán, N., Soreq, H., and Dierssen, M. (2010). Engineering DYRK1A overdosage yields Down syndrome-characteristic cortical splicing aberrations. Neurobiol. Dis. 40, 348–359. doi: 10.1016/j.nbd.2010.06.011
Valenti, D., Braidy, N., De Rasmo, D., Signorile, A., Rossi, L., Atanasov, A. G., et al. (2018). Mitochondria as pharmacological targets in Down syndrome. Free Radic. Biol. Med. 114, 69–83. doi: 10.1016/j.freeradbiomed.2017.08.014
Wang, C., Gong, B., Bushel, P. R., Thierry-Mieg, J., Thierry-Mieg, D., Xu, J., et al. (2014). The concordance between RNA-seq and microarray data depends on chemical treatment and transcript abundance. Nat. Biotechnol. 32, 926–932. doi: 10.1038/nbt.3001
Wang, W., Wang, Q., Wan, D., Sun, Y., Wang, L., Chen, H., et al. (2017). Histone HIST1H1C/H1.2 regulates autophagy in the development of diabetic retinopathy. Autophagy 13, 941–954. doi: 10.1080/15548627.2017.1293768
Waugh, K. A., Araya, P., Pandey, A., Jordan, K. R., Smith, K. P., Granrath, R. E., et al. (2019). Mass cytometry reveals global immune remodeling with multi-lineage hypersensitivity to type I interferon in Down syndrome. Cell Rep. 29, 1893–1908.e4. doi: 10.1016/j.celrep.2019.10.038
Weick, J. P., Held, D. L., Bonadurer, G. F., Doers, M. E., Liu, Y., Maguire, C., et al. (2013). Deficits in human trisomy 21 iPSCs and neurons. Proc. Natl. Acad. Sci. U.S.A. 110, 9962–9967. doi: 10.1073/pnas.1216575110
Weijerman, M. E., and de Winter, J. P. (2010). Clinical practice. The care of children with Down syndrome. Eur. J. Pediatr. 169, 1445–1452. doi: 10.1007/s00431-010-1253-0
Weltner, J., Balboa, D., Katayama, S., Bespalov, M., Krjutskov, K., Jouhilahti, E. M., et al. (2018). Human pluripotent reprogramming with CRISPR activators. Nat. Commun. 9:2643. doi: 10.1038/s41467-018-05067-x
Yang, Q., Jiang, W., and Hou, P. (2019). Emerging role of PI3K/AKT in tumor-related epigenetic regulation. Semin. Cancer Biol. 59, 112–124. doi: 10.1016/j.semcancer.2019.04.001
Yu, J., Vodyanik, M. A., Smuga-Otto, K., Antosiewicz-Bourget, J., Frane, J. L., Tian, S., et al. (2007). Induced pluripotent stem cell lines derived from human somatic cells. Science 318, 1917–1920. doi: 10.1126/science.1151526
Keywords: Down's syndrome, alternative splicing, iPSCs, gene regulation, differentially expressed genes
Citation: Wang Y, Li Z, Yang G, Cai L, Yang F, Zhang Y, Zeng Y, Ma Q and Zeng F (2021) The Study of Alternative Splicing Events in Human Induced Pluripotent Stem Cells From a Down's Syndrome Patient. Front. Cell Dev. Biol. 9:661381. doi: 10.3389/fcell.2021.661381
Received: 30 January 2021; Accepted: 16 March 2021;
Published: 30 September 2021.
Edited by:
Desheng Liang, Central South University, ChinaReviewed by:
Fu Xiong, Southern Medical University, ChinaCopyright © 2021 Wang, Li, Yang, Cai, Yang, Zhang, Zeng, Ma and Zeng. This is an open-access article distributed under the terms of the Creative Commons Attribution License (CC BY). The use, distribution or reproduction in other forums is permitted, provided the original author(s) and the copyright owner(s) are credited and that the original publication in this journal is cited, in accordance with accepted academic practice. No use, distribution or reproduction is permitted which does not comply with these terms.
*Correspondence: Qingwen Ma, cXdtYTEyMTNAMTYzLmNvbQ==; Fanyi Zeng, ZnplbmdAVklQLjE2My5jb20=
†These authors have contributed equally to this work and share first authorship
Disclaimer: All claims expressed in this article are solely those of the authors and do not necessarily represent those of their affiliated organizations, or those of the publisher, the editors and the reviewers. Any product that may be evaluated in this article or claim that may be made by its manufacturer is not guaranteed or endorsed by the publisher.
Research integrity at Frontiers
Learn more about the work of our research integrity team to safeguard the quality of each article we publish.