- 1Lianyungang Second People’s Hospital, Lianyungang, China
- 2School of Systems Biology, George Mason University, Fairfax, VA, United States
- 3Department of Psychiatry, First Hospital/First Clinical Medical College of Shanxi Medical University, Taiyuan, China
- 4Research Centre for Medical Genetics, Moscow, Russia
- 5Moscow Institute of Physics and Technology, Dolgoprudny, Russia
Pathogenesis of chronic obstructive pulmonary disease (COPD) is dependent on chronic inflammation and is hypothesized to represent organ-specific senescence phenotype. Identification of senescence-associated gene drivers for the development of COPD is warranted. By employing automated pipeline, we have compiled lists of the genes implicated in COPD (N = 918) and of the genes changing their activity along with cell senescence (N = 262), with a significant (p < 7.06e–60) overlap between these datasets (N = 89). A mega-analysis and a partial mega-analysis were conducted for gene sets linked to senescence but not yet to COPD, in nine independent mRNA expression datasets comprised of tissue samples of COPD cases (N = 171) and controls (N = 256). Mega-analysis of expression has identified CD38 and TNFRSF12A (p < 2.12e–8) as genes not yet explored in a context of senescence–COPD connection. Functional pathway enrichment analysis allowed to generate a model, which explains accelerated aging phenotypes previously observed in COPD patients. Presented results call for investigation of the role of TNFRSF12A/CD38 balance in establishing a vicious cycle of unresolvable tissue remodeling in COPD lungs.
Introduction
Aging is a fundamental biological process accompanied by changes in the structure and functions of vital organs, including the lungs. Chronic obstructive pulmonary disease (COPD) is associated with persistent airway inflammation and manifests as a steady decline in the lung function, increase in gas trapping, enlargement of the distal air spaces, and the loss of elastic recoil (Vestbo, 2014). According to the 2007–2012 NHANES survey, approximately 5.2% of US adults aged 40–79 were diagnosed with COPD (Tilert et al., 2018).
Current understanding of the pathophysiology of COPD emphasizes pivotal role of chronic inflammation, which is evident from an increase in neutrophils, macrophages, and CD8+ T-cell counts throughout the airways, and related release of inflammatory mediators including IL−8, TNF−α, leukotrienes, and reactive oxygen species (ROS) (Barnes, 2014). It is generally accepted that both cellular and molecular pro-inflammatory players act in concert to form an inflammation promoting feedback loop. Some experts, however, suggest that the confinement of the process of inflammation in the airway wall, where the COPD-related remodeling occurs, is debatable (Eapen et al., 2017). Moreover, epidemiological studies point toward a strong systemic component of COPD, with is often accompanied by muscle wasting, cachexia, and cardiovascular involvement (Rubinsztajn et al., 2019). This is one of the reasons why COPD is now considered as an example of organ-specific accelerated aging, with increase in oxidative stress and alteration in the extracellular matrix leading to the development of senescent cell phenotypes (Mercado et al., 2015). Previous gene expression studies produced a list of hundreds of genes, which are both linked to COPD and aging promoting (Brandsma et al., 2017; de Vries et al., 2018), paving a solid foundation to explore the association between COPD and aging at a genetic level. Here, we hypothesize that genes with activity or expression levels increased in aged individuals may also play roles for the etiology of COPD.
To test this hypothesis, we conducted large-scale literature-based disease–gene relationship analysis to compile the lists of genes implicated in COPD and, separately, in senescence, and subtracted the genes previously implicated in both of these phenotypes. For the genes previously identified as senescence-related but not yet highlighted by any COPD studies, a mega-analysis and a partial mega-analysis were completed to evaluate their expression patterns in multiple independently collected COPD-related transcriptomic datasets. Functional pathway enrichment analysis allowed us to generate a model that centers on two novel COPD genes, CD38 and TNFRSF12A, and explains the accelerated aging phenotypes previously observed in COPD patients.
Materials and Methods
The workflow was organized as follows. First, a large-scale literature mining effort for COPD-related and aging-promoting related gene sets were undertaken in the Pathway Studio environment; these gene sets were compared to identify common genes and age-promoting specific genes. Then, for each gene from the list implicated in aging alone, a mega-analysis, followed by a partial mega-analysis were conducted using nine publicly available COPD expression datasets retrieved from Gene Expression Omnibus (GEO)1. For these genes that showed a significant change in expression across analyzed datasets, a Gene Set Enrichment Analysis (GSEA) and literature-based functional pathway analysis were conducted. In addition, possible influences of sample size, population region, and study date on the gene expression levels in COPD were investigated by a multiple linear regression (MLR) model.
Extraction of Relation Data From Literature
Relation data for genes previously associated with either senescence or COPD were extracted from existing literature in the Pathway Studio environment2 and arranged in the database Aging_COPD, hosted at http://database.gousinfo.com. The downloadable form in Excel is available at http://gousinfo.com/database/Data_Genetic/Aging_COPD.xlsx. Beside the list of analyzed genes (Aging_COPD→Aging_alone genes, Aging_COPD→COPD_alone genes, and Aging_COPD→Common genes), supporting references for each disease-gene relation were retrieved (Aging_COPD→Ref for Aging_alone genes, Aging_COPD→Ref for COPD_alone genes, and Aging_COPD→Ref for Common genes) to include both titles of respective papers and particular sentences describing identified relationships. The database allows automated mining for supportive statements underlining the association of each candidate gene with senescence and/or with COPD.
Selection of Gene Expression Datasets
To compile the list of gene expression datasets, publicly available, the GEO database was searched using the keyword “chronic obstructive pulmonary disease,” which has returned 171 entries. Datasets were extracted with no selection bias and covered the entire GEO contents. The following standards were applied for the further filtering: (1) The organism is Homo sapiens; (2) The data type is RNA expression; (3) The sample size is no less than 10; and (4) the study design is case control. Finally, a total of nine datasets remained available for the mega-analysis of expression patterns (Table 1). For each dataset, raw data files rather than the reported research outputs were used to perform the analysis.
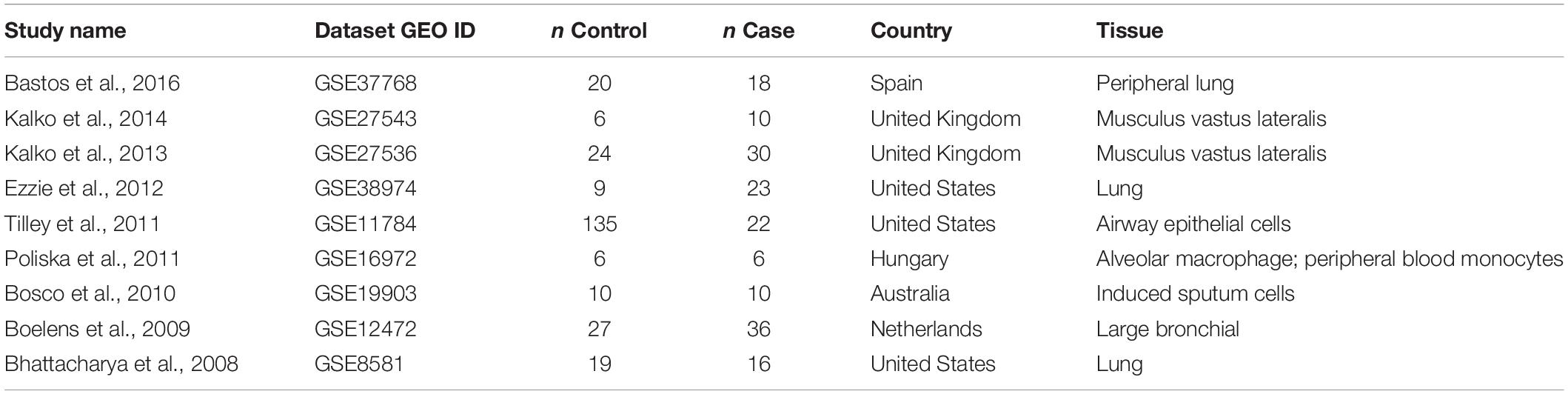
Table 1. Datasets utilized for chronic obstructive pulmonary disease (COPD)-senescence expression mega-analysis.
Mega-Analysis and Partial Mega-Analysis of Expression Datasets
The expression data were normalized and log2-transformed. Mega-analysis allows pooling of individual-level biological endpoint data across datasets by introducing appropriate correction for between-study variations selected by modeling (Seifuddin et al., 2013). Both the fixed-effect model and random-effect model (Borenstein et al., 2010) were employed to study the effect size of senescence-related genes on COPD. For each expression dataset, the log fold change (LFC) was calculated for the COPD samples and used as the index of effect size. Results from both mega-analysis models were compared. In order to study the variance within and between different datasets, the heterogeneity of the mega-analysis was assessed. In case when total variance Q was equal to, or smaller than, the expected between-study variance df, the statistic ISq = 100% × (Q – df)/Q was set to 0, and a fixed-effect model was selected for the mega-analysis. Otherwise, a random-effect model was selected. The Q–p represents the probability that the total variance is explained by within-study variance only.
To discover the genes significantly altered in some, but not all studied datasets, we performed a partial mega-analysis, where the top 50% datasets were employed for the mega-analysis of each gene. The “Top datasets” were defined for each gene individually as datasets demonstrating larger absolute value of effect size than the rest of the datasets. Analyses were conducted using MATLAB (R2017a) mega-analysis package.
Results from both mega-analysis and partial mega-analysis were compared to identify significant genes according to the following the criteria, p < 1.00e–7 and effect size (LFC) > 0.49 or < −0.74. When a gene presented an effect size LFC > 0.49 or < −0.74 in the mega-analysis, it means that the change in the expression level of the gene had increased by more than 40%, or decreased by more than 40%. While we present all the mega-analysis results in the Aging_COPD→Mega-analysis and Aging_COPD→Partial-Meta, the discussion will be focused on those genes that satisfy the significance criteria outlined above.
Gene Set Enrichment Analysis and Shortest Path Analysis
To gain functional insights into the set of genes previously described as involved in senescence and in COPD and, separately, the set of genes showing significance in the mega-analysis of senescence-related genes, which were not yet described as COPD contributors, the GSEA has been conducted in the Pathway Studio environment. The GSEA results were reported with enrichment p-values corrected using the Bejnamini–Hochberg false discovery ratio (FDR) procedure (Reiner et al., 2003). In addition to the GSEA, for each gene set, a literature-based functional pathway analysis was conducted using the “Shortest Path” module of the Pathway Studio3.
Multiple Linear Regression Analysis
A MLR analysis was employed to study the possible influence of the following three factors on the gene expression changes: sample size, population region, and study date. Values of p and 95% confidence interval (CI) were reported for each of these factors. The analysis was performed in MATLAB (R 2017a) with the “regress” statistical analysis package.
Results
Many Senescence-Associated Genes Are Not yet Studied in COPD Context
Pathway Studio-guided literature data mining for the genes associated with aging and senescence has yielded 262 genes, while COPD phenotypes were associated with 918 genes. Despite a significant overlap between aging/senescence-related genes and COPD-related genes (89 genes; p = 7.06e–60), over half of the set of aging/senescence-related genes (N = 173 or 66.03%) have not been previously implicated in COPD. A complete list of these 173 aging/senescence-related genes along with support evidence and references is presented in the following files: Aging_COPD→Aging_alone genes and Ref for Aging_alone genes.
Mega- and Partial Mega-Analysis of Gene Expression Highlights TNFRSF12A and CD38
Only two genes, TNFRSF12A and CD38, have satisfied the significance criteria outlined in Table 2. Specifically, for an increased expression of TNFRSF12A, both mega-analysis and partial-meta analysis results were positive, while CD38 was identified as significantly involved by the partial-meta analysis only.

Table 2. Novel aging/senescence-related genes as highlighted by mega-analysis (MA) and partial MA expression.
Analysis of study heterogeneity showed that, for TNFRSF12A, there was no significant between-datasets variance for both mega-analysis and partial mega-analysis (ISq = 0, p–Q > 0.96), and therefore, a fixed-effect model was selected for this gene. For CD38, in mega-analysis, the between-datasets variance had accounted for 17.34% of the overall variance. When the random-effect model was used, outputs were not significant. Therefore, for CD38, only partial mega-analysis results were employed. For each gene, effect sizes, 95% confidence intervals, and weights of each study are presented in Figure 1.
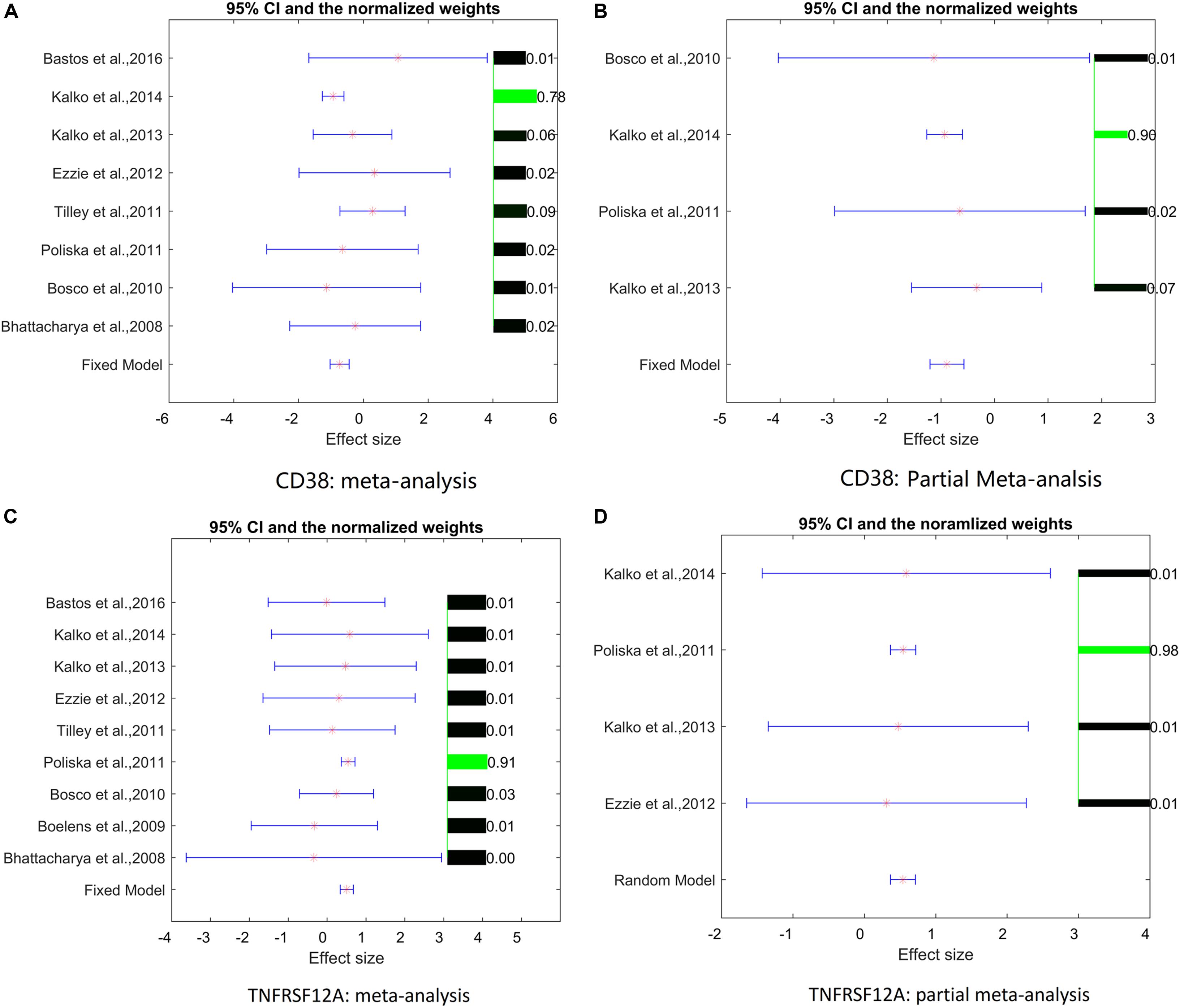
Figure 1. Effect sizes, 95% confidence intervals, and weights for each of two genes. (A) Mega-analysis results for CD38. (B) Partial mega-analysis results for CD38. (C) Mega-analysis results for TNFRSF12A. (D) Partial mega-analysis results for TNFRSF12A. The bar plot on the right of each figure represents the normalized weights for each dataset/study, ranged within (0, 1); the brighter (green) the color, the larger the relative weight of the study as labeled right next to the bar. For each dataset, the star (in red) and lines (in blue) on the left are the mean of effect size (log fold change), and 95% confidence interval (CI), respectively. References for datasets could be traced by their Gene Expression Omnibus (GEO) numbers.
As shown in Figure 1, two studies/datasets – GSE16972 for TNFRSF12A and GSE27543 for CD38 – demonstrated relatively small variances of effect size, leading to high z-scores and high weights within the mega-analysis. To determine whether these small variances were specific for the two genes, or were due to the peculiarities of data distribution within the datasets, we studied the distribution of the z-scores for all the genes differentially expressed in COPD patients suing empirical quantile–quantile plots (QQ plot), against the normal distribution, as shown in Figure 2. Even if the z-scores of these two datasets were not well fit to a normal distribution, their overall distribution was not that wide, with most of the genes demonstrating near-zero values. Therefore, the small variance corresponding to high z-scores observed for the TNFRSF12A and CD38 genes was not different to distribution expression values for other genes, not highlighted by mega-analysis.
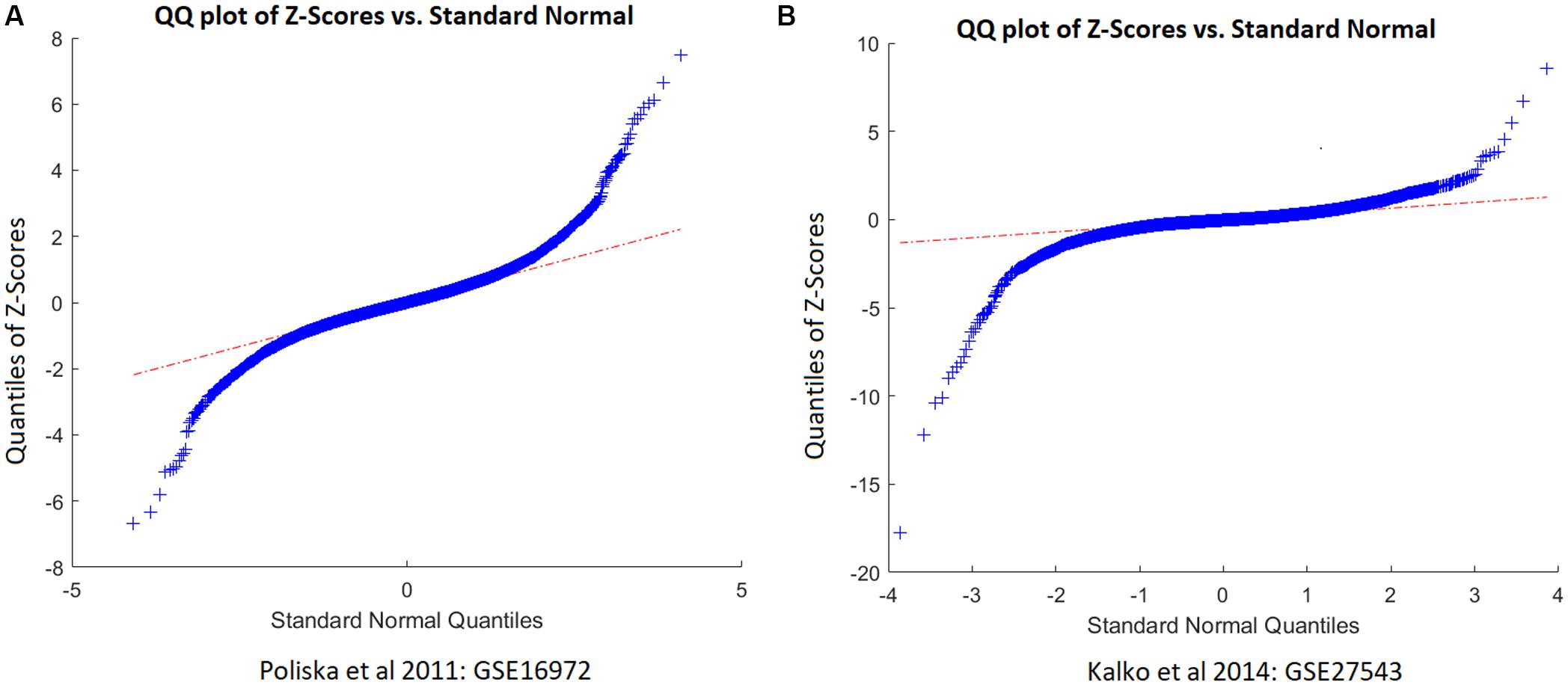
Figure 2. QQ plot of the z-scores of chronic obstructive pulmonary disease (COPD) patients versus standard normal distribution. (A) QQ plot built for dataset GSE16972. (B) QQ plot built for dataset GSE27543.
Multiple Linear Regression Analysis
A MLR analysis was conducted to explore the potential influence of three parameters on the expression levels of the genes in the case of COPD. Analysis of the MLR models shows that the region, where each study was performed, exerted significant influence on the expression fold changes detected for TNFRSF12A and CD38 genes with p < 3.10e–4 and 2.30 < e–3, respectively, nor were sample sizes not studying publishing dates were significant influencers.
GSEA Results
Input list for GSEA analysis is located in Aging_COPD→GSEA. In GSEA analysis of 91 genes implicated both in COPD and in senescence phenotypes (previously described genes, N = 89 genes; genes discovered in this study by mega-analysis, N = 2), a total of 113 GO terms were significantly enriched (p < 1e–10). Table 3 presents the Top 10 GO terms with enrichment values of p < 1.19e–21. Noteworthy, TNFRSF12A has been included in 9 of 113 enriched pathways, and in 3 of the Top 10 pathways (Table 3). CD38 was a part of 26 of the 113 enriched pathways, including in 4 of the top 10 pathways (Table 3). One of the GO terms, Apoptotic Signaling Pathway, retrieved a gene list with both TNFRSF12A and CD38.
Pathway Studio-Guided Analysis of Existing Literature
As COPD-related genes, TNFRSF12A and CD38, were identified de novo, after specific exclusion of the genes previously described as involved in COPD, gene-specific PubMed search confirmed that neither TNFRSF12A nor CD38 were previously discussed in the mechanistic context of COPD. However, the Shortest Path analysis performed in the Pathway Studio data mining environment revealed a number of plausible connections between these two genes and COPD, with a set of common interactions (Figure 3).
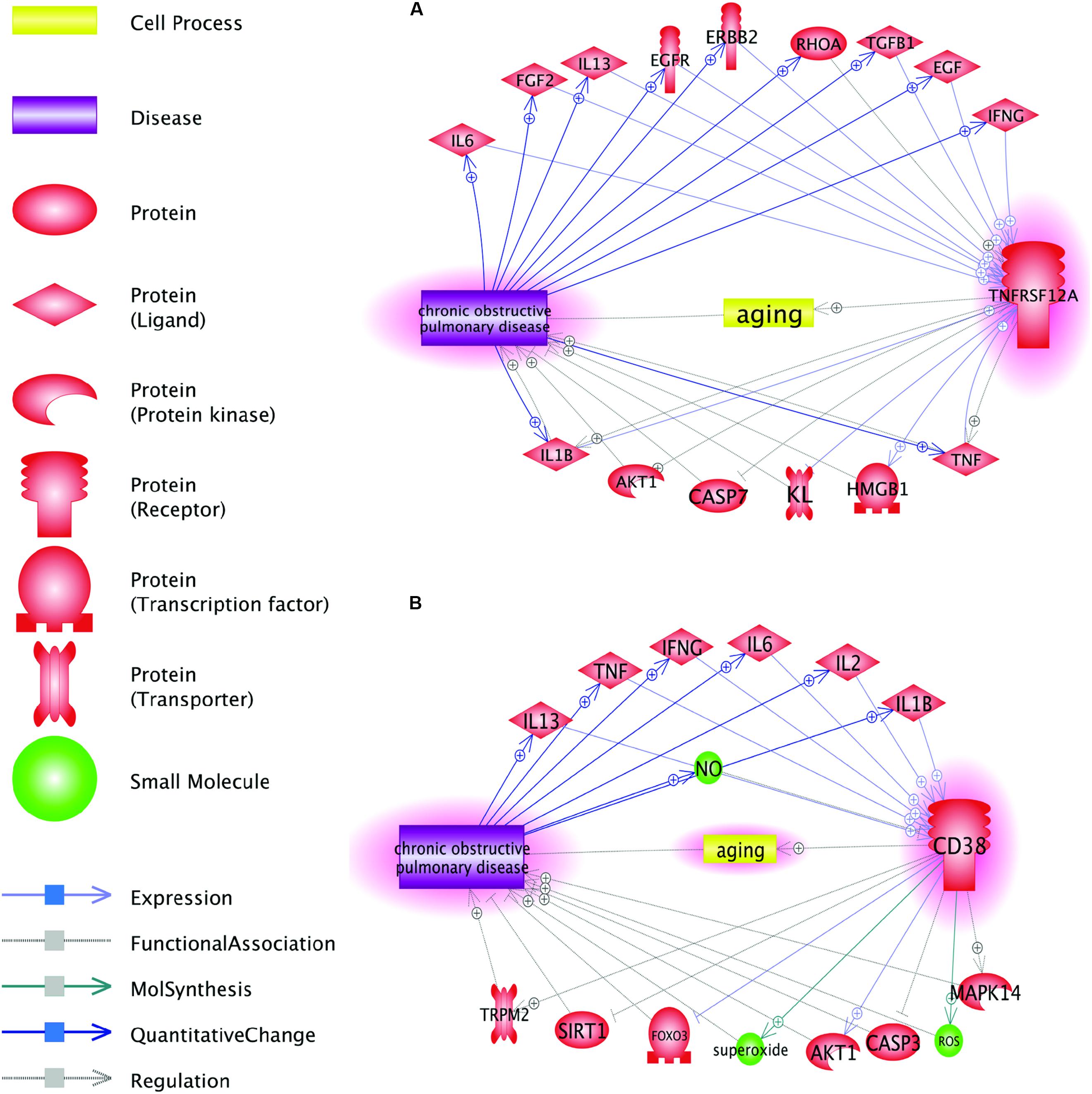
Figure 3. Function pathway analysis between COPD and the two genes: CD38 and TNFRSF12A. This network was generated in Pathway Studio environment (www.pathwaystudio.com). Each relation (edge) in the figure has one or more supporting references. (A) Pathways connecting COPD and gene TNFRSF12A; (B) Pathways connecting COPD and gene CD38.
Figure 3A shows that TNFRSF12A may influence the pathogenesis of COPD through multiple pathways. As an example, let us trace the connection TNFRSF12A→HMGB1→COPD. High-mobility group box-1 (HMGB1) modulates the balance between senescence and apoptosis in response to genotoxic stress, with higher expression levels of this protein profoundly shifting the balance toward senescence (Lee et al., 2019). Increased TWEAK signaling through larger amounts of available TNFRSF12A receptor potently induces HMGB1 expression and secretion (Moreno et al., 2013). Increases in extracellular concentrations of HMGB1 are proportional to the severity of COPD itself (Hou et al., 2011). Outside the cells, HMGB1 associates with numerous other proteins and signals back to the cell through the receptor for advanced glycation end products (RAGE) and toll-like receptor-4 (TLR-4), thus behaving as a typical damage-associated molecular pattern (DAMP) (Wong et al., 2018; Paudel et al., 2019). The details for all other relations presented in Figure 3 are described in Aging_COPD→Pathway Analysis. This reference information includes the types of the relationship, amount of underlying supporting references, and related sentences where these relationships have been identified and described. Evidence presented in Figure 3 also indicates that perpetuated overexpression of TNFRSF12A may serve one of the drivers for a vicious circle that keeps COPD patients from resolving inflammation in their lungs.
Discussion
We performed this study in an attempt to identify novel, not yet described molecular pathways, which link the process of tissue and cell aging and the development of COPD. By removing all known intersections between curated gene sets involved in each of these pathophysiological processes, we ensured that uncovered senescence-related contributors to COPD had not been already described as such. Through the Pathway Studio-guided literature mining, a total of 173 genes involved in the senescence, but not in COPD, were discovered. These genes were investigated for consistent evidence of the changes in their expression in COPD phenotypes across nine mRNA expression datasets acquired from GEO (Table 1).
In cross-dataset mega-analysis of gene-level expression patterns, expression levels of seven senescence-related genes were significantly altered in COPD compared to normal lungs (p < 0.05, see in Aging_COPD→Meta_Analysis). When across dataset thresholds were lowered by applying partial mega-analysis techniques, a total of 18 senescence-related genes were highlighted as possibly involved in COPD (see in Aging_COPD→Partial Meta). However, only two senescence-related genes, TNFRSF12A and CD38, have passed pre-selected criterion of the significance of association, which were set at p < 1e–7 and LFC of >0.49 or <−0.74 (Table 2 and Figure 2). In particular, in meta- and partial meta-analyses, LFCs of observed TNFRSF12A expression levels were at 0.51 and at 0.54, respectively, demonstrating that expression levels of TNFRSF12A in COPD samples were consistently increased by more than 40%. When similar criteria were applied to CD38, its expression levels were found to be decreased in COPD samples by more than 40% (Table 2), with the only difference that the changes in CD38 expression levels have passed the significance criteria in partial mega-analysis only.
For both molecules, their roles in the senescence and aging-associated diseases are well described, while no apparent connections to COPD have been reported so far. TNFRSF12A encodes for an exclusive receptor for tumor necrosis factor-related weak inducer of apoptosis (TWEAK); jointly, this interacting pair of molecules is involved in age-associated pathological changes in skeletal muscle and other organs (Van Kirk et al., 2011; Tajrishi et al., 2014; Hénaut et al., 2016). CD38 is one of the main NAD-degrading enzymes, which increases its expression in aging tissues and is directly responsible to age-related NAD decline (Horenstein et al., 2013; Camacho-Pereira et al., 2016). When the levels of NAD+ are low, a senescence-associated secretory phenotype (SASP) to a certain degree diminishes its damaging power as a part of a fundamental trade-off between aging and energy available to the cells (Mendelsohn and Larrick, 2019). Moreover, adenosine, which is produced in CD38-dependent reaction, further suppresses local immune response (Horenstein et al., 2013). In healthy aging, SASP cytokines promote an expression of CD38 (Chini et al., 2019). In case of lungs affected by COPD, a decrease in CD38 expression was noted in at least some datasets. It should be noted, however, that some COPD datasets do not comply, possibly due to the stage-specific differences in the SASP response of COPD lungs (Parikh et al., 2019). As senescent cells are also capable of inducing surface CD38 expression on macrophages and endothelial cells (Chini et al., 2019), particular balance between CD38-lowering and CD38-enhancing forces may be dependent upon tissue composition and/or disease stage of collected samples and, therefore, may be dataset specific.
Notably, a number of previous studies connected COPD to an increased risk of developing non-small cell lung carcinoma (NSCLC), irrespective of the pack-years history of their smoking (Houghton, 2013; Ng KeeKwong et al., 2017). Both TNFRSF12A/TWEAK pair (Whitsett et al., 2014) and CD38, along with its interaction partner CD31 (Malavasi et al., 2008) are involved in multiple cancers, including NSCLC, where they contribute to escape from PD-1/PD-L1 blockade (Chen et al., 2018; Konen et al., 2019) and cytotoxic therapy (Whitsett et al., 2014). Obvious importance of TNFRSF12A/TWEAK molecules for the survival of cancers cells prompted the development of the respective targeting agents, which already met initial success in clinical and pre-clinical trials [see Hu et al., 2017, for review]. Our findings point at the potential applicability of the abovementioned therapeutics in COPD context.
The results of the GSEA analysis support the association between senescence and COPD, with 113 pathways uncovered as significantly enriched and connected to both of these phenotypes (p < 1e–10; see Table 3 and Aging_COPD→GSEA). Among them, we have identified 9 and 26 significantly enriched pathways, where either TNFRSF12A or CD38 were listed as shared genes, respectively. Notably, CD38 plays a role in many enriched oxygen/hypoxia and steroid hormone-related pathways, which both have been linked to COPD previously (Marwick et al., 2013; Wada and Takizawa, 2013). On the other hand, a number of enriched cytokine-mediated signaling pathways, positively regulating cell apoptosis and influencing angiogenesis, include TNFRSF12A as their integral part; these pathways have also been implicated in COPD pathogenesis (Vanella et al., 2017; Kropski et al., 2018).
Employing Pathway Studio-guided “Shortest Path analysis” allowed us to explore plausible connections between these two genes and COPD, simultaneously highlighting a set of common interactors, which includes the signaling molecule and the pro-inflammatory cytokines. Among these, a connection of both TNFRSF12A and CD38 proteins to AKT1 is remarkable, as the stimulation of IGF1/AKT1 axis via the targeting of p16-induced senescence was recently shown to alleviate at least some phenotypic features of COPD (Cottage et al., 2019). Notably, among other connecting molecules are pro-inflammatory cytokines TNF-α, IL-1β, IFN-γ, and IL-6, which are the part of the SASP phenotype (Barnes, 2014; Ohtani, 2019).
Importantly, this study is not free of limitation. In particular, we acknowledge that the study pipeline was executed in silico, with no experimental validation of the findings, and that the study set was relatively small, in part, due to the strict quality control of selected datasets. Nevertheless, we believe that the overall conclusion of our study may further our understanding of COPD.
In particular, we noted that CD38/TNFRSF12A and COPD relationships demonstrate the features of bidirectional, self-propagating cycle rather than a unidirectional regulation pathway (Figure 4). We speculate that, in COPD, stimulation of TWEAK/TNFRSF12A signaling enhances tissue remodeling by stimulation migration of the cells and the SASP cytokine production [these statements are supported by experiments described in Refs. (Wang et al., 2017; Das et al., 2018; Liu et al., 2019)], which, in turn, induce CD38 mRNA and protein expression, and stimulate activity of this NADase in adjacent non-senescent cells, including populations of macrophages and endothelia [supported by experiments described in Parikh et al., 2019], with the resultant depletion of NAD+ contributing to accelerated aging observed in COPD patients (Triest et al., 2019), and to further increase in TWEAK/TNFRSF12A signaling. The resultant model is described in Figure 4, with relative levels of CD38/TWEAK expression establishing whether lung inflammation would resolve or would form a vicious cycle of COPD.
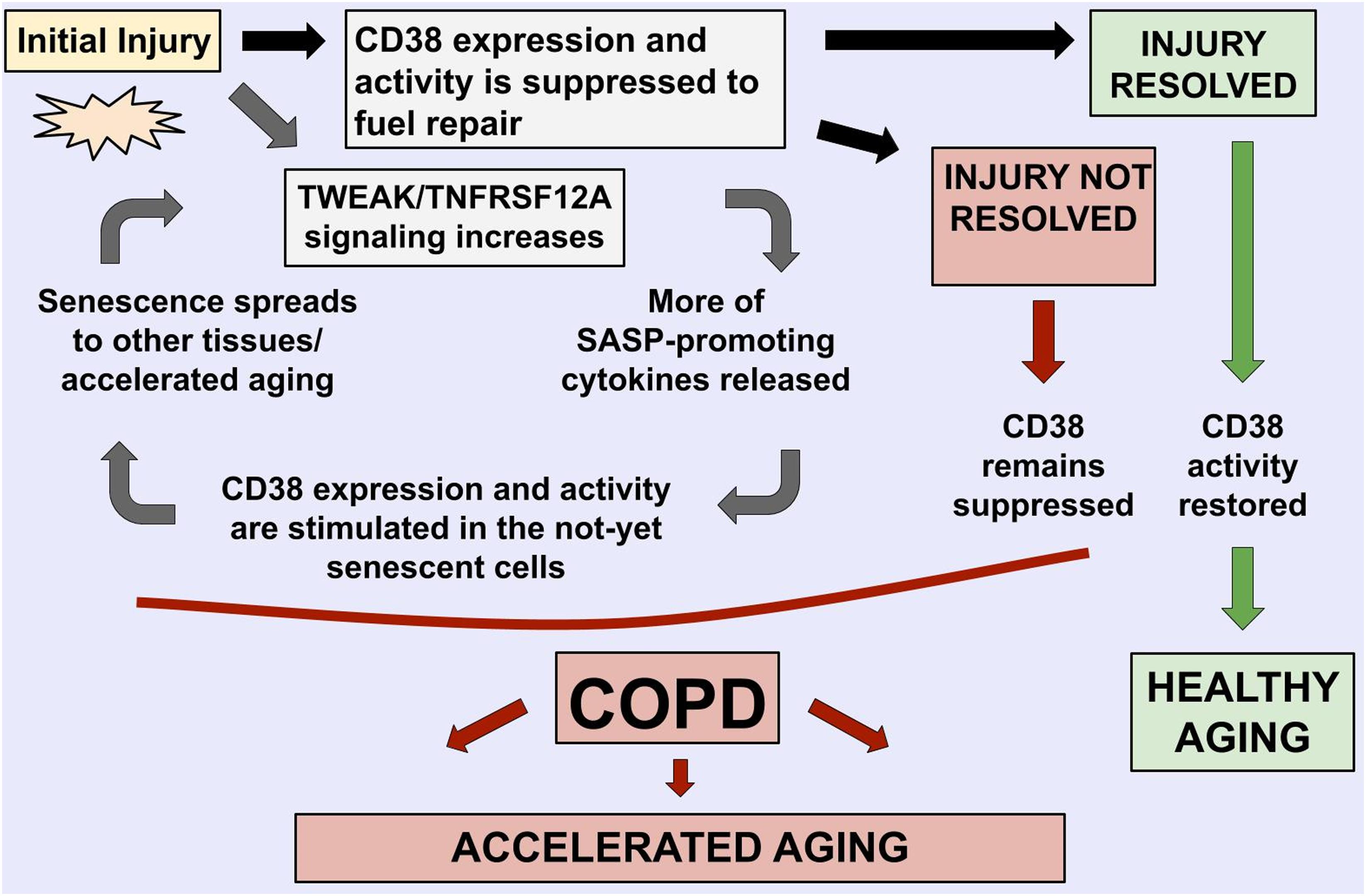
Figure 4. Mechanistic model highlights the roles of TNFRSF12A and CD38 in COPD-related accelerated aging phenotypes, with their balance defining if the lung injury is resolved.
Conclusion
Using a large-scale literature data mining and gene-level mega-analysis of multiple gene expression datasets, we specifically searched for novel senescence-associated genes, which may also contribute to COPD. We show that genes TNFRSF12A and CD38 encode factors previously unrecognized as COPD contributors, and have generated the model that explains accelerated aging phenotypes previously observed in COPD patients, and calls for investigation of the balance of TNFRSF12A/CD38 proteins as the key to establishing vicious cycle of unresolvable tissue remodeling in COPD lungs.
Data Availability Statement
The datasets analyzed in this study can be found in the GEO repository (https://www.ncbi.nlm.nih.gov/geo/). Relation data for genes previously associated either with senescence or with COPD were extracted from existing literature in the Pathway Studio environment (www.pathwaystudio.com) and arranged in the database Aging_COPD, hosted at http://database.gousinfo.com. The downloadable form in Excel is available at http://gousinfo.com/database/Data_Genetic/Aging_COPD.xlsx.
Author Contributions
YD, RC, and HC contributed to the conception and design of the study. HC organized the database and performed the Pathway Studio work. RC and YD retrieved the expression data and performed the statistical analysis. RC and HC wrote the first draft of the manuscript. AB provided the interpretation of the data and built the COPD model. AB and HC wrote the final version of the manuscript. All authors contributed to the manuscript revision, read, and approved the submitted version.
Funding
This project was partially supported by the 2018 Medical-Clinical Science and Technology Development Fund of Jiansu University (Project ID: JLY20180021) and Middle-aged and Youth Development Fund of Lianyungang Second People’s Hospital (Project ID: TQ201902). AB and HC have not received any funding.
Conflict of Interest
The authors declare that the research was conducted in the absence of any commercial or financial relationships that could be construed as a potential conflict of interest.
Acknowledgments
The authors are grateful to the School of Systems Biology, GMU, for providing access to the Pathway Studio environment. Image credits: Figure 4 was drawn by Elizaveta Plis, Luther Jackson Middle School (FCPS), Fairfax, VA, United States.
Footnotes
References
Barnes, P. J. (2014). Cellular and molecular mechanisms of chronic obstructive pulmonary disease. Clin. Chest Med. 35, 71–86. doi: 10.1016/j.ccm.2013.10.004
Borenstein, M., Hedges, L. V., Higgins, J. P., and Rothstein, H. R. (2010). A basic introduction tofixed-effect and random-effects models for meta-analysis. Res. Synth. Methods 1, 97–111. doi: 10.1002/jrsm.12
Brandsma, C. A., de Vries, M., Costa, R., Woldhuis, R. R., Königshoff, M., and Timens, W. (2017). Lungageing and COPD: is there a role for ageing in abnormal tissue repair? Eur. Respir. Rev. 26:170073. doi: 10.1183/16000617.0073-2017
Camacho-Pereira, J., Tarragó, M. G., Chini, C. C. S., Nin, V., and Escande, C. (2016). CD38 dictates age-related nad declineand mitochondrial dysfunction through an sirt3-dependent mechanism. Cell Metab. 23, 1127–1139. doi: 10.1016/j.cmet.2016.05.006
Chen, L., Diao, L., Yang, Y., Yi, X., Rodriguez, B. L., Li, Y., et al. (2018). CD38-mediated immunosuppression as a mechanism of tumor cell escape from PD-1/PD-L1 blockade. Cancer Discov. 8, 1156–1175. doi: 10.1158/2159-8290.CD-17-1033
Chini, C., Hogan, K. A., Warner, G. M., Tarragó, M. G., Peclat, T. R., Tchkonia, T., et al. (2019). The NADase CD38 is induced by factors secreted from senescent cells providing a potential link between senescence and age-related cellular NAD(+) decline. Biochem. Biophys. Res. Commun. 513, 486–493. doi: 10.1016/j.bbrc.2019.03.199
Cottage, C. T., Peterson, N., Kearley, J., Berlin, A., Xiong, X., Huntley, A., et al. (2019). Targetingp16-induced senescence prevents cigarette smoke-induced emphysema by promotingIGF1/Akt1 signaling in mice. Commun. Biol. 2:307. doi: 10.1038/s42003-019-0532-1
Das, N. A., Carpenter, A. J., Yoshida, T., Kumar, S. A., Gautam, S., Mostany, R., et al. (2018). TRAF3IP2 mediatesTWEAK/TWEAKR-induced pro-fibrotic responses in cultured cardiac fibroblasts andthe heart. J. Mol. Cell Cardiol. 121, 107–123. doi: 10.1016/j.yjmcc.2018.07.003
de Vries, M., Faiz, A., Woldhuis, R. R., Postma, D. S., de Jong, T. V., Sin, D. D., et al. (2018). Lung tissuegene-expression signature for the ageing lung in COPD. Thorax 73, 609–617. doi: 10.1136/thoraxjnl-2017-210074
Eapen, M. S., Myers, S., Walters, E. H., and Sohal, S. S. (2017). Airway inflammation in chronicobstructive pulmonary disease (COPD): a true paradox. Expert Rev. Respir. Med. 11, 827–839. doi: 10.1080/17476348.2017.1360769
Hénaut, L., Sanz, A. B., Martin-Sanchez, D., Carrasco, S., Villa-Bellosta, R., Aldamiz-Echevarria, G., et al. (2016). TWEAK favorsphosphate-induced calcification of vascular smooth muscle cells through canonicaland non-canonical activation of NFκB. Cell Death Dis. 7:e2305. doi: 10.1038/cddis.2016.220
Horenstein, A. L., Chillemi, A., Zaccarello, G., Bruzzone, S., Quarona, V., Zito, A., et al. (2013). A CD38/CD203a/CD73 ectoenzymatic pathway independent of CD39drives a novel adenosinergic loop in human T lymphocytes. Oncoimmunology 2:e26246. doi: 10.4161/onci.26246
Hou, C., Zhao, H., Liu, L., Li, W., Zhou, X., Lv, Y., et al. (2011). High mobility group protein B1 (HMGB1) in Asthma: comparison of patients with chronic obstructive pulmonary disease and healthy controls. Mol. Med. 17, 807–815. doi: 10.2119/molmed.2010.00173
Houghton, A. M. (2013). Mechanistic links between COPD and lung cancer. Nat. Rev. Cancer 13, 233–245. doi: 10.1038/nrc3477
Hu, G., Zeng, W., and Xia, Y. (2017). TWEAK/Fn14 signaling in tumors. Tumour Biol. 39:1010428317714624. doi: 10.1177/1010428317714624
Konen, J. M., Fradette, J. J., and Gibbons, D. L. (2019). The good, the bad and the unknown of cd38in the metabolic microenvironment and immune cell functionality of solid tumors. Cells 9:E52. doi: 10.3390/cells9010052
Kropski, J. A., Richmond, B. W., Gaskill, C. F., Foronjy, R. F., and Majka, S. M. (2018). Deregulatedangiogenesis in chronic lung diseases: a possible role for lung mesenchymalprogenitor cells (2017 Grover Conference Series). Pulm. Circ. 8:2045893217739807. doi: 10.1177/204589321779807
Lee, J. J., Park, I. H., Rhee, W. J., Kim, H. S., and Shin, J. S. (2019). HMGB1 modulates the balance betweensenescence and apoptosis in response to genotoxic stress. FASEB J. 33, 10942–10953. doi: 10.1096/fj.201900288R
Liu, J., Liu, Y., Peng, L., Li, J., Wu, K., Xia, L., et al. (2019). TWEAK/Fn14 signals mediate burn wound repair. J. Invest. Dermatol. 139, 224–234. doi: 10.1016/j.jid.2018.05.036
Malavasi, F., Deaglio, S., Funaro, A., Ferrero, E., Horenstein, A. L., Ortolan, E., et al. (2008). Evolution and function of the ADP ribosyl cyclase/CD38 gene family inphysiology and pathology. Physiol. Rev. 88, 841–886. doi: 10.1152/physrev.00035.2007
Marwick, J. A., Dorward, D. A., Lucas, C. D., Jones, K. O., Sheldrake, T. A., Fox, S., et al. (2013). Oxygen levels determine the ability of glucocorticoids to influence neutrophilsurvival in inflammatory environments. J. Leukoc. Biol. 94, 1285–1292. doi: 10.1189/jlb.0912462
Mendelsohn, A. R., and Larrick, J. W. (2019). Interacting NAD(+) and cell senescence pathwayscomplicate antiaging therapies. Rejuven. Res. 22, 261–266. doi: 10.1089/rej.2019.2218
Mercado, N., Ito, K., and Barnes, P. J. (2015). Accelerated ageing of the lung in COPD: newconcepts. Thorax 70, 482–489. doi: 10.1136/thoraxjnl-2014-206084
Moreno, J. A., Sastre, C., Madrigal-Matute, J., Muñoz-García, B., Ortega, L., Burkly, L. C., et al. (2013). HMGB1 expression and secretion areincreased via TWEAK-Fn14 interaction in atherosclerotic plaques and culturedmonocytes. Arterioscler. Thromb. Vasc. Biol. 33, 612–620. doi: 10.1161/ATVBAHA.112.300874
Ng KeeKwong, F., Nicholson, A. G., Harrison, C. L., Hansbro, P. M., Adcock, I. M., and Chung, K. F. (2017). Ismitochondrial dysfunction a driving mechanism linking COPD to nonsmall cell lung carcinoma? Eur. Respir. Rev. 26:170040. doi: 10.1183/16000617.0040-2017
Ohtani, N. (2019). Deciphering the mechanism for induction of senescence-associatedsecretory phenotype (SASP) and its role in aging and cancer development. J. Biochem. 12:mvz055. doi: 10.1093/jb/mvz055
Parikh, P., Wicher, S., Khandalavala, K., Pabelick, C. M., Britt, R. D. Jr., and Prakash, Y. S. (2019). Cellular senescence in the lung across the age spectrum. Am. J. Physiol. Lung Cell Mol. Physiol. 316, L826–L842. doi: 10.1152/ajplung.00424.2018
Paudel, Y. N., Angelopoulou, E., Piperi, C., Balasubramaniam, V. R. M. T., Othman, I., and Shaikh, M. F. (2019). Enlightening the role of high mobility group box 1 (HMGB1) in inflammation:Updates on receptor signalling. Eur. J. Pharmacol. 858:172487. doi: 10.1016/j.ejphar.2019.172487
Reiner, A., Yekutieli, D., and Benjamini, Y. (2003). Identifying differentially expressed genesusing false discovery rate controlling procedures. Bioinformatics 19, 368–375.
Rubinsztajn, R., Przybyłowski, T., Grabicki, M., Karwat, K., Maskey-Warzêchowska, M., Batura-Gabryel, H., et al. (2019). Comorbidities in chronic obstructive pulmonarydisease: results of a national multicenter research project. Adv. Clin. Exp. Med. 28, 319–324. doi: 10.17219/acem/78024
Seifuddin, F., Pirooznia, M., Judy, J. T., Goes, F. S., Potash, J. B., and Zandi, P. P. (2013). Systematicreview of genome-wide gene expression studies of bipolar disorder. BMC Psychiatry 13:213. doi: 10.1186/1471-244X-13-213
Tajrishi, M. M., Sato, S., Shin, J., Zheng, T. S., Burkly, L. C., and Kumar, A. (2014). The TWEAK-Fn14 dyadis involved in age-associated pathological changes in skeletal muscle. Biochem. Biophys. Res. Commun. 446, 1219–1224. doi: 10.1016/j.bbrc.2014.03.084
Tilert, T., Paulose-Ram, R., Howard, D., Butler, J., Lee, S., and Wang, M. Q. (2018). Prevalence andfactors associated with self-reported chronic obstructive pulmonary disease amongadults aged 40-79: the national health and nutrition examination survey (NHANES) 2007-2012. EC Pulmonol. Respir. Med. 7, 650–662.
Triest, F. J. J., Franssen, F. M. E., Reynaert, N., Gaffron, S., Spruit, M. A., Janssen, D. J. A., et al. (2019). Disease-specific comorbidity clusters in COPD and accelerated aging. J. Clin. Med. 8:E511. doi: 10.3390/jcm8040511
Van Kirk, C. A., VanGuilder, H. D., Young, M., Farley, J. A., Sonntag, W. E., and Freeman, W. M. (2011). Age-related alterations in retinal neurovascular and inflammatory transcripts. Mol. Vis. 17, 1261–1274.
Vanella, L., Li Volti, G., Distefano, A., Raffaele, M., Zingales, V., Avola, R., et al. (2017). A new antioxidant formulation reduces the apoptotic and damagingeffect of cigarette smoke extract on human bronchial epithelial cells. Eur. Rev. Med. Pharmacol. Sci. 21, 5478–5484. doi: 10.26355/eurrev_201712_13938
Vestbo, J. (2014). COPD: definition and phenotypes. Clin. Chest. Med. 35, 1–6. doi: 10.1016/j.ccm.2013.10.010
Wada, H., and Takizawa, H. (2013). Future treatment for COPD: targeting oxidative stress and its related signal. Recent Pat. Inflamm. Allergy Drug Discov. 7, 1–11.
Wang, A., Zhang, F., Xu, H., Xu, M., Cao, Y., Wang, C., et al. (2017). TWEAK/Fn14 promotes pro-inflammatory cytokine secretion in hepatic stellate cellsvia NF-κB/STAT3 pathways. Mol. Immunol. 87, 67–75. doi: 10.1016/j.molimm.2017.04.003
Whitsett, T. G., Mathews, I. T., Cardone, M. H., Lena, R. J., Pierceall, W. E., Bittner, M., et al. (2014). Mcl-1 mediates TWEAK/Fn14-induced non-small celllung cancer survival and therapeutic response. Mol. Cancer Res. 12, 550–559.
Wong, S. L., To, J., Santos, J., Allam, V. S. R. R., Dalton, J. P., Djordjevic, S. P., et al. (2018). Proteomic analysis of extracellular HMGB1 identifiesbinding partners and exposes its potential role in airway epithelial cell homeostasis. J. Proteome Res. 17, 33–45. doi: 10.1021/acs.jproteome.7b00212
Keywords: senescence, lung, chronic inflammation, aging, tissue remodeling, network analysis
Citation: Dong Y, Cao H, Cao R and Baranova A (2020) TNFRSF12A and CD38 Contribute to a Vicious Circle for Chronic Obstructive Pulmonary Disease by Engaging Senescence Pathways. Front. Cell Dev. Biol. 8:330. doi: 10.3389/fcell.2020.00330
Received: 02 December 2019; Accepted: 16 April 2020;
Published: 27 May 2020.
Edited by:
Vassilis G. Gorgoulis, National and Kapodistrian University of Athens, GreeceReviewed by:
Fabio Malavasi, University of Turin, ItalyFunlan Anvin, Ditmanson Medical Foundation Chia-Yi Christian Hospital, Taiwan
Haruhiro Higashida, Kanazawa University, Japan
Copyright © 2020 Dong, Cao, Cao and Baranova. This is an open-access article distributed under the terms of the Creative Commons Attribution License (CC BY). The use, distribution or reproduction in other forums is permitted, provided the original author(s) and the copyright owner(s) are credited and that the original publication in this journal is cited, in accordance with accepted academic practice. No use, distribution or reproduction is permitted which does not comply with these terms.
*Correspondence: Rongyuan Cao, cm9uZ3l1YW5jYW8yMDE5QGdvdXNpbmZvLmNvbQ==; Ancha Baranova, YWJhcmFub3ZAZ211LmVkdQ==