- 1Functional Pharmacology, Department of Neuroscience, Uppsala University, Uppsala, Sweden
- 2Institute for Translational Medicine and Biotechnology, Sechenov First Moscow State Medical University, Moscow, Russia
As the structure and functions of proteins are correlated, investigating groups of proteins with the same gross structure may provide important insights about their functional roles. Trispanins, proteins that contain three alpha-helical transmembrane (3TM) regions, have not been previously studied considering their transmembrane features. Our comprehensive identification and classification using bioinformatic methods describe 152 3TM proteins. These proteins are frequently involved in membrane biosynthesis and lipid biogenesis, protein trafficking, catabolic processes, and in particular signal transduction due to the large ionotropic glutamate receptor family. Proteins that localize to intracellular compartments are overrepresented in the dataset in comparison to the entire human transmembrane proteome, and nearly 45% localize specifically to the endoplasmic reticulum (ER). Furthermore, nearly 20% of the trispanins function in lipid metabolic processes and transport, which are also overrepresented. Nearly one-third of trispanins are identified as being targeted by drugs and/or being associated with diseases. A high number of 3TMs have unknown functions and based on this analysis we speculate on the functional involvement of uncharacterized trispanins in relationship to disease or important cellular activities. This first overall study of trispanins provides a unique analysis of a diverse group of membrane proteins.
Introduction
Cellular boundaries or membranes are imperative for cells to function properly and membrane formation is considered an essential step in the emergence of life. The plasma membrane is the most studied of cell membranes, and is composed of a phospholipid bilayer that contains phospholipids, glycolipids, sterols, and proteins. In addition to the plasma membrane, eukaryotic cells also contain intracellular membrane-bound organelles, including double membrane compartments such as the nucleus and mitochondria and single membrane-bound organelles such as the endoplasmic reticulum (ER), Golgi apparatus, lysosomes, peroxisomes, and vesicles. Each membrane-bound compartment is defined by its lipid and protein composition (Harayama and Riezman, 2018). For instance, localized sphingolipid metabolism is associated with membrane budding and formation of exosomes in multivesicular bodies, or in another example, membrane proteins are differentially transported to the apical or basal plasma membrane in polarized cells (Harayama and Riezman, 2018). Membranes act as barriers that not only physically separate the intracellular components from the extracellular environment or from each other, but selectively allow materials to flow in and out of the cell or other organelles, as well as extract energy from the environment (Thomas and Rana, 2007). Transmembrane proteins and complexes can form channels, pores, and gates that extend through the lipid bilayer and function in active and passive transport of substrates across a membrane. Membrane machinery and protein complexes on different organelles can be involved in specialized activities, for example vesicle formation and movement on the Golgi apparatus, protein targeting and transport at the ER, and specific receptor signaling and transduction at the plasma membrane. And due to the varied essential functional pathways that membrane proteins are involved in, they are of particular interest in investigating the pathophysiology of diseases. In fact, membrane proteins are major targets for pharmaceutical agents and more than 60% of drug targets are membrane proteins (Overington et al., 2006).
Of the ∼20,000 total protein coding genes in Homo sapiens, approximately 25–30% (∼5500) are coded for transmembrane proteins (Almén et al., 2009; Attwood et al., 2017). Further, ∼70% of these membrane proteins are estimated to be conserved since the last holozoa common ancestor, indicating the fundamental importance and evolutionary origins of many membrane proteins (Attwood et al., 2017). Investigating cell membrane structures and complexes harkens back to how life originated, i.e., how the lipid bilayer developed and how metabolism and replication evolved in cells (Griffiths, 2007; Thomas and Rana, 2007; Deamer, 2017). Further, membrane compartmentalization and expansion enabled the development of larger cells and the separation of cell functions in eukaryotic cells, which also inaugurated the need for communication between the different cellular compartments (Gabaldón and Pittis, 2015). Subcellular compartmentalization has been aided through evolutionary retargeting of membrane proteins to shared or different localizations, which is evident by the varied internal sorting of proteins across different physiological conditions, cell types, localizations, and lineages (Gabaldón and Pittis, 2015). Consequently, investigating the evolutionary developments of homologous protein families as well as where proteins localize to can provide insight into their different functional activities.
The membrane proteome can be characterized into functional groups based on the number of transmembrane helices; i.e., the tertiary structure of protein groups can promote specific functional activities. A widely known example is the large seven transmembrane spanning families of G protein-coupled receptors (GPCRs) that function in signaling (Lagerström and Schiöth, 2008) or the twelve transmembrane proteins of the major facilitator superfamily (MFS) that act as transporters (Reddy et al., 2012). Additionally, studies have shown that proteins that span the membrane once or twice are often engaged in enzymatic functions (Almén et al., 2009) or immune response signaling (Sällman Almén et al., 2012) while many tetra-spanning proteins are involved in transport activities (Attwood et al., 2016). Our previous investigation has suggested that there are many three transmembrane-spanning (3TM or trispanins) proteins that have unknown functions (Almén et al., 2009) and an in-depth analysis of membrane proteins that share a basic structural similarity with 3TM regions has yet to be published. Investigations of groups of proteins with the same gross structure may lead to important insights about the functional activities, perhaps in particular for proteins with unknown functions.
In this study we perform comprehensive bioinformatic analyses of all 3TM proteins in the human genome. We identify and characterize the 3TM group characteristics and incorporate information on relevant functional activities along with cellular localizations, tissue enrichment patterns, and protein-protein interaction networks to describe the predominant functional activities of this group of proteins. Additionally, we use this methodology to speculate on the functions of several uncharacterized trispanins that are associated with disease or potentially involved in important pathways.
Functional Analysis Results
We identified 152 proteins as 3TMs and these were classified into three primary functional classes plus a fourth group that has varied activities: 35 proteins were identified as enzymes that had an associated enzyme commission number (EC); 26 proteins had an associated transporter classification database identifier (TCDB) and were characterized as transporters; 21 receptors were identified based on IUPHARS guide to pharmacology descriptions; and 43 proteins with varied functional activities were characterized as well as 27 proteins that were classified as uncharacterized. Approximately one-third of trispanins localize to multiple membranes, with the plasma membrane and another intracellular organelle being the predominant combination. The original CCDS human protein sequences file contained 18,894 unique CCDS gene identifiers with 32,554 total entries including isoforms. The sequences were pre-processed and the signal peptides were excised, followed by evaluation with TOPCONS-single to predict transmembrane helices. The 3TM dataset was collated by removing ambiguous entries, isoforms, and manually adding proteins ascertained as 3TM through literature searches (see section “Materials and Methods” for details). Functional annotations and localizations were assessed through gene ontology (GO) descriptions (Ashburner et al., 2000; The Gene Ontology Consortium, 2019), the human protein atlas (Uhlén et al., 2015), the PANTHER classification database (Mi et al., 2019), and KEGG pathways database (Kanehisa et al., 2017). Supplementary File S1 contains the final 3TM dataset.
Membrane Complexes
Almost 35 of the proteins in the dataset are members of membrane complexes and localize to various membranes, including the plasma membrane, as well as organelles such as the mitochondrion, the endomembrane system with the ER and Golgi apparatus, and the nucleus (Figure 1). With the 17 proteins that localize to the mitochondrion, five of the nine proteins that are found in the inner mitochondrial membrane are involved in inner mitochondrial membrane complexes, including three members (TIMM23, TIMM17A, and TIMM17B) of the TIM23 translocase. The TIM23 complex is the major translocase for importing proteins across the inner mitochondrial membrane and into the mitochondrial matrix (Demishtein-Zohary and Azem, 2017). SDHC and SDHD are also members of inner mitochondrial membrane complexes. They are the two transmembrane components of succinate dehydrogenase, or the mitochondrial respiratory complex II, which functions in the mitochondrial electron transport chain, and these trispanins together contain one heme b and provide the binding site for ubiquinone (Yankovskaya et al., 2003). The trispanin TMEM177 has been recently discovered to form complexes with COX20 and associate with COX2, which are essential for the assembly of cytochrome c oxidase that is the final enzyme complex of the mitochondrial respiratory electron transport chain. TMEM177 dynamically interacts with COX2 subcomplexes in a COX20-dependant manner which in turn stabilizes COX2 during early synthesis (Lorenzi et al., 2018). TMEM11 is another inner mitochondrial protein and is associated with the multiple mitochondrial contact site and cristae junction organizing system (MICOS) complex, which dynamically regulates mitochondrial membrane architecture (Guarani et al., 2015). Both TMEM177 and TMEM11 have yet to be annotated as members of membrane protein complexes, which may contribute to an underrepresentation of complexes in the dataset.
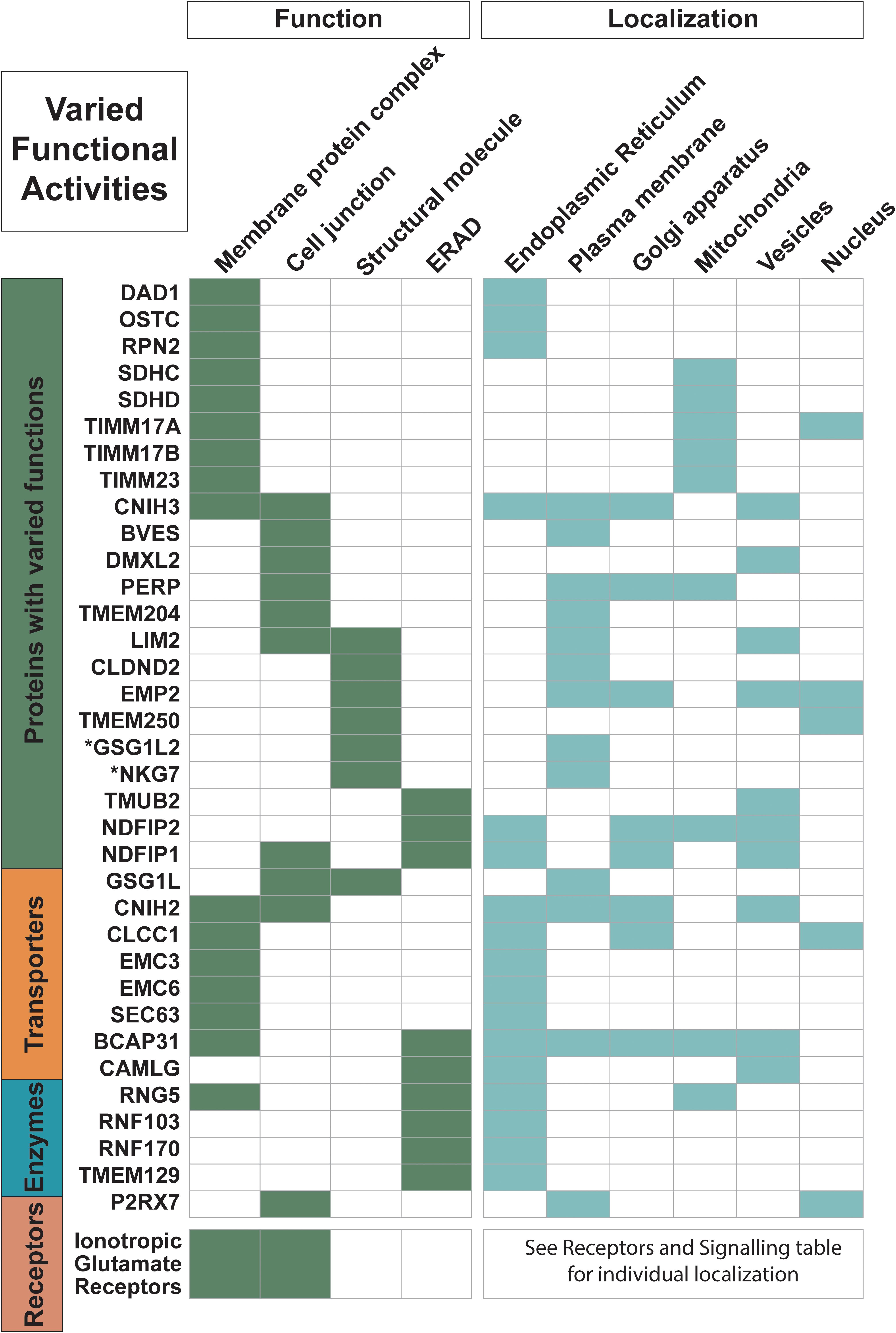
Figure 1. Proteins with varied functional activities and localization information. Trispanins from all four major classes involved in these common functional activities: membrane protein complexes; cell junctions; structural molecules; and endoplasmic reticulum (ER)-associated degradation processes (ERAD) and ubiquitination processes. All 18 ionotropic glutamate receptors are included in one row and individual localization information on them can be found in Figure 4. Functional activities and localization descriptions derived from gene ontology (GO) Annotations (Huntley et al., 2015), PANTHER classifications (Mi et al., 2019), and the human protein atlas (Uhlén et al., 2015).
Several membrane complexes that localize to the ER function in two different pathways that facilitate membrane insertion of proteins. One pathway uses the important signal recognition particle (SRP) dependent ER protein translocon, which consists of the co-translational protein-conducting channel Sec61 complex along with additional subunits involved in nascent chain processing and translocation (Mades et al., 2012). The SEC63 protein identified in the dataset is part of the Sec61 chaperone network that performs substrate-selective quantity control during co-translational ER import (Mades et al., 2012). An additional three proteins in the dataset are members of the oligosaccharyl-transferase (OST) complex: RPN2, DAD1, and OSTC (also known as DC2). The OST complex is also an integral component of the translocon that catalyzes co-translational N-glycosylation, which is one of the most common protein modifications in eukaryotic cells (Pfeffer et al., 2014; Braunger et al., 2018). Furthermore, homologs of BCAP29 and BCAP31, both members of the 3TM dataset, have been shown to form a complex together that is associated with the Sec61 complex and interacts with translocation substrates (Wilson and Barlowe, 2010). Two more proteins, WRB and CAML, are involved in another type of protein insertion into the ER membrane as subunits in the post-translational tail-anchored membrane protein insertion TRC40 complex (Yamamoto and Sakisaka, 2012).
Moreover, nearly 45% of the proteins in the dataset localize to the ER and function in activities such as protein biogenesis, folding, sorting, trafficking, and degradation. At least nine proteins are identified in the ER-associated protein degradation (ERAD) pathway and ubiquitination system. ERAD monitors the biogenesis and folding of membrane and secretory proteins in the ER and targets misfolded proteins for ubiquitination and subsequent degradation (Ruggiano et al., 2014). And at least two members of the ER membrane protein complex (EMC3 and EMC6), which is engaged in protein folding, are identified in the dataset. Additionally, several proteins are identified in ER morphogenesis and tubular organization network.
More than 70 proteins are described with engaging in various protein-protein interactions, with nearly half of them (33 proteins) annotated as forming homo- and/or heteromeric subunits of complexes. All 18 of the ionotropic glutamate receptors are identified in this group, which is expected as the structural arrangements of the subunit pairings, the ligand binding domains, as well as the N-terminal domains are the focus of intense research and several conformations have been experimentally determined; for review see Green and Nayeem (2015). Furthermore, several members of protein families in the dataset form heteromeric subunits including BCAP29 and BCAP31, NDFIP1, and NDFIP2 as well as other proteins that self-associate to form homomeric units such as BVES, MGST2, TMEM109, TMEM18, SGPL1, STS, SLC27A1, and SLC31A1.
Transport and Trafficking
Overall, 40% of trispanins are engaged in transport activities, with nearly 20% involved in intracellular transport and protein trafficking as well as membrane and vesicle trafficking (Figure 2). The majority of the trispanin transport activity is the movement of cargo, for example proteins and other macromolecules, around the cell utilizing small compartments of membranes called transport vesicles. Nine proteins participate in membrane trafficking and include the four members of the cornichon family. The cornichon (CNIH1-4) family members function as cargo receptors in ER export and are involved in the selective transport of TGF-alpha family proteins in COPII vesicles (Zhang and Schekman, 2016), while CNIH4 also acts as a cargo-sorting receptor that recruits GPCRs into COPII vesicles for export from the ER to the cell surface (Sauvageau et al., 2014). Additionally, CNIH2 and CNIH3 co-assemble as auxiliary subunits of AMPA receptors where they increase surface expression of AMPARs and alter channel gating (Schwenk et al., 2009). TBC1D20 is another predicted trispanin involved in COPII-coated vesicle cargo loading and Rab GTPase activating activity (Haas et al., 2007).
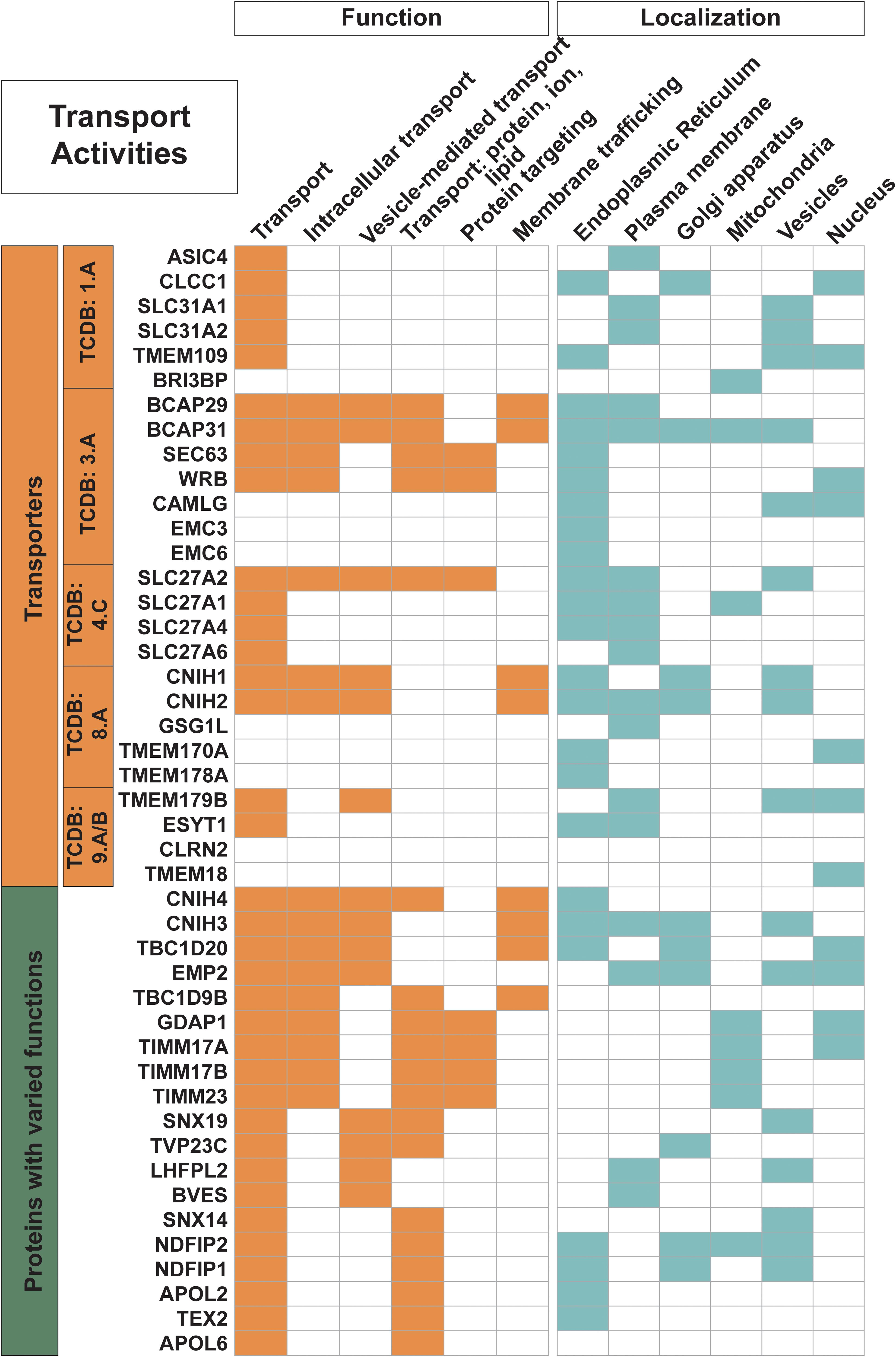
Figure 2. Trispanins involved in transport activities. All 26 proteins identified with a transporter classification database (TCDB) identifier (descriptive boxes on left) are included in this figure. Only 40 of the 61 total trispanins involved in transport activities are shown; however, all proteins are shown that are involved in intracellular transport; vesicle-mediated transport; transport of proteins, ions, and lipids; protein targeting; and membrane trafficking. The four members of the SLC27A1/2/4/6 also contain an enzyme commission number (EC) and are shown in Figure 3. Functional activities and localization descriptions derived from gene ontology (GO) Annotations (Huntley et al., 2015), PANTHER classifications (Mi et al., 2019), and the human protein atlas (Uhlén et al., 2015).
Nearly 30 proteins localize to vesicles including secretory vesicles (8 proteins), lysosomes (4), endosomes (8), exosomes (5), and Golgi-associated vesicles (4) to engage in a variety of activities. Furthermore, the dataset also contains proteins involved in vesicle formation and regulation, such as RAB5IF and EMC6, which both contain the Rab5 interacting protein Pfam domain (Rab5ip; PF07019). RAB5IF may function on endocytic vesicles as a receptor for rab5-GDP and be involved in the activation of RAB5A, which is an important regulator of the endocytic pathway (Hoffenberg et al., 2000), while EMC6 has been reported to interact with RAB5A and BECN1 and regulate autophagosome formation (Li et al., 2013). TBC1D9, also in the 3TM dataset, may regulate the membrane trafficking pathway to the late-endosome and/or Golgi apparatus in spermatocytes and in spermatogenesis (Nakamura et al., 2015). Another protein family with two mammalian homologs, the Nedd4 Family-interacting Proteins 1 and 2 (NDFIP1-2), is also involved in orchestrating protein trafficking, which may contribute to why they both localize to multiple regions, including the ER, Golgi apparatus and vesicles and NDFIP2 also localizes to the mitochondria. Both proteins bind to and activate members of the Nedd4 family of E3 ubiquitin ligases, which in turn targets proteins for degradation by the proteasome (Putz et al., 2008). NDFIP1-2 are important for protein trafficking via exosomes, which originate from late endosomes and multivesicular bodies (MVB) and provide a rapid manner of discarding unwanted proteins as well as functioning in cell to cell communication (Putz et al., 2008).
The 26 transporter proteins with an associated TCDB identifier include a variety of transporter classes. Six proteins are identified as alpha-type channels/pores (TCDB:1.A). This includes the important SLC31 family of copper transporters, which are involved in the maintenance of copper homeostasis and regulate intracellular copper concentration (Schweigel-Röntgen, 2014). Seven proteins are involved in transport systems that hydrolyze the diphosphate bond of inorganic pyrophosphate, ATP, or another nucleoside triphosphate to drive the active uptake or extrusion of a solute(s) (TCDB:3.A). Five of these proteins are involved in complexes of the previously mentioned Sec61 ER translocon. Four proteins are members of the acyl CoA ligase-coupled transporters (TCDB:4.C), which are group translocators that modify the transported substrate during the transport process. These four SLC27A proteins also engage in enzymatic activities; however, they are classified as transporters in this dataset to remove redundancy. Five proteins are identified as accessory factors involved in transport (TCDB:8.A) and four proteins are transporters of unknown classification (TCDB:9.A and 9.B). It is interesting to note that contrary to the many transporters that form oligomeric subunits to transport molecules across membranes (Alguel et al., 2016), only seven of the proteins identified in the dataset with a TCDB number are annotated to form homo- or heteromeric units. Many of the identified trispanin transporters appear to be engaged in other transport activity, for example intracellular transport, vesicle-mediated transport, and protein targeting.
Lipid Biogenesis and Metabolic Processes
Nearly 20% of the proteins in the dataset are involved in lipid metabolic processing and lipid transport (Figure 3). And as expected, nearly all of these proteins localize to the ER which is the main site for lipid synthesis. Four members of the 1-acylglycerol-3-phosphate O-acyltransferases (AGPAT) gene family (AGPAT1, LPCAT1, LPCAT2, and LPCAT4) are predicted to be trispanins. These enzymes are involved in maintaining the composition of fatty acyl chains that make up phospholipids, which are the major constituents of membranes (Wang and Tontonoz, 2019). Four members out of six of the SLC27A family of fatty acid transporters were predicted to contain three transmembrane helices (SLC27A1, SLC27A2, SLC27A4, and SLC27A6). The two other members of the family had conflicting transmembrane predictions from none to two to six and were not included in the dataset. This family is unusual in that their multifunctional activities include both transport and enzymatic activation of long chain fatty acids, which can then be used by the cell in many metabolic processes including phospholipid synthesis (Anderson and Stahl, 2013). Seven enzymes in the dataset are members of the Cytochrome P450 family, including four enzymes (CYP4F2, CYP4F11, CYP4F12, and CYP4B1) that play primary roles in the omega-hydroxylation of endogenous long chain and very long chain fatty acids, which include the physiologically important eicosanoids, prostaglandins, leukotrienes, and arachidonic acid (Johnson et al., 2015). Furthermore, CYP4F2 is primarily responsible for the formation of the important signaling metabolite 20-HETE. Catabolic processes are other functional activities trispanins are involved in, with approximately 16% (24 proteins) engaged in the dataset and 16 enzymes involved (shown in Figure 3). These proteins are involved in chemical reactions and pathways that result in the breakdown of substances including the apoptotic signaling pathway, alkaloid catabolic processes, steroid catabolic processes, and leukotriene B4 catabolic processes.
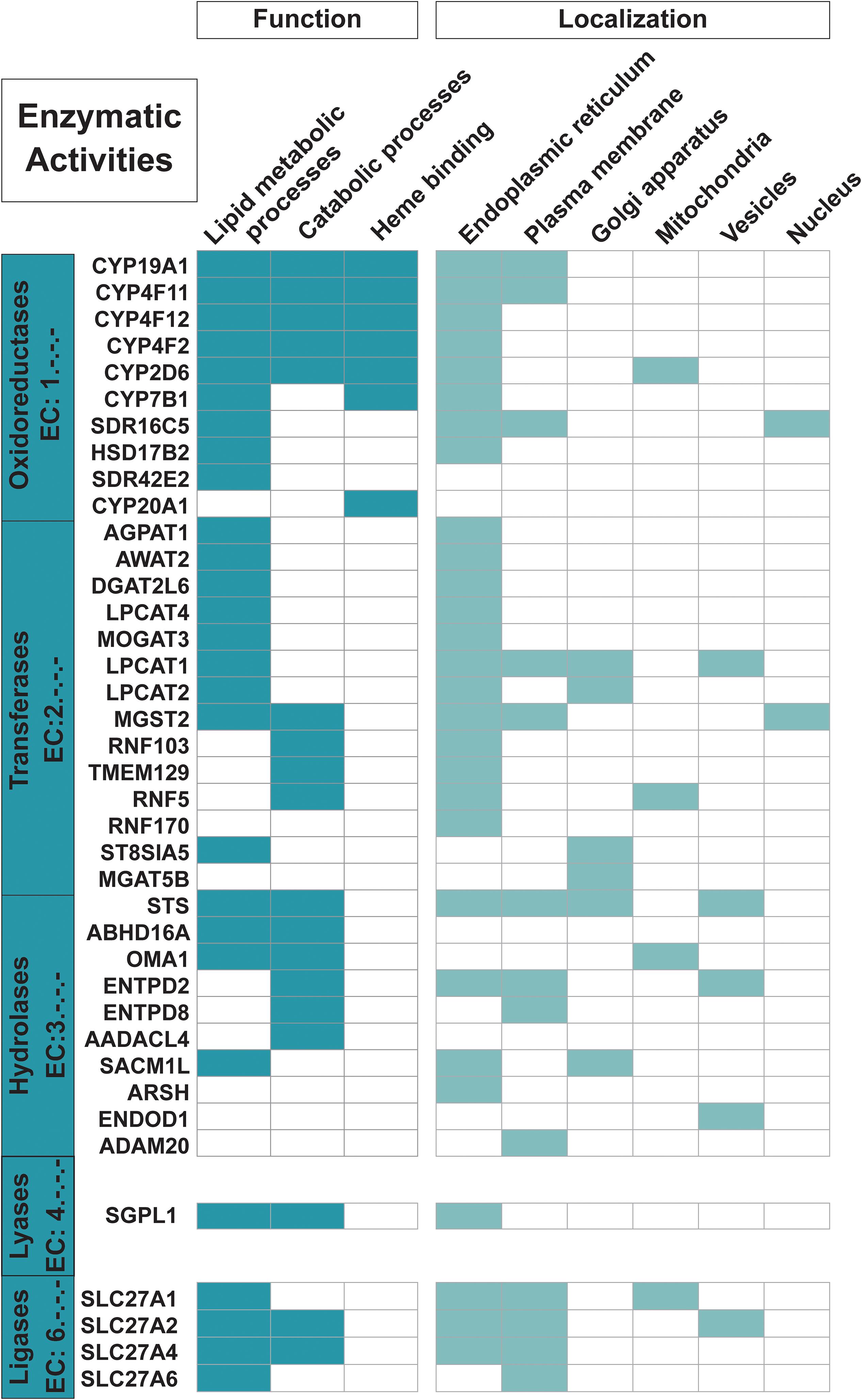
Figure 3. Proteins with enzymatic functions. The 35 trispanins with an associated Enzyme Commission (EC) number (descriptive boxes on left) are shown along with the predominant functional activities including lipid metabolic processes, catabolic processes, and heme binding. Only 22 of the 31 trispanins involved in lipid metabolic processes and 16 of the 24 total proteins active in catabolic processes are shown here. The four members of the SLC27A1/2/4/6 are also classified as transporters. Functional activities and localization descriptions derived from gene ontology (GO) annotations (Huntley et al., 2015), PANTHER classifications (Mi et al., 2019), and the human protein atlas (Uhlén et al., 2015).
Signal Transduction
Twenty-one proteins are identified as receptors while an additional five more proteins are involved in signal transduction activity (Figure 4). The 18 proteins of the ionotropic glutamate receptor family are characterized as trispanins. The proteins in this receptor family contain three transmembrane helices with a fourth central pore-like helix that does not fully span the membrane. Hence, membrane prediction software can give conflicting results with this family and the X-ray resolved structure for an AMPA-subtype glutamate receptor was referenced to include all members (Sobolevsky et al., 2009). The ionotropic glutamate receptor family is grouped into four different classes based on pharmacology and structural homology and include: the four AMPA receptors (GRIA1-4); the five kainate receptors (GRIK1-5); the seven NMDA receptors (GRIN1, GRIN2A-D, and GRIN3A-B); and two delta receptors (GRID1-2). Glutamate receptor subunits co-assemble to form ligand-gated ion channels that mediate fast excitatory synaptic transmission in the central nervous system and regulate a broad spectrum of processes in the brain, spinal cord, retina, and peripheral nervous system (Traynelis et al., 2010). As such, a significant volume of literature has been published regarding ionotropic glutamate receptors and will not be covered here; for example, see Traynelis et al. (2010); Reiner and Levitz (2018) for review. The three additional proteins identified as receptors in the dataset are: NACHT, LRR and PYD domains-containing protein 3 (NLRP3); P2X purinoceptor 7 (P2RX7); and Transmembrane protein PVRIG (PVRIG). As expected, virtually all of the receptors (except PVRIG) are annotated as involved in cell communication; moreover an additional 17 trispanins are also engaged cell communication such as Wnt signaling, insulin secretion, adiponectin-activated signaling, apoptotic signaling, and calcium-mediated signaling.
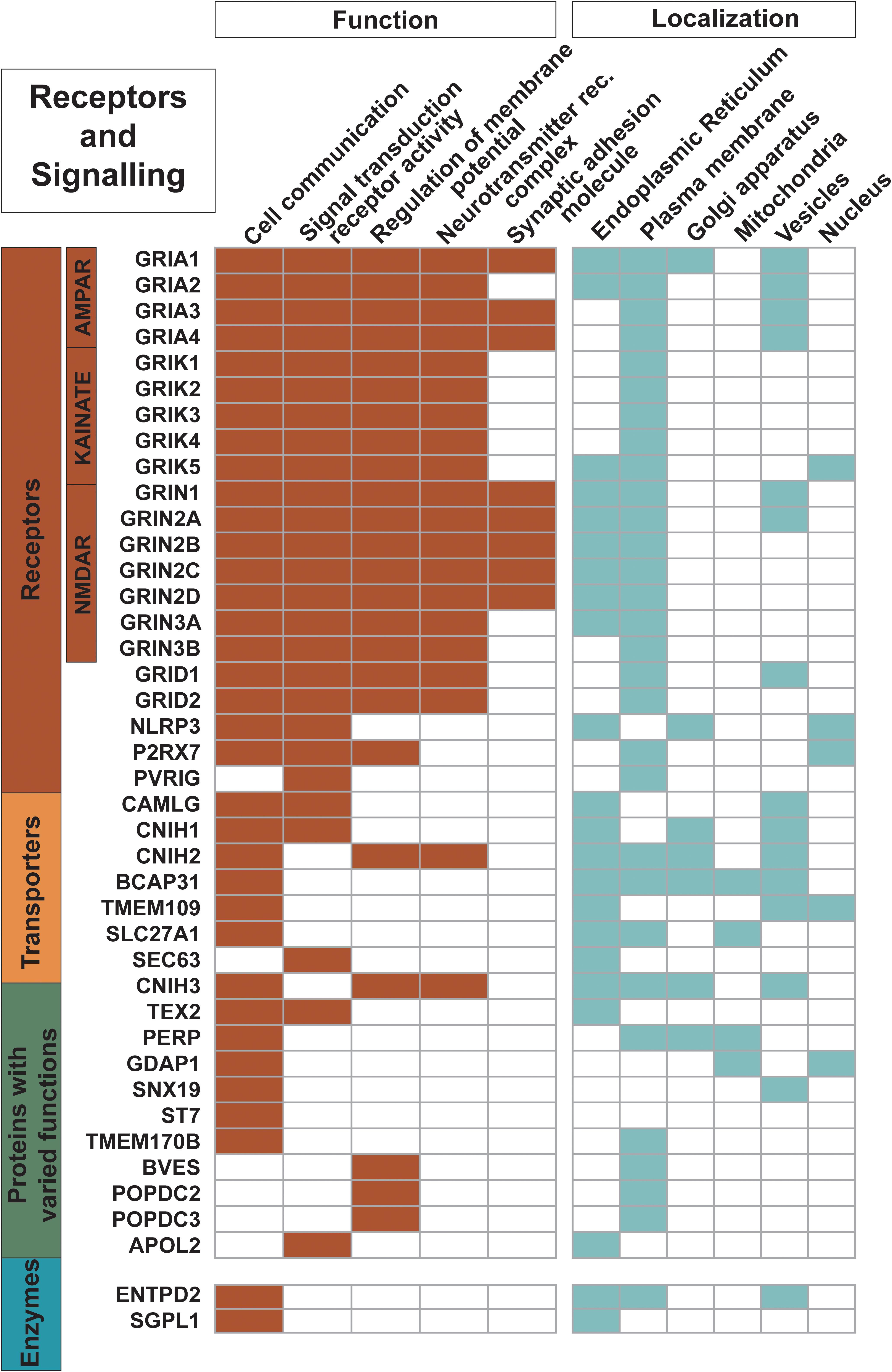
Figure 4. Proteins engaged as receptors and in signaling. The 21 receptors in the dataset along with other trispanins involved in cell communication; signal transduction receptor activity; regulation of membrane potential; neurotransmitter receptor complexes; and synaptic adhesion molecules are shown here. The 18 ionotropic glutamate receptors are heavily involved in these activities. Functional activities and localization descriptions derived from gene ontology (GO) annotations (Huntley et al., 2015), PANTHER classifications (Mi et al., 2019), and the human protein atlas (Uhlén et al., 2015).
Structural Support Activity
Seven proteins are identified as cytoskeletal proteins that provide structural support according to GO annotations and PANTHER protein class analysis (Figure 1). Four proteins (LIM2, NKG7, CLDND2, and EMP2) contain the PMP-22/EMP/MP20/Claudin family (PF00822) Pfam domain, whose members perform diverse functions. Proteins containing this domain are often predicted to have four transmembrane helices; however, these four more distantly related proteins to mammalian claudins were predicted to have 3TMs. The first N-terminal helix was identified to be a signal peptide that was detected with the recently released SignalP v5.0 software (Armenteros et al., 2019b). The 3TM predictions for these proteins are also in agreement with the membrane predictions of the human protein atlas data, which uses a majority decision method to determine presence of signal peptides and transmembrane regions (Fagerberg et al., 2010; Uhlén et al., 2015).
Membrane Topology and Signal Peptide Predictions
The orientation of the N- and C-termini in relation to the cytoplasmic side or non-cytoplasmic side (i.e., extracellular region or organelle lumen) can indicate functional activities proteins are engaged in. For example, GPCRs have extracellular N-termini that interact with cognate ligands and function in signal transduction (Coleman et al., 2017). However, the connection between function and membrane topology was difficult to distinguish with most of the trispanins as 72 of the trispanins had the N-terminus predicted in the cytoplasmic region (in) and 80 proteins had the N-terminus in a non-cytoplasmic environment (out). Receptors were the only group that had a skewed proportion with one protein with the N-terminus in the cytoplasmic environment while 20 proteins were non-cytoplasmic. The N-terminus of the 18 ionotropic glutamate receptors were all categorized as non-cytoplasmic (out) in accordance with the experimentally determined GRIA2 amino-terminal domain (Jin et al., 2009). Enzymes had 18 proteins with the N-terminus predicted in with 17 out; transporters had 11 in and 15 out; proteins with varied functional activities had 25 in and 18 out; and uncharacterized trispanins had 17 in and 10 out.
N-terminus signal peptides are short peptides that direct proteins to different locations in the cell including the ER, plasma membrane as well as other intracellular organelle membranes. Using multiple localization prediction resources (see section “Materials and Methods” for details), 50 N-terminal signal peptides were predicted in trispanins; 44 of these proteins have associated annotations that they localize to the ER, plasma membrane, mitochondria, and/or Golgi apparatus. Moreover, C-terminus signaling sequences as well as internal signal retention sequences are also methods of directing proteins to the ER (Ouzzine et al., 1999). Interestingly, in addition to the 64 trispanins that are already annotated as ER resident proteins, 53 more proteins in the dataset are predicted to localize to the ER and 17 are predicted to localize to the plasma membrane that do not have any associated annotation. Three additional trispanins were predicted to localize to the mitochondria and two more proteins were predicted to travel to the Golgi apparatus. One limitation with various localization resources seems to be that the results only give one localization area, while experimental evidence gives multiple locales the protein is found.
Disease Involvement and Drugs Targeting 3TMS
Nearly one-third (48 proteins) of the dataset was identified as being targeted by drugs and/or being associated with diseases. Disease-gene associations with the trispanins were particularly identified with neurological disorders including epilepsy, Alzheimer’s disease, amyotrophic lateral sclerosis, Huntington’s disease, Parkinson’s disease, and also mental or behavioral disorders such as substance dependence and intellectual disabilities (Figure 5A). The emphasis on neurodegenerative disorders is expected due to the important ionotropic glutamate receptor family and their involvement in the underlying pathophysiology of many disorders (Reiner and Levitz, 2018). Disease-gene associations with disorders of the eye, cancer, infectious viral diseases like pertussis, human papilloma virus, and hepatitis were also identified in the dataset.
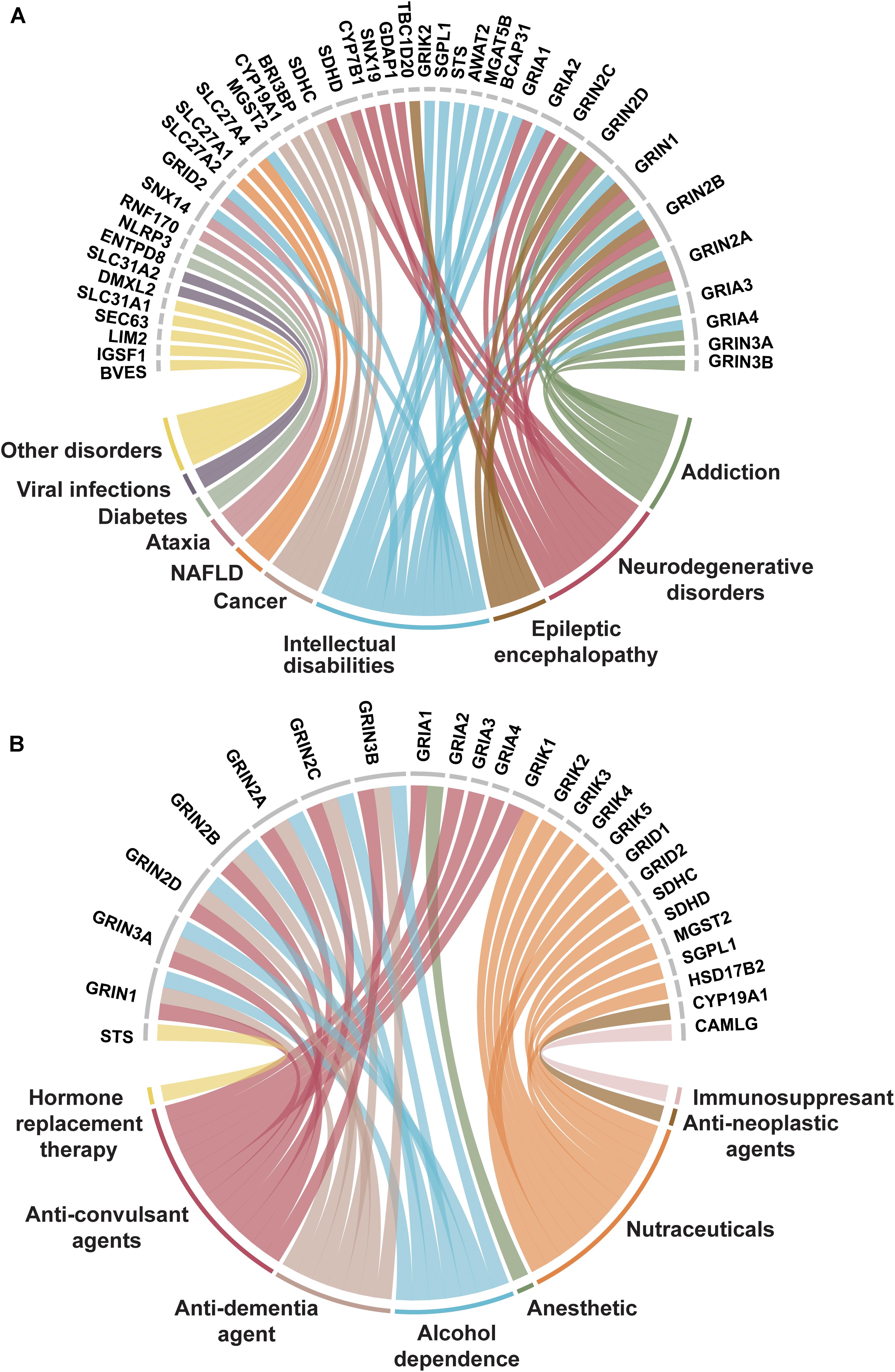
Figure 5. Trispanins identified in disease-gene associations and clinically established by FDA approved drugs. (A) Disease-gene associations with trispanins. The 41 proteins identified with disease-gene associations are shown at the top of the figure while the corresponding diseases are linked through color and identified in the bottom part. The disorders include: intellectual disability; addiction; neurodegenerative diseases including Alzheimer’s disease, amyotrophic lateral sclerosis, Huntington’s disease, and Parkinson’s disease; epileptic encephalopathy; cancer; non-alcoholic fatty liver disease (NAFLD); ataxia; diabetes mellitus; viral infections; and other. The disease-gene association data were collated from Jensen Diseases resource (Pletscher-Frankild et al., 2014) and DisGenet database (Piñero et al., 2017). (B) Trispanins targeted by FDA approved drugs. The 26 clinically established trispanins that have been targeted by FDA approved drugs are shown. The established drug targets are presented at the top of the figure and the corresponding therapeutic category of drugs that target each protein is color-linked and described on the bottom half. The FDA approved drug-target information comes from an updated dataset that provides curated information on FDA approved drug targets (Rask-Andersen et al., 2014; Attwood et al., 2018) as well as DrugBank (Wishart et al., 2017). Trispanins that were targeted and mediated the therapeutic action are included, while proteins as secondary targets or unknown mechanisms of action were not included. FDA: US Federal Drug Administration.
Of the 26 trispanins that are targeted by federal drug administration (FDA) approved drugs, approximately 70% of them are members of the ionotropic glutamate receptor family (Figure 5B). The FDA approved drugs that have established these trispanins as validated targets are therapeutically categorized as anesthetics, anti-neoplastic agents, anti-convulsants, nutraceuticals, and also anti-dementia agents for different conditions. The proteins in the dataset have also been targeted by immunosuppressive, and anti-addiction agents. The 3TM proteins have also been targeted by investigative drugs in clinical trials for such conditions as sleep disorders, depression, cardiovascular diseases, tinnitus, and Alzheimer’s disease. Membrane proteins have always been primary targets for pharmaceutical agents due to the critical functions they are involved with and the wide-reaching signaling pathways they participate in. And in fact, with the continued technological advancements in biopharmaceutical engineering, multipass membrane proteins are expected to be of increasing interest as antibody targets (Carter and Lazar, 2018).
Discussion
Many of the proteins that comprise the 3TM dataset function in aspects of cellular membrane remodeling and trafficking systems including membrane synthesis, lipid metabolism, protein trafficking, membrane imbedded complexes, and also ionotropic glutamate receptor signaling (Figure 6). Proteins that localize to organelle membranes are overrepresented in comparison to the entire Homo sapiens membrane proteome [Fold Enrichment (FE) = 1.57; FDR = 3.00e-04), and in particular the ER (FE = 2.15; FDR = 2.29e-07) and nuclear outer membrane- ER membrane network (FE = 2.40; FDR = 4.06e-07; all p-values < 0.05)] (Figure 7). This is consistent with the overrepresentation of proteins involved in fatty acid and lipid metabolic processes (FE = 6.15; FDR = 5.13e-04 and FE = 2.25; FDR = 1.41e-02, respectively) as the ER and Golgi apparatus are major sites of de novo membrane lipid synthesis and also hubs for directing protein trafficking (Fagone and Jackowski, 2009). Conjointly, the 3TM dataset contains a spectrum of proteins that are involved in membrane genesis through lipid biosynthesis and remodeling. Specifically, 3TM members of the SLC27A family uptake, transport and also have the unique ability to activate long chain fatty acids (Anderson and Stahl, 2013) while members of the AGPAT family are responsible for catalyzing fatty acid transfer between acyl donor and acceptor and the incorporation of fatty acids into various lipids such as phospholipids (Yamashita et al., 2014). Furthermore, the compositions of phospholipids are regulated after de novo synthesis in a process called fatty acid remodeling, and the dataset contains acyltransferases that are involved in both de novo synthesis as well as the important remodeling activities. The varied phospholipids that result from the remodeling process produce compositional membrane diversity, which is necessary for membrane fluidity and curvature (Hishikawa et al., 2008). Moreover, at least two of the AGPAT enzymes in the dataset function in phospholipid remodeling in the Lands’ cycle to produce phosphatidylcholine (PC) (Hishikawa et al., 2008), which is the most abundant glycerophospholipid in mammalian cell membranes – comprising ∼40–50% of total phospholipids (Wang and Tontonoz, 2019). Hence, with 20% of the proteins in the dataset involved in lipid metabolic processes and lipid transport, these are key activities for 3TMs.
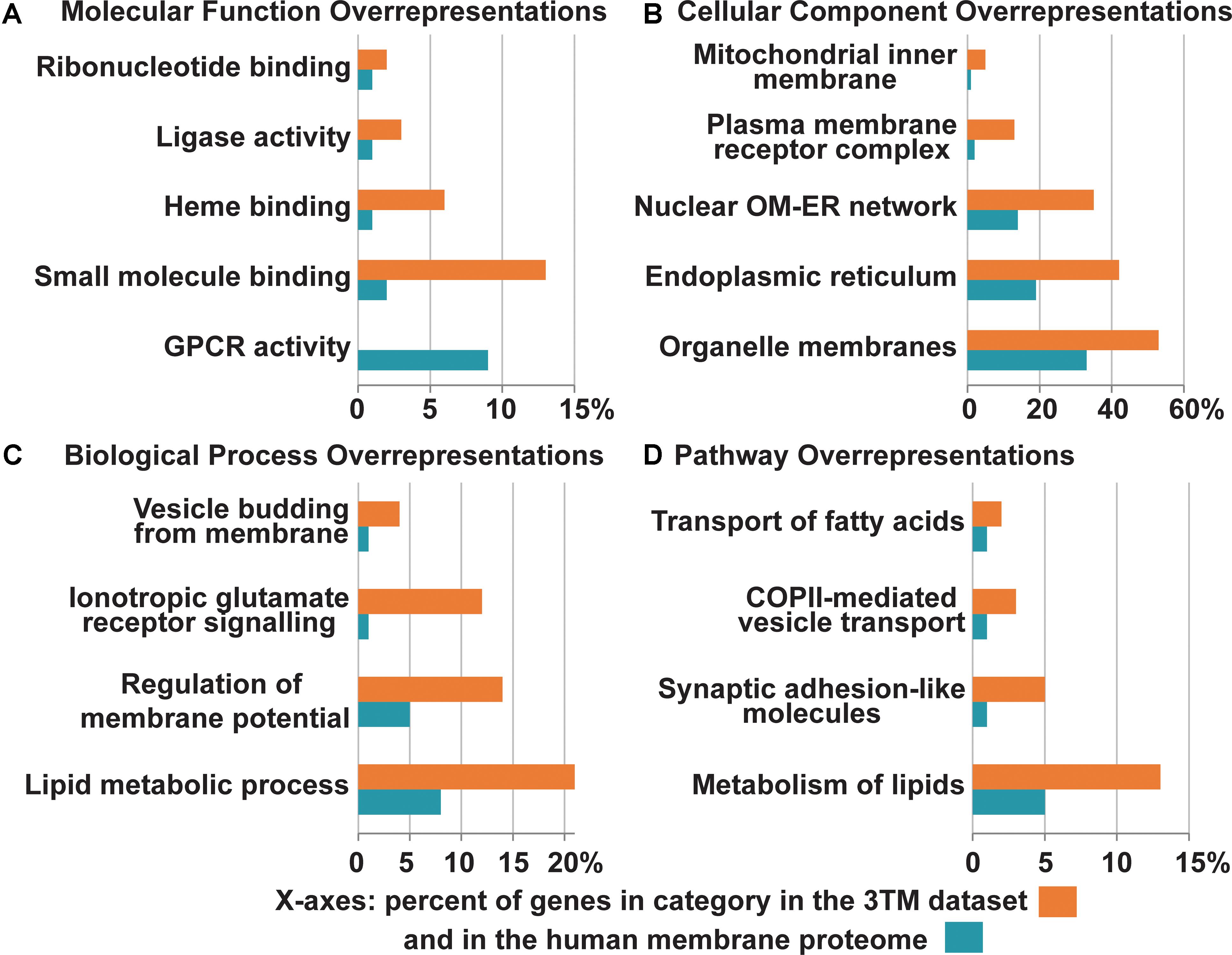
Figure 6. Overrepresentation of select Gene Ontology terms identified in the 3TM dataset in comparison to the entire human transmembrane proteome. The x-axes are the percent of genes in each category identified in the 3TM dataset (orange) in comparison to the percent of genes in the human transmembrane proteome (blue). Select pathway overrepresentations from the PANTHER Overrepresentation Test are presented in all four sections (see Supplementary Table S1 for full statistical information). (A) Molecular pathways. As the glutamate-gated ion channel protein family is an important receptor/transporter family involved in many activities, specific pathways were chosen to highlight the breadth of different activities that trispanins are overrepresented in. Twenty proteins are involved in small molecule binding, which are predominantly the ionotropic glutamate receptor families. Nine enzymes including the seven members of the cytochrome p450 family identified in the dataset engage in heme binding. However, one area that the 3TM dataset is significantly underrepresented in is in GPCR activity. (B) Cellular localizations. The 3TM dataset is rich in proteins that localize to organelle membranes, with the ER in particular and also the nuclear outer membrane-ER network. Trispanins that localize to the mitochondrial inner membrane are also overrepresented due to the proteins functioning in the mitochondrial import inner membrane translocase complex. The ionotropic glutamate receptor families compose the overrepresented group of plasma membrane receptor complexes. (C) Biological pathways. More than 30 trispanins are involved in lipid metabolic processes, including those involved in lipid synthesis and remodeling, activation of long chain fatty acids, and membrane genesis. Six proteins are involved with membrane trafficking and vesicle budding from the membrane. (D) Reactome pathway. Several pathways were found to be overrepresented in the Reactome pathways dataset (v65, released on 2019-03-12). The NMDAR and AMPAR glutamate families interact with synaptic adhesion-like proteins which function in protein-protein interactions at synapses in the neuronal system. Many of the proteins involved in the metabolism of lipids function in lipid biosynthesis, mobilization, transport, and activation. Several of the members of the SLC27A family function specifically in the transport of fatty acids. The proteins involved in vesicle-mediated transport via COPII components traffic cargo from the ER to the ER-Golgi intermediate compartment. The overrepresentation analysis is from the PANTHER (Mi et al., 2019) overrepresentation test (v14.1) with the gene ontology (GO) Annotation (Huntley et al., 2015) database released on 2019-07-03. Fisher’s Exact test was performed and the False Discovery Rate was calculated with p < 0.05. The human membrane protein identities is from Attwood et al. (2017) with 5723 of 5777 proteins and 150 of 152 3TM proteins successfully mapped using GO annotation. GPCR, G protein-coupled receptor; OM, outer membrane; ER, endoplasmic reticulum.
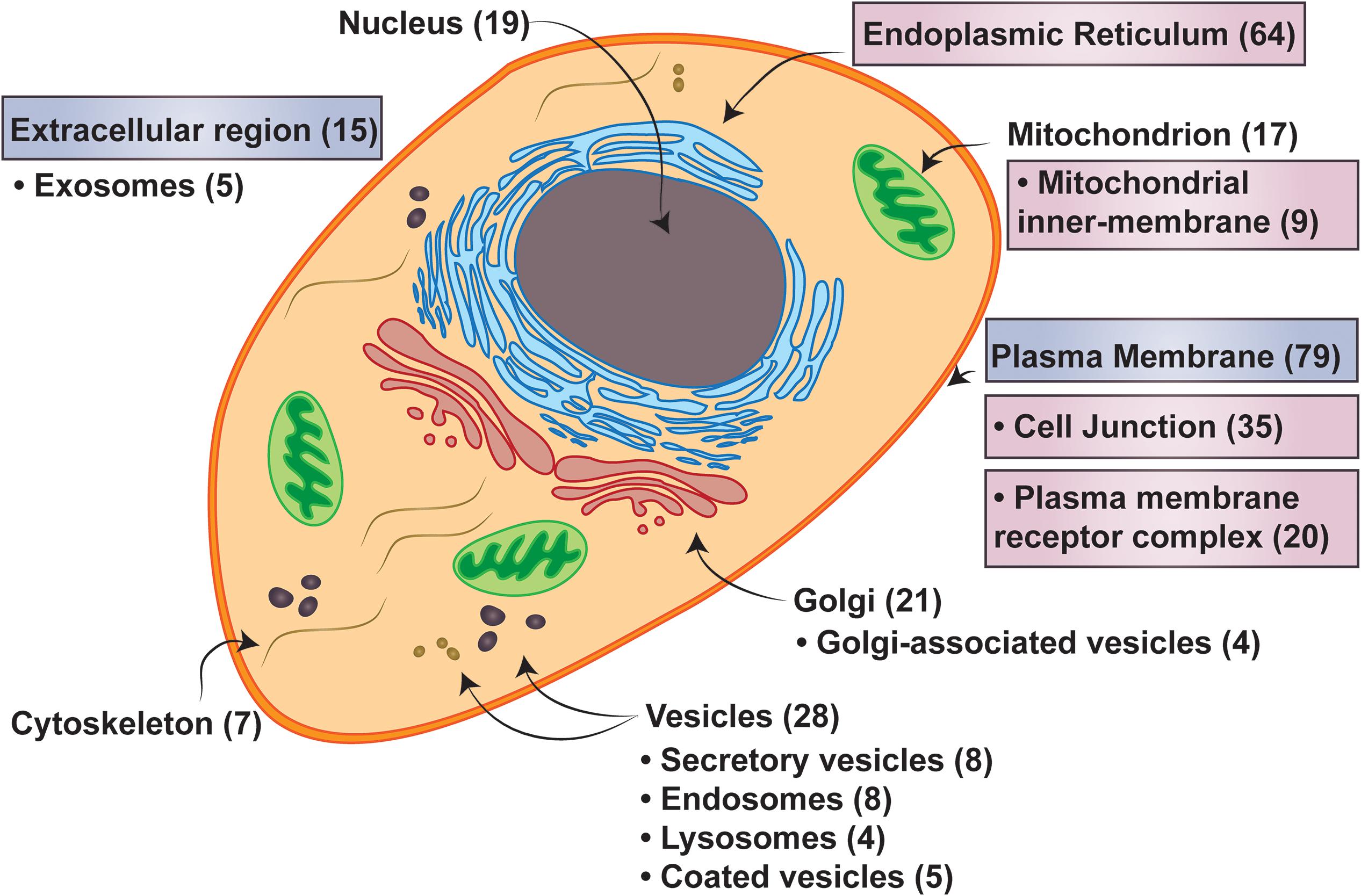
Figure 7. Cell localizations of three transmembrane spanning proteins. Major cellular localizations presented with the number of proteins identified in the 3TM dataset in parenthesis. Labels in boxes with pink hues are identified as statistically overrepresented, while the boxes with the blue hues are underrepresented in the 3TM dataset in comparison to the entire human membrane proteome (see Supplementary Table S1 for full statistical information). Localization data is extracted from QuickGO annotation web-based tool (Binns et al., 2009). The overrepresentation analysis is from the PANTHER (Mi et al., 2019) overrepresentation test (v14.1) with the gene ontology (GO) Annotation (Huntley et al., 2015) database released on 2019-07-03. Fisher’s Exact test was performed and the false discovery rate was calculated with p < 0.05. The human membrane protein identities is from Attwood et al. (2017) with 5723 of 5777 proteins successfully mapped and 150 of 152 trispanins successfully mapped using GO annotation.
Another interesting aspect of the 3TM dataset is that more than 20% of the proteins act as subunits of membrane-imbedded complexes, such as the TIMM23 translocase complex, OST complex, and the ER membrane complex. Proteins involved in both co-translational SRP dependent membrane insertion complexes as well as post-translational tail-anchored membrane insertion complexes are represented in the dataset. Moreover, proteins ensuring proper folding with the ER membrane complex as well as clearing misfolded proteins through the ERAD process are also classified in the dataset. Further literature investigation showed that GO annotation and PANTHER classifications have not yet included all relevant annotations for the 3TM dataset, and hence the overrepresentation analyses may yield conservative results in comparison to the actual functional activities the proteins are involved in. For example, BCAP29 and BCAP31 appear to form a complex that associates with the ER translocation apparatus and interacts with translation substrates (Wilson and Barlowe, 2010), but there is not associated GO annotations regarding these functions yet.
It is notable that proteins identified as receptors are under-represented in the 3TM dataset, with 13% identified in comparison to ∼25% in the entire Homo sapiens membrane proteome. However, the percent of enzymes (22%) and transporters (16%) are roughly comparable to the membrane proteome, which has 20% and 17%, respectively. As all eighteen members of the ionotropic glutamate receptor family are included in the 3TM dataset and they are involved in several unique essential activities, many of the functions they are involved in are overrepresented in the PANTHER analysis. Glutamate receptors play vital roles in the mediation of excitatory synaptic transmission in which neurons communicate with each other. Hence, for example, neurotransmitter receptor activity and regulation of postsynaptic membrane potential are overrepresented in the analysis (FE = 6.11; FDR = 1.73e-06 and FE = 7.51; FDR = 5.90e-06, respectively). However, aside from this glutamate receptor family, the 3TM dataset is not heavily involved in direct receptor signaling, although they may act as accessory proteins in receptor complexes such as with CNIH2 and CNIH3.
Tissue Expression and Enrichment
The TissueEnrich analysis demonstrated that almost 22% of the proteins in the 3TM dataset had enriched or enhanced expression in the cerebral cortex in comparison to the membrane proteome of Homo sapiens, and importantly that seven of these 37 proteins are uncharacterized. While it might be expected that protein expression might be overrepresented in the brain due to the large ionotropic glutamate receptor family, it is surprising that nearly 20% of the proteins specifically expressed in the brain are completely uncharacterized. Additionally, the testis had the second most abundant number of tissue specific genes expressed there, although they were not significantly overexpressed in comparison to the membrane proteome. Previous analysis has shown that more than 80% of all human proteins are expressed in the testis (Uhlén et al., 2015), so perhaps the high number of testis expressed genes is not unusual. However, three of the eleven proteins are uncharacterized, lending further curiosity into the nature of the not yet classified proteins into the dataset.
Uncharacterized Proteins and Proposed Functional Activities
Roughly 15% of the proteins in the dataset are uncharacterized, yet several interact with other 3TM proteins and, in particular, important membrane complexes. Furthermore, they are localized to specific subcellular locations, such as the ER, and expressed in specific tissues, including the cerebral cortex, testis, and spleen (Table 1). Hence, using bioinformatic analyses and literature research, we can speculate on the activities these uncharacterized proteins are engaged in. For example, we might suspect that FAM8A1 is involved in ER protein folding or targeting due to its localization in the Golgi apparatus, expression in many tissues, and interactions with the fellow 3TM protein EMC3, which is engaged in protein folding, and also HERPUD1, which is involved with ERAD. Recently, it was shown that FAM8A1 has a central role as a cofactor with HERPUD1 in the assembly and activity of the Hrd1 complex, which is engaged in ERAD of aberrant proteins (Schulz et al., 2017). Interestingly, researchers previously determined that FAM8A1, which is inserted within a human endogenous retrovirus, has ubiquitous mRNA expression and a testis-specific transcript present in the haploid phase of spermatogenesis (Jamain et al., 2001). TMCO4 is another uncharacterized protein we might infer is involved in protein targeting or trafficking as it localizes to the ER, is expressed at varying levels in many tissues, and has been annotated from experimental data to interact with VPS29, which is a vacuolar sorting protein that prevents missorting of transmembrane proteins into the lysosomal degradation pathway.
Intriguingly, the three uncharacterized proteins TMEM235, TMEM151A, and TMEM151B exhibit enriched tissue-expression in the cerebral cortex and all are identified in String interaction networks (Szklarczyk et al., 2015) to co-express with MAG, an adhesion molecule that is a myelin-associated glycoprotein, and also with OPALIN, which is the oligodendrocytic myelin paranodal and inner loop protein. TMEM151A and TMEM151B, which both contain a conserved but uncharacterized Pfam domain (PF14857), co-express with TMEM235, but not with each other. TMEM235, as well as TMEM114 which is another uncharacterized 3TM protein, both contain the Claudin_2 conserved Pfam domain (PF13903) and were shown to resolve within the distantly related voltage dependent calcium channel gamma subunits (CACNGs) rather than within the 27 members of the human Claudin family. However, the functional activities of these uncharacterized proteins have yet to be determined, although it is interesting to speculate that with the conserved claudin domain and co-expression with other adhesion and myelin associated proteins, this trio of uncharacterized proteins may be involved as structural components of oligodendrocytic cells or loops.
Piecing together the diverse information on the uncharacterized protein TMEM178B reveals a potentially important protein that exhibits enhanced expression in the cerebral cortex and localizes to vesicles and the nucleoli. It also contains the conserved Claudin_2 Pfam domain (PF13903), of which family members perform diverse functions. In a recent analysis that produced a protein-protein interaction map of the TNF-induced NF-κB signal transduction pathway, TMEM178B was found to interact with two proteins, TNIP2 and TRAF2, which both inhibit or regulate NF-κB signaling and kinase activity and are also involved in apoptotic functions (Van Quickelberghe et al., 2018). Furthermore, TMEM178B-BRAF gene fusions were identified in two primary mucosal malignant melanoma cases, which are more aggressive than cutaneous melanomas (Kim et al., 2017). And another recent study concluded that oncogenic BRAF fusions may be targeted therapeutically by the combination of a MEK inhibitor with a PI3K or CDK4/6 inhibitor (Kim et al., 2017). Hence, while the exact functional activities of TMEM178B remain unknown, results suggest that it may be oncogenic and involved in disease progression, and we might infer that it could be associated with important signaling pathways.
Materials and Methods
Homo Sapiens Proteome Retrieval
The Homo sapiens current protein sequences (CCDS_protein.current.faa) using the GRCh38.p7 assembly with consensus coding sequence (CCDS) (Pruitt et al., 2009) annotation was downloaded from the National Center for Biotechnology Information (NCBI) website (National Center for Biotechnology Information, 2018). The following files were also downloaded from this website: CCDS2UniProtKB.current.txt, which gives the UniProtKB identifier corresponding to each CCDS identifier; CCDS_attributes.current.txt, which shows the corresponding gene and various attributes for each CCDS identifier; CCDS2Sequence.current.txt, with information on the nucleotide and protein ID and status in CCDS; and CCDS.current.current.txt, specifying more on CCDS status along with other information. CCDS is a collaborative effort to produce consensus annotation of a standard set of human genes that also includes alternative splicing sequences. The annotation uses manual curation through the Havana (Havana Annotation by Wellcome Sanger Institute, 2018) and RefSeq groups as well as automatic methods from ENSEMBL (Ensembl genome browser 91, 2018) and NCBI computational pipelines.
Transmembrane Prediction
Transmembrane prediction algorithms have difficulty differentiating between N-terminal alpha-helices and cleavable signal peptides. Thus, the protein sequences were assessed using SignalP v4.1 on an installment on the UPPMAX high-performance computing service. The default parameters were used with eukaryotic type of organism and the best method was selected which indicated transmembrane regions might be present. The mature sequences with the signal peptides excised were collated and evaluated with TOPCONS-single (Hennerdal and Elofsson, 2011) transmembrane prediction web server to assess membrane topology, including the number of membrane-spanning helices and the orientation of the N- and C-terminals. Prediction methods use different algorithms to discriminate transmembrane helices which results in possibly different numbers of helices within a protein. To improve the accuracy of transmembrane prediction methods, a consensus or majority decision using several different algorithms is preferred. TOPCONS-single, which is suitable to use for large proteome datasets, is a consensus method that incorporates multiple methods and uses a hidden-Markov model to estimate the consensus topology for a predicted transmembrane protein. The default methods used were SCAMPI-single (Bernsel et al., 2008), S-TMHMM (Viklund and Elofsson, 2004), HMMTOP (Tusnády and Simon, 2001), and MEMSAT (Jones et al., 1994). The resulting proteins identified as 2TM 3TM, and 4TM were retrieved. This included canonical sequences as well as isoforms. In early 2019 SignalP v5.0 was released that improved signal peptide predictions by using deep neural networks (Armenteros et al., 2019b) and the original sequence versions of the 2TM, 3TM, and 4TM groups of proteins were re-evaluated using SignalP v5.0 and then assessed with TOPCONS2.0. This transmembrane prediction software is also a consensus membrane prediction server, however, it is a more recent iteration of the TOPCONS series and can more successfully predict membrane topologies (Tsirigos et al., 2015). Furthermore, TOPCONS2.0 was chosen due to the robust benchmark sets used in assessing the software which posited ∼80% accuracy in predicting transmembrane proteins (Tsirigos et al., 2015), whereas other resources used smaller benchmark sets to state possibly higher accuracy. As reliability is still an issue for any membrane prediction resource, we further attempted to assess questionable proteins and protein families in the dataset through corroboration with other transmembrane prediction resources such as the Human Transmembrane Proteome database (Dobson et al., 2015), and also the human protein atlas which also uses a majority consensus method to determine signal peptides and transmembrane topology (Fagerberg et al., 2010). Additionally, comparisons of specific proteins or domains to experimentally determined 3D structures were evaluated. SignalP v5.0, SignalP v4.1, and TOPCONS2 were used to assess the presence of signal peptides and N-terminal topology. Several localization prediction resources were used to asses predicted versus annotated localization regions. ERPred uses split amino acid composition as support vector machine input to predict ER resident proteins (Kumar et al., 2017). DeepLoc uses sequence information with deep neural networks to predict subcellular localization of proteins (Almagro Armenteros et al., 2017). TargetP-2.0 uses deep learning to predict N-terminal signal peptides and mitochondrial transit peptides among others (Armenteros et al., 2019a). The predicted 3TM dataset was assessed for canonical or alternative splicing sequences using the UniProt identifiers (obtained from CCDS2UniProtKB.current.txt), where isoforms typically have an additional number at the end of the identifier. The canonical sequence is determined as the most prevalent, the most similar to orthologous sequences, the amino acid properties in the sequence, or else the longest sequence.
Protein Annotation and Information
Universal protein resource, UniProt, is a central repository for protein annotation data with both manually curated and automatically analyzed information (Consortium, 2015). Protein annotations for the canonical 3TM proteins were obtained from the website and included: review status, transporter classification number, EC, GO annotation terms, and protein family information. The 3TM proteins were also searched against the Pfam (Finn et al., 2016) database (v31) using an installment on the UPPMAX high-performance computing service. Pfam is a collection of protein families and domains, and also higher-level groupings of related entries called clans, and are represented by multiple sequence alignments and hidden Markov models. The IUPHAR/BPS Guide to Pharmacology (Harding et al., 2018) targets_and_families.csv file was downloaded and evaluated to aid in functional classifications of the dataset.
The Jensen lab DISEASES database (Pletscher-Frankild et al., 2014) is a resource that integrates evidence by assigning confidence scores on disease-gene associations from automatic text mining, manually curated literature, cancer mutation data, and genome-wide association studies. The human_disease_knowledge_filtered.tsv file was downloaded from their website and evaluated for gene-disease associations with the 3TM dataset. Additionally the DisGeNET drug encyclopedia was also utilized to assess genes associated to human diseases (Piñero et al., 2017). The evidence metrics limited, moderate, and strong were used to identify relevant associations. To identify possible drug targets in the dataset, the DrugBank annotations that were obtained via UniProt were further investigated (Wishart et al., 2017). Furthermore, an updated dataset from Rask-Andersen et al. (2014) that provides curated information for all current targeted as well as investigative proteins in clinical trials was obtained (Attwood et al., 2018).
The Kyoto Encyclopedia of Genes and Genomes, KEGG, is a database resource that assigns functional meaning to genes and genomes at molecular and higher levels (Kanehisa et al., 2017). BlastKOALA, which is the KEGG webserver for automatic annotation of query sequences, was used to obtain information on the pathways, or molecular interactions and relations, for networks that included: metabolism, genetic information processing, environmental information processing, cellular processes, organismal systems, human diseases, and drug development.
Gene Enrichment Analyses: Gene Ontology Annotation and TissueEnrich Analyses
The PANTHER Classification System (version 14.1; released July 11, 2019) (Mi et al., 2019) overrepresentation test was used to analyze gene enrichment in the 3TM dataset in comparison to the entire human membrane proteome. PANTHER is a comprehensive resource for the functional classification of genes using the GO annotations (released July 03, 2019). The PANTHER Overrepresentation Test uses the Fisher’s exact test, which assumes a hypergeometric distribution that is more accurate for smaller gene lists, and also uses the Benjamini-Hochberg False Discovery Rate (FDR) correction (p < 0.05) to control the false positive rate in the statistical test results (Mi et al., 2019). The annotation data sets included PANTHER GO-Slim Molecular Function, PANTHER GO-Slim Biological Process, and PANTHER GO-Slim Cellular Component, as well as the GO complete sets. The Reactome pathways (version 65; released March 12, 2019) data set and also PANTHER protein classes (version 14.1; released March 12, 2019) were also used. QuickGO annotations were also applied for additional protein annotation (Binns et al., 2009; Huntley et al., 2015). The reference protein list for the homo sapiens membrane proteome was obtained from (Attwood et al., 2017).
The recently released website resource TissueEnrich: Tool for tissue-specific gene enrichment in human and mouse (Jain and Tuteja, 2019) was used to analyze tissue-specific gene enrichment in the 3TM dataset in comparison to the human membrane proteome. TissueEnrich defines tissue-specific genes using RNA-Seq data from the human protein atlas, GTEx, and mouse ENCODE data sets. Tissue-specific genes are defined as: Not Expressed: Genes with an expression level less than 1 (TPM or FPKM) across all the tissues; Tissue Enriched: Genes with an expression level greater than or equal to 1 (TPM or FPKM) that also have at least five-fold higher expression levels in a particular tissue compared to all other tissues; Group Enriched: Genes with an expression level greater than or equal to 1 (TPM or FPKM) that also have at least five-fold higher expression levels in a group of 2–7 tissues compared to all other tissues, and that are not considered Tissue Enriched; and Tissue Enhanced: Genes with an expression level greater than or equal to 1 (TPM or FPKM) that also have at least five-fold higher expression levels in a particular tissue compared to the average levels in all other tissues, and that are not considered Tissue Enriched or Group Enriched. TissueEnrich uses the hypergeometric test to calculate the enrichment of tissue-specific genes in the 3TM data set and the Benjamini-Hochberg correction for multiple hypotheses (Jain and Tuteja, 2019). The parameter P-adjusted was selected in the Histogram Plot Options. The human protein atlas dataset was used in the enrichment tests.
Uncharacterized Proteins
The uncharacterized proteins were investigated further using the Cell Atlas (Thul et al., 2017) to obtain subcellular localizations where possible. String Protein-Protein Interaction Networks (Szklarczyk et al., 2015) and IntAct Molecular Interaction Database (Orchard et al., 2014) were utilized to retrieve interacting proteins with emphasis selected for other 3TM proteins within the database. The functions of the interacting proteins were obtained from UniProt (Consortium, 2015).
All analysis and classifications were performed using local Python and Perl script and SQL databases (sqlite3). Adobe Illustrator CS6 was used for the figures.
Conclusion
This analysis characterizes the structurally similar 3TM group and assimilates information on statistically relevant functional activities along with cellular localizations, tissue enrichment patterns, and protein-protein interaction networks to describe the prevailing functional activities of this group of proteins. Trispanins contain many evolutionarily conserved proteins that are predominantly localized to intracellular organelles and specifically to the ER. This group of proteins is primarily involved in aspects of cellular membrane composition and trafficking systems including membrane synthesis, protein trafficking, structural components, and are members of important membrane complexes. Further, the 3TM dataset contains the large and important ionotropic glutamate receptor superfamily which is involved in fast signal transduction in the brain. The methodology employed in this study uses bioinformatic analyses to identify uncharacterized proteins potentially involved in significant activities or disease pathways and provides means to reasonably speculate on the functional activities of several intriguing uncharacterized proteins.
Data Availability Statement
All datasets generated for this study are included in the article/Supplementary Material.
Author Contributions
MA contributed in conceiving the project, created the dataset, analyzed the data, and drafted the manuscript. HS contributed in conceiving the project and editing the manuscript.
Funding
The computations were performed on resources provided by SNIC through Uppsala Multidisciplinary Center for Advanced Computational Science (UPPMAX) under Project SNIC b2010006. This work was supported by the Swedish Research Council.
Conflict of Interest
The authors declare that the research was conducted in the absence of any commercial or financial relationships that could be construed as a potential conflict of interest.
Supplementary Material
The Supplementary Material for this article can be found online at: https://www.frontiersin.org/articles/10.3389/fcell.2019.00386/full#supplementary-material
References
Alguel, Y., Cameron, A. D., Diallinas, G., and Byrne, B. (2016). Transporter oligomerization: form and function. Biochem Soc Trans 44, 1737–1744. doi: 10.1042/BST20160217
Almagro Armenteros, J. J., Sønderby, C. K., Sønderby, S. K., Nielsen, H., and Winther, O. (2017). DeepLoc: prediction of protein subcellular localization using deep learning. Bioinformatics 33, 3387–3395. doi: 10.1093/bioinformatics/btx431
Almén, M. S., Nordström, K. J., Fredriksson, R., and Schiöth, H. B. (2009). Mapping the human membrane proteome: a majority of the human membrane proteins can be classified according to function and evolutionary origin. BMC Biology 7:50. doi: 10.1186/1741-7007-7-50
Anderson, C. M., and Stahl, A. (2013). SLC27 fatty acid transport proteins. Molecular Aspects of Medicine 34, 516–528. doi: 10.1016/j.mam.2012.07.010
Armenteros, J. J. A., Salvatore, M., Emanuelsson, O., Winther, O., Heijne, G., von Elofsson, A., et al. (2019a). Detecting sequence signals in targeting peptides using deep learning. Life Science Alliance 2, e201900429. doi: 10.26508/lsa.201900429
Armenteros, J. J. A., Tsirigos, K. D., Sønderby, C. K., Petersen, T. N., Winther, O., Brunak, S., et al. (2019b). SignalP 5.0 improves signal peptide predictions using deep neural networks. Nature Biotechnology 37, 420. doi: 10.1038/s41587-019-0036-z
Ashburner, M., Ball, C. A., Blake, J. A., Botstein, D., Butler, H., Cherry, J. M., et al. (2000). Gene Ontology: tool for the unification of biology. Nat Genet 25, 25–29. doi: 10.1038/75556
Attwood, M. M., Krishnan, A., Almén, M. S., and Schiöth, H. B. (2017). Highly diversified expansions shaped the evolution of membrane bound proteins in metazoans. Scientific Reports 7, 12387. doi: 10.1038/s41598-017-11543-z
Attwood, M. M., Krishnan, A., Pivotti, V., Yazdi, S., Almén, M. S., and Schiöth, H. B. (2016). Topology based identification and comprehensive classification of four-transmembrane helix containing proteins (4TMs) in the human genome. BMC Genomics 17:268. doi: 10.1186/s12864-016-2592-7
Attwood, M. M., Rask-Andersen, M., and Schiöth, H. B. (2018). Orphan Drugs and Their Impact on Pharmaceutical Development. Trends in Pharmacological Sciences 39, 525–535. doi: 10.1016/j.tips.2018.03.003
Bernsel, A., Viklund, H., Falk, J., Lindahl, E., Heijne, G., and von Elofsson, A. (2008). Prediction of membrane-protein topology from first principles. PNAS 105, 7177–7181. doi: 10.1073/pnas.0711151105
Binns, D., Dimmer, E., Huntley, R., Barrell, D., O’Donovan, C., and Apweiler, R. (2009). QuickGO: a web-based tool for Gene Ontology searching. Bioinformatics 25, 3045–3046. doi: 10.1093/bioinformatics/btp536
Braunger, K., Pfeffer, S., Shrimal, S., Gilmore, R., Berninghausen, O., Mandon, E. C., et al. (2018). Structural basis for coupling protein transport and N-glycosylation at the mammalian endoplasmic reticulum. Science 360, 215–219. doi: 10.1126/science.aar7899
Carter, P. J., and Lazar, G. A. (2018). Next generation antibody drugs: pursuit of the “high-hanging fruit.” Nature Reviews Drug Discovery 17, 197–223. doi: 10.1038/nrd.2017.227
Coleman, J. L. J., Ngo, T., and Smith, N. J. (2017). The G protein-coupled receptor N-terminus and receptor signalling: N-tering a new era. Cellular Signalling 33, 1–9. doi: 10.1016/j.cellsig.2017.02.004
Consortium, T. U. (2015). UniProt: a hub for protein information. Nucl. Acids Res. 43, D204–D212. doi: 10.1093/nar/gku989
Deamer, D. (2017). The Role of Lipid Membranes in Life’s Origin. Life (Basel) 7, 5. doi: 10.3390/life7010005
Demishtein-Zohary, K., and Azem, A. (2017). The TIM23 mitochondrial protein import complex: function and dysfunction. Cell Tissue Res 367, 33–41. doi: 10.1007/s00441-016-2486-7
Dobson, L., Reményi, I., and Tusnády, G. E. (2015). The human transmembrane proteome. Biology Direct 10, 31. doi: 10.1186/s13062-015-0061-x
Ensembl genome browser 91 (2018). Available at: https://www.ensembl.org/index.html (accessed February 2, 2018).
Fagerberg, L., Jonasson, K., von Heijne, G., Uhlén, M., and Berglund, L. (2010). Prediction of the human membrane proteome. Proteomics 10, 1141–1149. doi: 10.1002/pmic.200900258
Fagone, P., and Jackowski, S. (2009). Membrane phospholipid synthesis and endoplasmic reticulum function. J Lipid Res 50, S311–S316. doi: 10.1194/jlr.R800049-JLR200
Finn, R. D., Coggill, P., Eberhardt, R. Y., Eddy, S. R., Mistry, J., Mitchell, A. L., et al. (2016). The Pfam protein families database: towards a more sustainable future. Nucleic Acids Res 44, D279–D285. doi: 10.1093/nar/gkv1344
Gabaldón, T., and Pittis, A. A. (2015). Origin and evolution of metabolic sub-cellular compartmentalization in eukaryotes. Biochimie 119, 262–268. doi: 10.1016/j.biochi.2015.03.021
Green, T., and Nayeem, N. (2015). The multifaceted subunit interfaces of ionotropic glutamate receptors. J Physiol 593, 73–81. doi: 10.1113/jphysiol.2014.273409
Griffiths, G. (2007). Cell evolution and the problem of membrane topology. Nature Reviews Molecular Cell Biology 8, 1018–1024. doi: 10.1038/nrm2287
Guarani, V., McNeill, E. M., Paulo, J. A., Huttlin, E. L., Fröhlich, F., Gygi, S. P., et al. (2015). QIL1 is a novel mitochondrial protein required for MICOS complex stability and cristae morphology. eLife 4, e06265. doi: 10.7554/eLife.06265
Haas, A. K., Yoshimura, S., Stephens, D. J., Preisinger, C., Fuchs, E., and Barr, F. A. (2007). Analysis of GTPase-activating proteins: Rab1 and Rab43 are key Rabs required to maintain a functional Golgi complex in human cells. Journal of Cell Science 120, 2997–3010. doi: 10.1242/jcs.014225
Harayama, T., and Riezman, H. (2018). Understanding the diversity of membrane lipid composition. Nature Reviews Molecular Cell Biology 19, 281–296. doi: 10.1038/nrm.2017.138
Harding, S. D., Sharman, J. L., Faccenda, E., Southan, C., Pawson, A. J., Ireland, S., et al. (2018). The IUPHAR/BPS Guide to PHARMACOLOGY in 2018: updates and expansion to encompass the new guide to IMMUNOPHARMACOLOGY. Nucleic Acids Res 46, D1091–D1106. doi: 10.1093/nar/gkx1121
Havana Annotation by Wellcome Sanger Institute. (2018). Available at: http://www.sanger.ac.uk/science/projects/manual-annotation (accessed February 2, 2018).
Hennerdal, A., and Elofsson, A. (2011). Rapid membrane protein topology prediction. Bioinformatics 27, 1322–1323. doi: 10.1093/bioinformatics/btr119
Hishikawa, D., Shindou, H., Kobayashi, S., Nakanishi, H., Taguchi, R., and Shimizu, T. (2008). Discovery of a lysophospholipid acyltransferase family essential for membrane asymmetry and diversity. Proc Natl Acad Sci U S A 105, 2830–2835. doi: 10.1073/pnas.0712245105
Hoffenberg, S., Liu, X., Nikolova, L., Hall, H. S., Dai, W., Baughn, R. E., et al. (2000). A Novel Membrane-anchored Rab5 Interacting Protein Required for Homotypic Endosome Fusion. J. Biol. Chem. 275, 24661–24669. doi: 10.1074/jbc.M909600199
Huntley, R. P., Sawford, T., Mutowo-Meullenet, P., Shypitsyna, A., Bonilla, C., Martin, M. J., et al. (2015). The GOA database: Gene Ontology annotation updates for 2015. Nucleic Acids Res 43, D1057–D1063. doi: 10.1093/nar/gku1113
Jain, A., and Tuteja, G. (2019). TissueEnrich: Tissue-specific gene enrichment analysis. Bioinformatics 35, 1966–1977. doi: 10.1093/bioinformatics/bty890
Jamain, S., Girondot, M., Leroy, P., Clergue, M., Quach, H., Fellous, M., et al. (2001). Transduction of the Human Gene FAM8A1 by Endogenous Retrovirus During Primate Evolution. Genomics 78, 38–45. doi: 10.1006/geno.2001.6642
Jin, R., Singh, S. K., Gu, S., Furukawa, H., Sobolevsky, A. I., Zhou, J., et al. (2009). Crystal structure and association behaviour of the GluR2 amino-terminal domain. The EMBO Journal 28, 1812–1823. doi: 10.1038/emboj.2009.140
Johnson, A. L., Edson, K. Z., Totah, R. A., and Rettie, A. E. (2015). Cytochrome P450 ω-Hydroxylases in Inflammation and Cancer. Adv Pharmacol 74, 223–262. doi: 10.1016/bs.apha.2015.05.002
Jones, D. T., Taylor, W. R., and Thornton, J. M. (1994). A Model Recognition Approach to the Prediction of All-Helical Membrane Protein Structure and Topology. Biochemistry 33, 3038–3049. doi: 10.1021/bi00176a037
Kanehisa, M., Furumichi, M., Tanabe, M., Sato, Y., and Morishima, K. (2017). KEGG: new perspectives on genomes, pathways, diseases and drugs. Nucleic Acids Res 45, D353–D361. doi: 10.1093/nar/gkw1092
Kim, H. S., Jung, M., Kang, H. N., Kim, H., Park, C.-W., Kim, S.-M., et al. (2017). Oncogenic BRAF fusions in mucosal melanomas activate the MAPK pathway and are sensitive to MEK/PI3K inhibition or MEK/CDK4/6 inhibition. Oncogene 36, 3334–3345. doi: 10.1038/onc.2016.486
Kumar, R., Kumari, B., and Kumar, M. (2017). Prediction of endoplasmic reticulum resident proteins using fragmented amino acid composition and support vector machine. PeerJ 5, e3561. doi: 10.7717/peerj.3561
Lagerström, M. C., and Schiöth, H. B. (2008). Structural diversity of G protein-coupled receptors and significance for drug discovery. Nat Rev Drug Discov 7, 339–357. doi: 10.1038/nrd2518
Li, Y., Zhao, Y., Hu, J., Xiao, J., Qu, L., Wang, Z., et al. (2013). A novel ER-localized transmembrane protein, EMC6, interacts with RAB5A and regulates cell autophagy. Autophagy 9, 150–163. doi: 10.4161/auto.22742
Lorenzi, I., Oeljeklaus, S., Aich, A., Ronsör, C., Callegari, S., Dudek, J., et al. (2018). The mitochondrial TMEM177 associates with COX20 during COX2 biogenesis. Biochim Biophys Acta 1865, 323–333. doi: 10.1016/j.bbamcr.2017.11.010
Mades, A., Gotthardt, K., Awe, K., Stieler, J., Döring, T., Füser, S., et al. (2012). Role of Human Sec63 in Modulating the Steady-State Levels of Multi-Spanning Membrane Proteins. PLoS One 7:e49243. doi: 10.1371/journal.pone.0049243
Mi, H., Muruganujan, A., Ebert, D., Huang, X., and Thomas, P. D. (2019). PANTHER version 14: more genomes, a new PANTHER GO-slim and improvements in enrichment analysis tools. Nucleic Acids Res 47, D419–D426. doi: 10.1093/nar/gky1038
Nakamura, Y., Asano, A., Hosaka, Y., Takeuchi, T., Iwanaga, T., and Yamano, Y. (2015). Expression and intracellular localization of TBC1D9, a Rab GTPase-accelerating protein, in mouse testes. Exp Anim 64, 415–424. doi: 10.1538/expanim.15-0016
National Center for Biotechnology Information (2018). Available at: https://www.ncbi.nlm.nih.gov/ (accessed February 2, 2018).
Orchard, S., Ammari, M., Aranda, B., Breuza, L., Briganti, L., Broackes-Carter, F., et al. (2014). The MIntAct project—IntAct as a common curation platform for 11 molecular interaction databases. Nucleic Acids Res 42, D358–D363. doi: 10.1093/nar/gkt1115
Ouzzine, M., Magdalou, J., Burchell, B., and Fournel-Gigleux, S. (1999). An Internal Signal Sequence Mediates the Targeting and Retention of the Human UDP-Glucuronosyltransferase 1A6 to the Endoplasmic Reticulum. J. Biol. Chem. 274, 31401–31409. doi: 10.1074/jbc.274.44.31401
Overington, J. P., Al-Lazikani, B., and Hopkins, A. L. (2006). How many drug targets are there? Nature Reviews Drug Discovery 5, 993–996. doi: 10.1038/nrd2199
Pfeffer, S., Dudek, J., Gogala, M., Schorr, S., Linxweiler, J., Lang, S., et al. (2014). Structure of the mammalian oligosaccharyl-transferase complex in the native ER protein translocon. Nature Communications 5, 3072. doi: 10.1038/ncomms4072
Piñero, J., Bravo, À, Queralt-Rosinach, N., Gutiérrez-Sacristán, A., Deu-Pons, J., Centeno, E., et al. (2017). DisGeNET: a comprehensive platform integrating information on human disease-associated genes and variants. Nucleic Acids Res 45, D833–D839. doi: 10.1093/nar/gkw943
Pletscher-Frankild, S., Pallejà, A., Tsafou, K., Binder, J. X., and Jensen, L. J. (2014). DISEASES: Text mining and data integration of disease–gene associations. bioRxiv [Preprint],Google Scholar
Pruitt, K. D., Harrow, J., Harte, R. A., Wallin, C., Diekhans, M., Maglott, D. R., et al. (2009). The consensus coding sequence (CCDS) project: Identifying a common protein-coding gene set for the human and mouse genomes. Genome Res. 19, 1316–1323. doi: 10.1101/gr.080531.108
Putz, U., Howitt, J., Lackovic, J., Foot, N., Kumar, S., Silke, J., et al. (2008). Nedd4 Family-interacting Protein 1 (Ndfip1) Is Required for the Exosomal Secretion of Nedd4 Family Proteins. J. Biol. Chem. 283, 32621–32627. doi: 10.1074/jbc.M804120200
Rask-Andersen, M., Masuram, S., and Schiöth, H. B. (2014). The Druggable Genome: Evaluation of Drug Targets in Clinical Trials Suggests Major Shifts in Molecular Class and Indication. Annual Review of Pharmacology and Toxicology 54, 9–26. doi: 10.1146/annurev-pharmtox-011613-135943
Reddy, V. S., Shlykov, M. A., Castillo, R., Sun, E. I., and Saier, M. H. (2012). The Major Facilitator Superfamily (MFS) Revisited. FEBS J 279, 2022–2035. doi: 10.1111/j.1742-4658.2012.08588.x
Reiner, A., and Levitz, J. (2018). Glutamatergic Signaling in the Central Nervous System: Ionotropic and Metabotropic Receptors in Concert. Neuron 98, 1080–1098. doi: 10.1016/j.neuron.2018.05.018
Ruggiano, A., Foresti, O., and Carvalho, P. (2014). ER-associated degradation: Protein quality control and beyond. J Cell Biol 204, 869–879. doi: 10.1083/jcb.201312042
Sällman Almén, M., Bringeland, N., Fredriksson, R., and Schiöth, H. B. (2012). The Dispanins: A Novel Gene Family of Ancient Origin That Contains 14 Human Members. PLoS ONE 7:e31961. doi: 10.1371/journal.pone.0031961
Sauvageau, E., Rochdi, M. D., Oueslati, M., Hamdan, F. F., Percherancier, Y., Simpson, J. C., et al. (2014). CNIH4 Interacts with Newly Synthesized GPCR and Controls Their Export from the Endoplasmic Reticulum. Traffic 15, 383–400. doi: 10.1111/tra.12148
Schulz, J., Avci, D., Queisser, M. A., Gutschmidt, A., Dreher, L.-S., Fenech, E. J., et al. (2017). Conserved cytoplasmic domains promote Hrd1 ubiquitin ligase complex formation for ER-associated degradation (ERAD). J Cell Sci 130, 3322–3335. doi: 10.1242/jcs.206847
Schweigel-Röntgen, M. (2014). The families of zinc (SLC30 and SLC39) and copper (SLC31) transporters. Curr Top Membr 73, 321–355. doi: 10.1016/B978-0-12-800223-0.00009-8
Schwenk, J., Harmel, N., Zolles, G., Bildl, W., Kulik, A., Heimrich, B., et al. (2009). Functional Proteomics Identify Cornichon Proteins as Auxiliary Subunits of AMPA Receptors. Science 323, 1313–1319. doi: 10.1126/science.1167852
Sobolevsky, A. I., Rosconi, M. P., and Gouaux, E. (2009). X-ray structure, symmetry and mechanism of an AMPA-subtype glutamate receptor. Nature 462, 745–756. doi: 10.1038/nature08624
Szklarczyk, D., Franceschini, A., Wyder, S., Forslund, K., Heller, D., Huerta-Cepas, J., et al. (2015). STRING v10: protein–protein interaction networks, integrated over the tree of life. Nucleic Acids Res 43, D447–D452. doi: 10.1093/nar/gku1003
The Gene Ontology Consortium. (2019). The Gene Ontology Resource: 20 years and still GOing strong. Nucleic Acids Res 47, D330–D338. doi: 10.1093/nar/gky1055
Thomas, J. A., and Rana, F. R. (2007). The Influence of Environmental Conditions, Lipid Composition, and Phase Behavior on the Origin of Cell Membranes. Orig Life Evol Biosph 37, 267–285. doi: 10.1007/s11084-007-9065-6
Thul, P. J., Åkesson, L., Wiking, M., Mahdessian, D., Geladaki, A., Blal, H. A., et al. (2017). A subcellular map of the human proteome. Science 356, eaal3321. doi: 10.1126/science.aal3321
Traynelis, S. F., Wollmuth, L. P., McBain, C. J., Menniti, F. S., Vance, K. M., Ogden, K. K., et al. (2010). Glutamate Receptor Ion Channels: structure, regulation, and function. Pharmacol Rev 62, 405–496. doi: 10.1124/pr.109.002451
Tsirigos, K. D., Peters, C., Shu, N., Käll, L., and Elofsson, A. (2015). The TOPCONS web server for consensus prediction of membrane protein topology and signal peptides. Nucleic Acids Res 43, W401–W407. doi: 10.1093/nar/gkv485
Tusnády, G. E., and Simon, I. (2001). The HMMTOP transmembrane topology prediction server. Bioinformatics 17, 849–850. doi: 10.1093/bioinformatics/17.9.849
Uhlén, M., Fagerberg, L., Hallström, B. M., Lindskog, C., Oksvold, P., Mardinoglu, A., et al. (2015). Tissue-based map of the human proteome. Science 347, 1260419. doi: 10.1126/science.1260419
Van Quickelberghe, E., De Sutter, D., van Loo, G., Eyckerman, S., and Gevaert, K. (2018). A protein-protein interaction map of the TNF-induced NF-κB signal transduction pathway. Scientific Data 5, 180289. doi: 10.1038/sdata.2018.289
Viklund, H., and Elofsson, A. (2004). Best α-helical transmembrane protein topology predictions are achieved using hidden Markov models and evolutionary information. Protein Sci 13, 1908–1917. doi: 10.1110/ps.04625404
Wang, B., and Tontonoz, P. (2019). Phospholipid Remodeling in Physiology and Disease. Annual Review of Physiology 81, 165–188. doi: 10.1146/annurev-physiol-020518-114444
Wilson, J. D., and Barlowe, C. (2010). Yet1p and Yet3p, the Yeast Homologs of BAP29 and BAP31, Interact with the Endoplasmic Reticulum Translocation Apparatus and Are Required for Inositol Prototrophy. J Biol Chem 285, 18252–18261. doi: 10.1074/jbc.M109.080382
Wishart, D. S., Feunang, Y. D., Guo, A. C., Lo, E. J., Marcu, A., Grant, J. R., et al. (2017). DrugBank 5.0: a major update to the DrugBank database for 2018. Nucleic Acids Res. 46, D1074–D1082. doi: 10.1093/nar/gkx1037
Yamamoto, Y., and Sakisaka, T. (2012). Molecular Machinery for Insertion of Tail-Anchored Membrane Proteins into the Endoplasmic Reticulum Membrane in Mammalian Cells. Molecular Cell 48, 387–397. doi: 10.1016/j.molcel.2012.08.028
Yamashita, A., Hayashi, Y., Nemoto-Sasaki, Y., Ito, M., Oka, S., Tanikawa, T., et al. (2014). Acyltransferases and transacylases that determine the fatty acid composition of glycerolipids and the metabolism of bioactive lipid mediators in mammalian cells and model organisms. Progress in Lipid Research 53, 18–81. doi: 10.1016/j.plipres.2013.10.001
Yankovskaya, V., Horsefield, R., Törnroth, S., Luna-Chavez, C., Miyoshi, H., Léger, C., et al. (2003). Architecture of Succinate Dehydrogenase and Reactive Oxygen Species Generation. Science 299, 700–704. doi: 10.1126/science.1079605
Keywords: ionotropic glutamate receptor, membrane biosynthesis, cerebral cortex, fatty acid transport, lipid metabolic process, transmembrane proteins, trispanins
Citation: Attwood MM and Schiƶth HB (2020) Classification of Trispanins: A Diverse Group of Proteins That Function in Membrane Synthesis and Transport Mechanisms. Front. Cell Dev. Biol. 7:386. doi: 10.3389/fcell.2019.00386
Received: 28 October 2019; Accepted: 19 December 2019;
Published: 22 January 2020.
Edited by:
Heike Folsch, Northwestern University, United StatesReviewed by:
Christopher Stroupe, The University of Virginia, United StatesNobuhiro Nakamura, Kyoto Sangyo University, Japan
Copyright © 2020 Attwood and Schiöth. This is an open-access article distributed under the terms of the Creative Commons Attribution License (CC BY). The use, distribution or reproduction in other forums is permitted, provided the original author(s) and the copyright owner(s) are credited and that the original publication in this journal is cited, in accordance with accepted academic practice. No use, distribution or reproduction is permitted which does not comply with these terms.
*Correspondence: Misty M. Attwood, bWlzdHkuYXR0d29vZEBuZXVyby51dS5zZQ==; Helgi B. Schiöth, aGVsZ2lzQGJtYy51dS5zZQ==