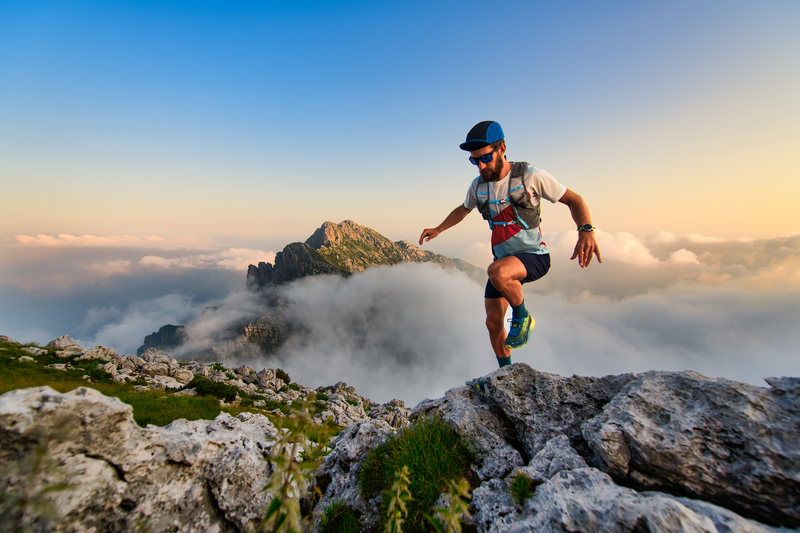
94% of researchers rate our articles as excellent or good
Learn more about the work of our research integrity team to safeguard the quality of each article we publish.
Find out more
SPECIALTY GRAND CHALLENGE article
Front. Catal. , 15 April 2021
Sec. Modelling, Theory and Computational Catalysis
Volume 1 - 2021 | https://doi.org/10.3389/fctls.2021.658965
Catalysis is a cornerstone of modern societies as over 90% of processes in the chemical industry are facilitated by catalysts, with the majority requiring a homogeneous or heterogeneous catalyst (Hagen, 2015). Future renewable energy scenarios also rely heavily on the utilization of electro-catalysts, e.g., for the production of clean hydrogen. This shift towards new feedstocks and benign processes entails the development of new generations of catalysts. While the discovery of catalysts has often relied on trial and error in the first half of the last century, the establishment of (design) rules has significantly improved the speed with which new catalysts are being discovered.
To this end, the knowledge-based improvement and design of new catalysts is increasingly supported by quantum chemical calculations of reaction mechanisms and kinetic modeling of corresponding reaction rates. In fact, first examples of catalyst design by means of computational screening have already emerged (Nørskov et al., 2009; Medford et al., 2015; Zhao et al., 2019). The extent to which computational modeling becomes a dominant factor in the catalysis community in the 21st century depends crucially on the accuracy with which predictions can be made, but also on the development of a reductionist approach, where the main contributing factors to the performance of a given class of catalysts are reduced to a few selected key parameters that can be used efficiently for computational screening.
Perhaps the most challenging issue in computational catalysis is the fact that the rate constant of a reaction step changes drastically with minor changes of the reaction barrier (e.g., for a reaction occurring at 500 K by a factor of about 120 for typical errors of ±20 kJ/mol, or a factor of approximately 3 for an error ±5 kJ/mol, that is commonly referred to as chemical accuracy) and that only approximate methods are computationally feasible for the calculation of enthalpy and entropy contributions of a transition states free energy as the catalytic systems are often large and complex.
In all fields discussed here (homogeneous, heterogeneous and electro-catalysis) density functional theory (DFT) has become the workhorse of computational studies as it exhibits the best compromise between accuracy and computational cost. In homogeneous catalysis for example, the enthalpy related to the active site of a transition-metal complex can be determined quite accurately with advanced hybrid functionals (Jiang et al., 2012). However, homogeneous catalysts often exhibit large ligands raising the issue of conformational complexity that is difficult to model. This is often getting even more problematic with solvation and leads to difficulties in determining the active conformational space and corresponding enthalpy and particularly entropy contributions to the free energy (Harvey et al., 2019). A balanced description of inter- and intramolecular interactions during solvation is similarly challenging (Schmidt et al., 2013).
In large parts of heterogeneous catalysis (e.g., supported transition metals) one needs to approximate the active site with a simple extended periodic surface model, that usually omits the effect of particle size and shape as well as particle-support interactions. Moreover, functionals using the generalized gradient approximation (GGA) are typically the only applicable choice, with the best functionals having errors of ±20 kJ/mol for adsorption energies (Wellendorff et al., 2015) and reaction barriers (Sharada et al., 2017). While this approach has been shown to be sufficient to describe trends observed in heterogeneous catalysis (Honkala et al., 2005; Medford et al., 2015), there is an ongoing challenge to include support effects and to accurately model the interface of a (nanometer sized) particle with the support (Zhao et al., 2017; Whittaker et al., 2018). All this means that approximate active site motifs need to be invoked and calculations of reaction barriers are time-consuming even at the GGA level of theory.
Arguably the most difficult field for computational studies is electro-catalysis. Not only does one have to deal with the complexity of a homogeneous or heterogeneous catalytic system, there is also the additional intricacy of the reaction being divided into electron and proton transfer steps. In heterogeneous electro-catalysis, for example, the reaction is taking place at the charged solid-liquid interface that is extremely difficult to model. Furthermore, the kinetics become a function of the applied electrochemical potential. Moreover, the calculation of reaction barriers starts with a separated proton/electron pair that leads to unnatural changes of the surface dipole and electric fields along the coordinate of the transition state such that reaction barriers need to be extrapolated. It is therefore not surprising that modeling efforts in heterogeneous electro-catalysis did first emerge after introducing the concept of the computational hydrogen electrode (CHE) model (Nørskov et al., 2004) that elegantly circumvents the necessity of treating reaction barriers explicitly. Many challenges remain, however, such as fast accurate calculations of reaction barriers, a detailed view on the diffusion through the Helmholtz layer and corresponding explicit modeling of the solvent and solid-liquid interface, to name a few (Gauthier et al., 2019; Chan, 2020; Li et al., 2020).
While calculations of accurate enthalpies are thus subject to many obstacles (from the quantum chemical method to the approximation of the active site), this might even be more problematic for the entropy associated with a catalytic reaction step. Commonly, the entropy contribution is derived from the vibrational part of the partition function. In heterogeneous catalysis, for example, both, the adsorbates and transition states are rather confined and due to its simplicity this is typically calculated using the harmonic oscillator approximation. More accurate methods are emerging (Kundu et al., 2016; Sprowl et al., 2016; Jørgensen and Grönbeck, 2017; Li et al., 2018; Amsler et al., 2021) but are often computationally demanding and imply a trade-off with respect to the accuracy of the corresponding enthalpy (e.g., molecular dynamics (MD) simulations yield more reliable entropies, but are typically only feasible at the GGA level of DFT). This is where developments of faster algorithms and methodologies such as machine learning (Bučko et al., 2020) are expected to move the frontiers of what is possible nowadays.
Importantly, accurate free energy data for adsorption energies and transition states is only one part of the equation. How the calculated reaction mechanisms translate into simulated conversion and hence reaction rates and selectivities depends crucially on the (micro)-kinetic model invoked to describe the reaction and whether this model is able to capture the important features of the real technical catalytic system. There is a broad range of approaches to deal with kinetics ranging from the simple mean-field, steady-state approximation to simulations using reactor models that include heat and mass transfer (Schlögl, 2017). Linking these various time and length scales with accurate free energy calculations in a multi-scale approach is still presenting a major challenge (Bruix et al., 2019; Plessow et al., 2019). There is thus ample room to improve the theoretical description of the kinetic model underlying a catalytic process and the 21st century will likely see that the improvement of kinetic models goes along with more accurate (free energy) data, keeping in mind the problem of still fairly large errors of rate constants (see above) even for extremely well described reaction steps.
On top of all of this, the vast majority of modeling efforts is focused on static active site motifs of a catalytic system. Catalysts, however, are often rather complex arrangements of atoms and can possess fairly dynamic behavior (Hansen et al., 2002; Kalz et al., 2017; Paolucci et al., 2017; Tang et al., 2019; Maurer et al., 2020), e.g., by switching between metallic and oxidic states, as evidenced for supported transition metal heterogeneous catalysts in oxidation reactions (Hellman et al., 2012). There may thus be several active sites with different reaction steps and rate constants, the ratio of which changes as a function of time, present in one catalytic system that all need to be modeled to mimic the experimentally observed properties of catalysts.
The extend to which computational catalysis will be successful in advancing the atomic-scale understanding of catalytic processes will also heavily rely on the collaboration with experimentalists, as this will help to obtain a better understanding of the exact nature of the active site and the dependence of reaction rates on factors such as temperature, pressure and applied potential. Likewise, computational studies can guide experimentalists to interpret their spectroscopic data, narrowing the search for active sites and helping to understand their dynamics.
While more realistic simulations will only be possible by including a greater complexity of the active sites as well as more sophisticated kinetic modeling, deriving a detailed mechanistic understanding of working catalysts is only one part of future research directions. The other grand challenge lies in the development of a knowledge-based approach enabling predictive modeling and thus fast computational screening for new catalyst leads. This means that one needs to reduce the complexity used to understand the functioning of a catalytic system in excruciating detail, to a few selected key parameters that grasp the entirety of what determines the properties of a catalyst such that changes from one catalyst to another are well described by them. This complexity reduction is the core of catalyst design strategies.
There are ample examples in homogeneous, heterogeneous and electro-catalysis where this has been achieved through so-called scaling relations that are able to predict reaction energies and their corresponding reaction barriers using simple descriptors (Nørskov et al., 2002; Michaelides et al., 2003; Bligaard et al., 2004; Abild-Pedersen et al., 2007; Greeley et al., 2009; Man et al., 2011; Busch et al., 2015; Medford et al., 2015; Wodrich et al., 2016; Zagal and Koper, 2016; Latimer et al., 2017; Pegis et al., 2017; Craig et al., 2019; Kropp and Mavrikakis, 2019; Anand et al., 2020; Yang and Hong, 2020). In conjunction with microkinetic models, volcano relationships can be derived, allowing for the fast computational screening of a large number of catalysts. Importantly, the same limitations in terms of accuracy of the calculated rate constant and complexity of the kinetic model and thus activity of a catalyst (see above) apply when searching for new catalyst leads—i.e., how can one discover new catalytic materials if the predictability of DFT has an uncertainty of two orders of magnitude with respect to calculated rate constants? Fortunately, DFT seems to be much better when describing trends from one catalyst to another, compared to its precision for single reaction steps, e.g., recent work has shown that errors for predictions of differences of the same reaction barriers between different catalysts are only ±5 kJ/mol for functionals that have intrinsic errors of 50 kJ/mol (Plessow and Studt, 2020). While catalyst screening needs to include measurements of the stability of potential catalysts, future studies might also include their feasibility in industrial applications (e.g., manufacturing costs, recyclability, associated environmental issues).
This is where new methodologies such as machine learning have the potential to play an important role in the future (Meyer et al., 2018), e.g., in correlating and identifying key parameters of interest. The main problem to date, however, is that these approaches will only work sufficiently, if large, well-defined amounts of theoretical (and/or experimental) data are available.
The future will show how new techniques shape computational catalysis from the acceleration of quantum chemical calculations and the possibility to include the entire complexity of the catalytic system to new ways of searching for correlations among classes of catalytic systems that translate to the knowledge-based design of catalytic materials for the chemical and energy industry of the future.
The author confirms being the sole contributor of this work and has approved it for publication.
The author declares that the research was conducted in the absence of any commercial or financial relationships that could be construed as a potential conflict of interest.
The author gratefully acknowledges support from the Helmholtz Association.
Abild-Pedersen, F., Greeley, J., Studt, F., Rossmeisl, J., Munter, T. R., Moses, P. G., et al. (2007). Scaling properties of adsorption energies for hydrogen-containing molecules on transition-metal surfaces. Phys. Rev. Lett. 99, 016105. doi:10.1103/physrevlett.99.016105
Amsler, J., Plessow, P. N., Studt, F., and Bučko, T. (2021). Anharmonic correction to adsorption free energy from DFT-based MD using thermodynamic integration. J. Chem. Theory Comput. 17, 1155–1169. doi:10.1021/acs.jctc.0c01022
Anand, M., Rohr, B., Statt, M. J., and Nørskov, J. K. (2020). Scaling relationships and volcano plots in homogeneous catalysis. J. Phys. Chem. Lett. 11, 8518–8526. doi:10.1021/acs.jpclett.0c01991
Bligaard, T., Nørskov, J. K., Dahl, S., Matthiesen, J., Christensen, C. H., and Sehested, J. (2004). The Brønsted-Evans-Polanyi relation and the volcano curve in heterogeneous catalysis. J. Catal. 224, 206–217. doi:10.1016/j.jcat.2004.02.034
Bruix, A., Margraf, J. T., Andersen, M., and Reuter, K. (2019). First-principles-based multiscale modelling of heterogeneous catalysis. Nat. Catal. 2, 659–670. doi:10.1038/s41929-019-0298-3
Bučko, T., Gešvandtnerová, M., and Rocca, D. (2020). Ab initio calculations of free energy of activation at multiple electronic structure levels made affordable: an effective combination of perturbation theory and machine learning. J. Chem. Theory Comput. 16, 6049–6060. doi:10.1021/acs.jctc.0c00486
Busch, M., Wodrich, M. D., and Corminboeuf, C. (2015). Linear scaling relationships and volcano plots in homogeneous catalysis - revisiting the Suzuki reaction. Chem. Sci. 6, 6754–6761. doi:10.1039/c5sc02910d
Chan, K. (2020). A few basic concepts in electrochemical carbon dioxide reduction. Nat. Commun. 11, 5954. doi:10.1038/s41467-020-19369-6
Craig, M. J., Coulter, G., Dolan, E., Soriano-Loṕez, J., Mates-Torres, E., Schmitt, W., et al. (2019). Universal scaling relations for the rational design of molecular water oxidation catalysts with near-zero overpotential. Nat. Commun. 10, 4993. doi:10.1038/s41467-019-12994-w
Gauthier, J. A., Dickens, C. F., Heenen, H. H., Vijay, S., Ringe, S., and Chan, K. (2019). Unified approach to implicit and explicit solvent simulations of electrochemical reaction energetics. J. Chem. Theory Comput. 15, 6895–6906. doi:10.1021/acs.jctc.9b00717
Greeley, J., Stephens, I. E. L., Bondarenko, A. S., Johansson, T. P., Hansen, H. A., Jaramillo, T. F., et al. (2009). Alloys of platinum and early transition metals as oxygen reduction electrocatalysts. Nat. Chem. 1, 552–556. doi:10.1038/nchem.367
Hagen, J. (2015). Industrial catalysis: a practical approach. Weinheim, Germany: Wiley VCH, 459–462.
Hansen, P. L., Wagner, J. B., Helveg, S., Rostrup-Nielsen, J. R., Clausen, B. S., and Topsøe, H. (2002). Atom-resolved imaging of dynamic shape changes in supported copper nanocrystals. Science 295, 2053–2055. doi:10.1126/science.1069325
Harvey, J. N., Himo, F., Maseras, F., and Perrin, L. (2019). Scope and challenge of computational methods for studying mechanism and reactivity in homogeneous catalysis. ACS Catal. 9, 6803–6813. doi:10.1021/acscatal.9b01537
Hellman, A., Resta, A., Martin, N. M., Gustafson, J., Trinchero, A., Carlsson, P.-A., et al. (2012). The active phase of palladium during methane oxidation. J. Phys. Chem. Lett. 3, 678–682. doi:10.1021/jz300069s
Honkala, K., Hellman, A., Remediakis, I. N., Logadottir, A., Carlsson, A., Dahl, S., et al. (2005). Ammonia synthesis from first-principles calculations. Science 307, 555–558. doi:10.1126/science.1106435
Jiang, W., Laury, M. L., Powell, M., and Wilson, A. K. (2012). Comparative study of single and double hybrid density functionals for the prediction of 3d transition metal thermochemistry. J. Chem. Theory Comput. 8, 4102–4111. doi:10.1021/ct300455e
Jørgensen, M., and Grönbeck, H. (2017). Adsorbate entropies with complete potential energy sampling in microkinetic modeling. J. Phys. Chem. C 121, 7199–7207. doi:10.1021/acs.jpcc.6b11487
Kalz, K. F., Kraehnert, R., Dvoyashkin, M., Dittmeyer, R., Gläser, R., Krewer, U., et al. (2017). Future challenges in heterogeneous catalysis: understanding catalysts under dynamic reaction conditions. ChemCatChem 9, 17–29. doi:10.1002/cctc.201600996
Kropp, T., and Mavrikakis, M. (2019). Brønsted-Evans-Polanyi relation for CO oxidation on metal oxides following the Mars-van Krevelen mechanism. J. Catal. 377, 577–581. doi:10.1016/j.jcat.2019.08.002
Kundu, A., Piccini, G., Sillar, K., and Sauer, J. (2016). Ab initio prediction of adsorption isotherms for small molecules in metal-organic frameworks. J. Am. Chem. Soc. 138, 14047–14056. doi:10.1021/jacs.6b08646
Latimer, A. A., Kulkarni, A. R., Aljama, H., Montoya, J. H., Yoo, J. S., Tsai, C., et al. (2017). Understanding trends in C-H bond activation in heterogeneous catalysis. Nat. Mater. 16, 225–229. doi:10.1038/nmat4760
Li, H., Paolucci, C., and Schneider, W. F. (2018). Zeolite adsorption free energies from ab initio potentials of mean force. J. Chem. Theory Comput. 14, 929–938. doi:10.1021/acs.jctc.7b00716
Li, Q., Ouyang, Y., Lu, S., Bai, X., Zhang, Y., Shi, L., et al. (2020). Perspective on theoretical methods and modeling relating to electro-catalysis processes. Chem. Commun. 56, 9937–9949. doi:10.1039/d0cc02998j
Man, I. C., Su, H. Y., Calle‐Vallejo, F., Hansen, H. A., Martínez, J. I., Inoglu, N. G., et al. (2011). Universality in oxygen evolution electrocatalysis on oxide surfaces. ChemCatChem 3, 1159–1165. doi:10.1002/cctc.201000397
Maurer, F., Jelic, J., Wang, J., Gänzler, A., Dolcet, P., Wöll, C., et al. (2020). Tracking the formation, fate and consequence for catalytic activity of Pt single sites on CeO2. Nat. Catal. 3, 824–833. doi:10.1038/s41929-020-00508-7
Medford, A. J., Vojvodic, A., Hummelshøj, J. S., Voss, J., Abild-Pedersen, F., Studt, F., et al. (2015). From the Sabatier principle to a predictive theory of transition-metal heterogeneous catalysis. J. Catal. 328, 36–42. doi:10.1016/j.jcat.2014.12.033
Meyer, B., Sawatlon, B., Heinen, S., Von Lilienfeld, O. A., and Corminboeuf, C. (2018). Machine learning meets volcano plots: computational discovery of cross-coupling catalysts. Chem. Sci. 9, 7069–7077. doi:10.1039/c8sc01949e
Michaelides, A., Liu, Z.-P., Zhang, C. J., Alavi, A., King, D. A., and Hu, P. (2003). Identification of general linear relationships between activation energies and enthalpy changes for dissociation reactions at surfaces. J. Am. Chem. Soc. 125, 3704–3705. doi:10.1021/ja027366r
Nørskov, J. K., Bligaard, T., Logadottir, A., Bahn, S., Hansen, L. B., Bollinger, M., et al. (2002). Universality in heterogeneous catalysis. J. Catal. 209, 275–278. doi:10.1006/jcat.2002.3615
Nørskov, J. K., Bligaard, T., Rossmeisl, J., and Christensen, C. H. (2009). Towards the computational design of solid catalysts. Nat. Chem. 1, 37–46. doi:10.1038/nchem.121
Nørskov, J. K., Rossmeisl, J., Logadottir, A., Lindqvist, L. R. K. J., Kitchin, J. R., Bligaard, T., et al. (2004). Origin of the overpotential for oxygen reduction at a fuel-cell cathode. J. Phys. Chem. B 108, 17886–17892. doi:10.1021/jp047349j
Pegis, M. L., Wise, C. F., Koronkiewicz, B., and Mayer, J. M. (2017). Identifying and breaking scaling relations in molecular catalysis of electrochemical reactions. J. Am. Chem. Soc. 139, 11000–11003. doi:10.1021/jacs.7b05642
Paolucci, C., Khurana, I., Parekh, A. A., Li, S., Shih, A. J., Li, H., et al. (2017). Dynamic multinuclear sites formed by mobilized copper ions in NOx selective catalytic reduction. Science 357, 898–903. doi:10.1126/science.aan5630
Plessow, P. N., Smith, A., Tischer, S., and Studt, F. (2019). Identification of the reaction sequence of the MTO initiation mechanism using ab initio-based kinetics. J. Am. Chem. Soc. 141, 5908–5915. doi:10.1021/jacs.9b00585
Plessow, P. N., and Studt, F. (2020). How accurately do approximate density functionals predict trends in acidic zeolite catalysis?. J. Phys. Chem. Lett. 11, 4305–4310. doi:10.1021/acs.jpclett.0c01240
Schmidt, S., Abkai, G., Rosendahl, T., Rominger, F., and Hofmann, P. (2013). Inter- and intramolecular interactions in triptycene-derived bisphosphite hydroformylation catalysts: structures, energies, and caveats for DFT-assisted ligand design. Organometallics 32, 1044–1052. doi:10.1021/om301027x
Sharada, S. M., Bligaard, T., Luntz, A. C., Kroes, G.-J., and Nørskov, J. K. (2017). SBH10: a benchmark database of barrier heights on transition metal surfaces. J. Phys. Chem. C 121, 19807–19815. doi:10.1021/acs.jpcc.7b05677
Sprowl, L. H., Campbell, C. T., and Árnadóttir, L. (2016). Hindered translator and hindered rotor models for adsorbates: partition functions and entropies. J. Phys. Chem. C 120, 9719–9731. doi:10.1021/acs.jpcc.5b11616
Tang, Y., Asokan, C., Xu, M., Graham, G. W., Pan, X., Christopher, P., et al. (2019). Rh single atoms on TiO2 dynamically respond to reaction conditions by adapting their site. Nat. Commun. 10, 4488. doi:10.1038/s41467-019-12461-6
Wellendorff, J., Silbaugh, T. L., Garcia-Pintos, D., Nørskov, J. K., Bligaard, T., Studt, F., et al. (2015). A benchmark database for adsorption bond energies to transition metal surfaces and comparison to selected DFT functionals. Surf. Sci. 640, 36–44. doi:10.1016/j.susc.2015.03.023
Whittaker, T., Kumar, K. B. S., Peterson, C., Pollock, M. N., Grabow, L. C., and Chandler, B. D. (2018). H2 oxidation over supported Au nanoparticle catalysts: evidence for heterolytic H2 activation at the metal-support interface. J. Am. Chem. Soc. 140, 16469–16487. doi:10.1021/jacs.8b04991
Wodrich, M. D., Busch, M., and Corminboeuf, C. (2016). Accessing and predicting the kinetic profiles of homogeneous catalysts from volcano plots. Chem. Sci. 7, 5723–5735. doi:10.1039/c6sc01660j
Yang, L.-C., and Hong, X. (2020). Scaling relationships and volcano plots of homogeneous transition metal catalysis. Dalton Trans. 49, 3652–3657. doi:10.1039/d0dt00187b
Zagal, J. H., and Koper, M. T. M. (2016). Reactivity descriptors for the activity of molecular MN4 catalysts for the oxygen reduction reaction. Angew. Chem. Int. Ed. 55, 14510–14521. doi:10.1002/anie.201604311
Zhao, Z.-J., Li, Z., Cui, Y., Zhu, H., Schneider, W. F., Delgass, W. N., et al. (2017). Importance of metal-oxide interfaces in heterogeneous catalysis: a combined DFT, microkinetic, and experimental study of water-gas shift on Au/MgO. J. Catal. 345, 157–169. doi:10.1016/j.jcat.2016.11.008
Keywords: computational catalysis, theory, DFT, kinetics, catalyst design
Citation: Studt F (2021) Grand Challenges in Computational Catalysis. Front. Catal. 1:658965. doi: 10.3389/fctls.2021.658965
Received: 26 January 2021; Accepted: 12 February 2021;
Published: 15 April 2021.
Edited and reviewed by:
Frank Hollmann, Delft University of Technology, NetherlandsCopyright © 2021 Studt. This is an open-access article distributed under the terms of the Creative Commons Attribution License (CC BY). The use, distribution or reproduction in other forums is permitted, provided the original author(s) and the copyright owner(s) are credited and that the original publication in this journal is cited, in accordance with accepted academic practice. No use, distribution or reproduction is permitted which does not comply with these terms.
*Correspondence: Felix Studt, ZmVsaXguc3R1ZHRAa2l0LmVkdQ==
Disclaimer: All claims expressed in this article are solely those of the authors and do not necessarily represent those of their affiliated organizations, or those of the publisher, the editors and the reviewers. Any product that may be evaluated in this article or claim that may be made by its manufacturer is not guaranteed or endorsed by the publisher.
Research integrity at Frontiers
Learn more about the work of our research integrity team to safeguard the quality of each article we publish.