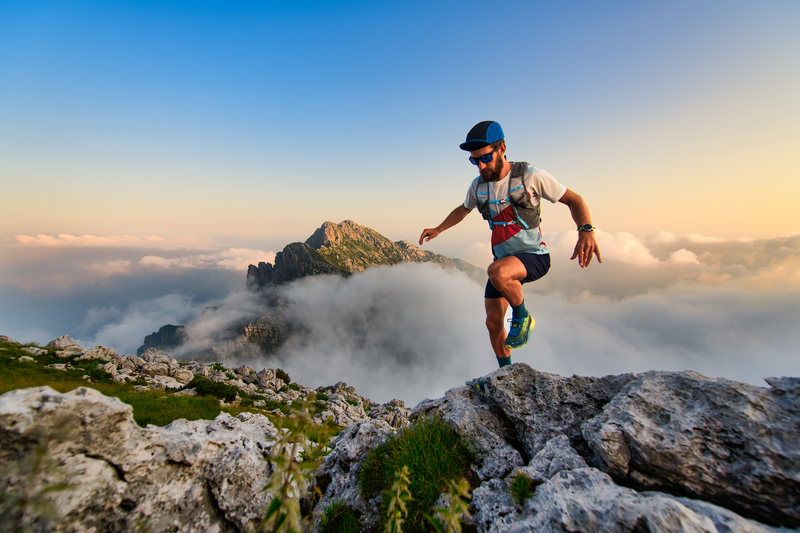
94% of researchers rate our articles as excellent or good
Learn more about the work of our research integrity team to safeguard the quality of each article we publish.
Find out more
ORIGINAL RESEARCH article
Front. Cardiovasc. Med.
Sec. Intensive Care Cardiovascular Medicine
Volume 12 - 2025 | doi: 10.3389/fcvm.2025.1573874
This article is part of the Research Topic Innovative Monitoring and Management of Perioperative Complications in Cardiac Surgery View all articles
The final, formatted version of the article will be published soon.
You have multiple emails registered with Frontiers:
Please enter your email address:
If you already have an account, please login
You don't have a Frontiers account ? You can register here
Objective: Prolonged mechanical ventilation (PMV) is a significant postoperative complication in cardiac surgery, associated with increased mortality and healthcare costs. This study aims to develop and validate a novel scoring model to predict the risk of PMV in cardiac surgery patients.Methods: A retrospective analysis was conducted using data from 14 comprehensive hospitals in Jiangsu Province, including adult patients who underwent coronary artery bypass grafting (CABG), valve surgery, and aortic surgery from January 2021 to December 2022. Predictive variables were selected based on clinical expertise and prior literature, and a nomogram was developed using LASSO regression and multiple logistic regression. Model performance was evaluated using the C-index, calibration plots, and decision curve analysis (DCA).: A total of 5,206 patients were included in the final analysis. The incidence rate of PMV were 11.83% in the training set, 8.65% in the internal validation set, and 15.4% in the external validation set. The nomogram identified 9 significant predictors, including age, gender, preoperative conditions, and surgical factors. The model demonstrated robust performance with C-index values of 0.79 in the training and internal validation sets and 0.75 in the external validation set, indicating good predictive capability. Calibration curves confirmed the accuracy of predicted probabilities, and DCA indicated substantial net benefits for clinical decision-making. Conclusions: This study presents a validated scoring model for predicting PMV in cardiac surgery patients, integrating a comprehensive range of clinical variables. The model facilitates early identification of high-risk patients, enabling tailored perioperative strategies and potentially improving patient outcomes and resource utilization in cardiac surgery.
Keywords: Prolonged mechanical ventilation, cardiac surgery, Predicting model, Multiple centers, Retrospective study
Received: 10 Feb 2025; Accepted: 11 Mar 2025.
Copyright: © 2025 Liu, Chen, Wang, zhou, Xu, Cao, Fan, Chen, Huang and Chen. This is an open-access article distributed under the terms of the Creative Commons Attribution License (CC BY). The use, distribution or reproduction in other forums is permitted, provided the original author(s) or licensor are credited and that the original publication in this journal is cited, in accordance with accepted academic practice. No use, distribution or reproduction is permitted which does not comply with these terms.
* Correspondence:
Fuhua Huang, Department of Thoracic and Cardiovascular Surgery, Nanjing First Hospital, Nanjing Medical University,, Nanjing, China
Xin Chen, Department of Thoracic and Cardiovascular Surgery, Nanjing First Hospital, Nanjing Medical University,, Nanjing, China
Disclaimer: All claims expressed in this article are solely those of the authors and do not necessarily represent those of their affiliated organizations, or those of the publisher, the editors and the reviewers. Any product that may be evaluated in this article or claim that may be made by its manufacturer is not guaranteed or endorsed by the publisher.
Research integrity at Frontiers
Learn more about the work of our research integrity team to safeguard the quality of each article we publish.