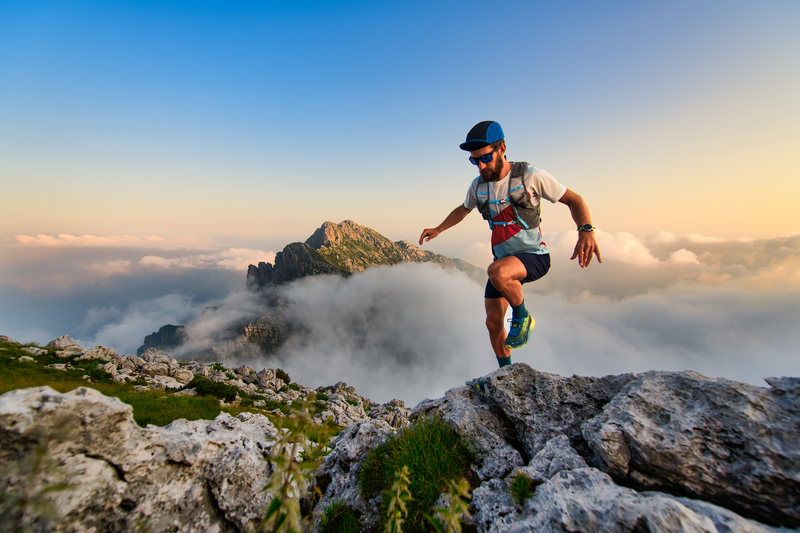
95% of researchers rate our articles as excellent or good
Learn more about the work of our research integrity team to safeguard the quality of each article we publish.
Find out more
ORIGINAL RESEARCH article
Front. Cardiovasc. Med. , 26 March 2025
Sec. General Cardiovascular Medicine
Volume 12 - 2025 | https://doi.org/10.3389/fcvm.2025.1569904
Background: Serum uric acid (SUA) and lipid metabolism disorders are closely associated with atrial fibrillation (AF) and its prognosis. In patients with non-valvular AF (NAF), we evaluated the combined predictive value of SUA, triglycerides (TG), and low-density lipoprotein (LDL) for stroke to enhance stroke risk prediction and management.
Methods and results: We included 3,176 NAF patients treated at the First Affiliated Hospital of Dalian Medical University from January 2020 to December 2023. We analyzed SUA concentration and lipid profile, along with relevant clinical data, to assess their impact on the occurrence of ischemic stroke (IS) in NAF patients. Due to gender differences in TG (1.39 mmol/L vs. 1.28 mmol/L for males, P = 0.031;1.57 mmol/L vs. 1.28 mmol/L for females, P = 0.001) and SUA levels (424 µmol/L vs. 397 µmol/L for males, P = 0.008; 361 µmol/L vs. 328 µmol/L for females, P = 0.004), we determined the thresholds for SUA (400 µmol/L in males and 330 µmol/L in females) and TG (1.28 mmol/L in males and 1.29 mmol/L in females) that predict stroke events in NAF patients by restricted cubic spline curves. Kaplan–Meier cumulative risk analysis indicates that a gender-based combined assessment of SUA and TG enhances stroke risk stratification in NAF patients. Compared to patients with low levels of SUA and TG, those with high levels of these biomarkers have a higher risk of IS (HR = 1.98). On multivariable Cox regression analysis with potential confounders, elevated SUA and low-density lipoprotein (LDL) levels were significantly associated with an increased risk of stroke. In summary, we developed the CHA2DS2-VASc+SUA+TG+LDL stroke risk prediction model. Its clinical predictive value was assessed using Harrell's C-statistic (C-index), integrated discrimination improvement (IDI) statistics, and net reclassification index (NRI) analysis.
Conclusions: SUA, TG and LDL were strongly associated with stroke for NAF. The combination of SUA, TG, and LDL effectively enhanced the predictive value of the CHA2DS2-VASc score for IS.
Atrial fibrillation (AF) stands out as the most prevalent cardiac arrhythmia, affecting approximately 1.6% of Chinese adults, a figure that rises sharply with age (1, 2). Numerous epidemiological studies have flagged AF as a major contributor to ischemic stroke (IS) and overall cardiovascular mortality (3–5). This chaotic heart rhythm disrupts effective atrial contractions, leading to stagnation of blood flow and significantly raising the risk of thrombus formation in the left atrium. As a result, individuals with AF face a staggering fivefold increase in their risk of experiencing an IS compared to those without the condition (3, 6). Moreover, AF can worsen other health issues, including metabolic disorders (7, 8), hypertension, and diabetes, further elevating the threat of IS.
While uric acid is often associated with gout, its role extends far beyond that. Increasingly recognized as a biomarker for systemic inflammation and oxidative stress—two critical factors in the development of AF—uric acid has gained momentum for its implications in cardiovascular health. Studies reveal that hyperuricemia affects 20.7% of men and 5.6% of women, with these figures climbing annually (9). Recent research even highlights a potential connection between elevated serum uric acid (SUA) levels and subclinical AF (SCAF) (10). Furthermore, hyperuricemia has been reported to contribute to the recurrence of AF after catheter ablation (11). Suggesting it plays a key role in the persistence and re-emergence of this condition.
The Framingham study has shown that prolonged exposure to lipid imbalances can lead to atherosclerotic cardiovascular disease and increased mortality (12). Research from Luanluan Sun et al. indicates that higher plasma levels of the low density of lipoprotein (LDL) and triglycerides (TG) are directly linked to an elevated risk of IS, while showing a reduced risk of intracerebral hemorrhage (13, 14). Dyslipidemia also significantly impacts dementia in patients with AF (15). The link between AF and metabolic syndrome involves complex mechanisms, including activation of the sympathetic nervous system and impaired mitochondrial calcium management (16).
Dyslipidemia and hyperuricemia are common metabolic disorders that often go unnoticed for extended periods, leading to a lack of targeted clinical care and risk assessment guidelines (7, 8, 17, 18). While the CHA2DS2-VASc score is a well-established tool for evaluating stroke risk in NAF patients, it doesn't capture all individuals at high risk for thromboembolic events (19). To improve detection and enhance clinical decision-making, we have developed a new model: CHA2DS2-VASc+SUA+TG+LDL. This innovative approach aims to more effectively identify high-risk stroke populations among NAF patients, paving the way for better-targeted management and prevention strategies.
The study was conducted on patients diagnosed with NAF between January 1, 2020, and December 31, 2023. It aimed to assess the risk factors for stroke in patients with NAF. Briefly, this is an ancillary study designed to explore the impact of SUA, TG, and LDL on the prognosis of AF patients. Our study protocol was registered with the Chinese Clinical Trial Registry on September 21, 2020 (https://www.chictr.org.cn, registration number: Chi- CTR2000038377).
This study initially recruited 4,205 patients aged between 18 and 80 years with NAF. Patients with hyperthyroidism or hypothyroidism, serum creatinine (Scr) levels ≥443 µmol/L, and chronic kidney disease stages 4 or 5 (CKD IV/V) were excluded from the study. Also, patients with tumors and those missing key clinical covariates were excluded from the study. Ultimately, the final analysis included a total of 3,176 patients. Figure 1 provides an overview of the participant selection process. The research was conducted in accordance with the Declaration of Helsinki guidelines and received approval from the institutional review board of the First Affiliated Hospital of Dalian Medical University (FAHDMU). Informed consent was obtained from all participants, and all procedures described were performed in compliance with the approved guidelines.
Figure 1. Flowchart of the included patients and follow-up. AF, atrial fibrillation; Scr, serum creatinine; CKD, chronic kidney diseases.
Demographic and clinical characteristics, such as age, gender, and major risk factors for AF—including serum lipid levels, diabetes mellitus (DM), arterial hypertension, alcohol consumption, smoking, and other cardiovascular comorbidities—were obtained from electronic health records. All measurements, including serum concentrations of SUA, TG, total cholesterol (TC), and LDL cholesterol, were conducted at the FAHDMU laboratory following standard protocols.
Follow-up was performed via database search and telephone call. The ischemic cerebrovascular events were recorded. IS includes all cardioembolic strokes —both definite and probable. We employed the Trial of ORG 10172 in Acute Stroke Treatment (TOAST) classification to differentiate stroke subtypes (20) with the aim of controlling selection bias. Diagnostic criteria for ischemic stroke include: (1) sudden onset of focal neurological deficits, (2) duration of ≥24 h or imaging evidence confirming ischemic injury to brain tissue, and (3) exclusion of cerebral hemorrhage through CT/MRI. All patients were followed up until the endpoint event occurred or December 31, 2023, whichever came first. The median follow-up time was 31 months (interquartile range, IQR 20–43 months).
The baseline characteristics were presented as the mean ± SD or interquartile ranges (IQR) for continuous variables, and categorical variables were reported as counts and percentages. Where appropriate, comparisons were made according to the Mann- Whitney U or Kruskal Wallis test. For categorical variables, differences were assessed using the χ2 or Fisher's exact test. Cox regression analyses were used to establish the relationship between the independent variables and stroke. Cox multivariate regression analyses were performed, adjusting for a pre-specified list of covariates identified based on significant indicators from the Cox univariate regression analyses. The results were presented as hazard ratios (HR) with 95% confidence intervals (CI). Given the gender differences in TG and SUA between the stroke and non-stroke groups (as shown in Table 1 and Figure 2), a restricted cubic spline analysis was conducted to quantitatively assess the threshold values for TG or SUA related to the occurrence of stroke events. The HR of 1 serves as the reference point; an HR greater than 1 indicates a higher risk of stroke, while an HR less than 1 suggests a lower risk. Kaplan–Meier curves were plotted to show the time to stroke based on SUA and TG levels among both stroke and non-stroke patients, with comparisons made using the log-rank test. To assess the discriminatory power for stroke incidence over 31 months, we evaluated several models: the CHA2DS2-VASc model, CHA2DS2-VASc+SUA model, CHA2DS2-VASc+SUA+TG model, and the CHA2DS2-VASc+SUA+TG+LDL model. The evaluation was assessed using receiver operating characteristics (ROC), C-index, NRI, and IDI. We employed calibration curves to evaluate the performance of the final model (CHA2DS2-VASc plus SUA, TG, and LDL).
Figure 2. Serum uric acid (A), TG (B) and age (C) in patients with or without stroke. The correlation of SUA and TG in total population (D), males (E) and females (F).
Furthermore, a decision curve analysis was performed to assess the clinical benefit of the final model. A two-sided P-value <0.05 was considered statistically significant. The statistical analyses were performed using SPSS software (version 26, IBM) and R software (version 4.4.2).
Between January 2020 and December 2023, a total of 3,176 patients were enrolled in the study. Among them, 231 patients had stroke attacks. Table 1 presents the baseline characteristics of the study population. Variables that revealed gender differences between stroke and non-stroke patients were age (66 vs. 65 years for males, 70 vs. 68 years for females), hypertension (34% vs. 37% for females, 45% vs. 41% for males), diabetes (39% vs. 38% for males, 49% vs. 34% for females), previous TIA/stroke (17% vs. 8% for males, 27% vs. 9% for females), TG levels (1.39 mmol/L vs. 1.28 mmol/L for males, 1.5 mmol/L vs. 1.28 mmol/L for females), and SUA levels (424 µmol/L vs. 397 µmol/L for males, 361 µmol/L vs. 328 µmol/L for females). The proportion of males using anti-platelet drugs, diuretics, and hypoglycemic agents was higher in the non-stroke group compared to the stroke group (all P < 0.05). Male stroke patients typically exhibited lower levels of HDL and reduced ejection fraction (EF). Strokes were more frequently observed in individuals with coronary artery disease (CAD, P = 0.002), as well as in those with larger measurements of left atrial diameter (LAD) and left ventricle diameter (LVD). In both men and women, there were no significant differences between stroke and non-stroke groups concerning smoking, alcohol use, persistent AF, heart failure (HF), CKD, chronic obstructive pulmonary disease (COPD), previous pulmonary embolism/deep vein thrombosis (PE/DVT), TC, LDL, QTc, anticoagulants, angiotensin-converting enzyme inhibitors angiotensin-converting enzyme receptor blockers (ACEI/ARB), inhibitors of angiotensin receptor enkephalin peptidase (ARNI), β-blockers, calcium channel blockers (CCB), and ablation treatments.
Given the observed differences in SUA, TG levels, and age in stroke events among males and females, we further analysed their impact on stroke occurrence. As illustrated in Figure 2, both SUA and TG levels, along with age, were significantly elevated in stroke patients compared to those without stroke (all P < 0.05). Importantly, irrespective of stroke status, men displayed higher SUA levels than women (Figure 2A). Additionally, we found that SUA and TG levels were significantly and positively correlated in the overall population, as well as within both male and female subgroups [R = 0.178, P < 0.001; R = 0.172, P < 0.001; and R = 0.236, P < 0.001, respectively (see Figures 2D–F)]. This suggests a potential mutual enhancement effect between these two metabolic biomarkers.
To evaluate the risk factors influencing stroke in NAF patients, we conducted a Cox regression analysis. In the univariate analysis, age, hypertension, DM, CAD, previous TIA stroke, LDL, TG, SUA, LAD, anti-plate drugs, CCBs, and diuretics were positively associated with an increased risk of stroke (Table 2). After adjusting for confounding variables, multivariable analysis revealed that age, previous TIA stroke, LDL, and SUA were independent predictors [with adjusted hazard ratio (HR) and confidence interval (CI) of 1.028 (1.011–1.044), 2.325 (1.674–3.23), 1.258 (1.058–1.495), 1.002 (1.001–1.003)] of stroke. This indicates that LDL could be regarded as a significant marker influencing stroke events in patients with NAF.
Table 2. Risk factors for stroke according to the Cox proportional hazards regression model in total population.
To determine the risk thresholds for increased stroke events associated with SUA and TG levels, we conducted a quantitative analysis employing restricted cubic spline curves for both SUA and TG (see Figure 3). The SUA and TG values corresponding to an HR of 1 were used as thresholds to distinguish between low-risk and high-risk groups. Specifically, the cut-off values were established at 400 µmol/L for SUA in males and 330 µmol/L in females, while for TG, the thresholds were set at 1.28 mmol/L for males and 1.29 mmol/L for females. To assess the effectiveness of these threshold values for risk stratification, we plotted Kaplan–Meier curves. The analysis revealed that individuals with elevated TG levels faced a significantly increased cumulative hazard of stroke events (Figure 4A, HR = 1.509, P = 0.002). Similar results were observed for SUA levels (Figure 4B, HR = 1.439, P = 0.0061). Stroke risk varied according to SUA and TG levels, as depicted in Figure 5. Based on the low- and high-risk categories defined by the spline curves for SUA and TG, we classified all patients into four groups: Group 1 included patients with low levels of both SUA and TG (blue); Group 2 consisted patients with high SUA and low TG levels (red); Group 3 comprised patients with high TG and low SUA levels (green); and Group 4 encompassed those with high levels of both TG and SUA (orange). Notably, patients in Group 4 exhibited a higher incidence of stroke (log-rank test, P = 0.0021). The HRs for patients in Groups 2, 3, and 4 were 1.435, 1.514, and 1.98, respectively. In summary, these findings indicate that the cut-off values effectively distinguish between lower-risk and higher-risk groups, with the combined effect of SUA and TG demonstrating an even more pronounced impact.
Figure 3. Multivariable adjusted hazard ratios for stroke incidence according to levels of SUA and TG on a continuous scale. Red lines are multivariable-adjusted hazard ratios, with pink areas showing 95% CIs derived from restricted cubic spline regressions with three knots at the 25th, 50th, and 75th percentiles. The dashed grey lines indicate reference lines for no association at a hazard ratio of 1.0. Analyses were adjusted for age, previous TIA stroke, SUA, and TG.
Figure 4. Kaplan–Meier curves showing the incidence of stroke for different levels of SUA and TG. (A) A Kaplan–Meier survival curves for TG, the cut-off points for TG 1.28 mmol/L in males and 1.29 mmol/L in females, respectively. (B) Kaplan–Meier survival curves for SUA; the cut points for SUA were 400 µmol/L in males and 330 µmol/L in females, respectively.
Figure 5. Kaplan–Meier curve showing the incidence of stroke. All patients were divided into 4 categories. Group 1: patients with both low-level SUA and TG (blue); Group 2: patients with high-level SUA and low-level TG (red); Group 3: patients with high-level TG and low-level SUA (green); Group 4: patients with high levels of both TG and SUA (orange).
Considering the variations in biomarkers SUA, TG, and LDL between stroke and non-stroke patients, we have developed new stroke assessment models, referred to as model 2: CHA2DS2-VASc+SUA, model 3: CHA2DS2-VASc+SUA+TG, model 4: CHA2DS2-VASc+SUA+TG+LDL. To evaluate the diagnostic utility of these models compared to CHA2DS2-VASc (model 1), we performed ROC analysis (Figure 6A). The area under the curve (AUC) for the models were as follows: model 1: 0.664 (95% CI: 0.628–0.664), model 2: 0.678 (95% CI: 0.644–0.678), model 3: 0.687 (95% CI: 0.653–0.687), and model 4: 0.689 (95% CI: 0.655–0.689). Notably, the clinical diagnostic utility of model 4 is more pronounced (P = 0.042, Table 3).
Figure 6. Model-comparison results of predicting the 31-month incidence of IS. (A) Receiver operating characteristics (ROC) curves of freedom from stroke at 31 months for the different models. (B) Calibration curves for the final model (CHA2DS2-VASc+SUA+TG+LDL). The dotted line represents the entire cohort and the solid line is the result after bias correction by bootstrapping (1,000 repetitions), indicating model performance (boot mean absolute error = 0.007).
To assess the accuracy and reliability of the predictive model 4 by C-index, IDI, and NRI. Model 4 achieved the highest C-index (0.689), indicating greater predictive capability. Model 4 improved classification ability by approximately 6.48% compared to model 1, including SUA, TG, and LDL, contributing to this enhanced predictive capability (P = 0.02). The IDI analysis further demonstrated that model 4 improved predictive ability by 1.1% compared to model 1 (P < 0.001). These findings suggest that model 4 significantly enhances predictive ability and offers more accurate stroke risk predictions. Furthermore, calibration curves illustrated a strong concordance between predicted probabilities and the observed incidence of stroke events (Figure 6B). The decision curves for model 1 and model 4 indicate that the net benefit of model 4 surpasses the threshold probabilities ranging from 0.0 to 0.26 (Figure 7).
Figure 7. Decision curve analyses (DCA) of the CHA2DS2-VASc model and final model for 31 months of stroke incidence. The x-axis indicates the threshold incidence for stroke at 31 months, and the y-axis indicates the net benefit. The horizontal dark grey line assumes no patients will experience the event; the light grey line assumes all patients will experience the event. The final model had enhanced net benefit compared with the CHA2DS2-VASc model at risk threshold >2.6%.
In this study, the incidence of ischemic stroke was 7.3%. Additionally, SUA, LDL, age, and previous TIA stroke were independently associated with IS in patients with NAF. Notably, a linear correlation was observed between TG and SUA. By integrating SUA, TG, and LDL with the CH2DS2-VASc score, the ability to identify stroke risk in NAF patients was significantly improved.
The relationship between SUA levels and AF reveals a fascinating interplay of pathophysiological mechanisms. Elevated SUA in the bloodstream, a condition known as hyperuricemia, is more than just a metabolic concern—it can lead to endothelial dysfunction, a critical precursor to atherosclerosis, often recognized as a significant risk factor for AF. But that's not all; uric acid also contributes to oxidative stress by generating free radicals, which can inflict damage on myocardial tissue. This disruption in heart function may pave the way for arrhythmias. Moreover, the inflammatory response is a major player in AF development, and uric acid is a known activator of inflammation (Figure 8). By triggering the NLRP3 inflammasome, it promotes the release of pro-inflammatory cytokines, like interleukin-1β (IL-1β), which can lead to structural changes in the atria and further exacerbate AF (21).
Figure 8. SUA, TG, and LDL promote and maintain AF through inflammation while acting directly on intracranial vessels, leading to IS.
Recent clinical studies underscore the urgency of this issue, demonstrating that individuals with elevated SUA levels face a significantly higher risk of developing AF compared to those within normal ranges. Notably, a large prospective cohort study indicated that hyperuricemia correlates with an increased incidence of AF and its related complications, hinting that elevated uric acid may act as a valuable prognostic marker (22). Elevated levels of SUA are believed to induce oxidative stress and inflammatory responses, which can lead to endothelial dysfunction and increase the risk of atherosclerosis, thereby heightening the likelihood of stroke (23, 24). Uric acid may directly damage endothelial cells, promoting thrombosis (25). Additionally, the uric acid to creatinine ratio has emerged as a noteworthy predictor for the recurrence of AF post-ablation (26).
In our study, we found that stroke patients exhibited elevated levels of SUA and TG, regardless of gender. The specific thresholds identified for increased stroke risk differ between men and women, indicating distinct pathophysiological mechanisms and underscoring the need for tailored approaches in clinical assessment and management. These findings are consistent with prior studies that suggest these patients tend to be older (23, 27–30). Our study revealed higher critical SUA thresholds for men (400 µmol/L) compared to women (330 µmol/L), which may be attributed to hormonal differences. Estrogen may enhance uric acid excretion in women, allowing men to accumulate more SUA before experiencing an increased stroke risk, thereby highlighting men's greater vulnerability to hyperuricemic conditions at elevated SUA levels.
Regarding triglycerides, the cutoff level shows only minor gender differences: 1.28 mmol/L for men and 1.29 mmol/L for women. This slight variation may relate to lipid metabolism changes influenced by sex hormones, smoking, and unhealthy lifestyles, which affect triglyceride synthesis and clearance, subsequently altering the lipid profile and stroke risk factors by gender. These findings emphasize the importance of exploring how gender differences in SUA and TG impact stroke risk stratification. Acknowledging these gender-specific physiological and hormonal dynamics is essential in developing individualized risk profiles. Estrogen not only facilitates uric acid excretion but also influences lipid metabolism, leading to distinctive cardiovascular risk patterns in women compared to men. Thus, a universal approach may be inadequate in stroke risk assessment. Incorporating gender-specific thresholds and risk factors into existing models could result in more precise and effective clinical strategies.
Cumulative risk analysis involving SUA and TG in the NAF population reveals an intriguing insight: elevated levels of these biomarkers not only raise stroke risk when considered individually, but when both are simultaneously elevated, the risk amplifies dramatically. This echoes the findings from earlier studies that explored the roles of SUA and homocysteine in detecting SCAF (10). In the past, a comprehensive nationwide cohort study further supported that the likelihood of clinical events increases progressively with the rise of TC and TG levels, with stroke risk comprising a striking 10% of these occurrences (14) (Figure 8). Elevated levels of TG can lead to increased plasma viscosity and hemodynamic changes, thereby enhancing the risk of thrombosis (27, 28). Additionally, TG metabolites may promote inflammatory responses in endothelial cells, exacerbating atherosclerosis (31–33). Adding another layer to this narrative, research examining the triglyceride-glucose index unveils interesting gender disparities in TG levels (34). Thus, incorporating SUA and TG into our risk assessment model seems logical and valuable. However, we recognize that further clinical studies are essential to better understand the relationship between TG and AF.
A retrospective cohort study sheds light on the connection between metabolic syndrome and atrial remodeling (35). Notably, LDL plays a pivotal role among the components of metabolic syndrome, significantly contributing to both atherosclerosis and its correlation with AF (36, 37). A prospective cohort study strengthens this association by demonstrating that higher LDL levels are linked to an increased risk of AF recurrence post-catheter ablation (38). Consistent with these observations, our findings indicate that elevated LDL levels correlate independently with heightened stroke risk in patients with NAF, even after controlling for potential confounders, yielding a hazard ratio of 1.25 (95% CI 1.058–1.495, P = 0.009). The established significance of LDL in cardiovascular risk cannot be overstated; prior research suggests that lowering LDL levels by just 1 mmol/L with statins can reduce ischemic stroke risk by 20% (39, 40). LDL is often oxidized, which promotes the formation of atherosclerosis. Oxidized LDL triggers inflammatory and immune responses (41), leading to endothelial dysfunction and potentially causing instability in atherosclerotic plaques (42, 43), which may increase the risk of stroke in NAF patients (44, 45). The above evidence underscores the importance of managing cholesterol levels in stroke prevention strategies. However, it is essential to adopt a balanced approach to cholesterol management, as excessively low cholesterol levels in the bloodstream may increase the permeability of vascular walls (46).
Management of AF primarily involves anticoagulation therapy to prevent stroke. Moreover, effective heart rate and rhythm control are crucial for comprehensive AF management in addition to anticoagulation. Despite these interventions, predicting individual stroke risk and managing the potential bleeding complications associated with anticoagulation therapy remain challenging. The CHA2DS2-VASc score, widely used for stroke risk stratification, considers variables such as congestive heart failure, hypertension, age, diabetes, prior stroke, vascular disease, and sex category. Since its introduction by the European Society of Cardiology in 2010 for stroke prevention in non-valvular AF, the landscape of patient management has shifted significantly, expanding beyond mere prevention to include the management of associated comorbidities and risk factors like obesity. In the current study, we have integrated SUA and lipid markers (TG and LDL) with the CHA2DS2-VASc model to refine stroke risk prediction further. This integration aims to enhance predictive accuracy and improve patient-specific management strategies.
SUA has emerged as the second most prevalent metabolic disorder, yet its management—alongside lipid levels—often receives insufficient attention in patients with AF. When it comes to preventing AF, maintaining a BMI within the healthy range of 20–25 kg/m2 is strongly recommended (47). In the past, research has underscored the influence of SUA and lipid markers in promoting atrial fibrosis through inflammation, which not only supports the persistence of AF but also increases the risk of stroke [ ]. Our study highlights the importance of addressing both SUA and lipid levels. Promisingly, our findings demonstrate that the CHA2DS2VASc+SUA+TG+LDL model significantly improves predictive capabilities regarding stroke risk. This enhanced model exhibits superior performance metrics—such as AUC, C-index, NRI, and IDI—compared to the traditional CHA2DS2-VASc model. In essence, integrating SUA and lipid markers into our predictive model not only elevates its efficacy but also advances our efforts toward more accurate stroke risk assessments.
Firstly, the study's design as a single-center retrospective analysis inherently limits its generalizability. By concentrating on a specific population from a single medical facility, the findings may not be applicable to broader and more diverse populations. Additionally, the relatively small sample size further constrains the statistical power of the study. With a limited number of participants, there is a heightened risk of statistical anomalies, which could lead to findings that are skewed or not representative of the larger population. Moreover, our study does not delve into the mechanisms by which SUA and lipid markers, particularly TG and low-density lipoprotein LDL, may contribute to IS. Therefore, further research is imperative to examine the lower threshold levels of these markers and their direct mechanisms of action concerning the vascular damage associated with IS.
In summary, our findings revealed a notable correlation between SUA, TG, and LDL levels and the incidence of IS events in patients with NAF. Interestingly, SUA and LDL emerged as key independent risk factors for IS in this patient group. By combining SUA, TG, and LDL with the CHA2DS2-VASc score, we found a significant boost in the accuracy of stroke predictions for those with NAF. This enhanced model could support clinicians in making more informed decisions.
The raw data supporting the conclusions of this article will be made available by the authors, without undue reservation.
The studies involving humans were approved by Ethics Committee of the First Affiliated Hospital of Dalian Medical University. The studies were conducted in accordance with the local legislation and institutional requirements. The participants provided their written informed consent to participate in this study. Written informed consent was obtained from the individual(s) for the publication of any potentially identifiable images or data included in this article.
YT: Data curation, Formal analysis, Methodology, Writing – original draft, Writing – review & editing. BS: Data curation, Investigation, Methodology, Writing – original draft. TH: Writing – review & editing, Conceptualization, Supervision. YY: Supervision, Validation, Visualization, Writing – review & editing. FL: Investigation, Supervision, Validation, Writing – review & editing. JL: Writing – review & editing, Methodology, Supervision. CL: Writing – review & editing, Investigation, Validation. YW: Writing – review & editing, Software, Data curation. ZY: Writing – review & editing, Software. YC: Writing – review & editing, Supervision. XY: Supervision, Writing – review & editing, Funding acquisition, Project administration, Resources, Visualization. YX: Supervision, Writing – review & editing, Funding acquisition, Project administration, Resources, Visualization.
The author(s) declare that financial support was received for the research and/or publication of this article. This work was supported by research grants from the Dalian Science Fund for Distinguished Young Scholars (2022RJ13), DMU-1&DICP UN202201, National Natural Science Foundation of China (82070427), National Key Research and Development Program of China (2022YFC2405002).
We acknowledge Yidu Cloud (Beijing) Technology Ltd. for their cooperation in data searching, extraction, and processing.
The authors declare that the research was conducted in the absence of any commercial or financial relationships that could be construed as a potential conflict of interest.
The author(s) declare that no Generative AI was used in the creation of this manuscript.
All claims expressed in this article are solely those of the authors and do not necessarily represent those of their affiliated organizations, or those of the publisher, the editors and the reviewers. Any product that may be evaluated in this article, or claim that may be made by its manufacturer, is not guaranteed or endorsed by the publisher.
1. Shi S, Tang Y, Zhao Q, Yan H, Yu B, Zheng Q, et al. Prevalence and risk of atrial fibrillation in China: a national cross-sectional epidemiological study. Lancet Reg Health West Pac. (2022) 11(23):100439. doi: 10.1016/j.lanwpc.2022.100439
2. Go AS, Hylek EM, Phillips KA, Chang Y, Henault LE, Selby JV, et al. Prevalence of diagnosed atrial fibrillation in adults: national implications for rhythm management and stroke prevention: the anticoagulation and risk factors in atrial fibrillation (atria) study. JAMA. (2001) 285(18):2370–5. doi: 10.1001/jama.285.18.2370
3. Lip GY, Lane DA. Stroke prevention in atrial fibrillation: a systematic review. JAMA. (2015) 313(19):1950–62. doi: 10.1001/jama.2015.4369
4. Virani SS, Alonso A, Aparicio HJ, Benjamin EJ, Bittencourt MS, Callaway CW, et al. Heart disease and stroke statistics-2021 update: a report from the American Heart Association. Circulation. (2021) 143(8):e254–743. doi: 10.1161/CIR.0000000000000950
5. Piccini JP, Hammill BG, Sinner MF, Jensen PN, Hernandez AF, Heckbert SR, et al. Incidence and prevalence of atrial fibrillation and associated mortality among medicare beneficiaries, 1993–2007. Circ Cardiovasc Qual Outcomes. (2012) 5(1):85–93. doi: 10.1161/CIRCOUTCOMES.111.962688
6. Björck S, Palaszewski B, Friberg L, Bergfeldt L. Atrial fibrillation, stroke risk, and warfarin therapy revisited: a population-based study. Stroke. (2013) 44(11):3103–8. doi: 10.1161/STROKEAHA.113.002329
7. Expert Panel on Detection E, Treatment of High Blood Cholesterol in A. Executive summary of the third report of the national cholesterol education program (NCEP) expert panel on detection, evaluation, and treatment of high blood cholesterol in adults (adult treatment panel III). JAMA. (2001) 285(19):2486–97. doi: 10.1001/jama.285.19.2486
8. Grundy SM, Cleeman JI, Daniels SR, Donato KA, Eckel RH, Franklin BA, et al. Diagnosis and management of the metabolic syndrome: an American Heart Association/national heart, lung, and blood institute scientific statement. Circulation. (2005) 112(17):2735–52. doi: 10.1161/CIRCULATIONAHA.105.169404
9. Han BA-O, Wang N, Chen Y, Li Q, Zhu C, Chen Y, et al. Prevalence of hyperuricaemia in an eastern Chinese population: a cross-sectional study. BMJ Open. (2020) 10(5):e035614. doi: 10.1136/bmjopen-2019-035614
10. Wang SA-OX, Wei Y, Hidru TH, Li D, Wang N, Yang Y, et al. Combined effect of homocysteine and uric acid to identify patients with high risk for subclinical atrial fibrillation. J Am Heart Assoc. (2022) 11(1):e021997. doi: 10.1161/JAHA.121.021997
11. Oseto HA-O, Yamashita SA-O, Tokuda MA-O, Sato H, Shiomi SA-O, Sakurai R, et al. The association between hyperuricemia and atrial fibrillation recurrence after catheter ablation. J Arrhythm. (2024) 40(3):520–6. doi: 10.1002/joa3.13030
12. Duncan MS, Vasan RS, Xanthakis V. Trajectories of blood lipid concentrations over the adult life course and risk of cardiovascular disease and all-cause mortality: observations from the Framingham study over 35 years. J Am Heart Assoc. (2019) 8(11):e011433. doi: 10.1161/JAHA.118.011433
13. Sun L, Clarke RA-O, Bennett D, Guo Y, Walters RA-O, Hill M, et al. Causal associations of blood lipids with risk of ischemic stroke and intracerebral hemorrhage in Chinese adults. Nat Med. (2019) 25(4):569–74. doi: 10.1038/s41591-019-0366-x
14. Lee H, Park JB, Hwang IC, Yoon YE, Park HE, Choi SY, et al. Association of four lipid components with mortality, myocardial infarction, and stroke in statin-naïve young adults: a nationwide cohort study. Eur J Prev Cardiol. (2020) 27(8):870–81. doi: 10.1177/2047487319898571
15. Rivard LA-O, Friberg LA-O, Conen DA-O, Healey JA-O, Berge TA-O, Boriani GA-O, et al. Atrial fibrillation and dementia: a report from the af-screen international collaboration. Circulation. (2022) 145(5):392–409. doi: 10.1161/CIRCULATIONAHA.121.055018
16. Fossier L, Panel M, Butruille L, Colombani S, Azria L, Woitrain E, et al. Enhanced mitochondrial calcium uptake suppresses atrial fibrillation associated with metabolic syndrome. J Am Coll Cardiol. (2022) 80(23):2205–19. doi: 10.1016/j.jacc.2022.09.041
17. Joint committee for Guideline Revision. 2016 Chinese guidelines for the management of dyslipidemia in adults. J Geriatr Cardiol. (2018) 15(1):1–29. doi: 10.11909/j.issn.1671-5411.2018.01.011
18. Zeng L, Ma P, Li Z, Liang S, Wu C, Hong C, et al. Multimodal machine learning-based marker enables early detection and prognosis prediction for hyperuricemia. Adv Sci (Weinh). (2024) 11(34):e2404047. doi: 10.1002/advs.202404047
19. Li Z, Pan L, Deng Y, Liu Q, Hidru TH, Liu F, et al. Development and validation of a nomogram for estimation of left atrial thrombus or spontaneous Echo contrast risk in non-valvular atrial fibrillation patients with low to borderline cha(2)Ds(2)-vasc score. Int J Gen Med. (2022) 15:7329–39. doi: 10.2147/IJGM.S384519
20. Adams HP Jr., Bendixen BH, Kappelle LJ, Biller J, Love BB, Gordon DL, et al. Classification of subtype of acute ischemic stroke. Definitions for use in a multicenter clinical trial. Toast. Trial of org 10172 in acute stroke treatment. Stroke. (1993) 24(1):35–41. doi: 10.1161/01.str.24.1.35
21. Albu A, Para I, Porojan M. Uric acid and arterial stiffness. Ther Clin Risk Manag. (2020) 16:39–54. doi: 10.2147/TCRM.S232033
22. Tamariz L, Harzand A, Palacio A, Verma S, Jones J, Hare J. Uric acid as a predictor of all-cause mortality in heart failure: a meta-analysis. Congest Heart Fail. (2011) 17(1):25–30. doi: 10.1111/j.1751-7133.2011.00200.x
23. Li X, Meng X, Timofeeva M, Tzoulaki I, Tsilidis KK, Ioannidis JP, et al. Serum uric acid levels and multiple health outcomes: umbrella review of evidence from observational studies, randomised controlled trials, and Mendelian randomisation studies. Br Med J. (2017) 357:j2376. doi: 10.1136/bmj.j2376
24. Kuwabara M, Niwa K, Hisatome I, Nakagawa T, Roncal-Jimenez CA, Andres-Hernando A, et al. Asymptomatic hyperuricemia without comorbidities predicts cardiometabolic diseases: five-year Japanese cohort study. Hypertension. (2017) 69(6):1036–44. doi: 10.1161/HYPERTENSIONAHA.116.08998
25. Falsetti L, Capeci W, Tarquinio N, Viticchi G, Silvestrini M, Catozzo V, et al. Serum uric acid, kidney function and acute ischemic stroke outcomes in elderly patients: a single-cohort, perspective study. Neurol Int. (2017) 9(1):6920. doi: 10.4081/ni.2017.6920
26. Zhang Y, Wang Y, Yang X, Li Z, Shang L, Hou Y. Serum uric acid: creatinine ratio (UCR) is associated with recurrence of atrial fibrillation after catheter ablation. Front Endocrinol (Lausanne). (2023) 14:1110102. doi: 10.3389/fendo.2023.1110102
27. Esan O, Wierzbicki AS. Triglycerides and cardiovascular disease. Curr Opin Cardiol. (2021) 36(4):469–77. doi: 10.1097/HCO.0000000000000862
28. Miller M, Stone NJ, Ballantyne C, Bittner V, Criqui MH, Ginsberg HN, et al. Triglycerides and cardiovascular disease: a scientific statement from the American Heart Association. Circulation. (2011) 123(20):2292–333. doi: 10.1161/CIR.0b013e3182160726
29. Peters SAE, Carcel C, Millett ERC, Woodward M. Sex differences in the association between major risk factors and the risk of stroke in the UK biobank cohort study. Neurology. (2020) 95(20):e2715–26. doi: 10.1212/WNL.0000000000010982
30. Appelman Y, van Rijn BB, Ten Haaf ME, Boersma E, Peters SA. Sex differences in cardiovascular risk factors and disease prevention. Atherosclerosis. (2015) 241(1):211–8. doi: 10.1016/j.atherosclerosis.2015.01.027
31. Nordestgaard BG. Triglyceride-rich lipoproteins and atherosclerotic cardiovascular disease: new insights from epidemiology, genetics, and biology. Circ Res. (2016) 118(4):547–63. doi: 10.1161/CIRCRESAHA.115.306249
32. Ginsberg HN, Packard CJ, Chapman MJ, Borén J, Aguilar-Salinas CA, Averna M, et al. Triglyceride-rich lipoproteins and their remnants: metabolic insights, role in atherosclerotic cardiovascular disease, and emerging therapeutic strategies-a consensus statement from the European atherosclerosis society. Eur Heart J. (2021) 42(47):4791–806. doi: 10.1093/eurheartj/ehab551
33. Borén J, Chapman MJ, Krauss RM, Packard CJ, Bentzon JF, Binder CJ, et al. Low-density lipoproteins cause atherosclerotic cardiovascular disease: pathophysiological, genetic, and therapeutic insights: a consensus statement from the European atherosclerosis society consensus panel. Eur Heart J. (2020) 41(24):2313–30. doi: 10.1093/eurheartj/ehz962
34. Liu X, Abudukeremu A, Jiang Y, Cao Z, Wu M, Ma J, et al. U-shaped association between the triglyceride-glucose index and atrial fibrillation incidence in a general population without known cardiovascular disease. Cardiovasc Diabetol. (2023) 22(1):118. doi: 10.1186/s12933-023-01777-9
35. Wali R, Wang X, Li C, Yang H, Liu F, Sama SD, et al. Early atrial remodeling predicts the risk of cardiovascular events in patients with metabolic syndrome: a retrospective cohort study. Front Cardiovasc Med. (2023) 10:1162886. doi: 10.3389/fcvm.2023.1162886
36. Alloubani A, Nimer R, Samara R. Relationship between hyperlipidemia, cardiovascular disease and stroke: a systematic review. Curr Cardiol Rev. (2021) 17(6):e051121189015. doi: 10.2174/1573403X16999201210200342
37. Danese MA-O, Sidelnikov E, Kutikova L. The prevalence, low-density lipoprotein cholesterol levels, and treatment of patients at very high risk of cardiovascular events in the United Kingdom: a cross-sectional study. Curr Med Res Opin. (2018) 34(8):1441–7. doi: 10.1080/03007995.2018.1463211
38. Zou H, Huang Q, Huang Q, Hu B, He W, Xia Z, et al. L-shaped association of plasma low-density lipoprotein cholesterol with atrial fibrillation recurrence after catheter ablation: a prospective cohort study. Sci Rep. (2024) 14(1):28434. doi: 10.1038/s41598-024-79836-8
39. Collins R, Reith C, Emberson J, Armitage J, Baigent C, Blackwell L, et al. Interpretation of the evidence for the efficacy and safety of statin therapy. Lancet. (2016) 388(10059):2532–61. doi: 10.1016/S0140-6736(16)31357-5
40. Fulcher J, O'Connell R, Voysey M, Emberson J, Blackwell L, Mihaylova B, et al. Efficacy and safety of ldl-lowering therapy among men and women: meta-analysis of individual data from 174,000 participants in 27 randomised trials. Lancet. (2015) 385(9976):1397–405. doi: 10.1016/S0140-6736(14)61368-4
41. Bäck M, Yurdagul A Jr., Tabas I, Öörni K, Kovanen PT. Inflammation and its resolution in atherosclerosis: mediators and therapeutic opportunities. Nat Rev Cardiol. (2019) 16(7):389–406. doi: 10.1038/s41569-019-0169-2
42. Taleb S, Tedgui A, Mallat Z. Il-17 and Th17 cells in atherosclerosis: subtle and contextual roles. Arterioscler Thromb Vasc Biol. (2015) 35(2):258–64. doi: 10.1161/ATVBAHA.114.303567
43. Gimbrone MA Jr., García-Cardeña G. Endothelial cell dysfunction and the pathobiology of atherosclerosis. Circ Res. (2016) 118(4):620–36. doi: 10.1161/CIRCRESAHA.115.306301
44. Kita T, Kume N, Minami M, Hayashida K, Murayama T, Sano H, et al. Role of oxidized LDL in atherosclerosis. Ann N Y Acad Sci. (2001) 947:199–205; discussion -6. doi: 10.1111/j.1749-6632.2001.tb03941.x
45. Libby P, Ridker PM, Hansson GK, Leducq Transatlantic Network on A. Inflammation in atherosclerosis: from pathophysiology to practice. J Am Coll Cardiol. (2009) 54(23):2129–38. doi: 10.1016/j.jacc.2009.09.009
46. Iso H, Jacobs DR Jr., Wentworth D, Neaton JD, Cohen JD. Serum cholesterol levels and six-year mortality from stroke in 350,977 men screened for the multiple risk factor intervention trial. N Engl J Med. (1989) 320(14):904–10. doi: 10.1056/NEJM198904063201405
47. Van Gelder IA-O, Rienstra MA-OX, Bunting KA-O, Casado-Arroyo RA-O, Caso VA-O, Crijns HA-O, et al. 2024 ESC guidelines for the management of atrial fibrillation developed in collaboration with the European association for cardio-thoracic surgery (EACTS). Eur Heart J. (2024) 45(36):3314–414. doi: 10.1093/eurheartj/ehae176
Keywords: non-valvular atrial fibrillation, stroke, serum uric acid, triglycerides, low-density lipoprotein
Citation: Tang Y, Song B, Hidru TH, Yang Y, Liu F, Li J, Li C, Wen Y, Yang Z, Chen Y, Yang X and Xia Y (2025) Combined predictive value of uric acid and serum lipid for stroke events in non-valvular atrial fibrillation patients. Front. Cardiovasc. Med. 12:1569904. doi: 10.3389/fcvm.2025.1569904
Received: 2 February 2025; Accepted: 4 March 2025;
Published: 26 March 2025.
Edited by:
Yan Zhang, Peking University, ChinaReviewed by:
Jianqing She, The First Affiliated Hospital of Xi'an Jiaotong University, ChinaCopyright: © 2025 Tang, Song, Hidru, Yang, Liu, Li, Li, Wen, Yang, Chen, Yang and Xia. This is an open-access article distributed under the terms of the Creative Commons Attribution License (CC BY). The use, distribution or reproduction in other forums is permitted, provided the original author(s) and the copyright owner(s) are credited and that the original publication in this journal is cited, in accordance with accepted academic practice. No use, distribution or reproduction is permitted which does not comply with these terms.
*Correspondence: Xiaolei Yang, eWFuZ3hsMTAxMkB5ZWFoLm5ldA==; Yunlong Xia, eXVubG9uZ194aWFAMTI2LmNvbQ==
†These authors have contributed equally to this work and share first authorship
Disclaimer: All claims expressed in this article are solely those of the authors and do not necessarily represent those of their affiliated organizations, or those of the publisher, the editors and the reviewers. Any product that may be evaluated in this article or claim that may be made by its manufacturer is not guaranteed or endorsed by the publisher.
Research integrity at Frontiers
Learn more about the work of our research integrity team to safeguard the quality of each article we publish.