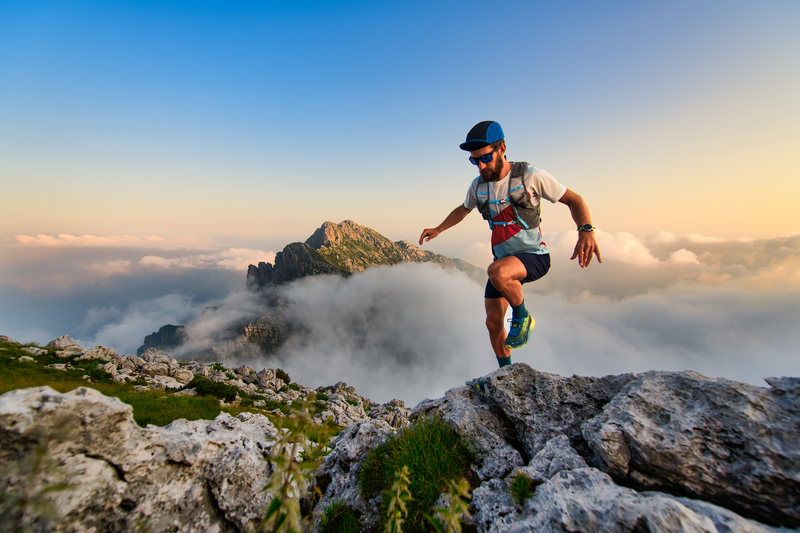
94% of researchers rate our articles as excellent or good
Learn more about the work of our research integrity team to safeguard the quality of each article we publish.
Find out more
BRIEF RESEARCH REPORT article
Front. Cardiovasc. Med.
Sec. Lipids in Cardiovascular Disease
Volume 12 - 2025 | doi: 10.3389/fcvm.2025.1546351
The final, formatted version of the article will be published soon.
You have multiple emails registered with Frontiers:
Please enter your email address:
If you already have an account, please login
You don't have a Frontiers account ? You can register here
Introduction: Lipoprotein(a) [Lp(a)] has been recognized as key factor in cardiovascular research. This study aimed to identify key patient profiles based on the characteristics of a Portuguese cohort of adults who were referred for Lp(a) measurement.Method: An unsupervised clustering analysis was performed on 661 Portuguese adults to identify patient profiles associated with lipoprotein a [Lp(a)] based on a range of demographic and clinical indicators. Lp(a) levels were deliberately excluded from the algorithm, to ensure an unbiased cluster formation. Results: The analysis revealed two distinct clusters based on Lp(a) levels. Cluster 1 (n=336) exhibited significantly higher median Lp(a) levels than Cluster 2 (n=325; p=0.004), with 46.4% of individuals exceeding the 75 nmol/L (30 mg/dL) risk threshold (p<0.001). This group was characterized by older age (median 57 vs. 45 years), lower body mass index (27.17 vs. 29.40), and a majority male composition (73.8% vs. 26.5%). Additionally, Cluster 1 displayed a higher prevalence of hypertension (56.5% vs. 31.1%), diabetes mellitus (38.7% vs. 17.2%), and dyslipidemia (88.7% vs. 55.4%). These data suggest that the Cluster 1 profile has a potential increased risk for cardiovascular complications and underscore the importance of considering specific patient profiles for Lp(a) screening and cardiovascular risk assessment. Conclusion: Despite the study limitations, including single-institution data and potential selection bias, this study highlights the utility of cluster analysis in identifying clinically meaningful patient profiles and suggests that proactive screening and management of Lp(a) levels, particularly in patients with characteristics resembling those of Cluster 1, may be beneficial.
Keywords: Lipoprotein (a) levels, Clustering analysis, Patient Profiling, unsupervised learning, Cardiovascular Risk Assessment
Received: 16 Dec 2024; Accepted: 05 Mar 2025.
Copyright: © 2025 Ferreira, Saraiva, Garcez, Tavares Da Silva, Ferreira, Oliveira and Palma. This is an open-access article distributed under the terms of the Creative Commons Attribution License (CC BY). The use, distribution or reproduction in other forums is permitted, provided the original author(s) or licensor are credited and that the original publication in this journal is cited, in accordance with accepted academic practice. No use, distribution or reproduction is permitted which does not comply with these terms.
* Correspondence:
Ana Ferreira, W4Research, Lisbon, Portugal
Miguel Saraiva, Hospital de Santo António, Porto, Portugal
Disclaimer: All claims expressed in this article are solely those of the authors and do not necessarily represent those of their affiliated organizations, or those of the publisher, the editors and the reviewers. Any product that may be evaluated in this article or claim that may be made by its manufacturer is not guaranteed or endorsed by the publisher.
Research integrity at Frontiers
Learn more about the work of our research integrity team to safeguard the quality of each article we publish.