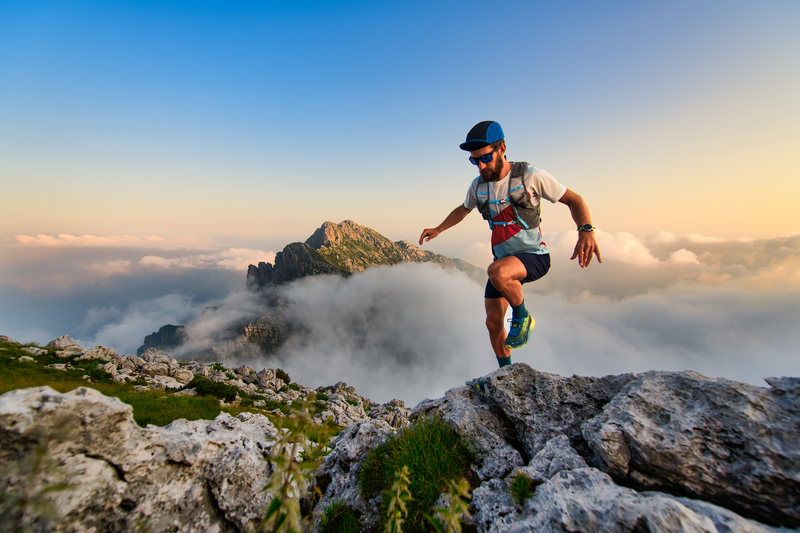
94% of researchers rate our articles as excellent or good
Learn more about the work of our research integrity team to safeguard the quality of each article we publish.
Find out more
ORIGINAL RESEARCH article
Front. Cardiovasc. Med.
Sec. Heart Valve Disease
Volume 12 - 2025 | doi: 10.3389/fcvm.2025.1546169
The final, formatted version of the article will be published soon.
You have multiple emails registered with Frontiers:
Please enter your email address:
If you already have an account, please login
You don't have a Frontiers account ? You can register here
The study aimed to determine the best prediction method for red blood cell (RBC) transfusion during or after mitral valve surgery by utilizing the feature selection method to determine preoperative variables that can forecast the risk of RBC transfusion. This information can aid in managing RBC transfusions during or after mitral valve surgery.: 1477 patients underwent mitral valve surgery at eight large tertiary hospitals in China, all receiving cardiopulmonary bypass treatment. Thirty preoperative factors were collected, including demographic characteristics, medical history, physical signs, laboratory indicators, and surgery type. Key variables were screened via The Max-Relevance and Min-Redundancy (mRMR) method. Various machine learning models were developed to sort and analyze patient data concerning mitral valve surgery. Every model was evaluated using five-fold crossvalidation. The SHapley Additive exPlanations (SHAP) was utilized to interpret the models with the best predictions.Results: Out of the 1,477 patients included in the study, 862 patients who underwent mitral valve surgery received RBC transfusions during or after mitral valve surgery.The mRMR method was used to screen ten preoperative variables from a pool of thirty for subsequent modeling analysis. Among all models, LightGBM exhibited superior performance (AUC of 0.935 in the training set and 0.734 in the validation set) and performed well in the prospective dataset with an accuracy of 74.2%. SHAP analysis visualized the ten most influential variables for outcome prediction, ranked as follows: hematocrit, red blood cells (RBCs), weight, body mass index (BMI), fibrinogen, hemoglobin, height, age, left ventricular dilation, and sex.This study evaluated preoperative variables related to RBC transfusion and determined that LightGBM was the best model for RBC transfusion during or after mitral valve surgery. The model demonstrated high accuracy in predicting RBC transfusion likelihood from clinical datasets. This facilitates clinicians in anticipating the need for RBC transfusion before the patient's surgery and assists them in making decisions about blood management.
Keywords: Mitral Valve, machine learning, Lightgbm, red blood cell transfusion, Shapley additive explanations
Received: 16 Dec 2024; Accepted: 17 Feb 2025.
Copyright: © 2025 Wang, Liu, Fan, Wang, Zhang, Ma, Huang, Wang, Chen and Gui. This is an open-access article distributed under the terms of the Creative Commons Attribution License (CC BY). The use, distribution or reproduction in other forums is permitted, provided the original author(s) or licensor are credited and that the original publication in this journal is cited, in accordance with accepted academic practice. No use, distribution or reproduction is permitted which does not comply with these terms.
* Correspondence:
Rong Gui, Department of Transfusion, Third Xiangya Hospital, Central South University, Changsha, China
Disclaimer: All claims expressed in this article are solely those of the authors and do not necessarily represent those of their affiliated organizations, or those of the publisher, the editors and the reviewers. Any product that may be evaluated in this article or claim that may be made by its manufacturer is not guaranteed or endorsed by the publisher.
Research integrity at Frontiers
Learn more about the work of our research integrity team to safeguard the quality of each article we publish.