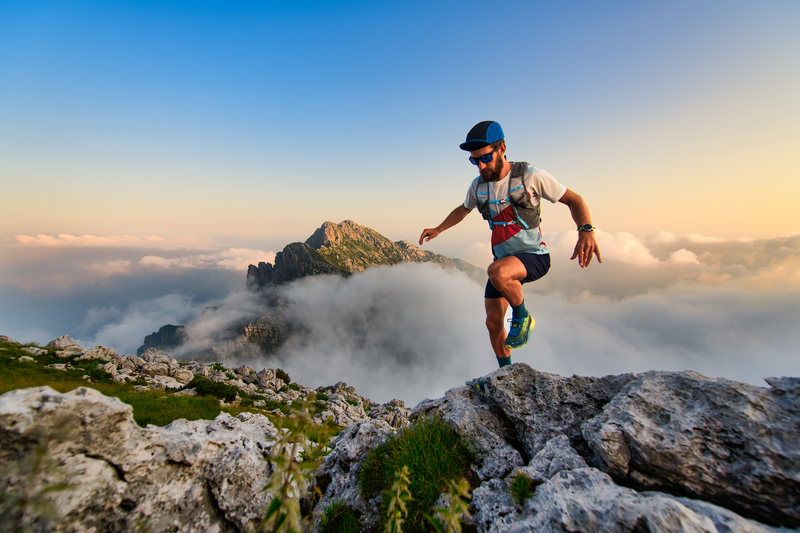
94% of researchers rate our articles as excellent or good
Learn more about the work of our research integrity team to safeguard the quality of each article we publish.
Find out more
ORIGINAL RESEARCH article
Front. Cardiovasc. Med.
Sec. General Cardiovascular Medicine
Volume 12 - 2025 | doi: 10.3389/fcvm.2025.1541762
This article is part of the Research Topic Immune Cell Dynamics and Biomarkers in Cardiac Surgery-Induced Systemic Inflammatory Response View all 4 articles
The final, formatted version of the article will be published soon.
You have multiple emails registered with Frontiers:
Please enter your email address:
If you already have an account, please login
You don't have a Frontiers account ? You can register here
Objective: This study aimed to explore the relationship between the systemic immune-inflammatory index (SII) and the probability of in-hospital mortality among acute ischemic stroke (AIS) with atrial fibrillation (AF) patients undergoing intravenous thrombolysis.Methods: This single-center, retrospective observational study included individuals among AIS with AF who received intravenous thrombolysis. The SII is determined by taking the product of the platelet and neutrophil counts, followed by dividing this result by the lymphocyte count. In-hospital mortality was defined as a Modified Rankin Scale (mRS) score of 6 point. The investigation applied logistic regression models, along with subgroup, sensitivity, and receiver operating characteristic (ROC) curve analyses assessments, to explore the relationship between the SII and in-hospital mortality.Results: 541 patients were included in this study, 50 (9.24%) of whom died during their hospital stay.Multifactorial logistic regression analyses using fully adjusted models, demonstrated that the SII is independently associated with the risk of in-hospital death. patients with elevated SII levels experienced a significantly increased risk of in-hospital mortality, which was found to be 2.557 (95% CI: 1.154-5.665, P = 0.021) times greater compared to those with lower SII levels. Through multivariate logistic regression analyses, a notable correlation between the SII and the probability of death during hospitalization was observed across various subgroups, including individuals aged ≤ 75 and > 75years, women, patients with persistent AF, those receiving thrombolytic therapy, diabetic and nondiabetic patients, individuals with BMI ≥ 24 kg/m 2 , and those with an admission National Institutes of Health Stroke Scale score ≤ 20 (P < 0.05). Two sensitivity analyses confirmed the robustness of this association from multiple perspectives (P < 0.05). ROC analysis demonstrated that the SII, the baseline model, and their combined model all showed strong predictive power for in-hospital mortality. Notably, the combined model outperformed the SII alone (P < 0.05). In addition, the predictive value of SII for in-hospital death was significantly higher than that of neutrophil-to-lymphocyte ratio (NLR) and platelet-to-lymphocyte ratio (PLR).A significant association has been observed between the risk of in-hospital death among AIS with AF individual undergoing intravenous thrombolysis and the SII.
Keywords: systemic immune-inflammation index, Acute ischemic stroke, Atrial Fibrillation, intravenous thrombolysis, In-hospital mortality
Received: 08 Dec 2024; Accepted: 18 Mar 2025.
Copyright: © 2025 Aierken, Ma, Zhu, Jin, Zhu, Zhou, Gao, Zhao, Wang and Li. This is an open-access article distributed under the terms of the Creative Commons Attribution License (CC BY). The use, distribution or reproduction in other forums is permitted, provided the original author(s) or licensor are credited and that the original publication in this journal is cited, in accordance with accepted academic practice. No use, distribution or reproduction is permitted which does not comply with these terms.
* Correspondence:
Hongling Zhao, Department of Neurology, Dalian Municipal Central Hospital, Dalian, China
Tao Wang, Department of Cardiology, Dalian municipal Central Hospital, Dalian, China
Shijun Li, Department of Cardiology, Dalian municipal Central Hospital, China Medical University, Shengyang, China
Disclaimer: All claims expressed in this article are solely those of the authors and do not necessarily represent those of their affiliated organizations, or those of the publisher, the editors and the reviewers. Any product that may be evaluated in this article or claim that may be made by its manufacturer is not guaranteed or endorsed by the publisher.
Research integrity at Frontiers
Learn more about the work of our research integrity team to safeguard the quality of each article we publish.