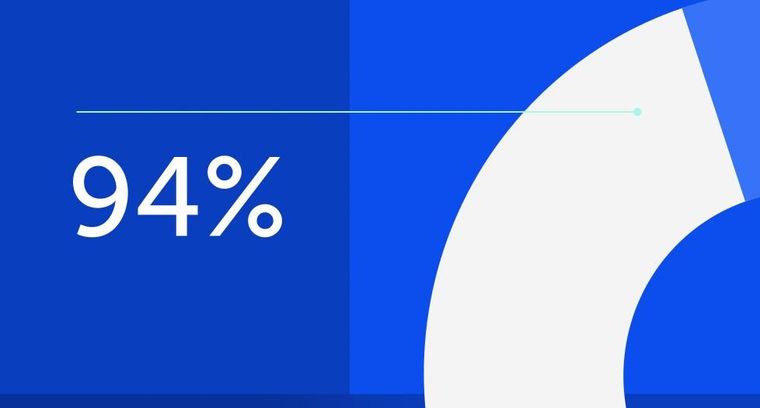
94% of researchers rate our articles as excellent or good
Learn more about the work of our research integrity team to safeguard the quality of each article we publish.
Find out more
ORIGINAL RESEARCH article
Front. Cardiovasc. Med.
Sec. Cardiovascular Pharmacology and Drug Discovery
Volume 12 - 2025 | doi: 10.3389/fcvm.2025.1526247
The final, formatted version of the article will be published soon.
Select one of your emails
You have multiple emails registered with Frontiers:
Notify me on publication
Please enter your email address:
If you already have an account, please login
You don't have a Frontiers account ? You can register here
Background: Cardiovascular diseases are diverse, intersecting, and characterized by multistage complexity. The growing demand for personalized diagnosis and treatment poses significant challenges to clinical diagnosis and pharmacotherapy, increasing potential medication risks for doctors and patients. The Cardiovascular Medication Guide (CMG) demonstrates distinct advantages in managing cardiovascular disease, serving as a critical reference for front-line doctors in prescription selection and treatment planning. However, most medical knowledge remains fragmented within written records, such as medical files, without a cohesive organizational structure, leading to an absence of clinical support from visualized expert knowledge systems. Purpose: This study aims to construct a comprehensive Expert Knowledge Graph of Cardiovascular Medication Guidelines (EKG-CMG) by integrating unstructured and semi-structured Cardiovascular Medication Knowledge (CMK), including clinical guidelines and expert consensus, to create a visually integrated cardiovascular expert knowledge system. Methods: This study utilized consensus and guidelines from cardiovascular experts to organize and manage structured knowledge. BERT and knowledge extraction techniques capture drug attribute relationships, leading to the construction of the EKG-CMG with fine-grained information. The Neo4j graph database stores expert knowledge, visualizes knowledge structures and semantic relationships, and supports retrieval, discovery, and reasoning of knowledge about medication. A hierarchical-weighted, multidimensional relational model to mine medication relationships through reverse reasoning. Experts participated in an iterative review process, allowing targeted refinement of expert medication knowledge reasoning.We construct an ontology encompassing 12 cardiovascular "medication types" and their "attributes of medication types". Approximately 15,000 entity-relationships include 22,475 medication entities, 2,027 entity categories, and 3,304 relationships. Taking beta-blockers (β) as an example demonstrates the complete process of ontology to knowledge graph construction and application, encompassing 41 AMTs, 1,197 entity nodes, and 1,351 relationships. The EKG-CMG can complete knowledge retrieval and discovery linked to "one drug for multiple uses," "combination therapy," and "precision medication." Additionally, we analyzed the knowledge reasoning case of cross-symptoms and complex medication for complications.The EKG-CMG systematically organizes CMK, effectively addressing the "knowledge island" issues between diseases and drugs. Knowledge potential relationships have been exposed by leveraging EKG-CMG visualization technology, which can facilitate medication semantic retrieval and the exploration and reasoning of complex knowledge relationships.
Keywords: Cardiovascular Medication Knowledge, Expert Knowledge Graph, Multidimensional relationships, Ontology construction, Knowledge Discover and Reasoning, EKG-CMG
Received: 14 Nov 2024; Accepted: 09 Apr 2025.
Copyright: © 2025 Cui, Zhu, Zhang, Piao and Peng. This is an open-access article distributed under the terms of the Creative Commons Attribution License (CC BY). The use, distribution or reproduction in other forums is permitted, provided the original author(s) or licensor are credited and that the original publication in this journal is cited, in accordance with accepted academic practice. No use, distribution or reproduction is permitted which does not comply with these terms.
* Correspondence:
Hongzhen Cui, School of Computer and Communication Engineering, University of Science and Technology Beijing, Beijing, 100083, Beijing, China
Wei Zhang, Key Laboratory of Computing Power Network and Information Security, Ministry of Education, Shandong Computer Science Center, Qilu University of Technology (Shandong Academy of Sciences), Jinan, China
Meihua Piao, School of Nursing, Chinese Academy of Medical Sciences and Peking Union Medical College, Beijing, 100006, Beijing Municipality, China
Yunfeng Peng, School of Computer and Communication Engineering, University of Science and Technology Beijing, Beijing, 100083, Beijing, China
Disclaimer: All claims expressed in this article are solely those of the authors and do not necessarily represent those of their affiliated organizations, or those of the publisher, the editors and the reviewers. Any product that may be evaluated in this article or claim that may be made by its manufacturer is not guaranteed or endorsed by the publisher.
Supplementary Material
Research integrity at Frontiers
Learn more about the work of our research integrity team to safeguard the quality of each article we publish.