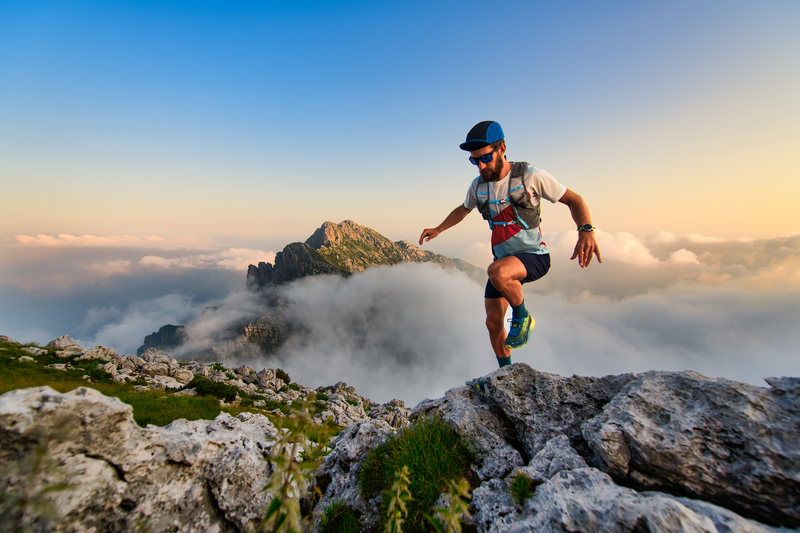
94% of researchers rate our articles as excellent or good
Learn more about the work of our research integrity team to safeguard the quality of each article we publish.
Find out more
ORIGINAL RESEARCH article
Front. Cardiovasc. Med.
Sec. Cardiovascular Epidemiology and Prevention
Volume 12 - 2025 | doi: 10.3389/fcvm.2025.1491643
The final, formatted version of the article will be published soon.
You have multiple emails registered with Frontiers:
Please enter your email address:
If you already have an account, please login
You don't have a Frontiers account ? You can register here
Objective: Advanced glycation end-products (AGEs) may contribute to the pathogenesis of atherosclerotic cardiovascular disease (ASCVD), potentially influencing the development and progression of ASCVD differently at various life stages. The study was performed to elucidate the associations between AGEs and the risk of ASCVD across different age groups.In this cross-sectional study, 1240 subjects were enrolled and divided into three groups (group Ⅰ: 20-39 years old, n=468; group Ⅱ: 40-59 years old, n=471; group Ⅲ: 60-79 years old, n=301). Skin AGEs were measured by skin autofluorescence (SAF). ASCVD risk was assessed by a validated Framingham Risk Score calculator. Other proven ASCVD risk factors were also measured, including glycosylated hemoglobin, uric acid, lipid profile, homocysteine, and Cystatin C.Results: An increasing trend of skin AGEs was observed from group Ⅰ, group Ⅱ to group Ⅲ.Skin AGEs were significantly associated with ASCVD risk in all subjects (OR 1.029, 95% CI 1.003-1.056, P = 0.018), independent of some of the proven cardiovascular risk factors. This association was particularly significant in individuals aged 40 -59 and 60 -79 (OR = 1.047, 95% CI: 1.025 -1.069; OR = 1.022, 95% CI: 1.002 -1.042; both P < 0.05). ROC analysis showed that skin AGEs predicted the diagnosis of medium or high ASCVD risk in pooled group, group Ⅱ and group Ⅲ, respectively.Our study substantiates that skin AGEs play an important role as an independent risk factor for ASCVD, highlighting their significance beyond traditional risk assessment models, particularly in middle-aged and older populations.
Keywords: skin advanced glycation end-products, Framingham Cardiovascular Risk Score, Atherosclerotic cardiovascular disease, Different age groups, skin autofluorescence
Received: 06 Sep 2024; Accepted: 21 Mar 2025.
Copyright: © 2025 Wang, Liang, Zhou, Tang, Ye, Huang, Tang, Jiang and Pan. This is an open-access article distributed under the terms of the Creative Commons Attribution License (CC BY). The use, distribution or reproduction in other forums is permitted, provided the original author(s) or licensor are credited and that the original publication in this journal is cited, in accordance with accepted academic practice. No use, distribution or reproduction is permitted which does not comply with these terms.
* Correspondence:
Boxiong Jiang, Department of VIP Medical Service Center, Third Affiliated Hospital of Sun Yat-sen University, Guangzhou, China
Yunfeng Pan, Department of Rheumatology and Immunology, Third Affiliated Hospital of Sun Yat-sen University, Guangzhou, China
Disclaimer: All claims expressed in this article are solely those of the authors and do not necessarily represent those of their affiliated organizations, or those of the publisher, the editors and the reviewers. Any product that may be evaluated in this article or claim that may be made by its manufacturer is not guaranteed or endorsed by the publisher.
Research integrity at Frontiers
Learn more about the work of our research integrity team to safeguard the quality of each article we publish.